[ARDD 2024 Recap] Dual-Purpose Target Discovery and Aging Research with Precious GPT Models
Summary
TLDRFrank presents the advancements in AI-driven drug discovery, focusing on dual-purpose target discovery for both aging and cancer treatments. He discusses the integration of multiomic data and AI models to identify potential therapeutic targets. The research highlights the discovery of the target TEIC for IPF (idiopathic pulmonary fibrosis), which progressed to clinical trials within 30 months. He also showcases the identification of the KD M1A target, validated through experiments, demonstrating how AI facilitates the discovery of targets that impact both cancer and aging simultaneously.
Takeaways
- đ Frank discusses the application of AI in drug discovery, specifically in target discovery, indication expansion, and drug repurposing.
- đ„ The paper published in Translational Pharmacological Sciences highlights AI's role in drug discovery, with AI-identified targets advancing to clinical trials.
- đ§Ź The AI and bioinformatics models utilize multiomic data to calculate disease-gene interactions in real-time, aiding in target discovery and indication expansion.
- đ A paper published in Nature about 'teic' as a target for IPF (Idiopathic Pulmonary Fibrosis) showcases AI's comprehensive impact on drug discovery.
- đ The AI platform 'Pandemics' identified 'teic' as a promising target for IPF, emphasizing the importance of targeting age-associated diseases.
- đ§Ș Generative AI, specifically 'Chemistry 42', was used to design novel molecules to target 'teic', demonstrating AI's role in molecular design.
- â±ïž The development from target identification to clinical trials was achieved in a record 30 months, showcasing the efficiency of AI-assisted drug discovery.
- đŹ The study integrates panomics to identify targets implicated in multiple aging diseases, considering aging as a primary driver of diseases.
- đ Collaboration with the University of Oso focuses on identifying dual-purpose targets effective for both cancer and aging treatments.
- đ§Ș KDM1A is highlighted as a potential dual-purpose target, being upregulated in multiple solid tumors and negatively associated with aging hallmarks.
Q & A
What is the main focus of the presentation?
-The presentation focuses on the use of AI, specifically GPT models, in drug discovery, target discovery, indication expansion, and drug repurposing.
What success has been achieved since the use of AI in target discovery?
-Since 2020, several AI-identified targets have been validated in the lab, and one of the identified targets has progressed to human clinical phase 2 trials.
What approach is used for target discovery in the research?
-The approach uses multiomic data and text data, combined with AI and bioinformatics models, to calculate interaction scores between diseases, genes, and biological processes in real time. This helps identify target candidates for diseases or match the best indications for specific targets.
What specific program is highlighted as a success story in the use of AI for drug discovery?
-The program highlighted is TEIC for idiopathic pulmonary fibrosis (IPF), which was identified as the best target using the AI platform Panomics in 2019. It successfully moved through preclinical trials and into clinical phase 1 and 2 within 30 months.
How does the research relate aging to drug discovery?
-The research identifies therapeutic targets implicated in aging, as aging is seen as a driving factor for many diseases. The platform assesses how these targets are integrated into the hallmarks of aging to develop more effective treatments.
What is the significance of dual-purpose targets in this research?
-Dual-purpose targets refer to targets that can be used to treat both cancer and aging simultaneously. The research aims to identify such targets by examining shared biological pathways in cancer and aging.
What is the significance of KDM1A in the study?
-KDM1A is highlighted as a dual-purpose target, upregulated in 10 out of 11 solid tumors, and negatively associated with aging. Experiments show that knocking down KDM1A may increase lifespan, making it a promising target for both cancer and aging treatment.
What AI tool is used to design molecules targeting specific diseases?
-The AI tool 'Chemistry 42' is used to design novel molecules that target specific diseases, such as TEIC for IPF, after the targets are identified.
How does the AI platform facilitate drug repurposing?
-The AI platform facilitates drug repurposing by providing a list of the best indications for specific targets, allowing researchers to find new uses for existing drugs.
What collaboration is mentioned in the research regarding dual-purpose targets?
-The research mentions a collaboration with Evandro from the University of Oslo, focusing on identifying targets integrated in both cancer and aging, particularly across 11 common solid tumors.
Outlines
đŹ Introduction to Dual Purpose Target Discovery and GPT Models
Frank opens by thanking the introduction and expressing excitement about discussing the dual-purpose target discovery and agent research using GPT models. He highlights a paper published in 'Trends in Pharmacological Science' that covers how AI assists in drug discovery, specifically target discovery, indication expansion, and drug repurposing. He notes the increasing validation of AI-identified targets, mentioning that one of their targets has progressed to human clinical trials (Phase 2). Frank briefly introduces their approach to using multiomic data and bioinformatics models to identify targets for diseases or discover the best indications for known targets.
đĄ AI-Driven Target Discovery and Drug Repurposing Process
Frank dives deeper into their target discovery approach, using multiomic and tax data alongside 23 AI and bioinformatics models. These models calculate interactions between diseases, genes, and biological processes, allowing researchers to either start from a disease and find potential targets or start from a target and discover its best indications. He discusses a lead program, TEIC, identified as the best target for idiopathic pulmonary fibrosis (IPF) using their AI platform. The process, from identifying the target to designing new molecules and conducting preclinical and clinical experiments, has moved very quickly, with TEIC already in Phase 2 clinical trials in China and the US.
Mindmap
Keywords
đĄDual Purpose Target Discovery
đĄAI in Drug Discovery
đĄTarget Discovery
đĄIndication Expansion
đĄDrug Repurposing
đĄMultiomic Data
đĄHallmarks of Aging
đĄClinical Phase II
đĄKDM1A
đĄGenerative AI Chemistry
Highlights
Introduction of Dual Purpose Target Discovery and agent research using GPT models.
AI aids in drug discovery, including target discovery, indication expansion, and drug repurposing.
Paper chosen as Editor's Choice and published in Trans Pharmacological Science.
AI-identified targets validated in the lab, with one moving to human clinical phase two trials.
Use of multiomic and tax data, along with 23 AI and bioinformatics models, to calculate disease and gene interactions in real-time.
AI platform helps generate target candidates for diseases or identify best indications for specific targets.
Published paper on TEIC for IPF in Nature Tech Journal, showcasing AI's impact on drug discovery.
Identified TEIC as a target for IPF using the AI platform, with confirmation of its role in several hallmarks of aging.
Generative AI used to design novel molecules for the identified target, resulting in rapid progress to clinical trials.
TEIC progressed from target identification to clinical phase one within 30 months, a record pace.
AI identifies therapeutic targets linked to aging, with focus on multiple aging diseases.
Collaboration with University of Oslo researchers on cancer and aging treatment using AI.
Discovery of dual-purpose targets that address both cancer and aging simultaneously.
KDM1A identified as a promising dual-purpose target for cancer treatment and lifespan extension.
Successful RNA experiment in C. elegans showing knockdown of KDM1A increases lifespan, showcasing AI's role in identifying dual-purpose targets.
Transcripts
thank you very much for the introduction
of Alex and hello everyone uh I'm Frank
I'm really excited today uh to talk
about our work on the Dual Purpose
Target Discovery as well as the agent
research with our precious uh GPT
models um yes this is the paper that we
published last year in Trans in
pharmacological science that we talk
about how AI can help um in the drug
Discovery um including for example the
uh uh Target Discovery indication
expansion and Drug repurposing okay and
the paper was also chosen by the as the
addit choice last year so since 2020 we
can see that more and more AI identified
targets has been being validated with v
lab and one of the targets has been even
moved to the human clinical phase two
that is our teic targets so it's a very
uh exciting okay okay and then this is
our inal approach how we do the target
Discovery so basically we use multiomic
data and tax data um we use our 23 Ai
and bio informatics models to calculate
the score for the interactions of the
disease and genes and the biological
process in real time so that let's say
we can start from the disease of
interest and then the system will help
us to provide a list of of the target
candidates um for the best Target for
the disease or we can start from the
target of interest and then this to Pro
the system will provide a list of the
best indication for that particular
targets so that is the way how we do the
uh Target Discovery indication expansion
and drw
repurposing so early this year we
published a paper uh that is our lead
program the teique um for ipf on the
nature about Tech Journal so uh as far
as I know it is the most successful and
the most comprehensive story example to
show how AI can really help uh drug
Discovery so you can see that since
2019 we use our AI platform pandemics to
identify teic as the best Target for ipf
and since ipf you know the fibrosis is
kind of a age associate diseases so
that's why we also do the hmark of Aging
assessment it turns out teic has been
inegrated in several Hallmarks of Aging
for example the Ed uh intracellular
Communications and cellular
senz so once we confirmed the targets
and then we use our generative AI uh the
chemistry 42 to design a truly noal um
molecules to tackle the this Target and
then we went through a series of the uh
invitro inval uh preclinical experiment
and then we moved to the clinical within
30 months so this is an other industry
record U that it move very fast and now
we already finished the phase one and we
have moved to phase two in both China
and
us so since we identified teique in 2019
as the best Target for ipf and then we
truly believe uh finding the therapeutic
Targets implicated in aging is very
helpful so that's why we try to use our
panomics to very uh course effectively
to find the targets integrated in
multiple aging disease because we
believe that aging is the driving force
for most of the diseases and then we can
also see tenix is implicated in several
hormones of Aging here and uh after we
identify the targets for multi um age so
disase we further classify them uh into
different horor of
aging and then uh last year we building
on this momentum um we focus on whether
we can find the DU Pro Target uh for the
treatment of cancer and aging so that's
a very interesting so um we collaborate
with evandro from University of Oso to
focus on this study so basically we try
to identify the target um that is
commonly uh integrated in 11 solar
tumors um those are the common cancer
targets and then we also used the uh GTS
half RN SE data to find the targets
associated with aging and then
we um classify those Target into several
groups so you can see that the green
targets are those uh with evidence in
extending lifespan so here our
definition of the D Target is that uh
with the same direction of interaction
that we can achieve the goal for the
treatment of cancer at aging at the same
time so probably you know the best
example is the Amor uh the rber meing
example so however you can also see that
there's a over 100 U cancer targets that
do not have uh the known effect on
lifespan so next uh we are going to
propose uh some uh D propose targets at
that we propose tr3 of them and the most
interesting one is the KD M1A so from
this figure from panel a you can see
that KD M11 a is uppr regulated in 10
out of 11 solid tumors at the same time
uh kdm1a is negatively associated with h
i mean these Expressions uh so you can
see that kdm1 is is down regulated so uh
I'm not surprised because you know Mt
also have the similar situation so
that's why we want to see whether the
knockdown of kdm1a can really help to
incre increase the LIF span so that's um
we did the uh RNA uh Northern experiment
in the sea elegant and the result is
very impressive so it is a very good
example to show that how AI can
facilitate the identification of the DU
purose targets so uh yeah this is uh uh
the the story I want to share today and
now I will pass to my colleag
uh he will introduce a very interesting
uh gbd models thank you
Voir Plus de Vidéos Connexes
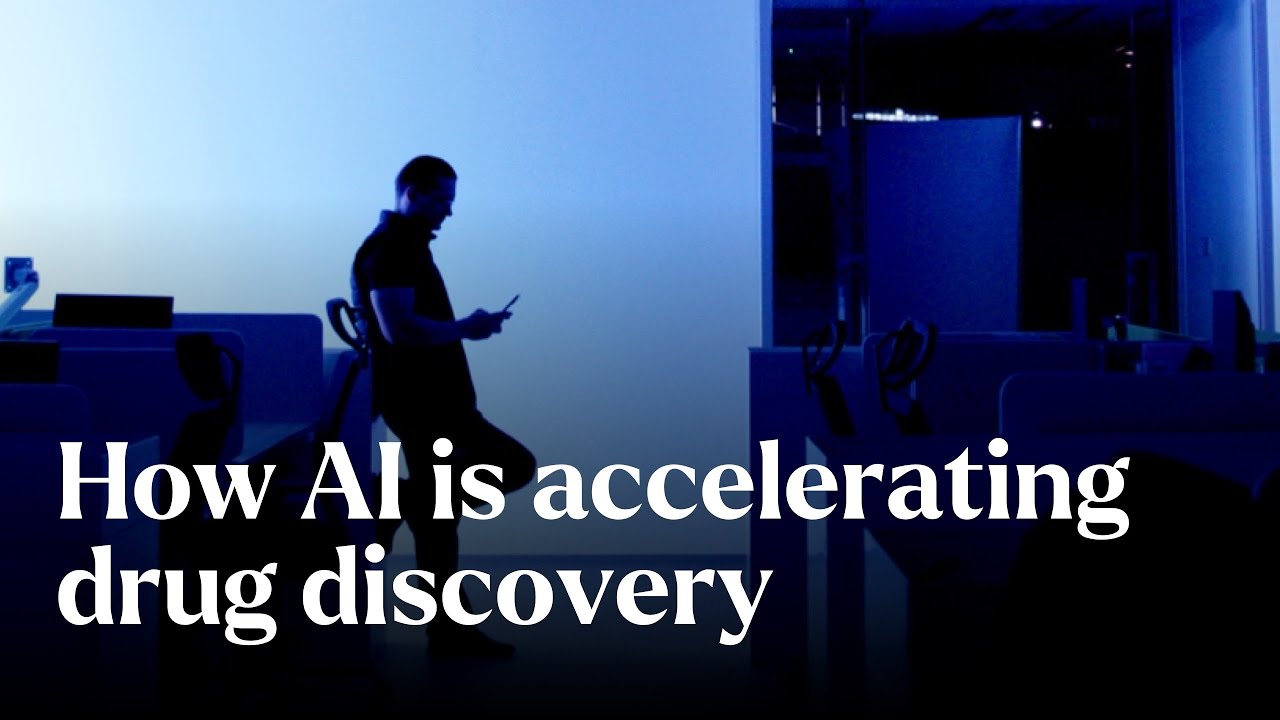
How AI is accelerating drug discovery - Nature's Building Blocks | BBC StoryWorks
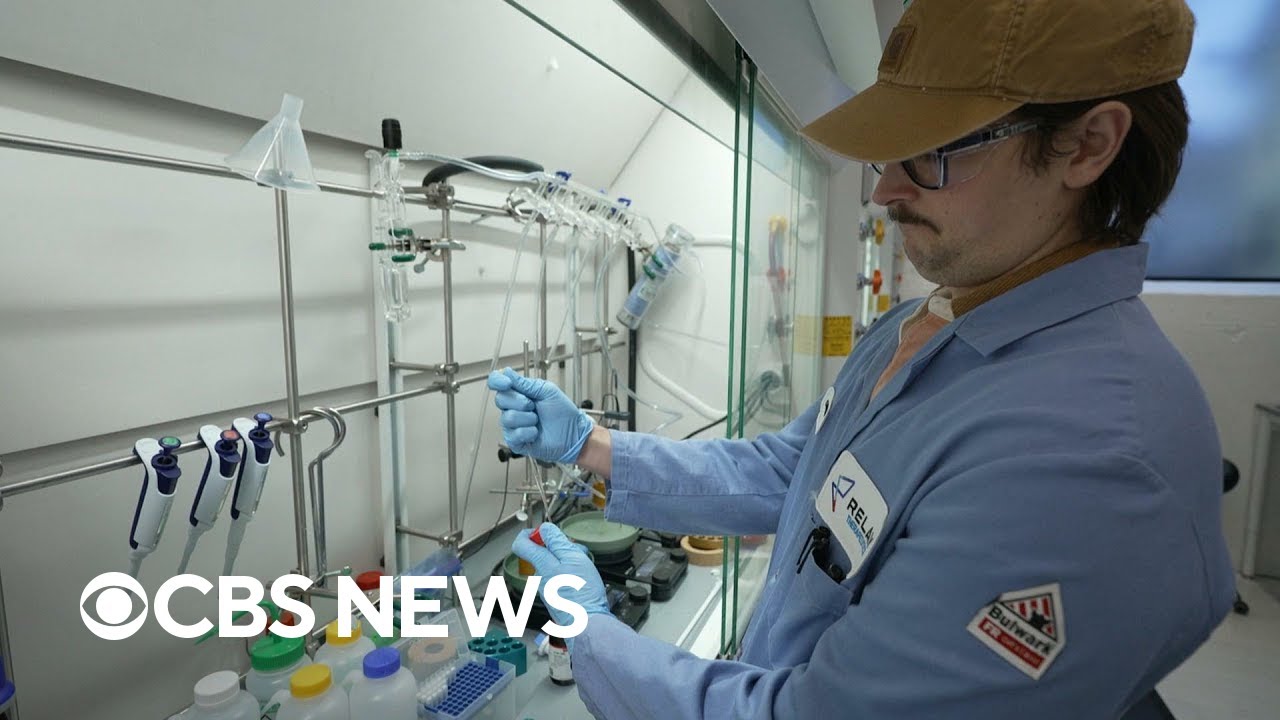
How AI might change medical care
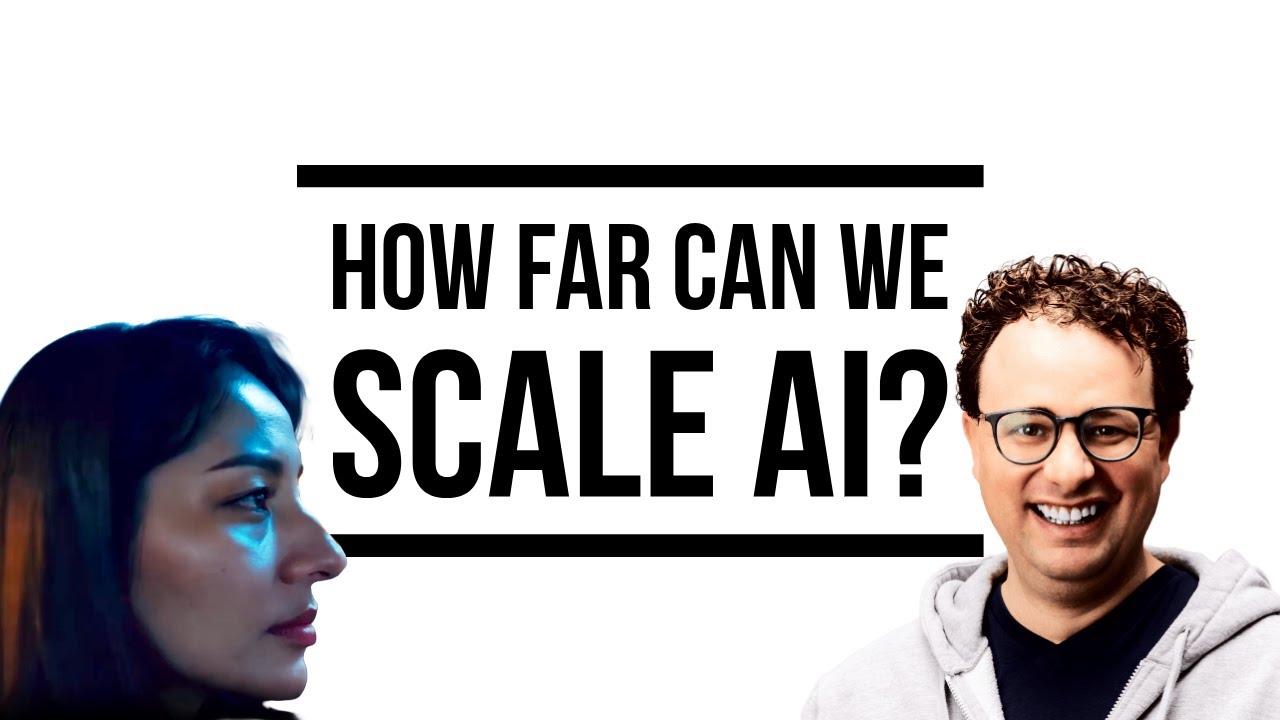
How Far Can We Scale AI? Gen 3, Claude 3.5 Sonnet and AI Hype
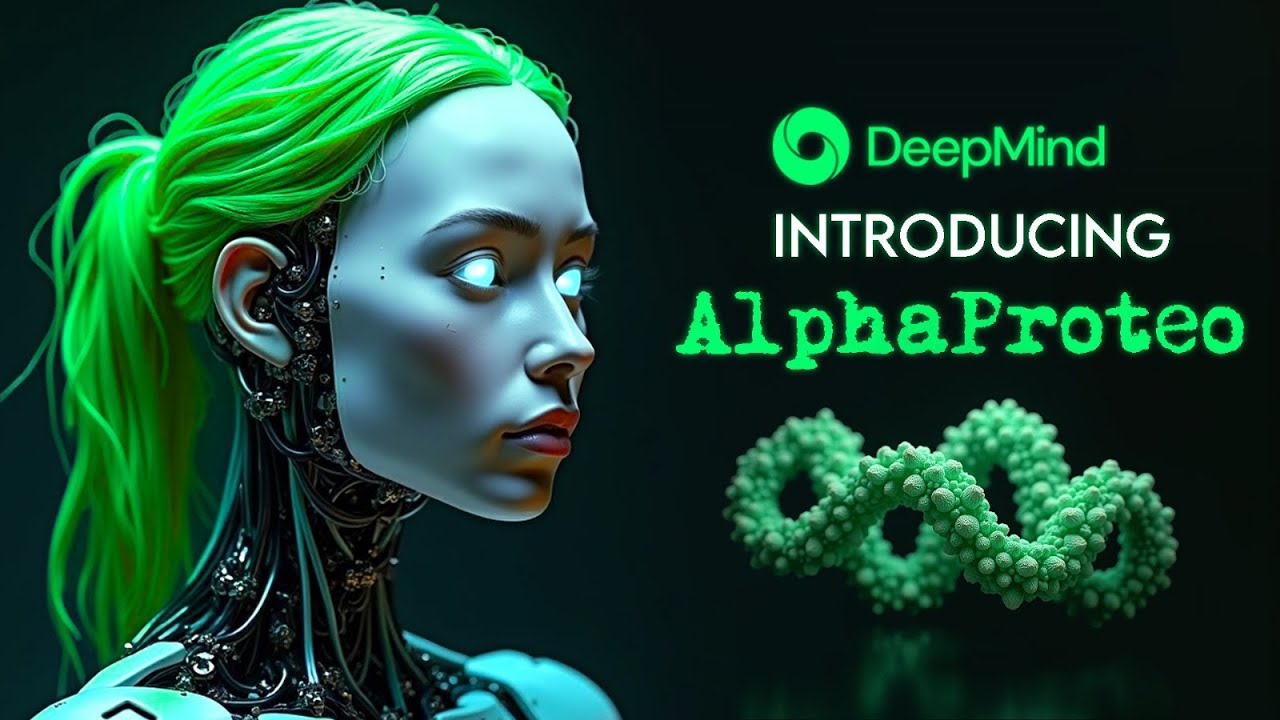
Google DeepMind's New AI Just Did in Minutes What Took Scientists Years

Introduction to pharmacology

Introduction to Drug Development & Regulation
5.0 / 5 (0 votes)