The Normal Distribution and the 68-95-99.7 Rule (5.2)
Summary
TLDREl video proporciona una introducción a la distribución normal y la regla 68-95-99.7, explicando la diferencia entre parámetros y estadísticas, y cómo estos describen datos de una muestra o población. Se describe la forma de la curva de la distribución normal, su tendencia a agruparse alrededor del valor central, y cómo el valor medio y la desviación estándar determinan su posición y dispersión. La regla 68-95-99.7 se utiliza para aproximar áreas bajo la curva, mostrando que la mayoría de los datos se encuentran cerca del promedio. El video incluye ejercicios prácticos para aplicar estos conceptos.
Takeaways
- 📊 La distribución normal es una curva de densidad de forma campana que describe la tendencia de los datos a agruparse alrededor de un valor central, que es la media de la población.
- 🔢 Los parámetros de una población, la media (μ) y la desviación estándar (Σ), son fundamentales para describir una distribución normal y determinan su posición y dispersión.
- 📈 La media de la población (mu) indica la posición de la distribución normal en el eje de los valores, mientras que la desviación estándar (Sigma) indica cuánto se dispersan los datos.
- 📚 La diferencia entre parámetro y estadística es crucial; los parámetros describen la población, mientras que las estadísticas describen la muestra.
- 📐 La normal分布 es simétrica respecto a su media y es unimodal, lo que significa que tiene un solo pico.
- 🌐 La distribución normal se utiliza para describir variables como el peso, la altura, el volumen, la presión arterial, entre otros, que a menudo se distribuyen de esta forma en la naturaleza.
- 📉 El cambio en la desviación estándar afecta la forma de la curva normal: una mayor desviación estándar hace que la curva se extienda más y sea más plana, mientras que una menor desviación estándar la hace más alta y estrecha.
- 🔢 El 68/95/99.7% regla indica que aproximadamente el 68% de los datos se encuentran dentro de un desviación estándar de la media, el 95% dentro de dos desviaciones estándar y el 99.7% dentro de tres desviaciones estándar.
- 📚 La regla 68/95/99.7 es una herramienta útil para aproximar áreas bajo la curva de una distribución normal y es aplicable a cualquier distribución normal, independientemente de su forma o tamaño.
- 📉 Aunque la distribución normal nunca toca el eje x y se extiende hasta el infinito, las áreas más allá de tres desviaciones estándar de la media son muy pequeñas.
- 📝 La notación para una población que sigue una distribución normal se escribe como X ~ N(μ, Σ), indicando que la variable X sigue una distribución normal con media μ y desviación estándar Σ.
Q & A
¿Qué es una distribución normal y por qué se le llama también curva de campana?
-Una distribución normal es un tipo especial de curva de densidad que tiene una forma de campana. Se le llama así porque describe la tendencia de los datos a agruparse alrededor de un valor central, que es la media de la población.
¿Cuál es la diferencia entre un parámetro y una estadística en el contexto de una distribución normal?
-Un parámetro es un número que describe los datos de una población, mientras que una estadística es un número que describe los datos de una muestra. Ejemplos de parámetros son la media de la población (mu) y la desviación estándar de la población (Sigma), y los ejemplos de estadísticas son la media de la muestra (x-bar) y la desviación estándar de la muestra (s).
¿Cómo afecta el valor de la media poblacional (mu) en la forma de la distribución normal?
-La media poblacional (mu) determina la posición de la distribución normal en el eje de los valores. Si aumenta el valor de mu, la curva se desplaza hacia la derecha, y si disminuye, la curva se desplaza hacia la izquierda.
¿Cómo se relaciona la desviación estándar poblacional (Sigma) con la dispersión de los datos en una distribución normal?
-La desviación estándar poblacional (Sigma) caracteriza la dispersión de los datos en una distribución normal. Cuanto mayor sea Sigma, más dispersos estarán los datos, y cuanto menor, menos dispersión habrá.
¿Qué significa la regla 68-95-99.7 en el contexto de una distribución normal?
-La regla 68-95-99.7 describe las áreas que contienen la mayoría de los datos en una distribución normal. Entre ±1 Sigma, hay aproximadamente el 68% de los datos; entre ±2 Sigmas, hay aproximadamente el 95%, y entre ±3 Sigmas, hay aproximadamente el 99.7%.
¿Por qué la distribución normal nunca toca el eje X, incluso si se desplaza más allá de tres desviaciones estándar?
-La distribución normal es una curva continua que nunca toca el eje X, ya que se extiende hacia el infinito en ambos lados. A pesar de que se puede desplazar más allá de tres desviaciones estándar, la área contenida en estas regiones es muy pequeña.
¿Cómo se notifica matemáticamente que una variable sigue una distribución normal con una media mu y una desviación estándar Sigma?
-Se escribe como X ~ N(mu, Sigma), lo que indica que la variable X sigue una distribución normal con una media de mu y una desviación estándar de Sigma.
¿Qué porcentaje de la población tiene una altura entre 5 y 6 pies si la altura sigue una distribución normal con una media de 5.5 pies y una desviación estándar de 0.5 pie?
-Dado que el rango de 5 a 6 pies está dentro de una desviación estándar de la media, según la regla 68-95-99.7, aproximadamente el 68% de la población caerá en este rango.
Si una distribución normal tiene una desviación estándar de 10, ¿cuál es el área contenida entre 70 y 90?
-Dado que el 95% de la población está dentro de dos desviaciones estándar de la media, y el rango de 70 a 90 es parte de ese intervalo, se puede calcular que el área de interés es aproximadamente el 47.5%.
¿Cuál es el área total contenida entre -2 y 1 en una distribución normal con una media de 0 y una desviación estándar de 1?
-Al dividir el intervalo en dos partes y aplicar la regla 68-95-99.7, se puede determinar que la área total contenida entre -2 y 1 es del 81.5%.
Outlines
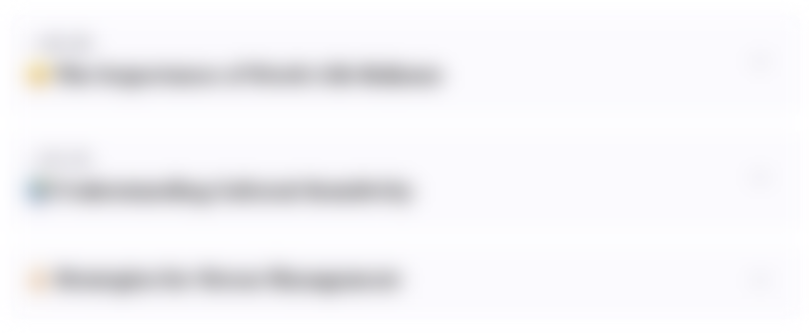
Esta sección está disponible solo para usuarios con suscripción. Por favor, mejora tu plan para acceder a esta parte.
Mejorar ahoraMindmap
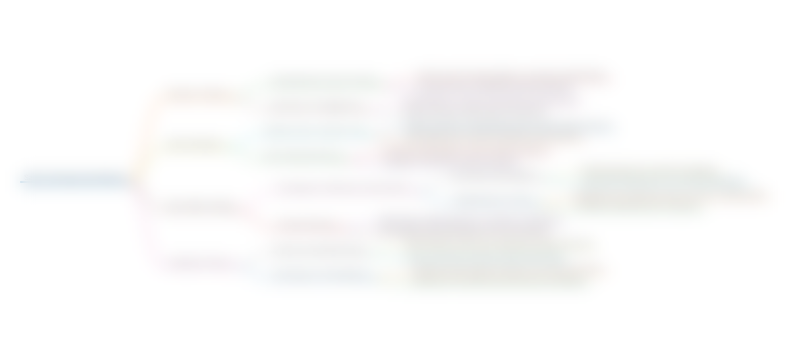
Esta sección está disponible solo para usuarios con suscripción. Por favor, mejora tu plan para acceder a esta parte.
Mejorar ahoraKeywords
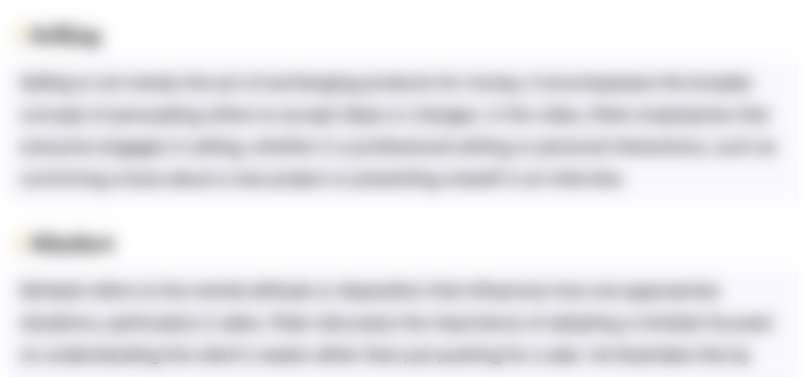
Esta sección está disponible solo para usuarios con suscripción. Por favor, mejora tu plan para acceder a esta parte.
Mejorar ahoraHighlights
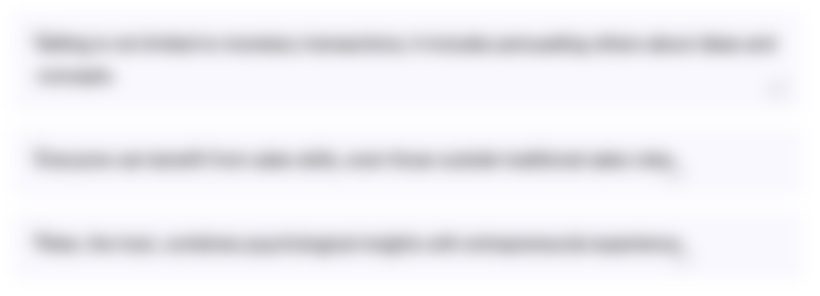
Esta sección está disponible solo para usuarios con suscripción. Por favor, mejora tu plan para acceder a esta parte.
Mejorar ahoraTranscripts
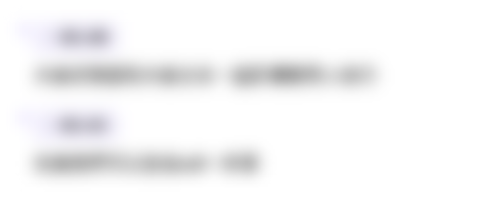
Esta sección está disponible solo para usuarios con suscripción. Por favor, mejora tu plan para acceder a esta parte.
Mejorar ahora5.0 / 5 (0 votes)