95% of People Writing Prompts Miss These Two Easy Techniques
Summary
TLDRIn this video, Mark, the founder of AI automation agency Prompt Advisors, explains how to optimize AI-generated outputs by adjusting the temperature and top P settings. Temperature affects creativity and structure, while top P influences word choice. Mark recommends focusing on just one of these parameters for better control over results. He demonstrates how these settings impact outputs through examples and a detailed matrix, offering insights for anyone looking to fine-tune their AI prompts. Mark also shares tips for troubleshooting and maximizing the effectiveness of AI tools.
Takeaways
- 🤖 Temperature and top P are two key parameters that control how a language model generates text.
- 🔥 Temperature controls how creative and random the model's responses are. Higher values (close to 1) make responses more creative, while lower values (close to 0) make responses more focused and deterministic.
- 🧠 Top P, or nucleus sampling, limits the token choices to the most likely words based on general patterns, regardless of the specific context of the prompt.
- 🔀 High temperature with high top P results in more creative and unpredictable responses, while low values for both create very rigid, straightforward outputs.
- 🧩 The difference between temperature and top P: Temperature focuses on context-specific tokens, while top P biases towards more frequent, familiar tokens.
- 💡 The recommendation is to adjust either temperature or top P in a prompt but not both, as using both can make it harder to troubleshoot and understand the output.
- 🎯 For simple creativity adjustments, start by tweaking the temperature, and for tighter word control, adjust the top P.
- ⚙️ Experimentation and testing different combinations of temperature and top P are key to mastering their use and optimizing the results for specific tasks.
- 📝 In some cases, using low top P (closer to 0) may be useful, such as legal or highly specific tasks that require stringent word choices.
- 🥼 The video suggests using a methodical approach by testing combinations in controlled prompts to understand the impact of each parameter and improve results for practical applications.
Q & A
What is the main topic of the video?
-The video explains how to use temperature and top P parameters in ChatGPT to tweak and transform outputs without changing the underlying prompt.
What is the primary difference between temperature and top P?
-Temperature controls the creativity and randomness of the output by selecting words relevant to the prompt, whereas top P filters words based on their general probability of being chosen, making the word selection more rigid or open depending on the setting.
Why does the speaker recommend adjusting only one of either temperature or top P at a time?
-The speaker recommends adjusting only one of the two because both parameters have a significant influence on the output. Using both simultaneously makes it harder to troubleshoot and understand which parameter is affecting the result.
How does a high temperature setting affect the output?
-A high temperature setting (close to 1) makes the output more creative, unpredictable, and diverse in terms of word choice and structure.
What happens when the temperature is set to a low value?
-When the temperature is set to a low value (closer to 0), the output becomes more focused, predictable, and rigid, with less creative and diverse word choices.
How does top P influence the selection of words in the output?
-Top P limits the selection of words by favoring tokens that are more commonly used in general, and the lower the top P value, the more rigid and selective the model becomes in choosing words.
In which cases would using a low top P setting be beneficial?
-A low top P setting can be beneficial in scenarios where precise and rigid language is required, such as legal contexts or cases where the wording needs to follow strict guidelines.
What does the speaker mean by 'hallucination' in the context of language models?
-In the context of language models, 'hallucination' refers to when the model generates output that is incorrect or not aligned with the prompt, due to incorrect probabilities behind the scenes.
What is the recommended approach for prompt engineering, according to the speaker?
-The speaker recommends using markdown to structure prompts, choosing either temperature or top P for tweaking the output, and experimenting with one parameter at a time to better control the result.
What is the purpose of the Easter egg mentioned by the speaker?
-The Easter egg refers to a part of the video where the speaker experiments with combining different temperature and top P settings, showing viewers how they can mix and match these parameters to see their effects on output.
Outlines
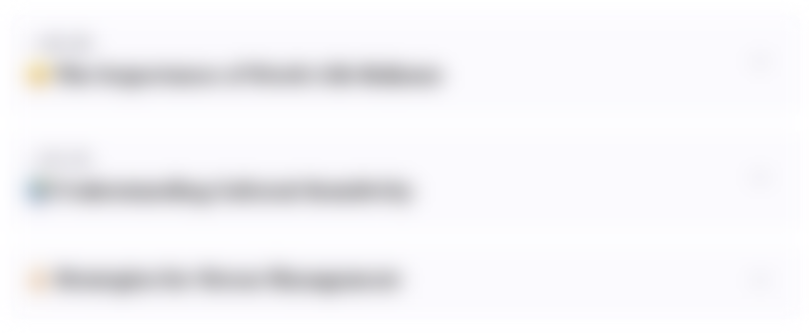
Cette section est réservée aux utilisateurs payants. Améliorez votre compte pour accéder à cette section.
Améliorer maintenantMindmap
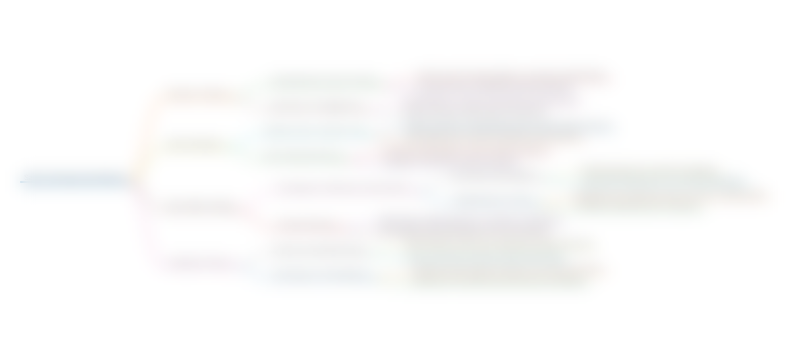
Cette section est réservée aux utilisateurs payants. Améliorez votre compte pour accéder à cette section.
Améliorer maintenantKeywords
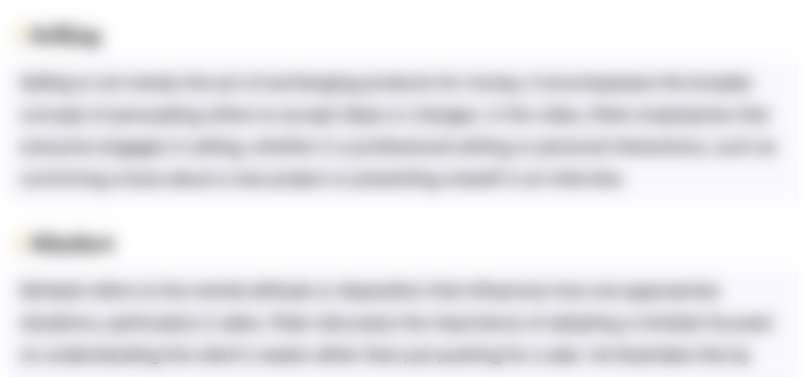
Cette section est réservée aux utilisateurs payants. Améliorez votre compte pour accéder à cette section.
Améliorer maintenantHighlights
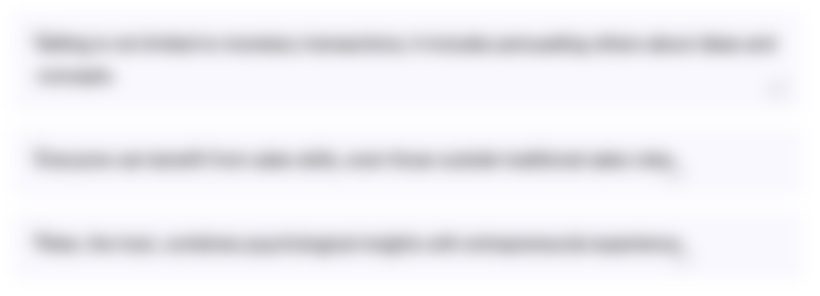
Cette section est réservée aux utilisateurs payants. Améliorez votre compte pour accéder à cette section.
Améliorer maintenantTranscripts
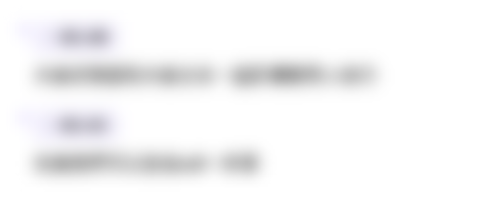
Cette section est réservée aux utilisateurs payants. Améliorez votre compte pour accéder à cette section.
Améliorer maintenantVoir Plus de Vidéos Connexes
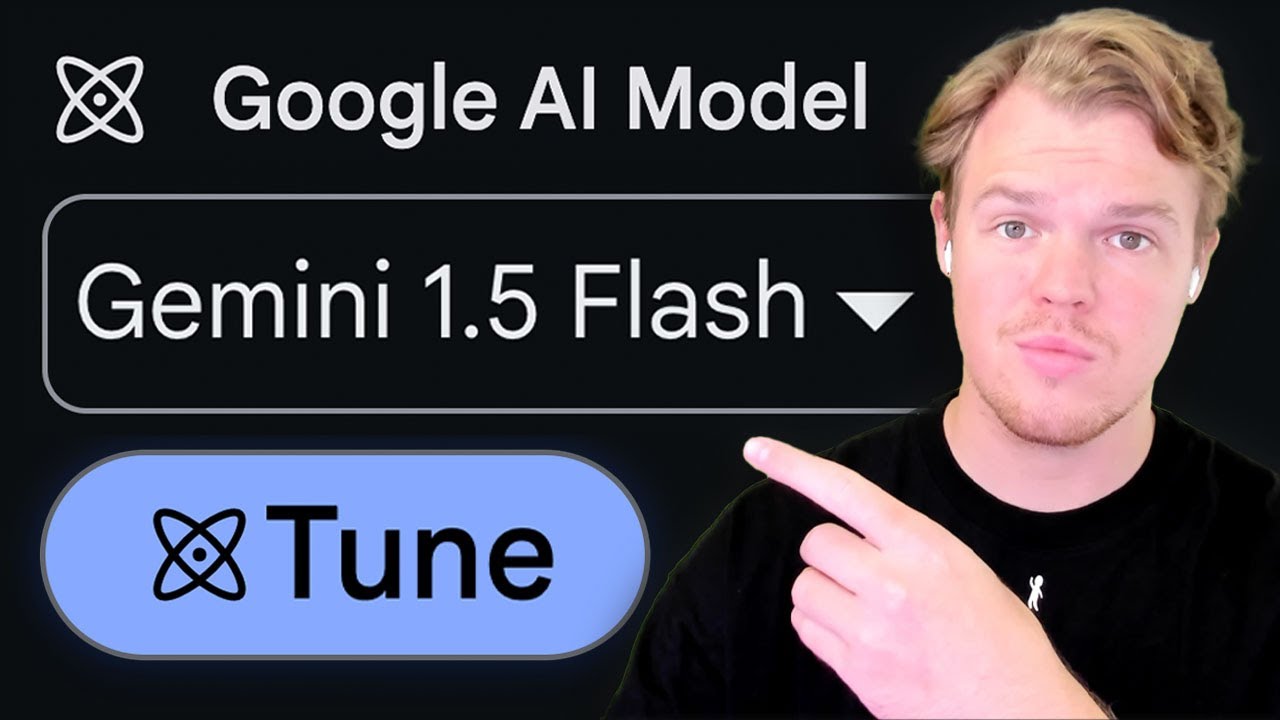
Fine-tuning Gemini with Google AI Studio Tutorial - [Customize a model for your application]

How Prompt Compression Can Make You a Better Prompt Engineer
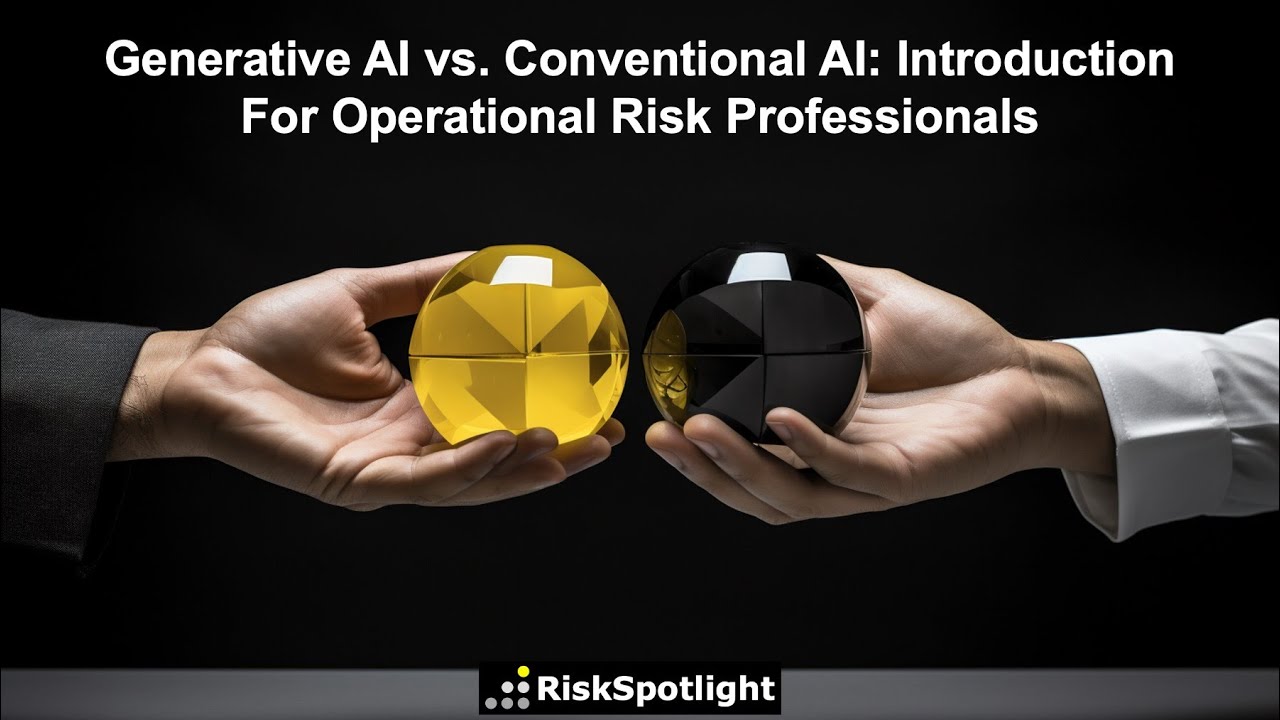
Generative AI vs. Conventional AI: Introduction For Operational Risk Professionals

How to Unlock ANY Framework's Potential with Meta Prompting
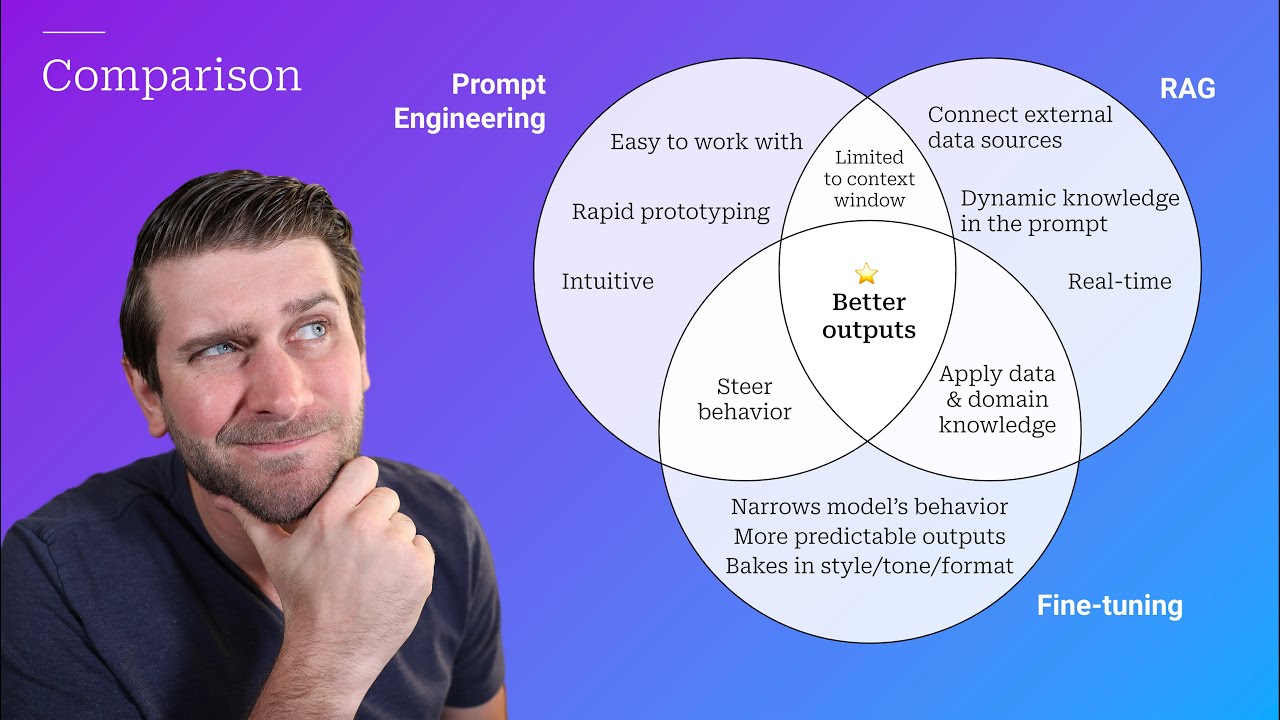
Prompt Engineering, RAG, and Fine-tuning: Benefits and When to Use
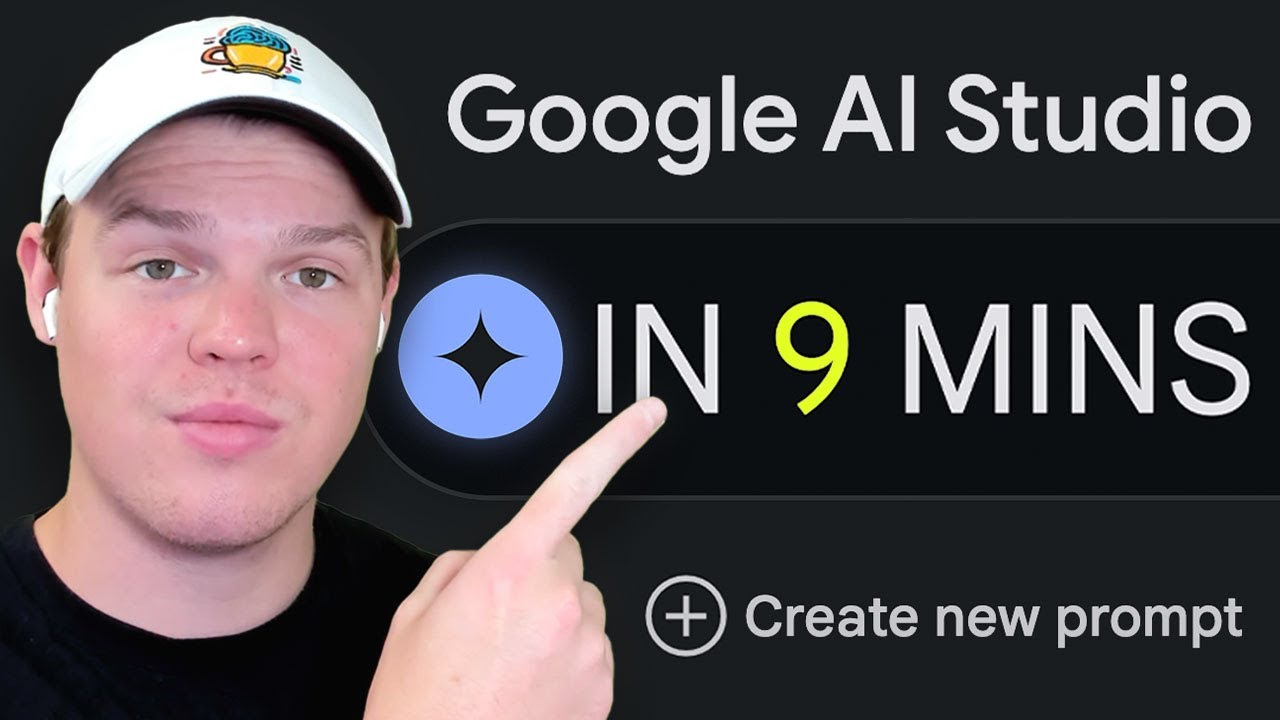
How To Use Google AI Studio For Beginners
5.0 / 5 (0 votes)