Easy 100% Local RAG Tutorial (Ollama) + Full Code
Summary
TLDREl guion del video muestra un tutorial sobre cómo crear un sistema RAG (Retrieval-Augmented Generation) sin conexión utilizando AMA y un modelo de lenguaje. El creador muestra cómo convertir un PDF en texto, crear embeddings y realizar consultas de búsqueda para obtener respuestas relevantes. Se ofrece un enlace a un repositorio de GitHub con instrucciones y código para configurar el sistema. El tutorial es fácil de seguir y se destaca por su sencillez y eficiencia, aunque no es perfecto, es adecuado para casos de uso personales.
Takeaways
- 📄 El script habla sobre cómo extraer información de un PDF utilizando un sistema local llamado 'ama'.
- 🔍 Se menciona que el proceso se realiza sin conexión a Internet, lo que implica que todo se ejecuta de manera local.
- 📝 El script fue convertido en un PDF y luego se extrajo el texto para su uso en la creación de 'embeddings'.
- 📖 Se destaca la importancia de tener los 'chunks' de texto en líneas separadas para un mejor funcionamiento del sistema.
- 🤖 Se utiliza un modelo llamado 'mral' para realizar consultas y obtener respuestas contextuales de los documentos.
- 💻 Se describe cómo configurar el sistema 'ama' en un ordenador usando Windows, incluyendo la instalación y la descarga del modelo.
- 🔗 Se proporciona un enlace en el repositorio de GitHub para seguir los pasos y configurar el sistema.
- 🛠️ Se sugiere realizar ajustes en el código para adaptar el sistema a diferentes necesidades, como cambiar el número de resultados mostrados.
- 🔧 Se menciona la posibilidad de ajustar el tamaño de los 'chunks' de texto para obtener mejores resultados.
- 📈 Se destaca la facilidad de configuración y la brevedad del código, que consta de aproximadamente 70 líneas.
- 🌐 Aunque el sistema es local y no requiere conexión a Internet, se admite que no es perfecto pero es suficiente para casos de uso personales.
Outlines
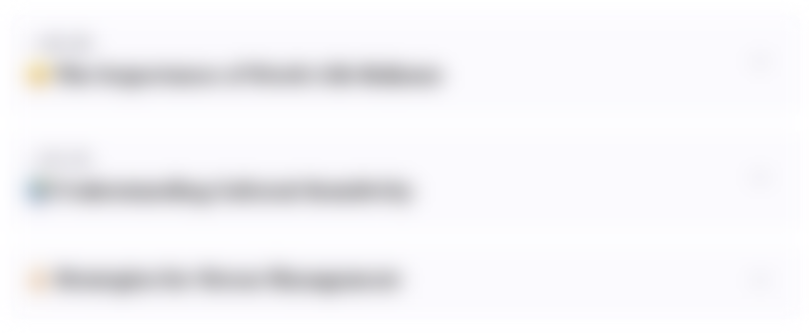
Esta sección está disponible solo para usuarios con suscripción. Por favor, mejora tu plan para acceder a esta parte.
Mejorar ahoraMindmap
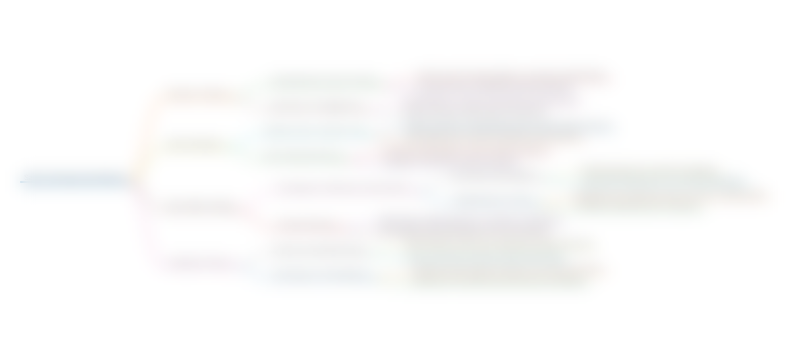
Esta sección está disponible solo para usuarios con suscripción. Por favor, mejora tu plan para acceder a esta parte.
Mejorar ahoraKeywords
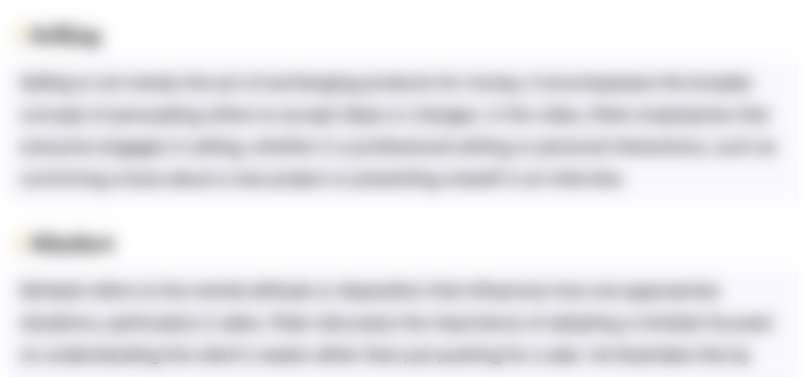
Esta sección está disponible solo para usuarios con suscripción. Por favor, mejora tu plan para acceder a esta parte.
Mejorar ahoraHighlights
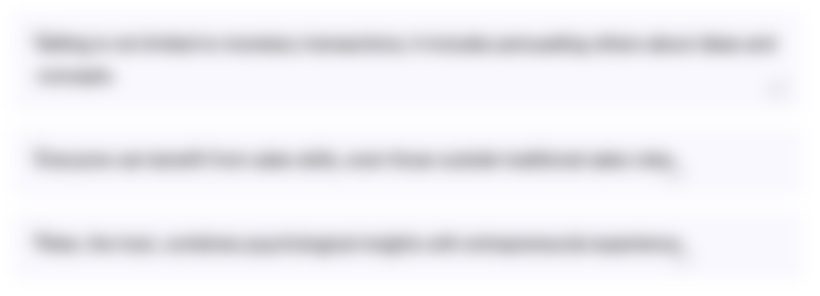
Esta sección está disponible solo para usuarios con suscripción. Por favor, mejora tu plan para acceder a esta parte.
Mejorar ahoraTranscripts
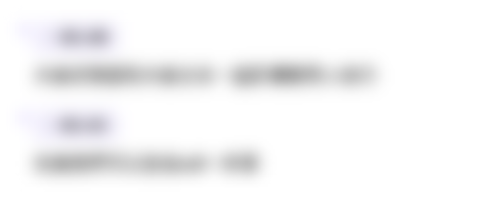
Esta sección está disponible solo para usuarios con suscripción. Por favor, mejora tu plan para acceder a esta parte.
Mejorar ahoraVer Más Videos Relacionados
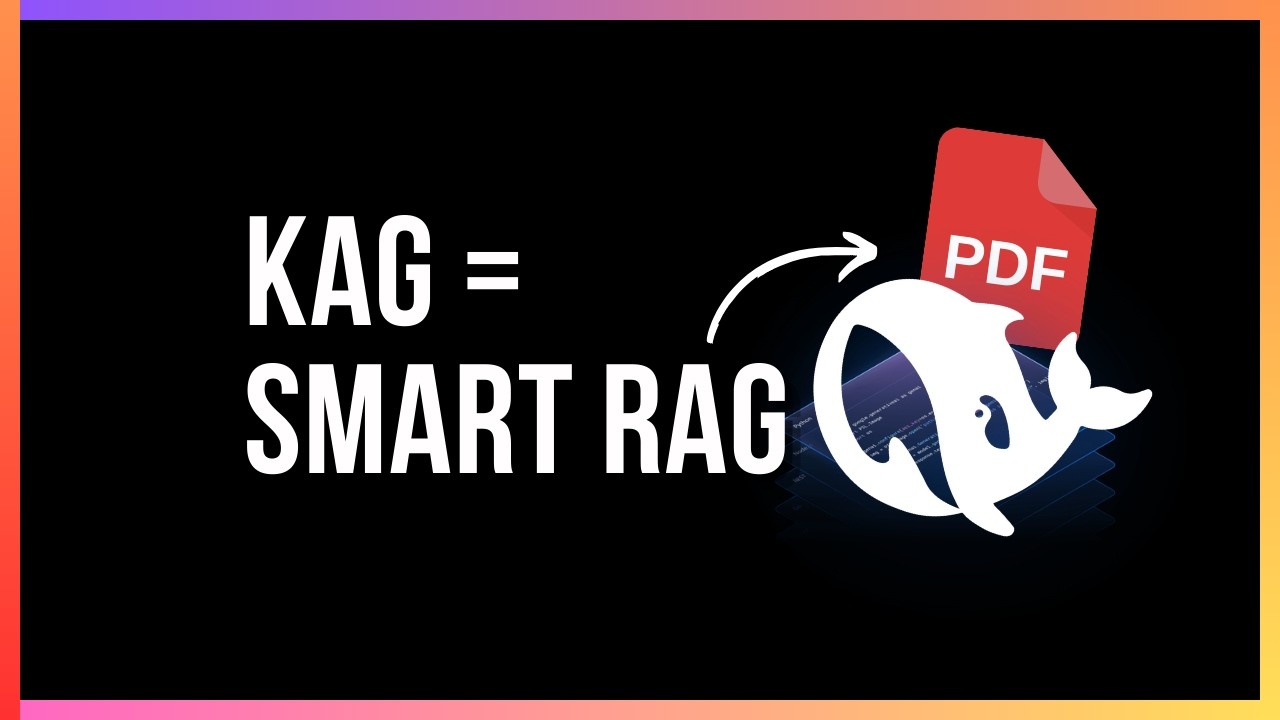
Meet KAG: Supercharging RAG Systems with Advanced Reasoning
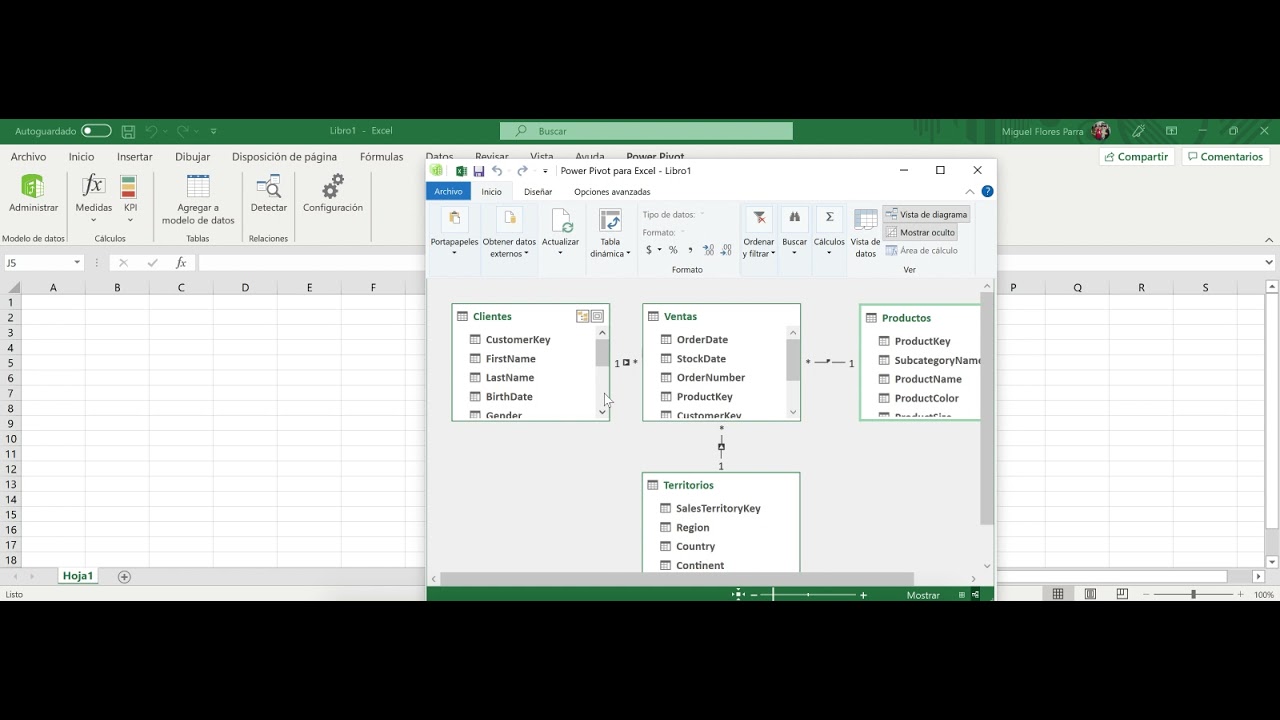
Video 7 Modelo Datos Tabla Dinamica

🌱 SISTEMA DE RIEGO CON ARDUINO UNO 🌱 | Paso a paso ✅ | Proyecto escolar
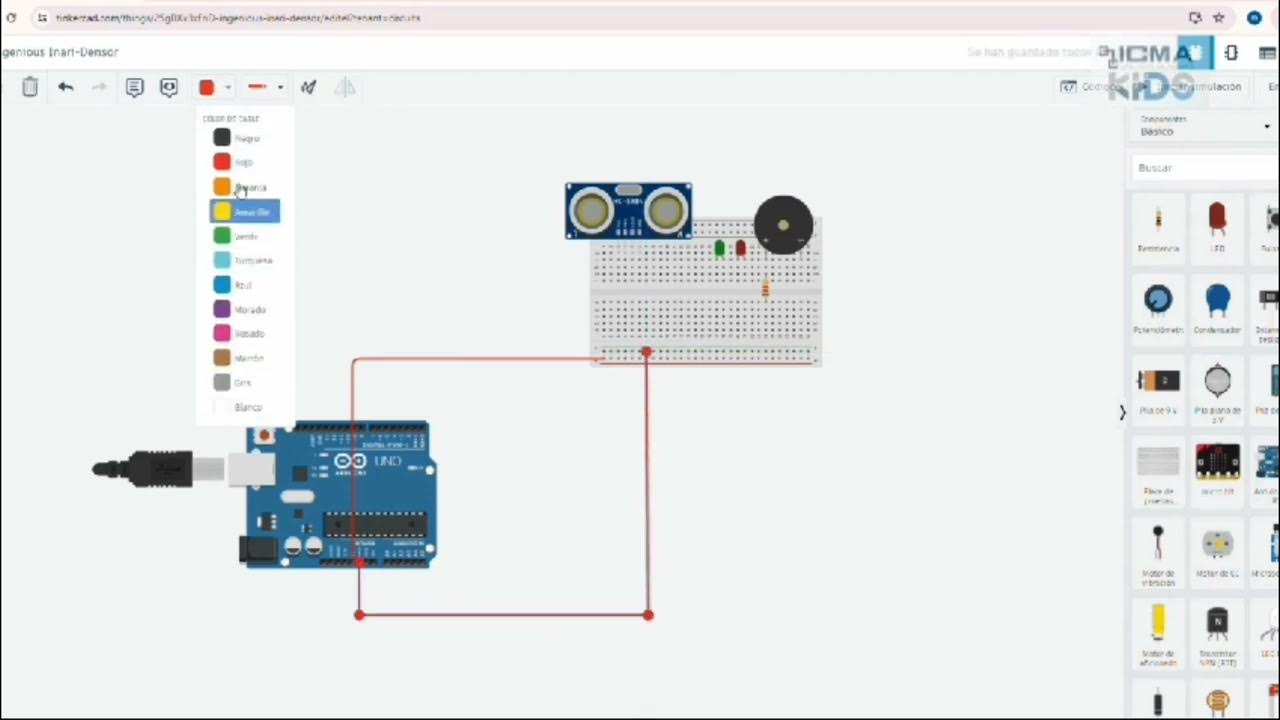
Cómo hacer un Sensor ultrasónico a 10 cm con ARDUINO | TINKERCAD | ICMA KIDS

Envía E-MAIL 📧 AUTOMÁTICO al crear registro | Tarea o acción automatizada SIN código Odoo

Cómo Hacer Un Sistema de Riego Automatico FÁCIL:🌱OLVÍDATE de REGAR🌱 | Ideal para Vacaciones CLHI
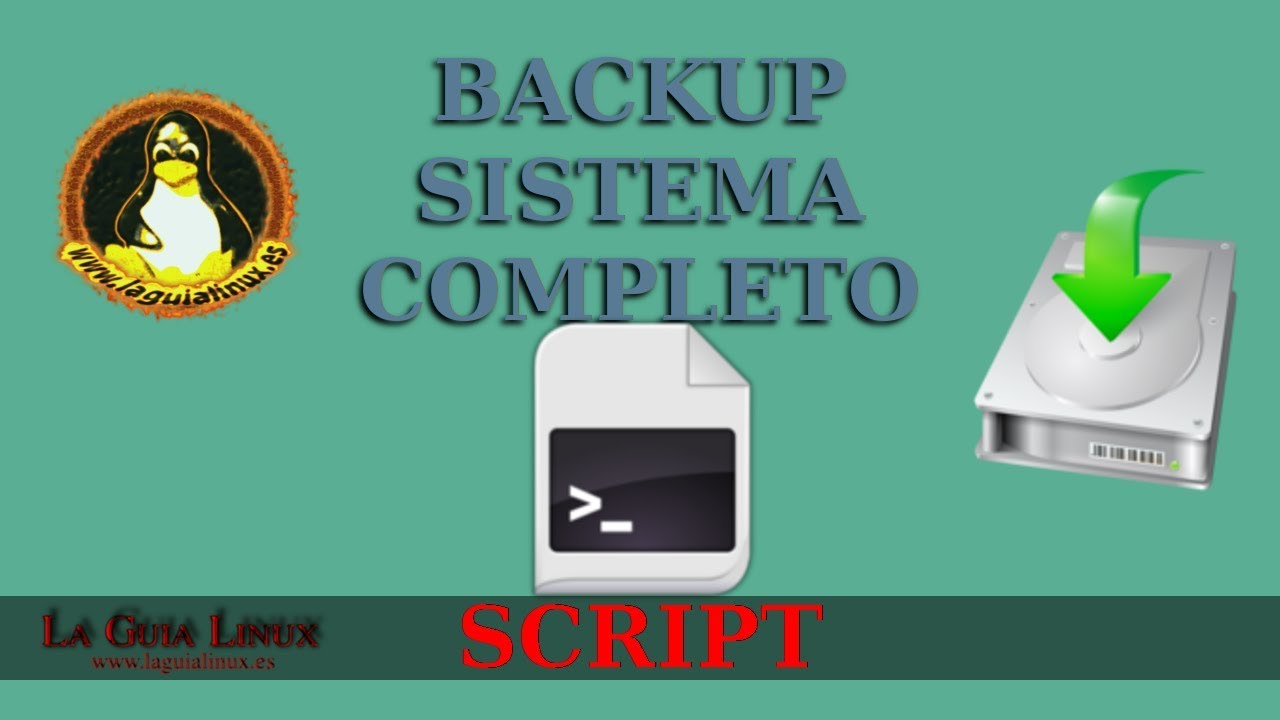
Script para crear Backup del sistema Linux Completo
5.0 / 5 (0 votes)