Andrew Ng - Why Data Engineering is Critical to Data-Centric AI
Summary
TLDRIn this podcast, Andrew and Joe discuss the paradigm shift from model-centric to data-centric AI. They explore how data engineering is pivotal to successful AI implementation, especially with the rise of generative AI and large language models. Andrew highlights the importance of curating high-quality data for training and fine-tuning models. The conversation also touches on emerging AI applications in education and the future of work, emphasizing the potential for generative AI to transform these sectors.
Takeaways
- 📈 Data Centric AI is gaining momentum as a shift from the traditional model-centric approach, emphasizing the importance of data quality and engineering.
- 🔄 The process of training AI models involves a significant amount of data handling, from selection to fine-tuning, which is often more labor-intensive than model engineering itself.
- 🌐 Data issues are prevalent in generative AI, affecting training, fine-tuning, and practical deployment, highlighting the need for robust data strategies.
- 🔄 An example of data curation in AI is Meta's use of an earlier model, llama 3, to generate coding puzzles that trained the subsequent model, llama 3.1.
- 📚 Licensing and procurement of data are significant challenges, especially when aligning data with human values and labeling schemas.
- 🔧 Data engineering is mission-critical for AI, with decisions around data storage, cloud services, and database schemes being vital for performance.
- 🏢 Companies are urged to invest in data infrastructure with specific use cases in mind to avoid over-optimization and to build a strong foundation for AI applications.
- 🤖 Agentic workflows, where AI models engage in a process of thinking and refining their outputs, are emerging as a key strategy to enhance AI output quality.
- 📊 The intelligence of AI models is largely derived from the data they've been trained on, suggesting that data curation is paramount for achieving intelligent behavior.
- 🎓 Generative AI has the potential to transform education, making coding companions and customized courses more accessible and effective.
Q & A
What is the main topic of discussion in the transcript?
-The main topic of discussion is Data Centric AI and its significance in contrast to the traditional model-centric approach in AI development.
What is the significance of data engineering in Data Centric AI?
-Data engineering is critical to Data Centric AI as it involves structuring the data effectively to build successful AI systems, including error analysis, data curation, and making trade-offs between data quality and quantity.
How does Andrew view the shift from model-centric to data-centric AI?
-Andrew sees the shift as a practical evolution where focusing on data engineering and curation is more fruitful than solely engineering the mathematical models or AI algorithms.
What is the role of data in training large language models?
-Data plays a significant role in training large language models, with a large fraction of effort dedicated to acquiring the right data to feed into these models for effective training and fine-tuning.
What is an agentic workflow in the context of AI?
-An agentic workflow in AI involves a series of steps where an AI model generates content, reflects on it, possibly does additional research, and iteratively improves the output before finalizing it.
How does Andrew perceive the current momentum in the Data Centric AI community?
-Andrew finds the momentum in the Data Centric AI community quite exciting, noting the increased focus on data issues across various stages of AI model development.
What is the importance of data infrastructure in building AI applications?
-Data infrastructure is mission critical in building AI applications as it provides the foundation for data storage, management, and accessibility, which are essential for developing and deploying AI models effectively.
What challenges do companies face regarding data in AI, according to Andrew?
-Companies face challenges such as deciding how to store data, choosing cloud services, database schemes, and making trade-offs between cost and performance. Additionally, they struggle with architecting data for specific AI purposes.
How does Andrew suggest companies should approach improving their data infrastructure?
-Andrew advises companies to invest in data for specific purposes, using AI wins as a way to drive data architecture improvements, rather than attempting to fix all data issues at once.
What is Andrew's perspective on the future of AI and its impact on education?
-Andrew anticipates a transformation in education with generative AI playing a significant role, potentially acting as a coding companion or customizing courses, but he emphasizes the need for people to understand coding concepts to effectively use these tools.
What are Andrew's thoughts on the current state and future of Transformer architectures in AI?
-While Andrew acknowledges the dominance of Transformer architectures, he also expresses interest in alternative models like SSRM and diffusion models, suggesting that the field should continue exploring new architectures.
Outlines
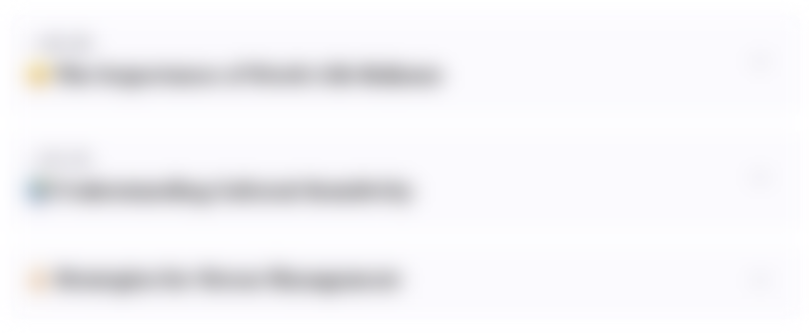
Cette section est réservée aux utilisateurs payants. Améliorez votre compte pour accéder à cette section.
Améliorer maintenantMindmap
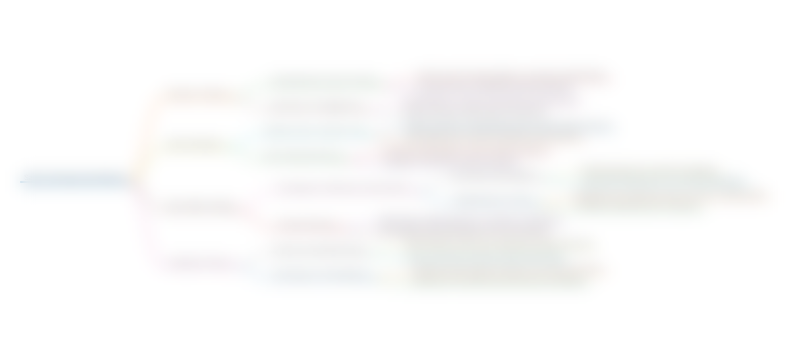
Cette section est réservée aux utilisateurs payants. Améliorez votre compte pour accéder à cette section.
Améliorer maintenantKeywords
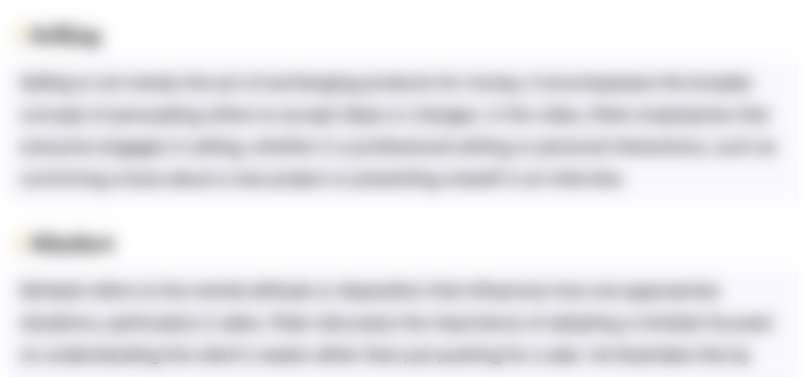
Cette section est réservée aux utilisateurs payants. Améliorez votre compte pour accéder à cette section.
Améliorer maintenantHighlights
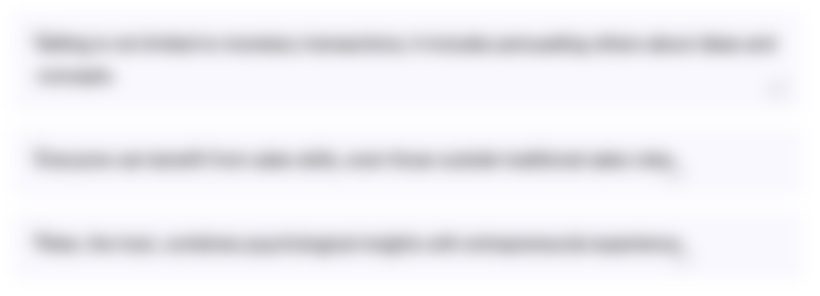
Cette section est réservée aux utilisateurs payants. Améliorez votre compte pour accéder à cette section.
Améliorer maintenantTranscripts
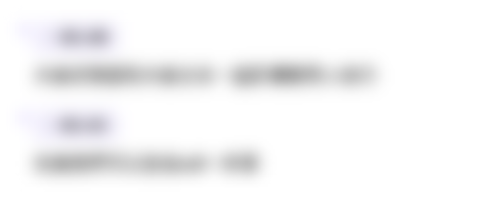
Cette section est réservée aux utilisateurs payants. Améliorez votre compte pour accéder à cette section.
Améliorer maintenantVoir Plus de Vidéos Connexes
5.0 / 5 (0 votes)