Generative AI: Moving Beyond The Hype & Hysteria
Summary
TLDRThe video explores why generative AI is gaining significant attention. It compares generative AI's growth to the 'Big Data' trend, noting that generative AI goes beyond traditional machine learning by creating new data rather than classifying existing data. The speaker highlights real-world applications, like Wayfair's AI-powered room designs, and discusses how AI tools streamline creative processes without threatening jobs. The challenges of AI, such as hallucinations and legal concerns, are also mentioned. The speaker concludes by emphasizing AI's future, combining multiple models and applications for better, task-specific results.
Takeaways
- 📈 **Generative AI's Rise**: Generative AI is gaining popularity because it uses historical data to create new data instances, unlike traditional analytics that append information to existing data.
- 🚀 **Innovation Overload**: The ability to generate new data instances opens up new use cases that were not possible before, similar to how Big Data revolutionized the industry a decade ago.
- 🏠 **Practical Applications**: Companies like Wayfair use generative AI to allow customers to visualize room designs with actual purchasable products, streamlining the buying process.
- 🎨 **Creative Freedom**: Generative AI could free up designers to focus on more complex tasks by handling routine design mockups that customers might not purchase.
- 🤖 **Seamless Integration**: The technology is being integrated into tools like Adobe's, allowing users to interact with AI capabilities without needing to understand the underlying technology.
- 🤝 **Contrarian View on Fair Use**: The speaker argues that generative AI's use of training data is analogous to how artists and musicians learn from past works, suggesting AI should not be held to a higher standard.
- 📚 **Educational Parallel**: Just as students learn from existing works to create their own, generative AI learns from vast amounts of data to produce new creations.
- 🔍 **Hallucination Misconception**: Generative AI does not 'hallucinate' or match prompts to existing data; instead, it creates entirely new outputs based on mathematical probabilities.
- ⏲️ **Recency and Obscurity**: The accuracy of generative AI is influenced by the recency and obscurity of the information; well-documented recent events are more likely to be accurately represented.
- 📉 **Model Collapse Risk**: There's a risk of 'model collapse' where the AI's output becomes less diverse and converges towards the mean, reducing its effectiveness over time.
- 🛠️ **Ensemble Approaches**: The future of generative AI may involve using multiple models in an ensemble approach to increase accuracy and reliability.
Q & A
Why has generative AI gained so much attention recently?
-Generative AI has gained attention because it creates new examples of data entirely, such as new images or strings of text, instead of simply classifying or predicting based on existing data. This ability opens up new possibilities that were not possible with traditional AI models.
How does generative AI differ from traditional machine learning models?
-Traditional machine learning models classify or make predictions based on existing data, such as identifying if an image contains a cat or not. In contrast, generative AI creates new data by generating unique examples rather than analyzing existing ones.
What is an example of a business application of generative AI mentioned in the transcript?
-Wayfair uses generative AI in a tool that allows customers to upload an image of their space and input design preferences. The AI generates a visual of the room with purchasable products, allowing customers to see what their space could look like and easily shop for items.
Does generative AI threaten jobs, such as interior designers?
-The transcript suggests that generative AI doesn't necessarily threaten jobs like those of interior designers. Instead, it allows them to focus on more complex, creative tasks rather than routine tasks, such as creating multiple mockups for undecided customers.
How has the integration of analytics and AI changed over time?
-In the past, analytics were more visible, and users knew they were interacting with algorithms. Today, AI functionalities are seamlessly embedded into products and services, so users often interact with AI without even realizing it, such as generating images or analyzing credit scores.
What is the 'contrarian view' on AI and copyright raised in the transcript?
-The speaker argues that AI should not be held to a higher standard than humans when it comes to learning from existing content. Just as art or music students learn by studying past works, generative AI uses large datasets to create new works, and this should not inherently be seen as problematic.
What are AI 'hallucinations' and why do they occur?
-AI hallucinations refer to situations where AI generates incorrect or made-up information. This happens because generative AI doesn't retrieve real-world documents or facts but creates responses based on mathematical probabilities derived from its training data.
What is one of the major limitations of current public AI models?
-One major limitation is that public AI models, such as GPT, are trained on data up to a certain point (e.g., 2021). As a result, they may not be able to provide accurate information about recent events or conflicting documentation.
What risk does 'model collapse' pose for generative AI?
-Model collapse occurs when AI models are trained on data that includes a large amount of generated content instead of human-created content. Over time, this can cause the model to produce less accurate or more generic outputs, which diminishes its usefulness.
How can companies maximize the value of generative AI according to the transcript?
-Companies can maximize the value of generative AI by building application layers that parse user prompts and direct parts of the task to the appropriate models, such as using a search engine for factual data and a math engine for calculations. This ensemble approach leverages AI’s strengths for different types of tasks.
Outlines
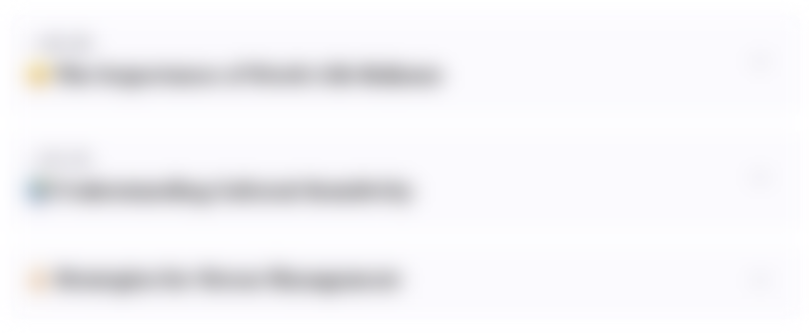
Cette section est réservée aux utilisateurs payants. Améliorez votre compte pour accéder à cette section.
Améliorer maintenantMindmap
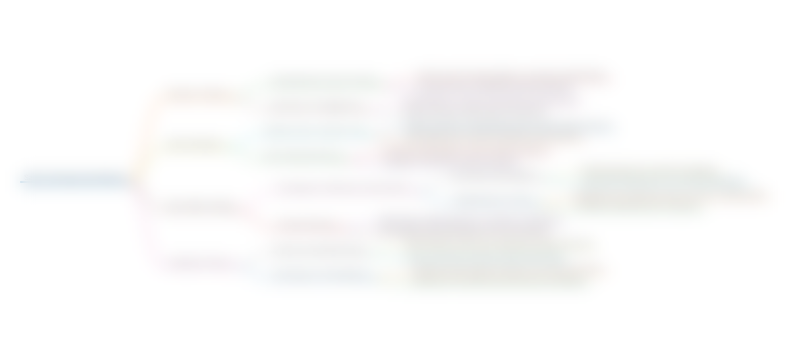
Cette section est réservée aux utilisateurs payants. Améliorez votre compte pour accéder à cette section.
Améliorer maintenantKeywords
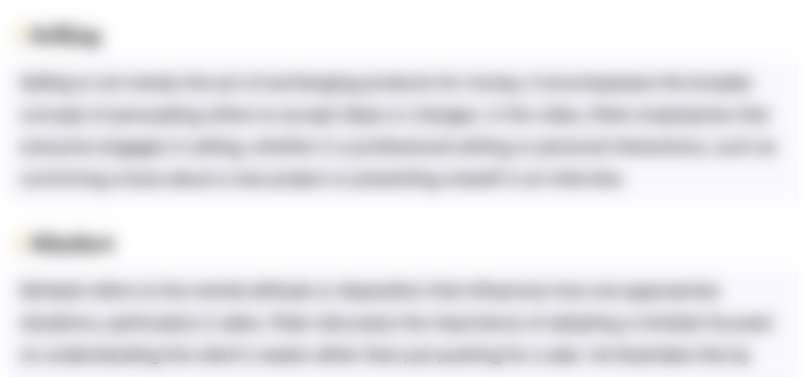
Cette section est réservée aux utilisateurs payants. Améliorez votre compte pour accéder à cette section.
Améliorer maintenantHighlights
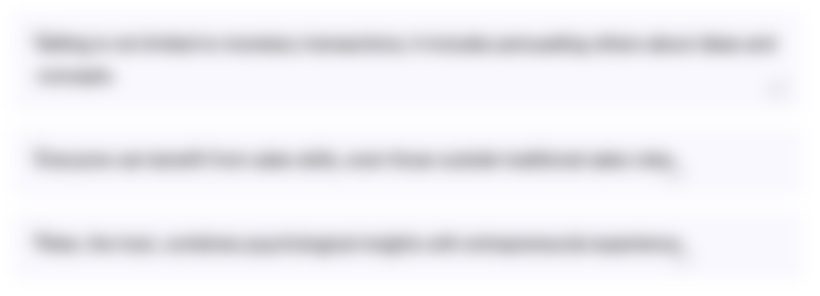
Cette section est réservée aux utilisateurs payants. Améliorez votre compte pour accéder à cette section.
Améliorer maintenantTranscripts
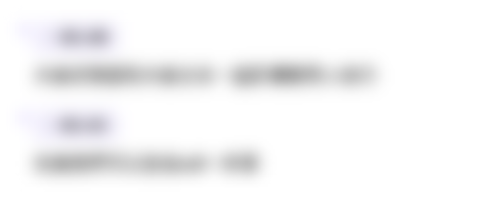
Cette section est réservée aux utilisateurs payants. Améliorez votre compte pour accéder à cette section.
Améliorer maintenantVoir Plus de Vidéos Connexes
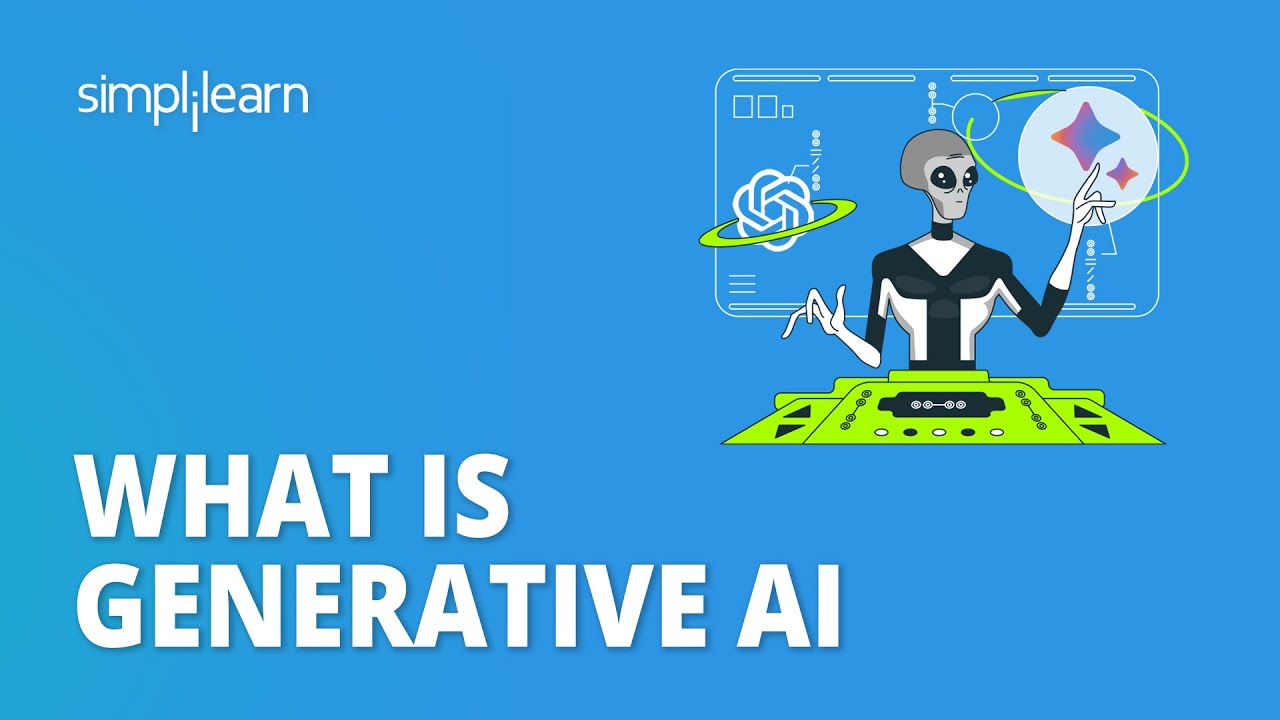
What is Generative AI | Introduction to Generative AI | Generative AI Explained | Simplilearn
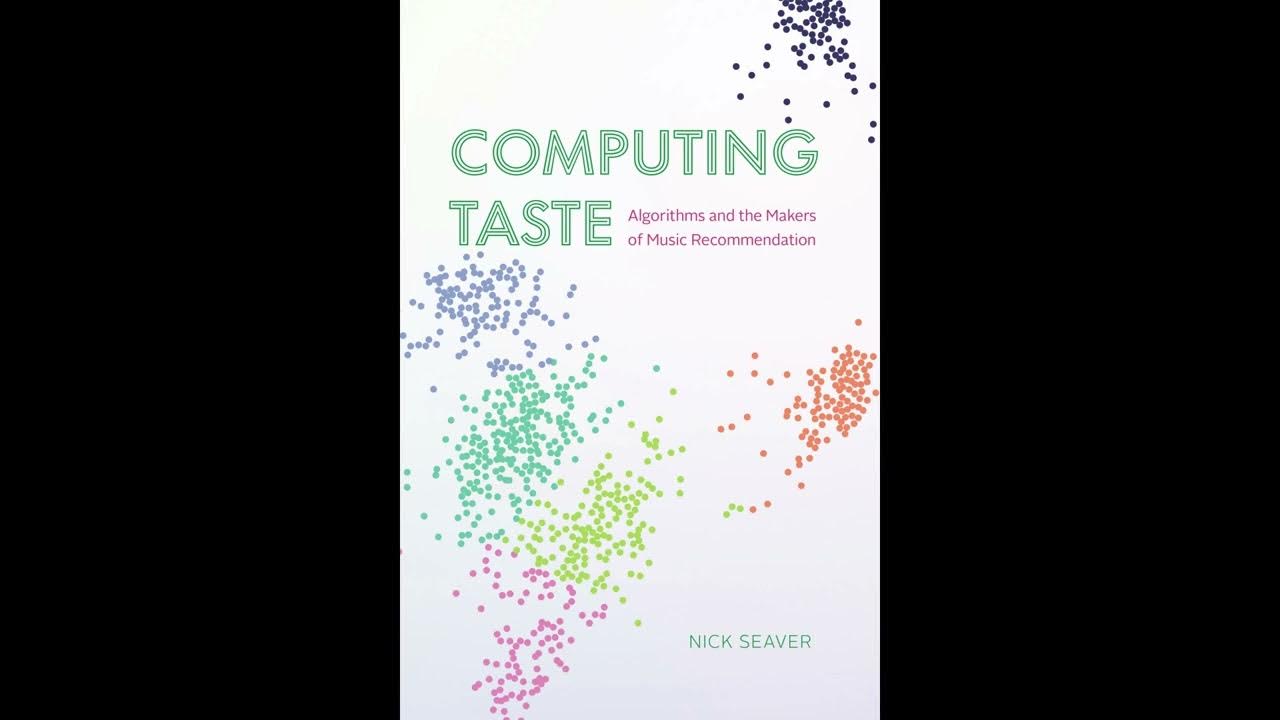
Nick Seaver discussing his book, Computing Taste: Algorithms and the Makers of Music Recommendation
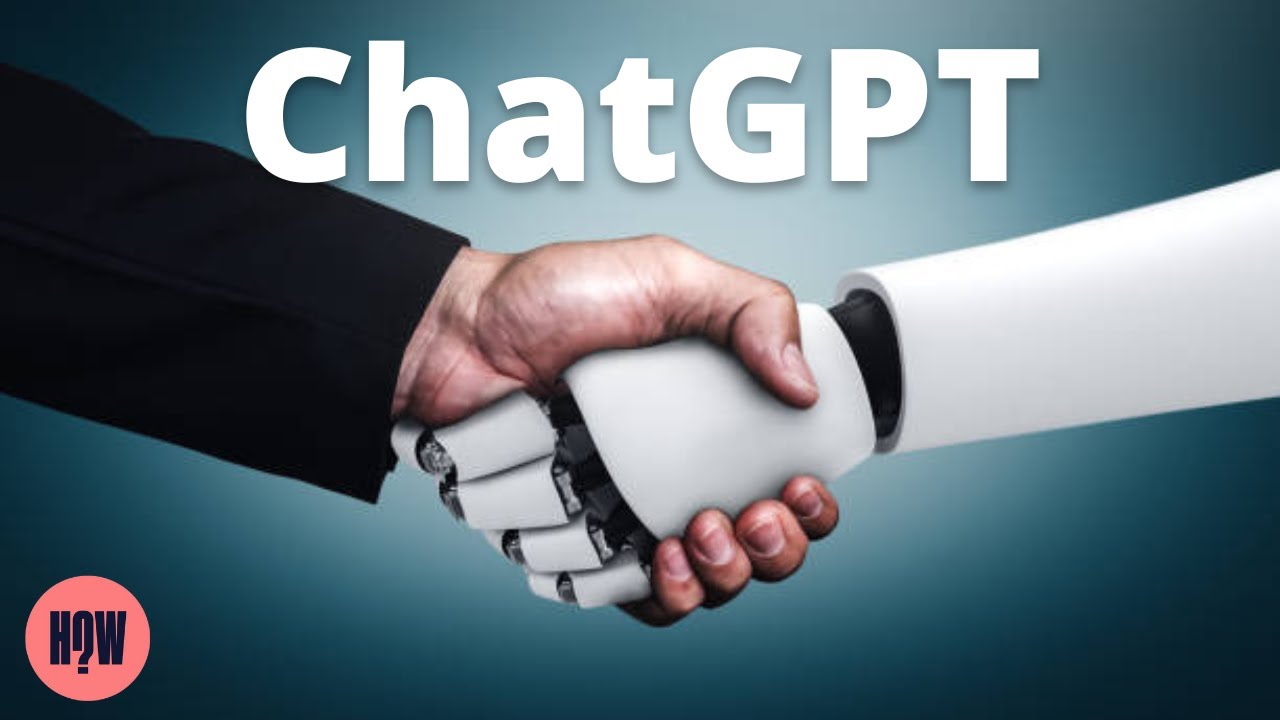
What is ChatGPT? OpenAI's Chat GPT Explained
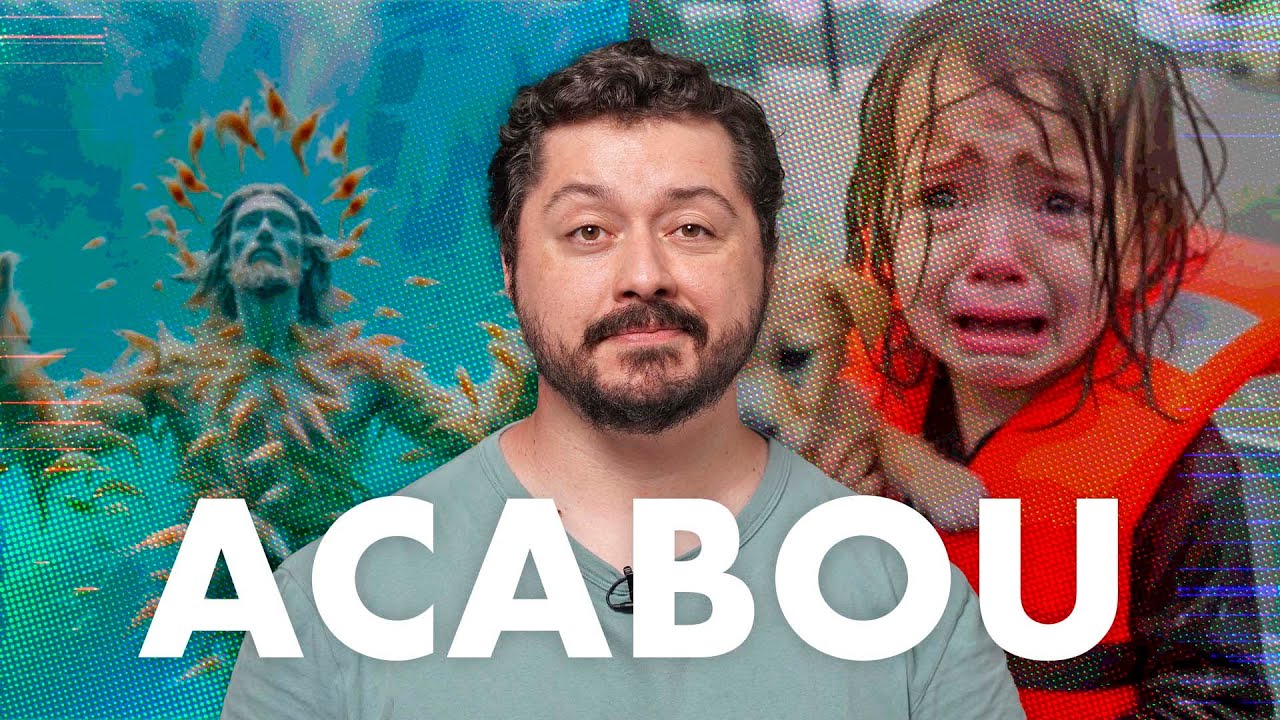
O fim da realidade nas redes

These AI Cryptos Will Print MILLIONS this ALTCOIN SEASON (I’M ALL IN)
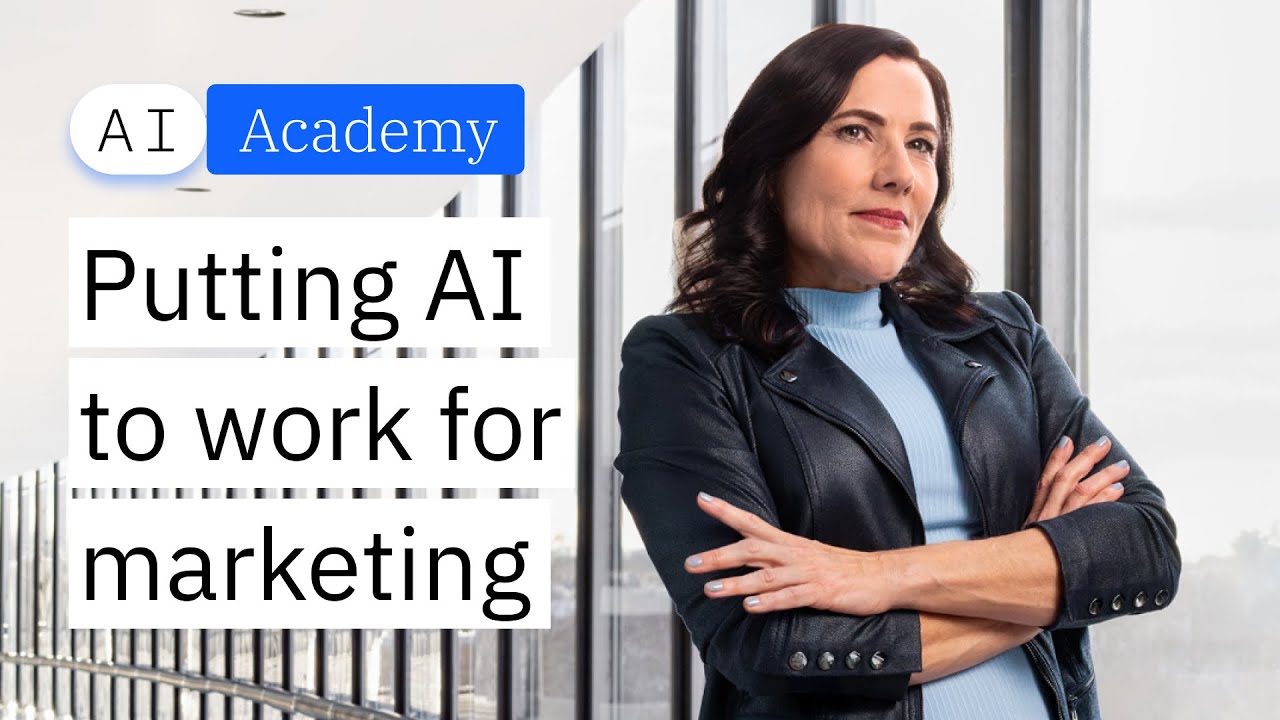
Putting AI to Work for Marketing
5.0 / 5 (0 votes)