Think more rationally with Bayes’ rule | Steven Pinker
Summary
TLDRThe script discusses the concept of Bayesian reasoning, emphasizing the importance of evidence in belief calibration. It explains how posterior probability is calculated by considering prior beliefs, the likelihood of evidence given a hypothesis, and the overall commonness of the data. The talk also touches on the ethical dilemmas of applying Bayes' theorem in various life scenarios, such as fairness and avoiding perpetuation of stereotypes, and the need to discern when its application is appropriate.
Takeaways
- 🌟 Carl Sagan and David Hume emphasized the importance of extraordinary evidence for extraordinary claims, which is a Bayesian concept.
- 📚 Bayesian reasoning involves calibrating our degree of belief in something based on the strength of the evidence.
- 🧠 Bayes' theorem is conceptually simple, despite its intimidating appearance with symbols and letters.
- 🔍 Posterior probability is the updated belief in an idea after considering the evidence.
- 📈 The prior probability represents the initial belief in an idea before new evidence is considered.
- 🌱 The prior should be informed by all relevant knowledge, data, and established theories.
- 🌟 The likelihood is the probability of observing the evidence given that the hypothesis is true.
- 📊 The commonness of data, or the probability of the data, is a factor in updating beliefs according to Bayes' theorem.
- 🏥 An example of Bayesian reasoning in medicine is not diagnosing an exotic disease based on common symptoms without strong evidence.
- 🤔 There are ethical considerations in deciding when to apply Bayesian reasoning, such as in issues of fairness and avoiding perpetuation of stereotypes.
- 📝 Bayes' theorem is crucial in fields like journalism and social science for understanding the world, but its application must be carefully considered in other areas.
- 🚫 The dilemma of when to use Bayes' theorem and when it might be forbidden is a significant and sensitive issue.
Q & A
What is the famous saying by Carl Sagan that relates to the concept of evidence and claims?
-Carl Sagan's famous saying is 'Extraordinary claims require extraordinary evidence.' This means that the more unlikely or extraordinary a claim is, the higher the standard of evidence needed to support it.
What does David Hume's argument contribute to the discussion on belief and evidence?
-David Hume's argument questions the likelihood of the laws of the Universe being wrong versus someone misremembering something. It emphasizes the importance of considering the probability of events when evaluating evidence.
What is Bayesian reasoning, and how does it relate to the concept of belief?
-Bayesian reasoning is a method of updating the probabilities of hypotheses when given evidence. It involves assigning a degree of belief to a hypothesis before and after seeing evidence, rather than just believing or disbelieving it outright.
What are the components of Bayes' theorem as mentioned in the script?
-The components of Bayes' theorem mentioned are the posterior probability (credence after evidence), the prior (initial credence before evidence), the likelihood (probability of evidence given the hypothesis is true), and the probability of the data (how common the evidence is, regardless of the hypothesis).
How does the script explain the concept of 'prior' in the context of Bayes' theorem?
-The 'prior' in Bayes' theorem is the degree of belief in an idea before considering new evidence. It should be based on all relevant knowledge, past data, established theories, and anything that influences belief before the new evidence is taken into account.
What is the role of 'likelihood' in Bayes' theorem?
-The 'likelihood' in Bayes' theorem refers to the probability of observing the current evidence assuming the hypothesis is true. It helps in estimating how likely the observed evidence is, given the hypothesis.
Why is it important to consider the commonness of data when applying Bayes' theorem?
-Considering the commonness of data is important because it helps to normalize the product of the prior and the likelihood. It accounts for how often the evidence is expected to be observed, which is crucial for accurately updating beliefs based on the evidence.
What is the medical education cliche about hoof beats and zebras, and what does it illustrate?
-The cliche 'If you hear hoof beats outside the window, don't conclude that it's a zebra, it's much more likely to be a horse' illustrates the importance of considering Bayesian priors. It suggests that common causes are more likely than rare ones, even when the symptoms are similar.
In what areas of life should we apply Bayes' theorem according to the script?
-The script suggests applying Bayes' theorem in realms where we aim to be optimal statisticians, such as in understanding the world through journalism and social science. It also mentions the importance of considering fairness and avoiding perpetuation of disadvantage in other areas of life.
What dilemma does the script present regarding the use of Bayes' theorem?
-The dilemma presented is deciding when Bayes' theorem is permissible and when it is forbidden. It raises the question of balancing statistical prediction with other life values such as fairness and avoiding false accusations.
How does the script define the role of evidence in updating our beliefs according to Bayes' theorem?
-According to the script, Bayes' theorem states that our degree of belief in a hypothesis should be determined by how likely the hypothesis is beforehand and then scaled by the likelihood of the observed evidence, considering how common that evidence is, whether the hypothesis is true or false.
Outlines
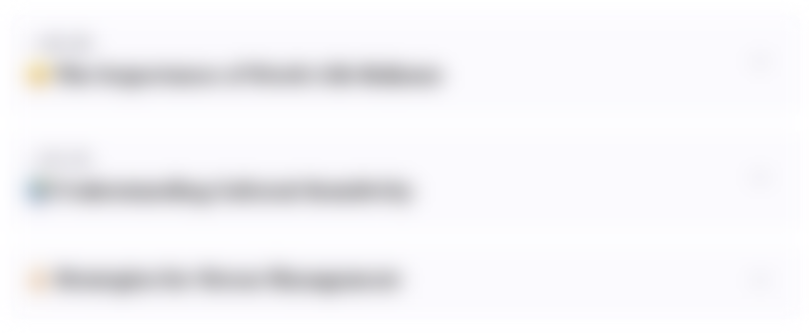
Esta sección está disponible solo para usuarios con suscripción. Por favor, mejora tu plan para acceder a esta parte.
Mejorar ahoraMindmap
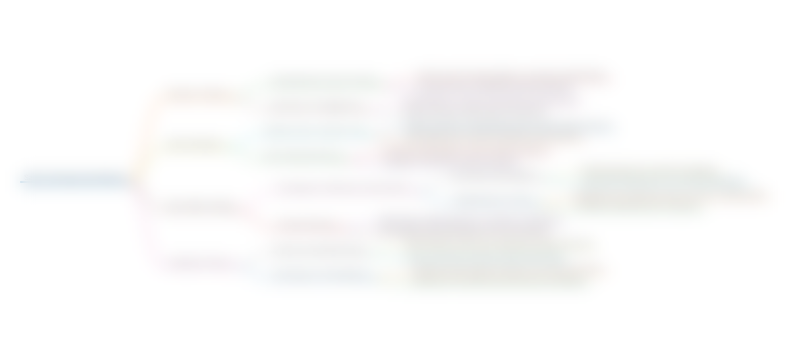
Esta sección está disponible solo para usuarios con suscripción. Por favor, mejora tu plan para acceder a esta parte.
Mejorar ahoraKeywords
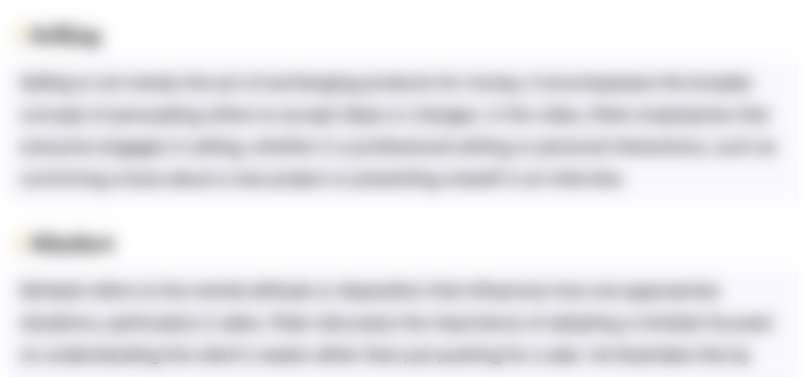
Esta sección está disponible solo para usuarios con suscripción. Por favor, mejora tu plan para acceder a esta parte.
Mejorar ahoraHighlights
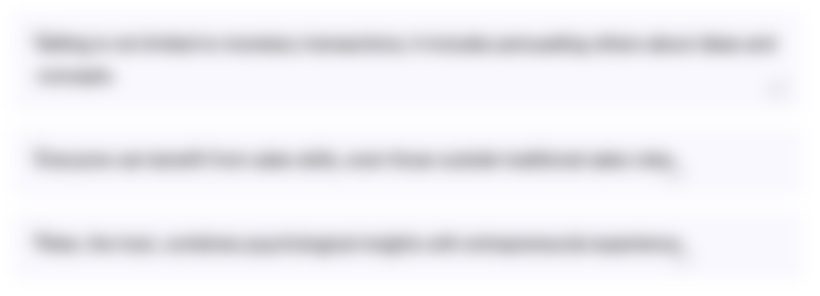
Esta sección está disponible solo para usuarios con suscripción. Por favor, mejora tu plan para acceder a esta parte.
Mejorar ahoraTranscripts
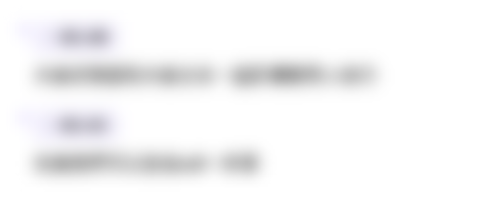
Esta sección está disponible solo para usuarios con suscripción. Por favor, mejora tu plan para acceder a esta parte.
Mejorar ahora5.0 / 5 (0 votes)