How computers are learning to be creative | Blaise Agüera y Arcas
Summary
TLDRThis transcript outlines a presentation on machine intelligence and its connection to human brain functions, particularly in perception and creativity. The speaker delves into the history of neuroscience and machine learning, drawing parallels between neural networks and human cognition. By explaining how computers can now identify images and generate art through machine learning, the speaker highlights the shared mechanisms between perception and creation. The talk touches on the progress in AI, from early experiments to modern neural networks, showing how machines can both recognize and create, much like the human brain.
Takeaways
- 🧠 The speaker leads a team at Google focused on machine intelligence, aiming to make computers capable of tasks similar to brain functions.
- 🔍 Perception and creativity are central to both human intelligence and machine learning; perception turns sensory data into concepts, and creativity turns concepts into tangible outputs.
- 🖼️ The team’s machine perception algorithms allow images to become searchable, as seen in Google Photos.
- 🧑🎨 The speaker emphasizes the connection between perception and creativity, citing Michelangelo's insight that creativity is about discovery through perception.
- 🧬 Santiago Ramón y Cajal’s groundbreaking 19th-century neuroanatomy work is still influential in understanding brain structures and neurons today.
- 🖥️ Computers, modeled after the brain's neural networks, are now capable of recognizing images and even generating new ones using machine learning.
- 🦅 Neural networks can recognize patterns in images and create artistic renditions, as seen in experiments where machines generate images of birds or morphing animals.
- 🔢 Learning in neural networks mimics human learning, where the system iteratively reduces error by adjusting its internal weights based on training data.
- 🎨 The speaker demonstrates how machine-generated images resemble creative works, with neural networks producing surreal, abstract forms based on familiar objects.
- 💻 The talk concludes with the idea that computers, modeled after human brains, are helping us not only extend our intelligence but also gain a deeper understanding of how our minds work.
Q & A
What is the main focus of the team at Google that the speaker leads?
-The team focuses on machine intelligence, specifically making computers and devices able to perform tasks that brains can do, such as perception and creativity.
Why is the team interested in studying real brains and neuroscience?
-The team is interested in understanding how brains outperform computers in certain tasks, like perception, to enhance machine perception and intelligence systems.
What role does perception play in machine intelligence, according to the speaker?
-Perception is the process by which sensory input, like sounds and images, is converted into concepts in the mind. In machine intelligence, it enables systems like Google Photos to recognize and make images searchable.
How does the speaker connect perception with creativity?
-The speaker suggests that perception and creativity are closely linked because creating often involves perceiving, as exemplified by Michelangelo’s idea that a sculptor discovers a statue within a block of stone.
Who was Santiago Ramón y Cajal, and what was his contribution to neuroscience?
-Santiago Ramón y Cajal was a 19th-century Spanish neuroanatomist who pioneered the study of brain cells (neurons) using microscopy and specialized staining techniques, leading to detailed drawings of neurons.
How did early computer scientists model the brain in their research?
-Early computer scientists like Warren McCulloch and Walter Pitts modeled the brain’s visual cortex as a circuit diagram, viewing it as a series of computational elements that process information similarly to how computers work.
What challenge does the speaker highlight when it comes to machine perception?
-The challenge for machine perception is to take an image and accurately classify it, such as recognizing a bird in a picture. This task, which is simple for the human brain, was once nearly impossible for computers.
What is the significance of 'x,' 'w,' and 'y' in the neural network model described by the speaker?
-'x' represents the input pixels, 'w' the synaptic weights in the neural network, and 'y' the output, such as the classification of an object (e.g., 'bird'). These variables form the core of the neural network's computation.
How does the neural network ‘learn’ according to the speaker?
-The neural network learns by minimizing error through iterative adjustments of the weights ('w'). This process mimics how humans learn by refining their understanding through trial and error based on feedback.
What does the speaker mean by solving for 'x' in the context of machine creativity?
-Solving for 'x' means using a trained neural network to generate images based on its learned weights ('w') and a known concept ('y'). This process allows the machine to create visual outputs, like a picture of a bird, by reconstructing what it has learned about that concept.
Outlines
🤖 Machine Perception and Creativity
The speaker leads a team at Google working on machine intelligence, focusing on making computers capable of brain-like tasks. They emphasize that while computers have become proficient in perception, such as turning images into searchable content, creativity—transforming concepts into reality—remains a significant area of interest. Machine perception has evolved to the point where it unexpectedly connects to creativity, giving rise to machine art. The speaker references Michelangelo's quote about sculpture to illustrate how perception and creativity are interconnected. Furthermore, they highlight that studying brains and understanding perception enhances machine learning and vice versa, marking a significant crossover between neuroscience and artificial intelligence.
💡 The Historical Evolution of Brain Study
The speaker delves into the history of neuroscience, beginning with early brain anatomists and their superficial understanding of the brain's structure. The breakthrough came with Santiago Ramón y Cajal, a 19th-century neuroanatomist who used staining techniques to visualize individual brain cells. His detailed drawings of neurons were groundbreaking and still hold significance today. The speaker emphasizes the similarities between neurons and electrical wiring, noting how Ramón y Cajal's work laid the foundation for modern neuroscience, where scientists continue to explore and map the brain using advanced techniques like serial electron microscopy.
👀 Visual Processing and Neural Networks
The speaker transitions to discussing how brains and computers process visual information. They reference early computer scientists, like Alan Turing and Warren McCulloch, who saw brain structures as akin to circuit diagrams. By explaining the basic task of perception—recognizing a bird in an image—the speaker introduces neural networks, both biological and artificial, which handle complex layers of information to arrive at an output. They describe how modern neural networks can now perform tasks previously impossible for computers, such as identifying birds and even species from images. The discussion illustrates how perception works in both human and machine learning models.
🧠 Learning and Error Minimization in Neural Networks
The speaker dives into how machines learn, particularly through a process of error minimization. They explain that while it's relatively easy to solve for known variables in a simple equation, the challenge lies in figuring out the unknowns, such as the weights (w) in neural networks. By using error minimization, machines take guesses, adjust, and iteratively improve their predictions, similar to how humans learn through experience. The speaker illustrates this process with an example of a neural network recognizing birds on a mobile phone, showcasing how modern technology can perform trillions of operations to identify objects in real-time.
🔄 Reverse Engineering Neural Networks for Creativity
The speaker introduces the concept of reverse-engineering neural networks to generate images instead of recognizing them. By solving for the input (x) rather than the output (y), neural networks trained to recognize birds can generate images of birds. The speaker shares examples of neural networks creating art, including an 'Animal Parade' video morphing from one animal to another, highlighting how machine learning can produce creative outcomes. The speaker also demonstrates how adjusting parameters in a neural network can produce new, surreal images, further merging the worlds of machine learning and creativity.
🎨 Machine Art and Perception: Hallucination and Creativity
The speaker discusses how machine learning models can generate images by 'hallucinating' or interpreting random noise or existing images. They describe how neural networks, when left to iterate over images like clouds, begin to perceive recognizable objects, similar to how humans see patterns in ambiguous visuals. The speaker highlights experiments in which networks are pushed into a sort of 'free association,' creating continuous loops of generated images. This capability, the speaker notes, demonstrates how machines, like humans, use perception to fuel creativity.
🎭 Expanding Creativity Beyond Visual Art
The speaker concludes by pointing out that machine learning isn't limited to visual art. They mention an experiment where a neural network generates poetry based on images, highlighting a collaboration with artist Ross Goodwin. The poetry, informed by 20th-century works, demonstrates that machines can extend their creative capabilities to other forms of expression. The speaker reiterates Michelangelo's idea that perception and creativity are deeply linked, suggesting that machines, like human brains, can perform both tasks, thus expanding the horizons of artificial intelligence in creative fields.
Mindmap
Keywords
💡Machine Intelligence
💡Perception
💡Creativity
💡Neural Networks
💡Synapses
💡Santiago Ramón y Cajal
💡Error Minimization
💡Inference
💡3D Reconstructions
💡Artificial Neural Networks
Highlights
The team at Google is working on machine intelligence to make computers perform brain-like functions, especially focusing on perception and creativity.
Machine perception is crucial for both human brains and computers, enabling tasks like image recognition in Google Photos.
Creativity, the ability to turn concepts into reality, is closely linked with perception, as Michelangelo’s famous quote on sculpture suggests.
Santiago Ramón y Cajal's groundbreaking 19th-century work on neuroanatomy laid the foundation for modern neuroscience, helping us understand brain cells (neurons).
Modern technologies, like those from the Max Planck Institute, allow us to image tiny brain tissue, advancing our understanding of neurons and their connections.
Neurons use electricity, which led to groundbreaking studies in the mid-20th century on how neurons communicate, forming the basis for artificial neural networks.
Warren McCulloch and Walter Pitts's work on visual cortex laid the groundwork for understanding how neurons process images in a computational manner.
The brain's process of image recognition is mirrored in artificial neural networks, where pixel data (input) is processed layer by layer to identify objects (output).
Training neural networks to recognize images involves solving for the unknown variables (synapse weights), similar to how humans learn through trial and error.
Artificial neural networks can now generate images based on trained data, such as creating bird images by solving for input variables in the network.
‘Animal Parade’ is an example of machine creativity, generating morphing images of animals using neural networks trained to recognize them.
Neural networks can generate surreal images of faces by reconstructing them based on trained data, highlighting the challenges of ambiguity in perception.
Machines can perceive and create; generating images or concepts from neural networks shows the link between recognition and creation, a process similar to human imagination.
Computer models of perception and creativity suggest that these processes aren't unique to humans, as computers are computational models of the mind.
The development of computing, initially inspired by the human brain, is now fulfilling the early visions of pioneers like Alan Turing and John von Neumann by extending human cognitive abilities.
Transcripts
So, I lead a team at Google that works on machine intelligence;
in other words, the engineering discipline of making computers and devices
able to do some of the things that brains do.
And this makes us interested in real brains
and neuroscience as well,
and especially interested in the things that our brains do
that are still far superior to the performance of computers.
Historically, one of those areas has been perception,
the process by which things out there in the world --
sounds and images --
can turn into concepts in the mind.
This is essential for our own brains,
and it's also pretty useful on a computer.
The machine perception algorithms, for example, that our team makes,
are what enable your pictures on Google Photos to become searchable,
based on what's in them.
The flip side of perception is creativity:
turning a concept into something out there into the world.
So over the past year, our work on machine perception
has also unexpectedly connected with the world of machine creativity
and machine art.
I think Michelangelo had a penetrating insight
into to this dual relationship between perception and creativity.
This is a famous quote of his:
"Every block of stone has a statue inside of it,
and the job of the sculptor is to discover it."
So I think that what Michelangelo was getting at
is that we create by perceiving,
and that perception itself is an act of imagination
and is the stuff of creativity.
The organ that does all the thinking and perceiving and imagining,
of course, is the brain.
And I'd like to begin with a brief bit of history
about what we know about brains.
Because unlike, say, the heart or the intestines,
you really can't say very much about a brain by just looking at it,
at least with the naked eye.
The early anatomists who looked at brains
gave the superficial structures of this thing all kinds of fanciful names,
like hippocampus, meaning "little shrimp."
But of course that sort of thing doesn't tell us very much
about what's actually going on inside.
The first person who, I think, really developed some kind of insight
into what was going on in the brain
was the great Spanish neuroanatomist, Santiago Ramón y Cajal,
in the 19th century,
who used microscopy and special stains
that could selectively fill in or render in very high contrast
the individual cells in the brain,
in order to start to understand their morphologies.
And these are the kinds of drawings that he made of neurons
in the 19th century.
This is from a bird brain.
And you see this incredible variety of different sorts of cells,
even the cellular theory itself was quite new at this point.
And these structures,
these cells that have these arborizations,
these branches that can go very, very long distances --
this was very novel at the time.
They're reminiscent, of course, of wires.
That might have been obvious to some people in the 19th century;
the revolutions of wiring and electricity were just getting underway.
But in many ways,
these microanatomical drawings of Ramón y Cajal's, like this one,
they're still in some ways unsurpassed.
We're still more than a century later,
trying to finish the job that Ramón y Cajal started.
These are raw data from our collaborators
at the Max Planck Institute of Neuroscience.
And what our collaborators have done
is to image little pieces of brain tissue.
The entire sample here is about one cubic millimeter in size,
and I'm showing you a very, very small piece of it here.
That bar on the left is about one micron.
The structures you see are mitochondria
that are the size of bacteria.
And these are consecutive slices
through this very, very tiny block of tissue.
Just for comparison's sake,
the diameter of an average strand of hair is about 100 microns.
So we're looking at something much, much smaller
than a single strand of hair.
And from these kinds of serial electron microscopy slices,
one can start to make reconstructions in 3D of neurons that look like these.
So these are sort of in the same style as Ramón y Cajal.
Only a few neurons lit up,
because otherwise we wouldn't be able to see anything here.
It would be so crowded,
so full of structure,
of wiring all connecting one neuron to another.
So Ramón y Cajal was a little bit ahead of his time,
and progress on understanding the brain
proceeded slowly over the next few decades.
But we knew that neurons used electricity,
and by World War II, our technology was advanced enough
to start doing real electrical experiments on live neurons
to better understand how they worked.
This was the very same time when computers were being invented,
very much based on the idea of modeling the brain --
of "intelligent machinery," as Alan Turing called it,
one of the fathers of computer science.
Warren McCulloch and Walter Pitts looked at Ramón y Cajal's drawing
of visual cortex,
which I'm showing here.
This is the cortex that processes imagery that comes from the eye.
And for them, this looked like a circuit diagram.
So there are a lot of details in McCulloch and Pitts's circuit diagram
that are not quite right.
But this basic idea
that visual cortex works like a series of computational elements
that pass information one to the next in a cascade,
is essentially correct.
Let's talk for a moment
about what a model for processing visual information would need to do.
The basic task of perception
is to take an image like this one and say,
"That's a bird,"
which is a very simple thing for us to do with our brains.
But you should all understand that for a computer,
this was pretty much impossible just a few years ago.
The classical computing paradigm
is not one in which this task is easy to do.
So what's going on between the pixels,
between the image of the bird and the word "bird,"
is essentially a set of neurons connected to each other
in a neural network,
as I'm diagramming here.
This neural network could be biological, inside our visual cortices,
or, nowadays, we start to have the capability
to model such neural networks on the computer.
And I'll show you what that actually looks like.
So the pixels you can think about as a first layer of neurons,
and that's, in fact, how it works in the eye --
that's the neurons in the retina.
And those feed forward
into one layer after another layer, after another layer of neurons,
all connected by synapses of different weights.
The behavior of this network
is characterized by the strengths of all of those synapses.
Those characterize the computational properties of this network.
And at the end of the day,
you have a neuron or a small group of neurons
that light up, saying, "bird."
Now I'm going to represent those three things --
the input pixels and the synapses in the neural network,
and bird, the output --
by three variables: x, w and y.
There are maybe a million or so x's --
a million pixels in that image.
There are billions or trillions of w's,
which represent the weights of all these synapses in the neural network.
And there's a very small number of y's,
of outputs that that network has.
"Bird" is only four letters, right?
So let's pretend that this is just a simple formula,
x "x" w = y.
I'm putting the times in scare quotes
because what's really going on there, of course,
is a very complicated series of mathematical operations.
That's one equation.
There are three variables.
And we all know that if you have one equation,
you can solve one variable by knowing the other two things.
So the problem of inference,
that is, figuring out that the picture of a bird is a bird,
is this one:
it's where y is the unknown and w and x are known.
You know the neural network, you know the pixels.
As you can see, that's actually a relatively straightforward problem.
You multiply two times three and you're done.
I'll show you an artificial neural network
that we've built recently, doing exactly that.
This is running in real time on a mobile phone,
and that's, of course, amazing in its own right,
that mobile phones can do so many billions and trillions of operations
per second.
What you're looking at is a phone
looking at one after another picture of a bird,
and actually not only saying, "Yes, it's a bird,"
but identifying the species of bird with a network of this sort.
So in that picture,
the x and the w are known, and the y is the unknown.
I'm glossing over the very difficult part, of course,
which is how on earth do we figure out the w,
the brain that can do such a thing?
How would we ever learn such a model?
So this process of learning, of solving for w,
if we were doing this with the simple equation
in which we think about these as numbers,
we know exactly how to do that: 6 = 2 x w,
well, we divide by two and we're done.
The problem is with this operator.
So, division --
we've used division because it's the inverse to multiplication,
but as I've just said,
the multiplication is a bit of a lie here.
This is a very, very complicated, very non-linear operation;
it has no inverse.
So we have to figure out a way to solve the equation
without a division operator.
And the way to do that is fairly straightforward.
You just say, let's play a little algebra trick,
and move the six over to the right-hand side of the equation.
Now, we're still using multiplication.
And that zero -- let's think about it as an error.
In other words, if we've solved for w the right way,
then the error will be zero.
And if we haven't gotten it quite right,
the error will be greater than zero.
So now we can just take guesses to minimize the error,
and that's the sort of thing computers are very good at.
So you've taken an initial guess:
what if w = 0?
Well, then the error is 6.
What if w = 1? The error is 4.
And then the computer can sort of play Marco Polo,
and drive down the error close to zero.
As it does that, it's getting successive approximations to w.
Typically, it never quite gets there, but after about a dozen steps,
we're up to w = 2.999, which is close enough.
And this is the learning process.
So remember that what's been going on here
is that we've been taking a lot of known x's and known y's
and solving for the w in the middle through an iterative process.
It's exactly the same way that we do our own learning.
We have many, many images as babies
and we get told, "This is a bird; this is not a bird."
And over time, through iteration,
we solve for w, we solve for those neural connections.
So now, we've held x and w fixed to solve for y;
that's everyday, fast perception.
We figure out how we can solve for w,
that's learning, which is a lot harder,
because we need to do error minimization,
using a lot of training examples.
And about a year ago, Alex Mordvintsev, on our team,
decided to experiment with what happens if we try solving for x,
given a known w and a known y.
In other words,
you know that it's a bird,
and you already have your neural network that you've trained on birds,
but what is the picture of a bird?
It turns out that by using exactly the same error-minimization procedure,
one can do that with the network trained to recognize birds,
and the result turns out to be ...
a picture of birds.
So this is a picture of birds generated entirely by a neural network
that was trained to recognize birds,
just by solving for x rather than solving for y,
and doing that iteratively.
Here's another fun example.
This was a work made by Mike Tyka in our group,
which he calls "Animal Parade."
It reminds me a little bit of William Kentridge's artworks,
in which he makes sketches, rubs them out,
makes sketches, rubs them out,
and creates a movie this way.
In this case,
what Mike is doing is varying y over the space of different animals,
in a network designed to recognize and distinguish
different animals from each other.
And you get this strange, Escher-like morph from one animal to another.
Here he and Alex together have tried reducing
the y's to a space of only two dimensions,
thereby making a map out of the space of all things
recognized by this network.
Doing this kind of synthesis
or generation of imagery over that entire surface,
varying y over the surface, you make a kind of map --
a visual map of all the things the network knows how to recognize.
The animals are all here; "armadillo" is right in that spot.
You can do this with other kinds of networks as well.
This is a network designed to recognize faces,
to distinguish one face from another.
And here, we're putting in a y that says, "me,"
my own face parameters.
And when this thing solves for x,
it generates this rather crazy,
kind of cubist, surreal, psychedelic picture of me
from multiple points of view at once.
The reason it looks like multiple points of view at once
is because that network is designed to get rid of the ambiguity
of a face being in one pose or another pose,
being looked at with one kind of lighting, another kind of lighting.
So when you do this sort of reconstruction,
if you don't use some sort of guide image
or guide statistics,
then you'll get a sort of confusion of different points of view,
because it's ambiguous.
This is what happens if Alex uses his own face as a guide image
during that optimization process to reconstruct my own face.
So you can see it's not perfect.
There's still quite a lot of work to do
on how we optimize that optimization process.
But you start to get something more like a coherent face,
rendered using my own face as a guide.
You don't have to start with a blank canvas
or with white noise.
When you're solving for x,
you can begin with an x, that is itself already some other image.
That's what this little demonstration is.
This is a network that is designed to categorize
all sorts of different objects -- man-made structures, animals ...
Here we're starting with just a picture of clouds,
and as we optimize,
basically, this network is figuring out what it sees in the clouds.
And the more time you spend looking at this,
the more things you also will see in the clouds.
You could also use the face network to hallucinate into this,
and you get some pretty crazy stuff.
(Laughter)
Or, Mike has done some other experiments
in which he takes that cloud image,
hallucinates, zooms, hallucinates, zooms hallucinates, zooms.
And in this way,
you can get a sort of fugue state of the network, I suppose,
or a sort of free association,
in which the network is eating its own tail.
So every image is now the basis for,
"What do I think I see next?
What do I think I see next? What do I think I see next?"
I showed this for the first time in public
to a group at a lecture in Seattle called "Higher Education" --
this was right after marijuana was legalized.
(Laughter)
So I'd like to finish up quickly
by just noting that this technology is not constrained.
I've shown you purely visual examples because they're really fun to look at.
It's not a purely visual technology.
Our artist collaborator, Ross Goodwin,
has done experiments involving a camera that takes a picture,
and then a computer in his backpack writes a poem using neural networks,
based on the contents of the image.
And that poetry neural network has been trained
on a large corpus of 20th-century poetry.
And the poetry is, you know,
I think, kind of not bad, actually.
(Laughter)
In closing,
I think that per Michelangelo,
I think he was right;
perception and creativity are very intimately connected.
What we've just seen are neural networks
that are entirely trained to discriminate,
or to recognize different things in the world,
able to be run in reverse, to generate.
One of the things that suggests to me
is not only that Michelangelo really did see
the sculpture in the blocks of stone,
but that any creature, any being, any alien
that is able to do perceptual acts of that sort
is also able to create
because it's exactly the same machinery that's used in both cases.
Also, I think that perception and creativity are by no means
uniquely human.
We start to have computer models that can do exactly these sorts of things.
And that ought to be unsurprising; the brain is computational.
And finally,
computing began as an exercise in designing intelligent machinery.
It was very much modeled after the idea
of how could we make machines intelligent.
And we finally are starting to fulfill now
some of the promises of those early pioneers,
of Turing and von Neumann
and McCulloch and Pitts.
And I think that computing is not just about accounting
or playing Candy Crush or something.
From the beginning, we modeled them after our minds.
And they give us both the ability to understand our own minds better
and to extend them.
Thank you very much.
(Applause)
Voir Plus de Vidéos Connexes
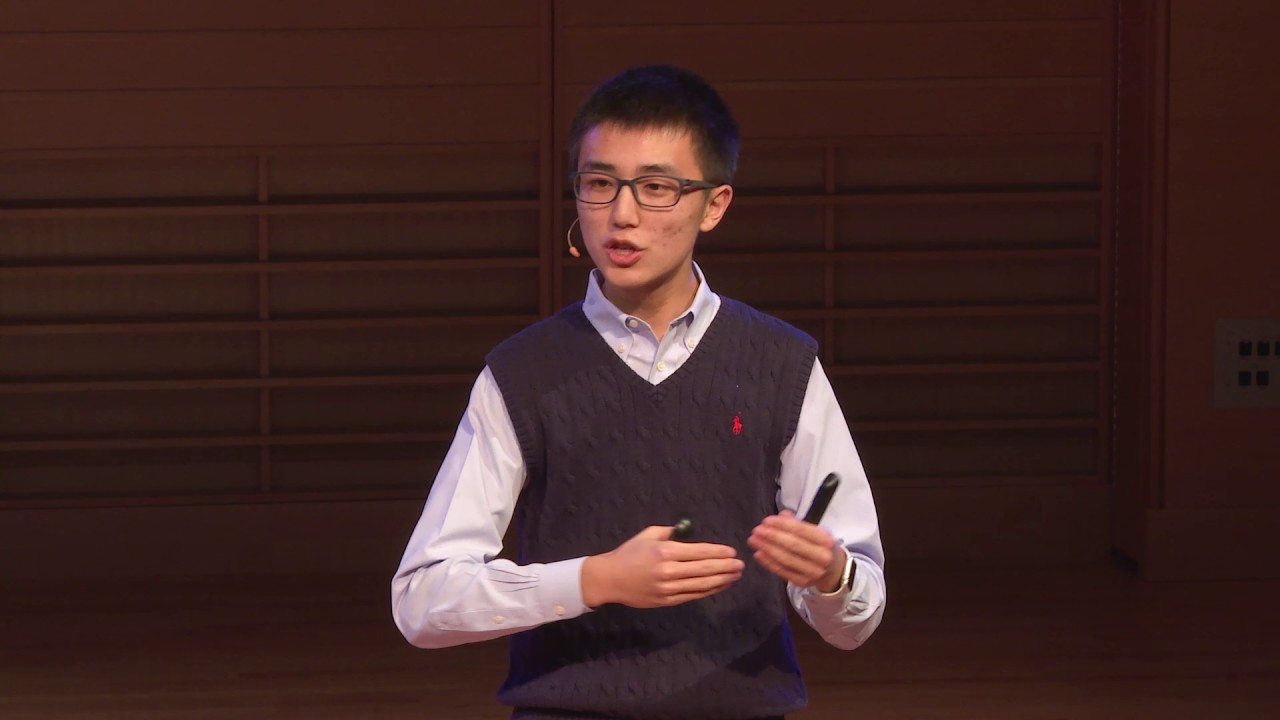
Understanding Artificial Intelligence and Its Future | Neil Nie | TEDxDeerfield

You Don't Understand AI Until You Watch THIS
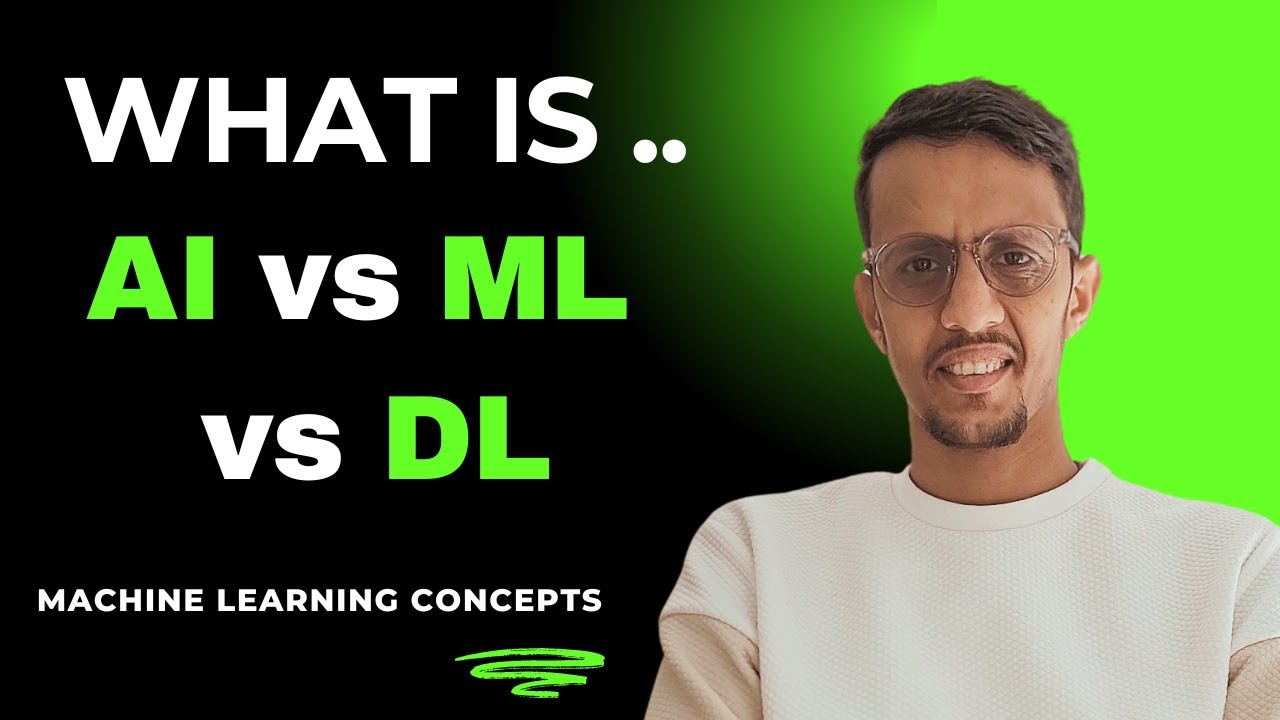
AI Vs Machine Learning Vs Deep Learning - Explained in 4 min!!
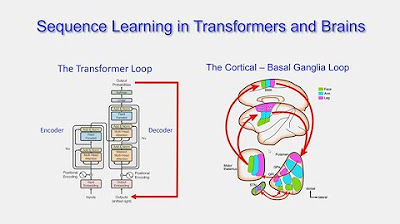
CARTA: Computational Neuroscience and Anthropogeny with Terry Sejnowski
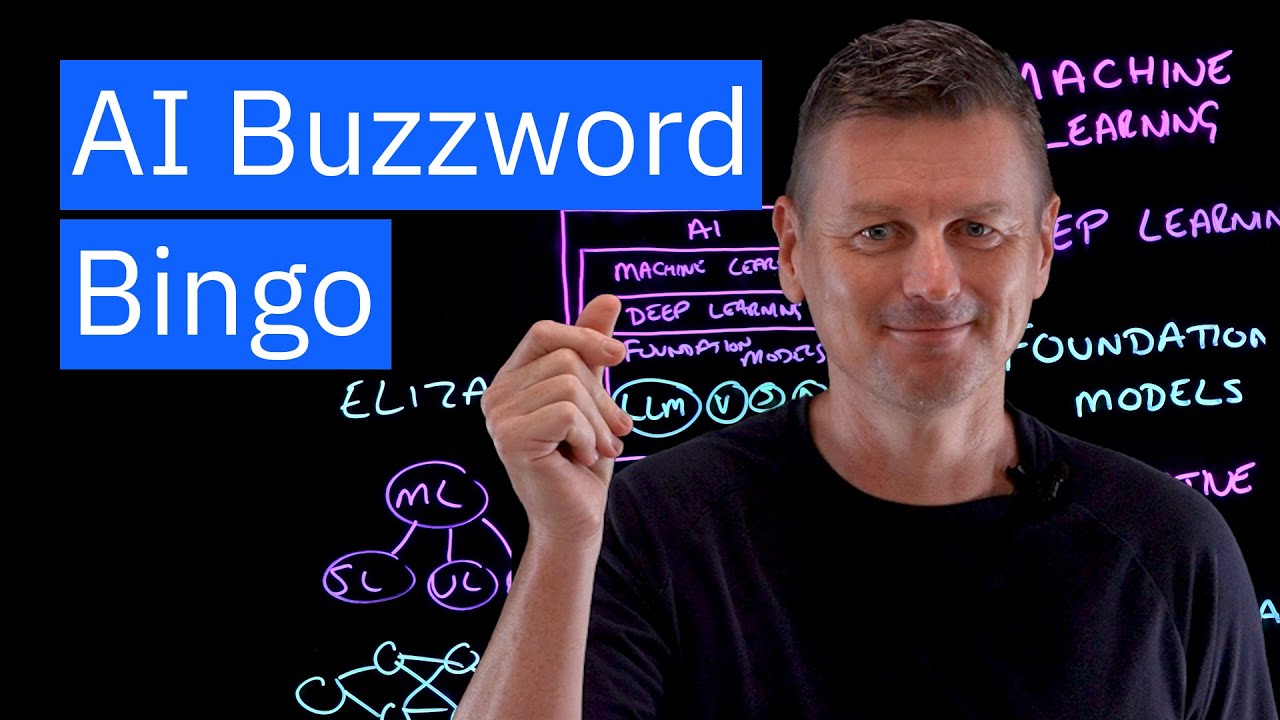
Machine Learning vs. Deep Learning vs. Foundation Models

Understanding the World: Divine Machinery
5.0 / 5 (0 votes)