Model Autotuning, Generative AI | SAS Viya March 2023 Release
Summary
TLDRIn the April 2023 SAS Viya release highlight show, host Thiago Doza discusses new features with experts. Sasha Karpinsky introduces Microsoft Word integration for SAS, allowing users to embed and update SAS reports in Word documents. Joe Madden presents updates in machine learning, including the new ASTORE model format for neural networks and autotuning capabilities in Light GBM. Alex Vilan covers enhancements in SAS Studio, such as data engineering steps and custom column steps. The show also features part two of an interview with Mary Osborne on generative AI models, exploring their potential and ethical considerations in various applications.
Takeaways
- 😀 The SAS Viya release as of April 2023 includes significant updates and integrations.
- 📈 Integration with Microsoft Word allows for seamless sharing of SAS analytical insights within Word documents.
- 💡 Machine learning updates feature a new ASTORE model format for neural networks, enhancing deployability and scoring speed.
- 🔧 Autotuning capabilities in Light GBM have been introduced to improve efficiency and scalability for large datasets.
- 🎨 SAS Studio has been enhanced with new data engineering steps, custom column steps, and an analyst step for ranking data.
- 🔄 The ability to redeploy Studio flows using SAS and Python programs has been added for greater flexibility.
- 🤖 Generative AI models, like the BERT-based classifier, are being integrated into SAS Viya to improve natural language processing.
- 👩🏫 Generative AI has the potential to revolutionize personalized education and product recommendation systems.
- 🏥 Synthetic data generation can aid researchers in modeling rare diseases by expanding data sets for better analysis.
- 🛡️ SAS is taking a cautious approach to generative AI, prioritizing ethical considerations and risk mitigation.
- 📚 Users are advised to verify the information generated by AI tools like chat GPT to ensure accuracy and reliability.
Q & A
What is the main topic of the SAS Viya Release Highlight Show for April 2023?
-The main topic is the new features and updates in SAS Viya as of April 2023, including integrations and enhancements in areas like Microsoft Word, machine learning, and SAS Studio.
How does the new Microsoft Word integration in SAS Viya work?
-The integration allows users to view and interact with SAS visual analytics reports, insert visual insights directly into Word documents, and update embedded SAS content with the latest data, all without leaving the Word application.
What is the significance of the aStore model format for neural networks in SAS Viya?
-The aStore model format is significant because it is easily deployable, more portable, and faster than traditional data step methods, leading to a significant improvement in scoring time for neural networks.
What is the new autotuning capability in Light GBM as discussed in the show?
-The new autotuning capability in Light GBM allows for efficiency and scalability for large datasets with many features, and it includes unique parameters like bagging fraction, bagging frequency, lasso, and ridge.
What are the new features introduced in SAS Studio for data engineering?
-SAS Studio introduces advanced data engineering capabilities such as the ability to create outputs as views, a new 'rank data' step for assigning rank values, and extended scheduling functionality for Studio flows.
How can users deploy or redeploy Studio flows using SAS and Python programs?
-Users can deploy Studio flows as jobs without specifying a scheduling trigger and redeploy jobs that have been previously deployed from a specific Studio flow, with this option also supported for SAS and Python programs.
What is the focus of the interview with Mary Osborne in the show?
-The interview focuses on generative AI models, discussing the recent release of a BERT-based classifier in SAS Viya, the concept of generative AI, and its potential impact on everyday life and business.
What are some examples of how generative AI can impact everyday life?
-Generative AI can impact everyday life by providing personalized instruction through 'teacher in the box' concepts, curating product information, and generating synthetic data for research purposes, among other applications.
What are the key technologies behind generative AI as discussed in the interview?
-The key technologies behind generative AI include large language models based on transformer architecture, such as BERT and GPT, and generative adversarial models for generating synthetic tabular data.
What is SAS's approach to the development and deployment of generative AI models?
-SAS is taking a conservative approach to the development and deployment of generative AI models, focusing on trustworthiness, ethical considerations, mitigating risks, and ensuring a strong return on investment for customers.
What advice does Mary Osborne give to casual users of generative AI like chat GPT?
-Mary Osborne advises casual users to use generative AI with caution, always verify the information it provides, and be aware that the models can generate very plausible but incorrect or non-existent content.
Outlines
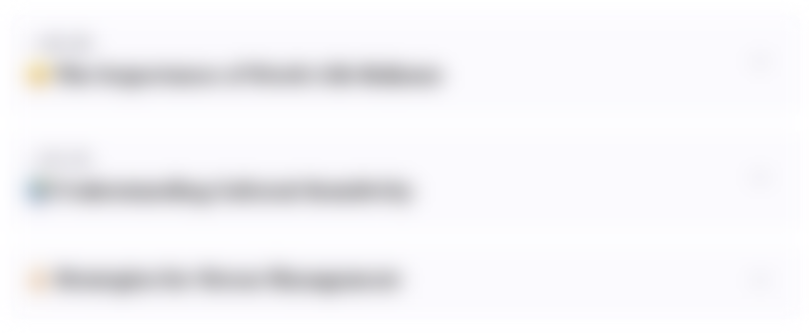
Cette section est réservée aux utilisateurs payants. Améliorez votre compte pour accéder à cette section.
Améliorer maintenantMindmap
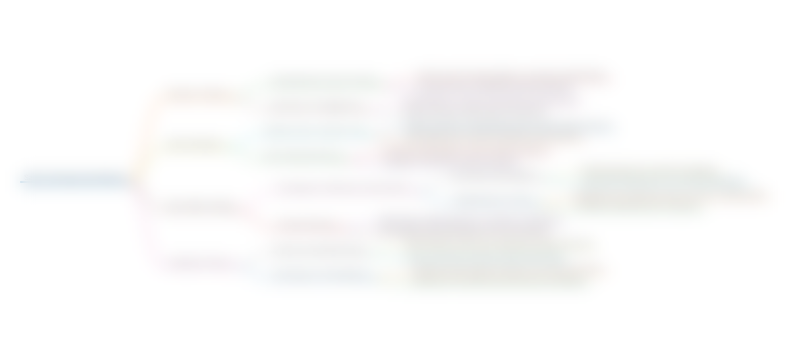
Cette section est réservée aux utilisateurs payants. Améliorez votre compte pour accéder à cette section.
Améliorer maintenantKeywords
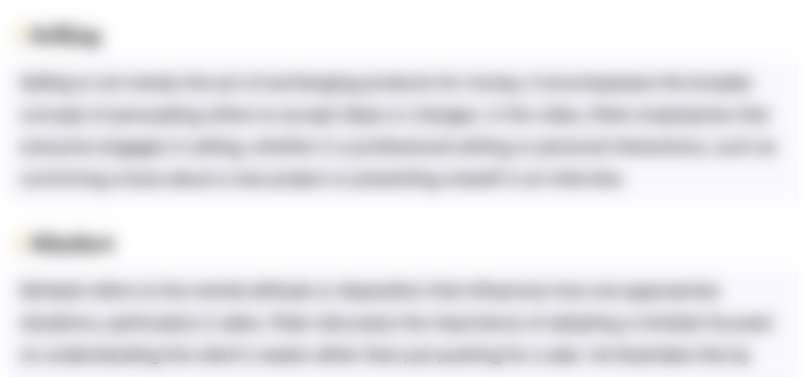
Cette section est réservée aux utilisateurs payants. Améliorez votre compte pour accéder à cette section.
Améliorer maintenantHighlights
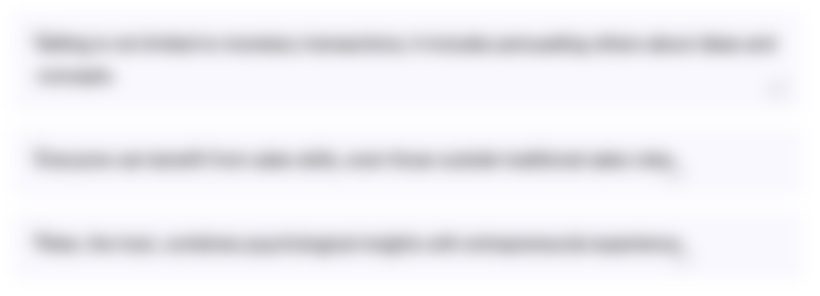
Cette section est réservée aux utilisateurs payants. Améliorez votre compte pour accéder à cette section.
Améliorer maintenantTranscripts
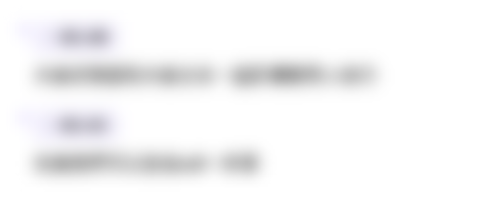
Cette section est réservée aux utilisateurs payants. Améliorez votre compte pour accéder à cette section.
Améliorer maintenant5.0 / 5 (0 votes)