Building trust: Strategies for creating ethical and trustworthy AI systems
Summary
TLDRThe video discusses the importance of AI governance in businesses, highlighting challenges and risks associated with generative AI, such as bias, data privacy, and security. It emphasizes the need for comprehensive governance strategies to ensure legal, ethical, and operational compliance. IBM's Watson Governance platform is introduced as a solution that automates AI lifecycle governance, manages risks, and ensures regulatory compliance. The video also addresses the evolving AI landscape, the necessity of collaboration between technical and non-technical teams, and the role of governance in ensuring trustworthy AI implementation across enterprises.
Takeaways
- 🧑💼 IBM emphasizes the need for AI governance in business to address ethical concerns and ensure AI is used safely.
- 📈 Generative AI could increase global GDP by 7% within 10 years, with 80% of enterprises planning to adopt it.
- ⚖️ Business leaders are concerned about ethical issues like bias, safety, and lack of transparency in generative AI.
- 💼 Common generative AI use cases include content generation, summarization, entity recognition, and insight extraction.
- 🚨 AI risks involve data bias, legal concerns, and the potential for adversarial attacks or misuse during model training and inference.
- 🧠 IBM's AI governance focuses on lifecycle monitoring, risk management, and regulatory compliance for both predictive and generative models.
- ⚙️ Automating governance processes, such as tracking model performance, metadata, and compliance, is essential for reducing risks and improving efficiency.
- 🔐 Managing sensitive data and ensuring models handle it responsibly is key to maintaining business trust and meeting regulatory standards.
- 📊 IBM's governance platform aims to ensure transparency, automate documentation, and monitor AI models continuously throughout their lifecycle.
- 🚀 IBM's Watson Governance platform provides end-to-end governance for AI, helping organizations balance performance, risk, and compliance across different environments.
Q & A
What are the key issues AI introduces at the business level?
-AI introduces challenges such as ethical concerns, lack of explainability, safety risks, and biases in generative AI. These issues require careful governance to avoid reputational damage, legal risks, and operational inefficiencies.
Why is AI governance necessary for businesses?
-AI governance ensures that AI models are transparent, accountable, and compliant with legal and ethical standards. It helps businesses mitigate risks, ensure AI models remain fair and accurate, and prevent misuse or harm.
What are the main use cases of generative AI mentioned in the script?
-Generative AI use cases include retrieval-augmented generation, summarization, content generation, named entity recognition, insight extraction, and classification.
What are the risks associated with the training phase of AI models?
-Training-phase risks include biases present in the training data, data poisoning attacks, and legal restrictions related to the use of sensitive or copyrighted data.
What are adversarial attacks during the inference phase, and how can they affect AI models?
-Adversarial attacks, such as evasion or prompt injection, occur when attackers manipulate input during the inference phase to produce harmful or biased outputs, compromising the AI model’s reliability.
What are some real-world cases illustrating AI model risks?
-Examples include a dealership's AI bot mistakenly selling a Chevy Tahoe for $1 and Microsoft's Twitter chatbot turning offensive due to learning inappropriate behavior from user interactions.
How does IBM's Watson Governance platform address AI governance needs?
-The Watson Governance platform automates life cycle management, risk governance, and regulatory compliance for AI models. It helps businesses ensure model accuracy, fairness, and transparency across development and deployment.
What are the three critical capabilities identified by IBM for AI governance?
-The three capabilities are monitoring and evaluating models, tracking facts and metrics, and managing the life cycle and risks of AI models.
What is 'prompt governance,' and why is it important for foundation models?
-Prompt governance involves tracking and evaluating text-based instructions (prompts) used with foundation models. It is essential to ensure that prompts are properly managed, evaluated for quality, and monitored for safety to avoid generating harmful content.
What role does monitoring model performance play in AI governance?
-Monitoring model performance ensures that AI models and prompts remain accurate, efficient, and safe over time. It helps detect issues like performance degradation, data drift, and the presence of toxic language or personal information in outputs.
Outlines
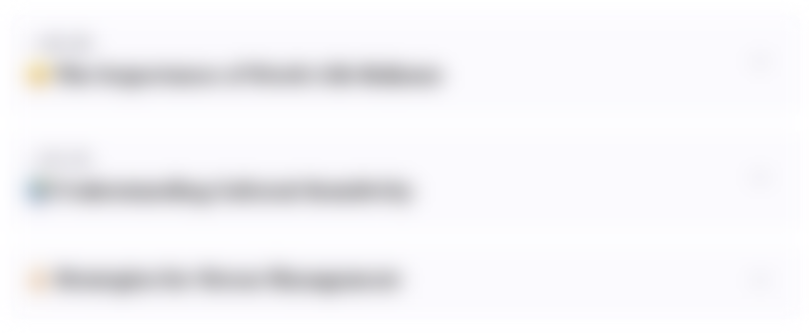
Cette section est réservée aux utilisateurs payants. Améliorez votre compte pour accéder à cette section.
Améliorer maintenantMindmap
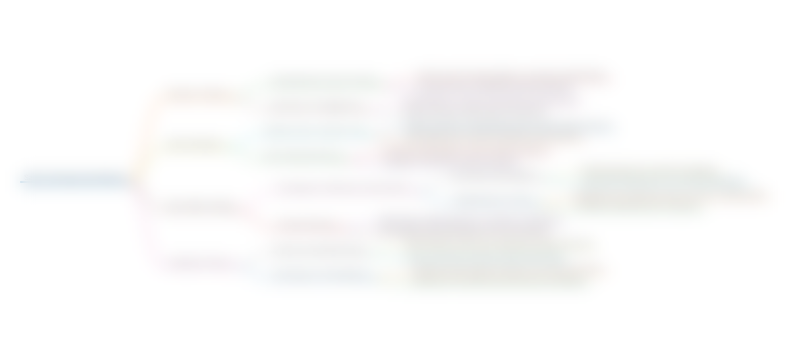
Cette section est réservée aux utilisateurs payants. Améliorez votre compte pour accéder à cette section.
Améliorer maintenantKeywords
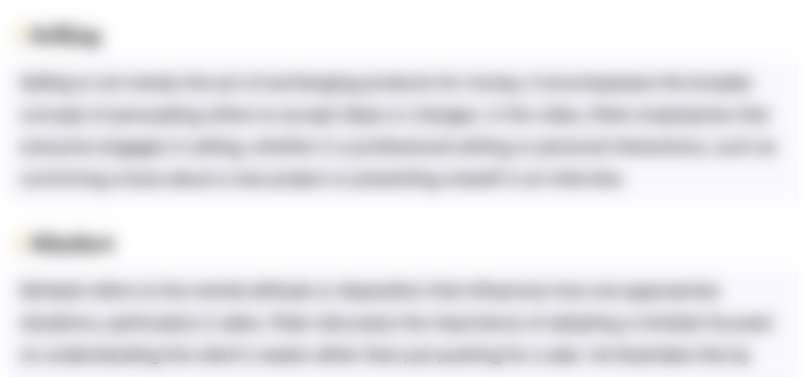
Cette section est réservée aux utilisateurs payants. Améliorez votre compte pour accéder à cette section.
Améliorer maintenantHighlights
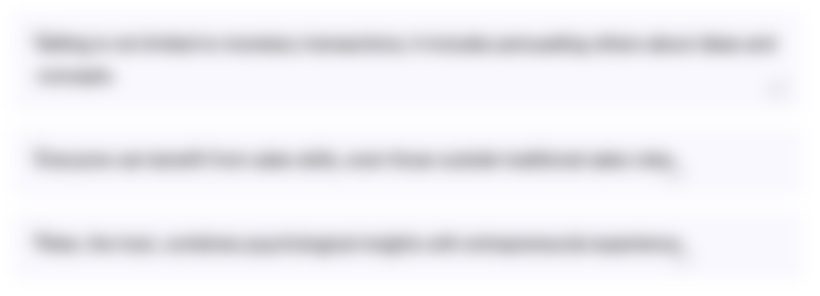
Cette section est réservée aux utilisateurs payants. Améliorez votre compte pour accéder à cette section.
Améliorer maintenantTranscripts
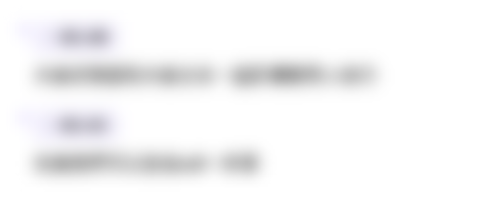
Cette section est réservée aux utilisateurs payants. Améliorez votre compte pour accéder à cette section.
Améliorer maintenantVoir Plus de Vidéos Connexes
5.0 / 5 (0 votes)