Principles For Human-Centered AI | Michael I Jordan (UC Berkeley)
Summary
TLDRThe speaker advocates for a market-driven approach to AI, emphasizing decision-making and economic principles over human imitation. They argue that intelligent systems should create value by connecting producers and consumers, like Amazon and Uber, rather than just mimicking human intelligence. The talk highlights the potential of machine learning in creating robust, enjoyable human experiences by focusing on market mechanisms and data-driven decision-making.
Takeaways
- 🚀 The future of business models should focus on becoming more like Netflix, Uber, or Amazon by leveraging intelligent systems and market mechanisms.
- 🤔 The term 'intelligent systems' is often used without a clear definition, prompting the need to look at Earth from a Martian perspective to identify what is truly intelligent.
- 🧠 Traditional AI has focused on mimicking human intelligence, but this may not be the most effective approach for creating robust and beneficial systems.
- 🏙️ Cities and markets demonstrate a form of intelligence through their ability to coordinate complex systems for the distribution of goods and services.
- 🛠️ The new frontier in computer science is not just about what happens inside the computer, but also how computers interact with the world through market mechanisms.
- 🚗 The concept of 'autonomous' vehicles is flawed; instead, vehicles should interact with a larger system, similar to air traffic control, for better coordination.
- 🔢 Machine learning has evolved from backend data analysis to customer-facing recommendation systems, and now to pattern recognition and decision-making.
- 💡 The speaker advocates for a shift in AI research from human imitation to creating systems that make better decisions in the context of markets and scarcity.
- 💼 Businesspeople should consider machine learning as having two parts: pattern recognition and decision-making, with the latter being crucial for creating value.
- 🎵 The music industry is an example where a market exists but is not fully monetized, suggesting opportunities for new business models that connect producers and consumers.
- 🌐 The potential for AI is vast, extending beyond traditional areas like music to art, cooking, and personal services, where connecting providers with consumers can create jobs and wealth.
Q & A
What is the main focus of the talk given in the transcript?
-The talk focuses on the business model of the future, discussing how to become a company like Netflix, Uber, or Amazon, and the importance of technical fields driving business strategic level thinking.
Why does the speaker suggest considering oneself as a Martian computer scientist?
-The speaker suggests this perspective to encourage out-of-the-box thinking and to look for inspiration for intelligent systems on Earth that could be mimicked or improved upon in computer algorithms.
What is the speaker's view on the current approach to artificial intelligence and its focus on imitating human intelligence?
-The speaker believes that the focus on imitating human intelligence is misguided and that a more promising approach involves creating market mechanisms and systems that interact with the world in a more integrated way.
What does the speaker consider as an example of intelligence on Earth that could inspire computer algorithms?
-The speaker considers the market systems, like the coordination of ingredients and supplies in a city's restaurants, as an example of intelligence that operates efficiently and could inspire computer algorithms.
Why does the speaker argue that the term 'autonomy' in the context of self-driving cars is incorrect?
-The speaker argues that 'autonomy' is incorrect because self-driving cars should not operate in isolation like humans but should interact with all other cars in a more integrated system, similar to air traffic control.
What is the speaker's opinion on the current state of machine learning and AI in terms of economic impact?
-The speaker believes that while machine learning and AI have had significant impacts, especially in backend operations and recommendation systems, the next generation of AI should focus more on decision-making and market mechanisms for greater economic value.
What is the concept of 'two-way markets' as mentioned by the speaker?
-The concept of 'two-way markets' refers to a system where both sides of a transaction can see and bid on each other, creating a more efficient and dynamic market that reduces noise and increases the likelihood of successful transactions.
How does the speaker suggest using recommendation systems in a new way to create value?
-The speaker suggests using recommendation systems not just to suggest items but to facilitate real-time bidding and transactions, such as recommending a customer to a restaurant and allowing the restaurant to offer a discount in return.
What is the speaker's view on the potential of market-based AI and its impact on jobs and the economy?
-The speaker believes that market-based AI has the potential to create jobs and generate wealth by connecting producers and consumers directly, rather than relying on advertising or other indirect monetization methods.
What are some of the technical problems the speaker is working on to advance market-based AI?
-The speaker is working on problems such as multiple decisions, markets under uncertainty, cloud-edge interactions, and provenance, which are essential for powering the next generation of market-based AI.
Can you provide an example of how the speaker suggests applying market-based AI to the music industry?
-The speaker suggests creating a platform that not only streams music but also provides data to musicians about their popularity in different cities, enabling them to negotiate performances and create a sustainable income, thus fixing the broken music market.
Outlines
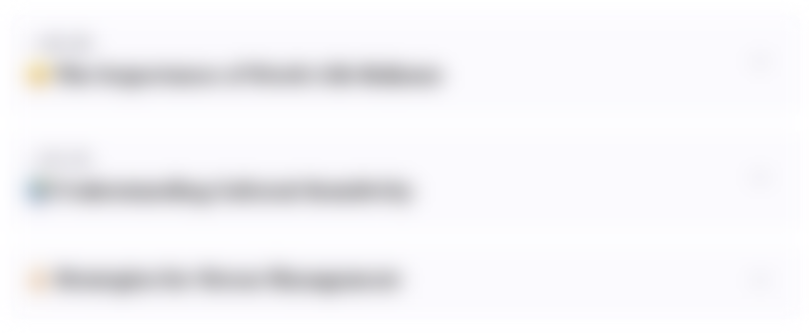
Esta sección está disponible solo para usuarios con suscripción. Por favor, mejora tu plan para acceder a esta parte.
Mejorar ahoraMindmap
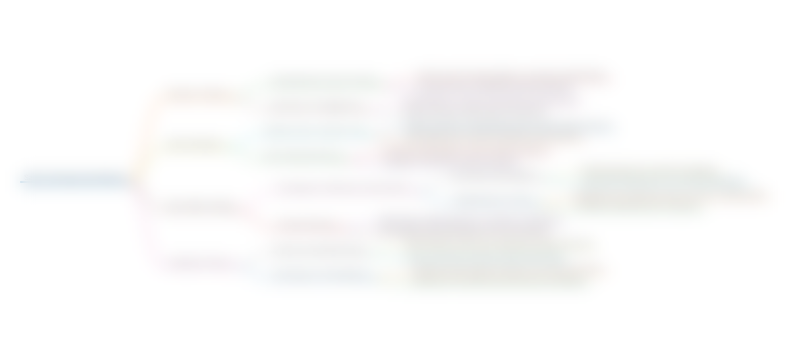
Esta sección está disponible solo para usuarios con suscripción. Por favor, mejora tu plan para acceder a esta parte.
Mejorar ahoraKeywords
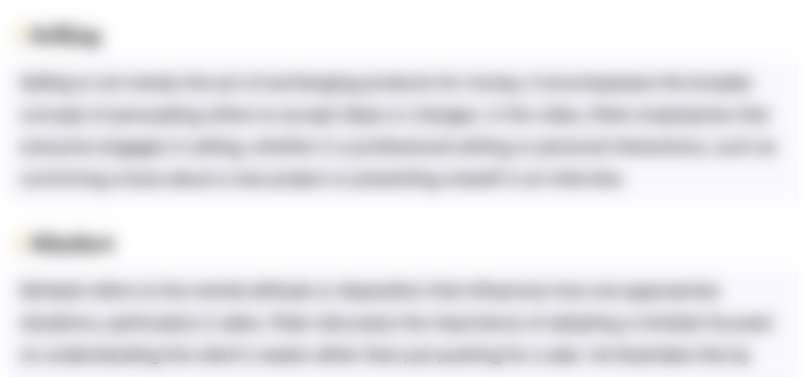
Esta sección está disponible solo para usuarios con suscripción. Por favor, mejora tu plan para acceder a esta parte.
Mejorar ahoraHighlights
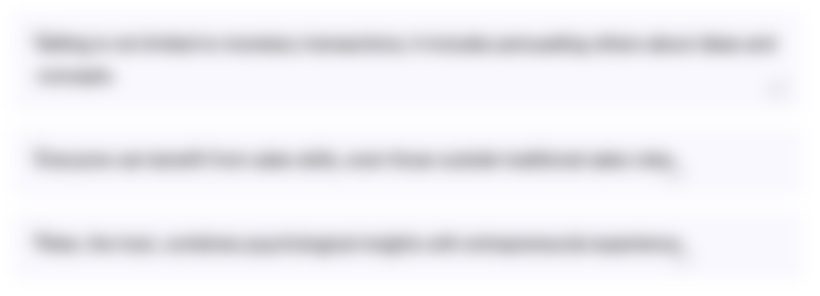
Esta sección está disponible solo para usuarios con suscripción. Por favor, mejora tu plan para acceder a esta parte.
Mejorar ahoraTranscripts
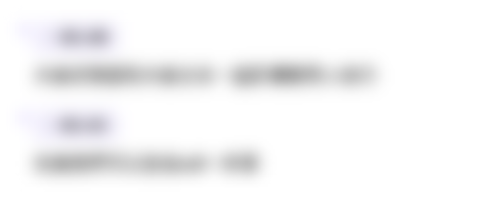
Esta sección está disponible solo para usuarios con suscripción. Por favor, mejora tu plan para acceder a esta parte.
Mejorar ahoraVer Más Videos Relacionados
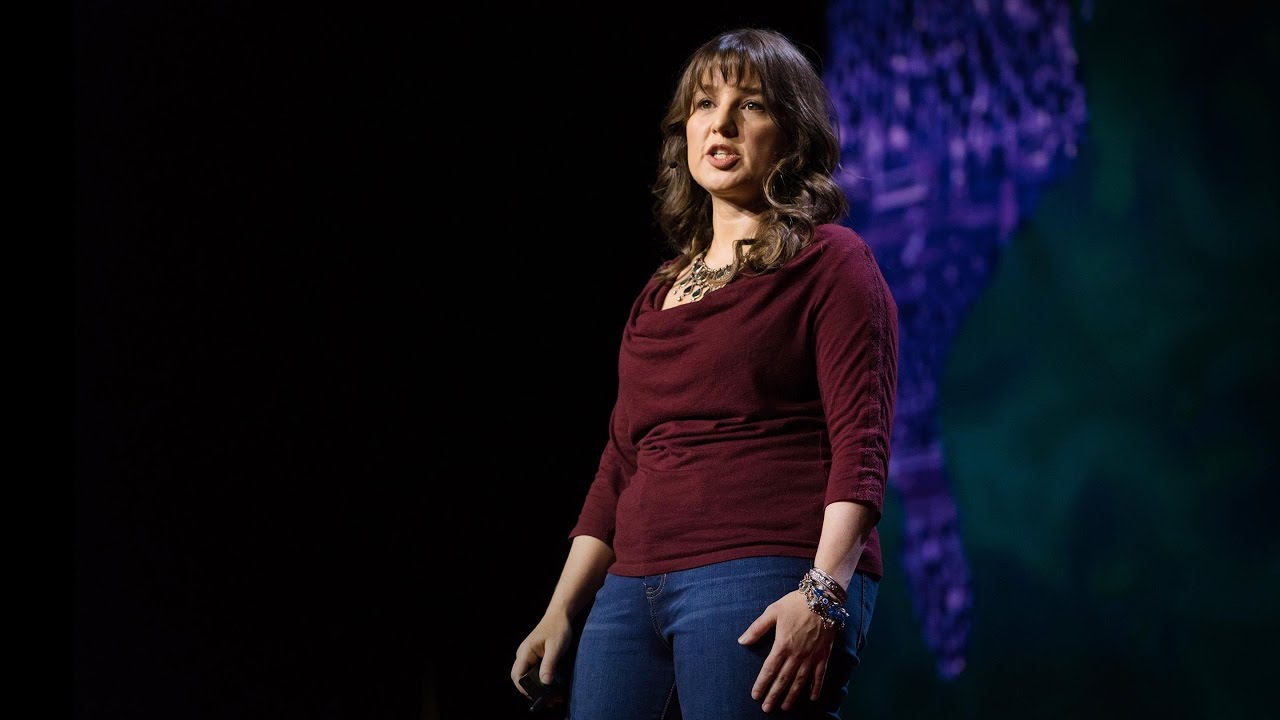
Machine intelligence makes human morals more important | Zeynep Tufekci
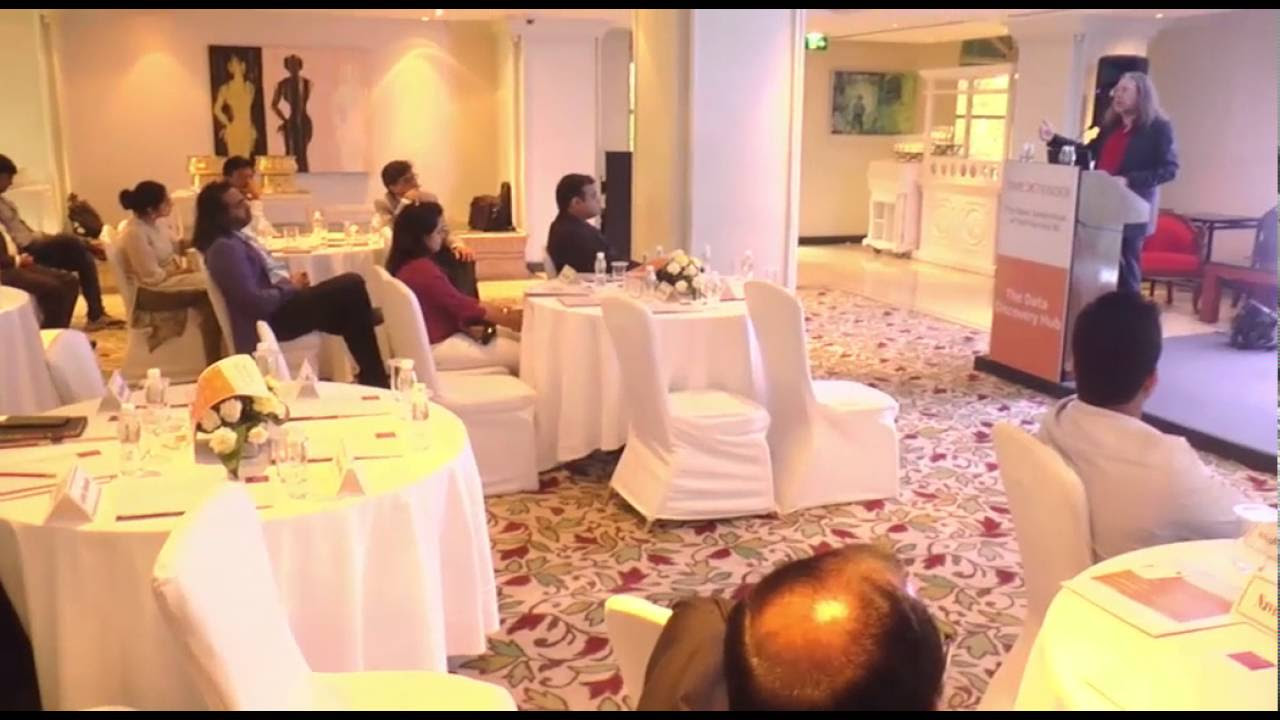
From Business Intelligence to Intelligent Business
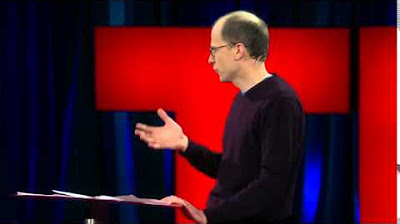
Nick Bostrom What happens when our computers get smarter than we are?

Barbara Gallavotti | Che cosa pensa l'Intelligenza artificiale
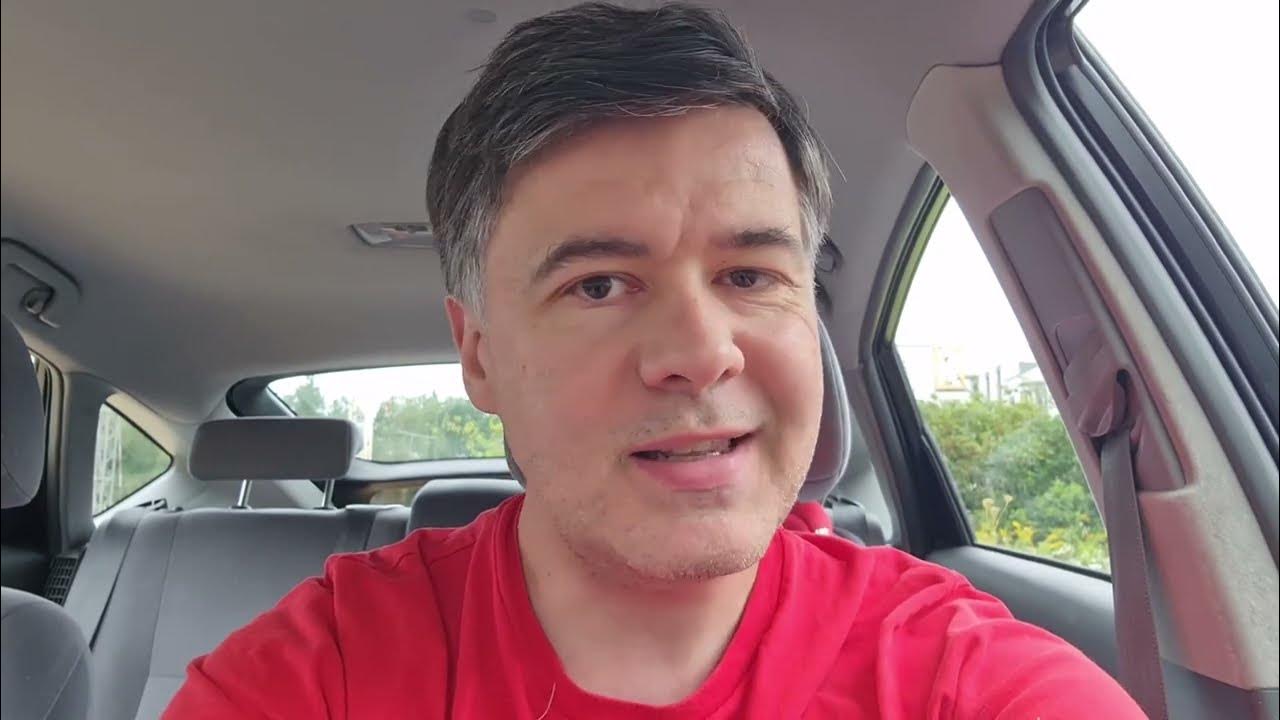
Dev Jobs are being taken. But not by AI.
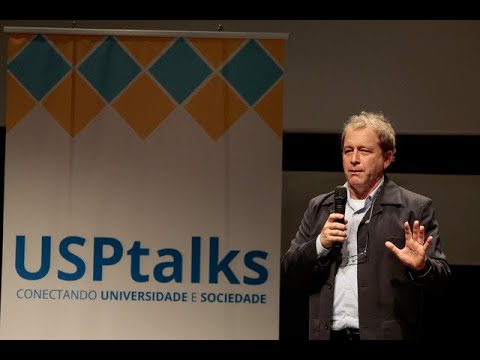
Implicações éticas e sociais da inteligência artificial - Marcelo Finger - USP Talks #48
5.0 / 5 (0 votes)