Challenges and Current Trends of Big Data Technologies: Part 1
Summary
TLDRThis lecture explores the challenges and current trends in Big Data technologies, focusing on their application in enterprise data warehouses and business intelligence. It highlights the complexities of handling structured, semi-structured, and unstructured data from diverse sources like websites, social media, and IoT devices. Key challenges include data storage, integration, latency, and real-time processing. The lecture also covers the characteristics of Big Data, known as the five Vs—volume, velocity, variety, veracity, and value. Additionally, it introduces the Big Data technology stack, emphasizing its flexibility, efficiency, and advanced analytics capabilities.
Takeaways
- 💡 Big Data technologies enhance business insights and decision-making but come with significant challenges in enterprise adoption.
- 🔍 The lecture covers an introduction to Big Data, enterprise data landscape, Big Data characteristics, and adoption challenges.
- 📊 Large amounts of structured, semi-structured, and unstructured data come from various sources like websites, social media, and sensors.
- 🧠 Big Data technologies enable the analysis of all available data, which is crucial for making intelligent business decisions.
- 🚧 The evolving nature of Big Data technologies presents challenges, particularly in adhering to enterprise quality-of-service requirements.
- 🏗️ Enterprise data can be categorized into transactional data, observational data, social interaction data, and Enterprise Content data.
- ⚙️ Key challenges in Big Data adoption include integrating large volumes of data, managing data latency, and ensuring real-time processing.
- 🔄 Data flexibility is crucial in supporting various sources and consumption mechanisms, while accuracy and validation of Big Data remain critical.
- 📈 The five characteristics of Big Data are volume, velocity, variety, veracity, and value, each playing a critical role in enterprise use.
- 🔧 The Big Data technology stack offers features like flexible schema, real-time processing, advanced analytics, and reliable management capabilities.
Q & A
What are the main challenges in enterprise adoption of Big Data technologies?
-The main challenges include storing, integrating, and linking data, latency between data generation and consumption, flexibility in data in and out, data cleansing and validation, and return on investment.
How does Big Data technology enable better business insights and decisions?
-Big Data technology allows for the analysis of large amounts of structured, semi-structured, and unstructured data from various sources, which helps in making intelligent business decisions.
What are the four types of data in the enterprise data landscape?
-The four types of data are transactional data, observational data, social interaction data, and Enterprise Content data.
What are the five Vs associated with the characteristics of Big Data?
-The five Vs are Volume, Velocity, Variety, Veracity, and Value.
What does Volume in Big Data refer to?
-Volume refers to the huge amounts of data generated every day that contribute to the size of data.
How is Velocity defined in the context of Big Data?
-Velocity refers to the rapid changes in data generated from various devices and sources like RFID tags and sensors.
What is the significance of Variety in Big Data?
-Variety signifies that 80% of the world's data is unstructured and comes from a variety of sources, making the data varied in nature.
Why is Veracity an important characteristic of Big Data?
-Veracity is important because it refers to the accuracy of data, which is crucial for business leaders to trust the information they use for decision-making.
What is the role of Value in Big Data?
-Value is about driving overall business value from various data sources and analyzing them to extract insights.
What are some of the key features of the Big Data technology stack?
-Key features include flexible schema, efficient batch and real-time processing, indexing of distributed data, support for advanced analytics and modeling, ease of management with auto-sharding and partitioning, reliability, high availability, and standard access mechanisms like JDBC, ODBC, JSON, and REST.
What is the importance of data lineage in the enterprise data landscape?
-Data lineage is crucial for understanding the flow of data across the entire supply chain, which is important for data integration and traceability.
How does the evolving nature of Big Data technologies pose challenges to quality of service requirements?
-The evolving nature of Big Data technologies can make it difficult for enterprises to adhere to quality of service requirements due to the need for continuous adaptation and updates to keep up with new developments.
Outlines
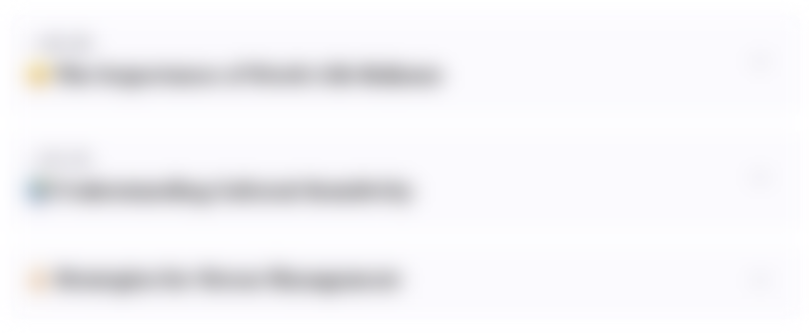
Cette section est réservée aux utilisateurs payants. Améliorez votre compte pour accéder à cette section.
Améliorer maintenantMindmap
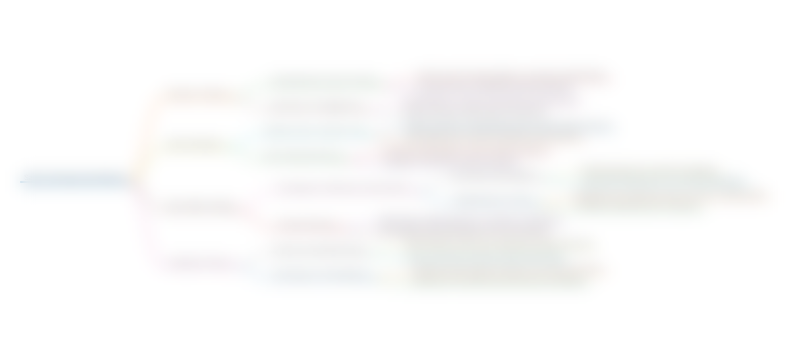
Cette section est réservée aux utilisateurs payants. Améliorez votre compte pour accéder à cette section.
Améliorer maintenantKeywords
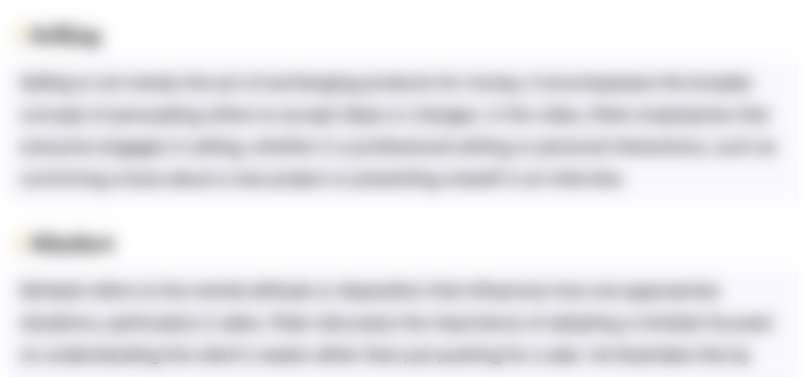
Cette section est réservée aux utilisateurs payants. Améliorez votre compte pour accéder à cette section.
Améliorer maintenantHighlights
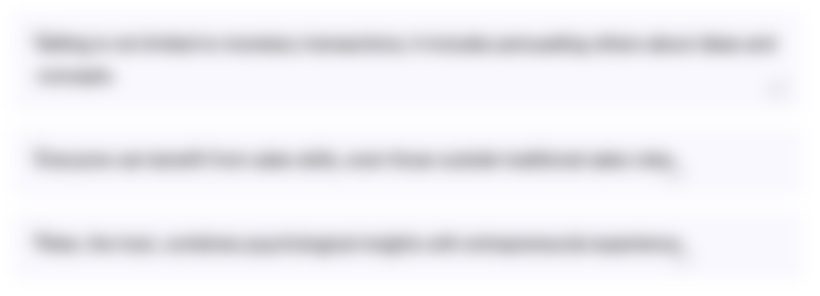
Cette section est réservée aux utilisateurs payants. Améliorez votre compte pour accéder à cette section.
Améliorer maintenantTranscripts
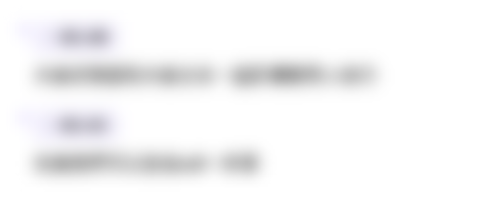
Cette section est réservée aux utilisateurs payants. Améliorez votre compte pour accéder à cette section.
Améliorer maintenantVoir Plus de Vidéos Connexes

The 5Vs of Big Data (characteristics) #BigData #bigdataanalytics
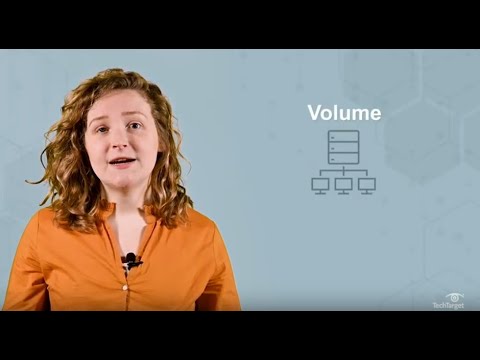
What is Big Data and What is it Used For?
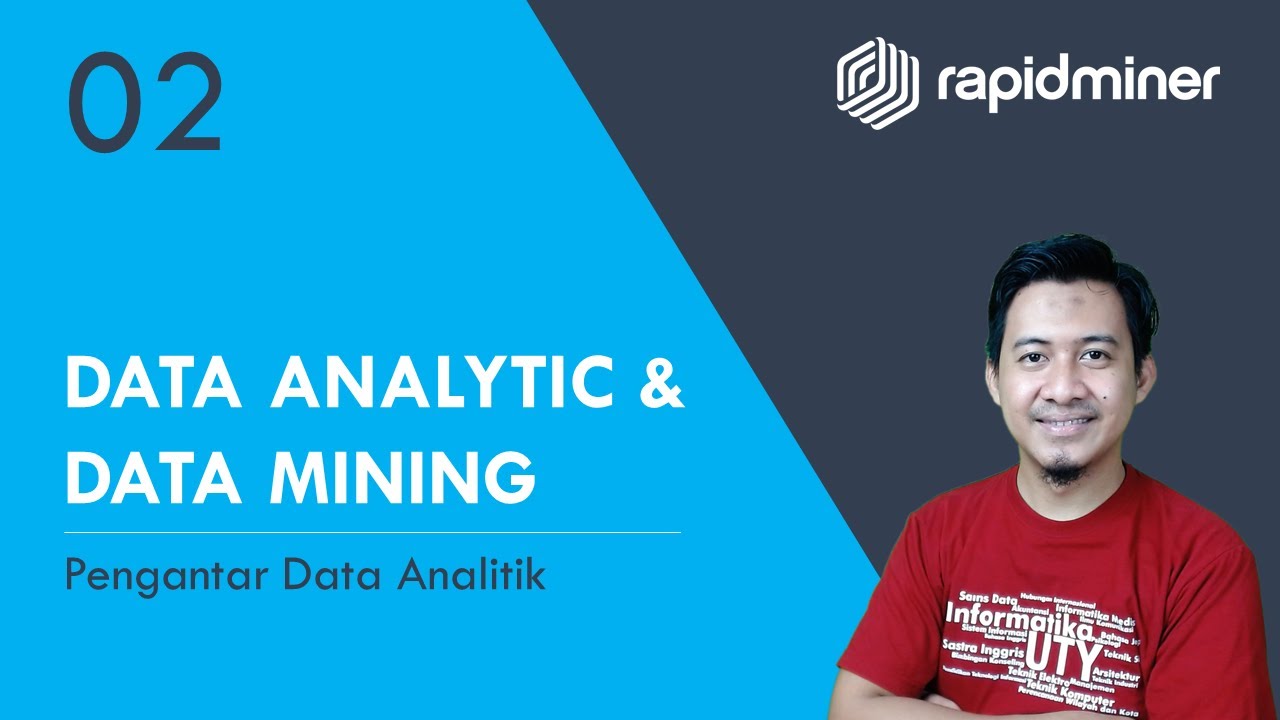
Pengantar Data Analitik - Perkuliahan Data Analytic & Data Mining #02

ArcGIS GeoAnalytics Engine: On-demand geospatial data analysis
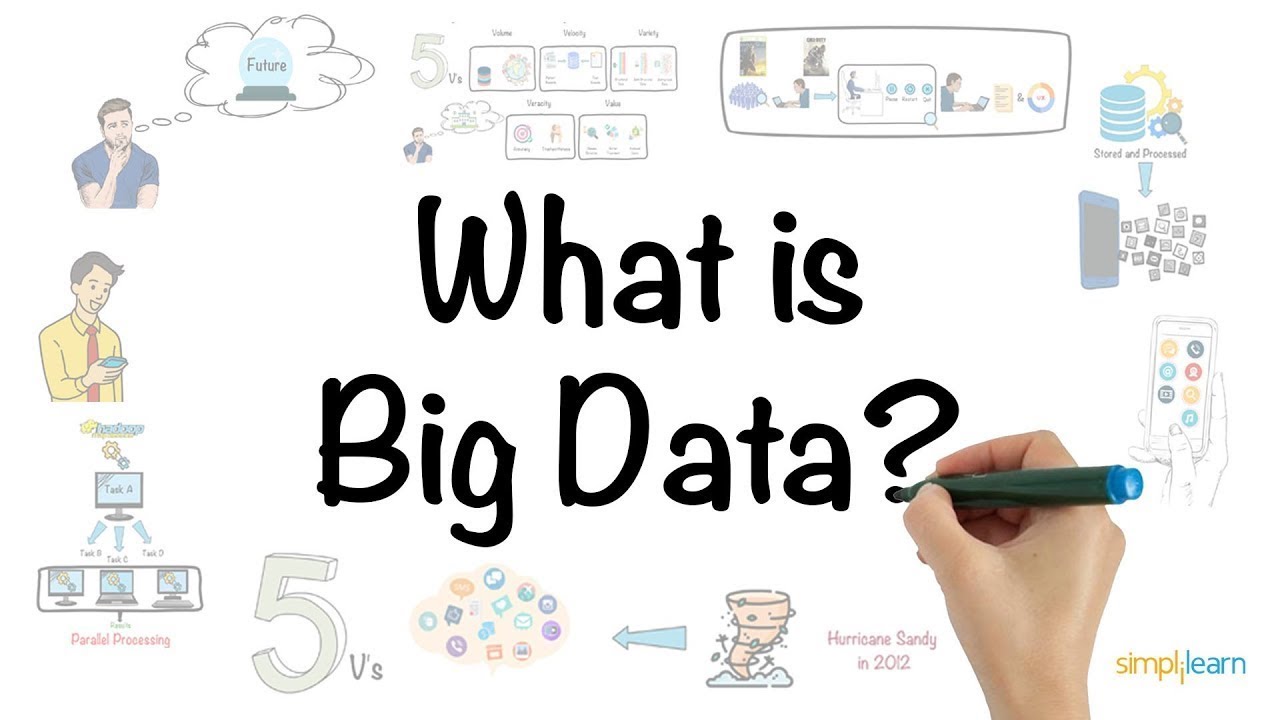
Big Data In 5 Minutes | What Is Big Data?| Big Data Analytics | Big Data Tutorial | Simplilearn

Simulasi Perhitungan 7 V Big Data Analytic Google Sheet
5.0 / 5 (0 votes)