Google Research Unveils "Transformers 2.0" aka TITANS
Summary
TLDRGoogle's new Titans paper introduces a groundbreaking approach to AI memory by integrating long-term memory at test time. Unlike traditional Transformer models, which struggle with large context windows due to quadratic complexity, Titans mimics human memory by incorporating short-term, long-term, and persistent memory types. The model learns to memorize data at inference time, using a 'surprise mechanism' to prioritize important or unexpected events. This method allows Titans to scale beyond current models' limits, showing superior performance in tasks requiring long-context understanding, such as video analysis and time series forecasting.
Takeaways
- 😀 Titans is a new AI architecture introduced by Google Research, designed to solve memory limitations in Transformers during inference time.
- 😀 Transformers struggle with long context windows due to quadratic time and memory complexity, which Titans aims to overcome.
- 😀 Titans model allows learning to happen during test time (inference), not just during pre-training, enabling the model to adapt dynamically to new data.
- 😀 The Titans architecture incorporates multiple types of memory: short-term, long-term, and persistent memory, inspired by how the human brain works.
- 😀 A key feature of Titans is the 'surprise mechanism', which helps the model focus on and memorize unexpected events or inputs.
- 😀 The surprise metric in Titans measures how different an input is from the model's expectations, guiding what gets memorized.
- 😀 Titans include an adaptive forgetting mechanism that decides what to forget based on surprise and available memory, preventing overfitting.
- 😀 The architecture of Titans scales beyond the 2 million token context window limit of current models, improving performance with large context windows.
- 😀 Different memory integration approaches in Titans include memory as context, memory as gate, and memory as a layer, each with distinct trade-offs for efficiency and flexibility.
- 😀 Titans outperform other modern models in various benchmarks, particularly excelling in tasks requiring long context windows, such as needle-in-the-haystack retrieval tasks.
Q & A
What is the main goal of the Titans paper by Google Research?
-The main goal of the Titans paper is to overcome the limitations of transformers in handling long-term memory by introducing a memory architecture that updates during inference (test time) and is capable of managing large context windows effectively.
How do transformers struggle with long context windows?
-Transformers face quadratic time and memory complexity when processing long context windows. As the input grows larger, their performance deteriorates, especially when the context exceeds a certain limit, making them less effective for complex tasks that require handling large sequences of data.
What are the types of memory proposed in the Titans architecture?
-The Titans architecture introduces three types of memory: short-term memory (core memory), long-term memory, and persistent memory. Each type serves a different purpose in storing and processing information during the inference phase.
What is the 'surprise mechanism' in Titans, and how does it relate to human memory?
-The 'surprise mechanism' in Titans is inspired by human memory, where surprising events are more memorable. In the model, events that violate expectations are given higher priority for memorization. Over time, the model learns to prioritize more surprising events and forget less important ones, similar to how human memory works.
What is the purpose of memory decay in Titans?
-Memory decay in Titans serves to prevent the model from retaining unnecessary information over time. As certain events become less relevant, the model forgets them, allowing it to manage memory more efficiently, especially when dealing with very long sequences.
How does the Titans architecture manage memory at test time?
-Titans manages memory at test time by incorporating a long-term memory module that allows the model to store and update memories during inference. This enables the model to learn from inputs and adjust its memory based on surprising events, rather than relying solely on pre-trained knowledge.
What are the trade-offs between the three memory implementations in Titans?
-The three memory implementations in Titans—memory as context, memory as gate, and memory as a layer—each have distinct advantages. 'Memory as context' is best for tasks requiring historical context, 'memory as gate' is more flexible, switching between short-term and long-term focus, and 'memory as a layer' is the most efficient but slightly less powerful.
How do Titans perform compared to other models like GPT-4 and Gemini in long context tasks?
-Titans outperform models like GPT-4 and Gemini in tasks that require handling long context windows. In particular, Titans show more consistent performance when retrieving and managing information from large context windows, whereas other models struggle as the context length increases.
What is the 'needle in a haystack' task, and how do Titans excel at it?
-The 'needle in a haystack' task refers to the challenge of retrieving important information from a vast context, such as finding a relevant detail in a large sequence. Titans excel at this task by maintaining high accuracy even with very large context windows, unlike other models that experience a sharp drop in performance as context size increases.
What is the significance of the surprise metric in the Titans model?
-The surprise metric in Titans helps the model determine which events are more memorable. It is calculated based on how much an input deviates from the model's expectations. Larger deviations (surprises) are prioritized for memorization, allowing the model to focus on important and unexpected information.
Outlines
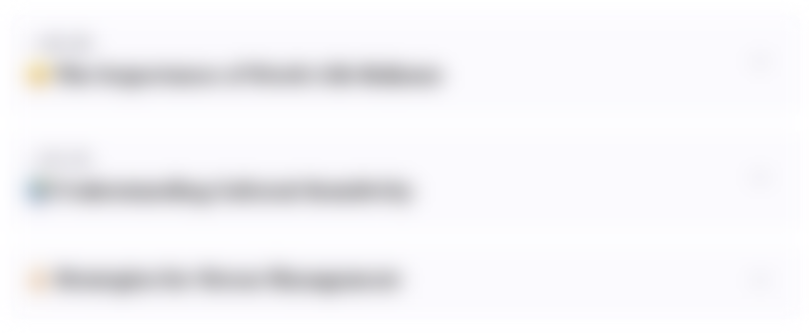
Esta sección está disponible solo para usuarios con suscripción. Por favor, mejora tu plan para acceder a esta parte.
Mejorar ahoraMindmap
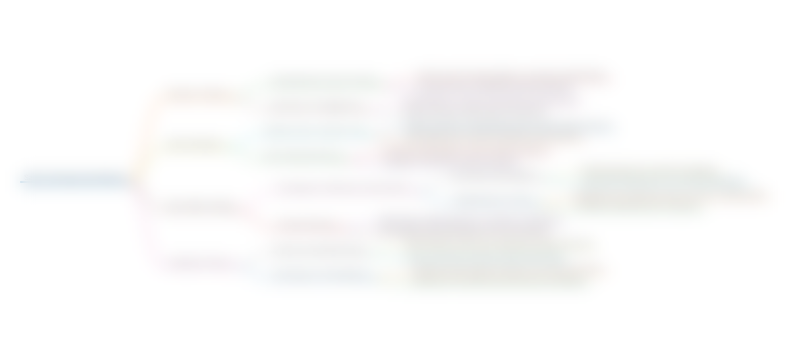
Esta sección está disponible solo para usuarios con suscripción. Por favor, mejora tu plan para acceder a esta parte.
Mejorar ahoraKeywords
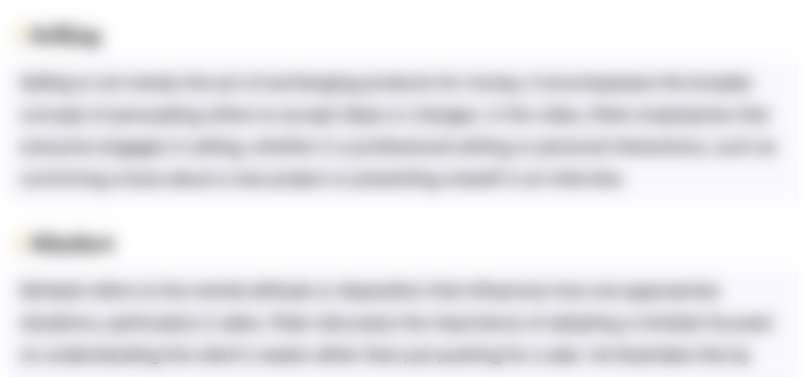
Esta sección está disponible solo para usuarios con suscripción. Por favor, mejora tu plan para acceder a esta parte.
Mejorar ahoraHighlights
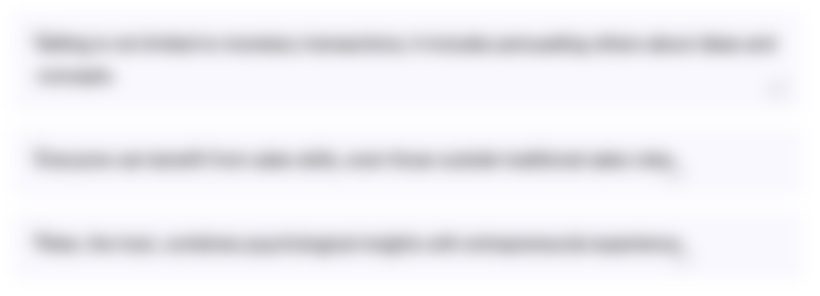
Esta sección está disponible solo para usuarios con suscripción. Por favor, mejora tu plan para acceder a esta parte.
Mejorar ahoraTranscripts
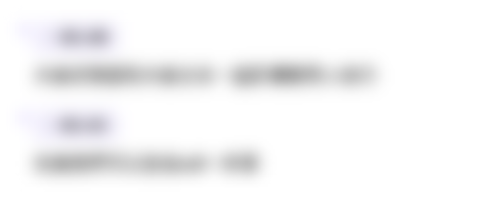
Esta sección está disponible solo para usuarios con suscripción. Por favor, mejora tu plan para acceder a esta parte.
Mejorar ahoraVer Más Videos Relacionados
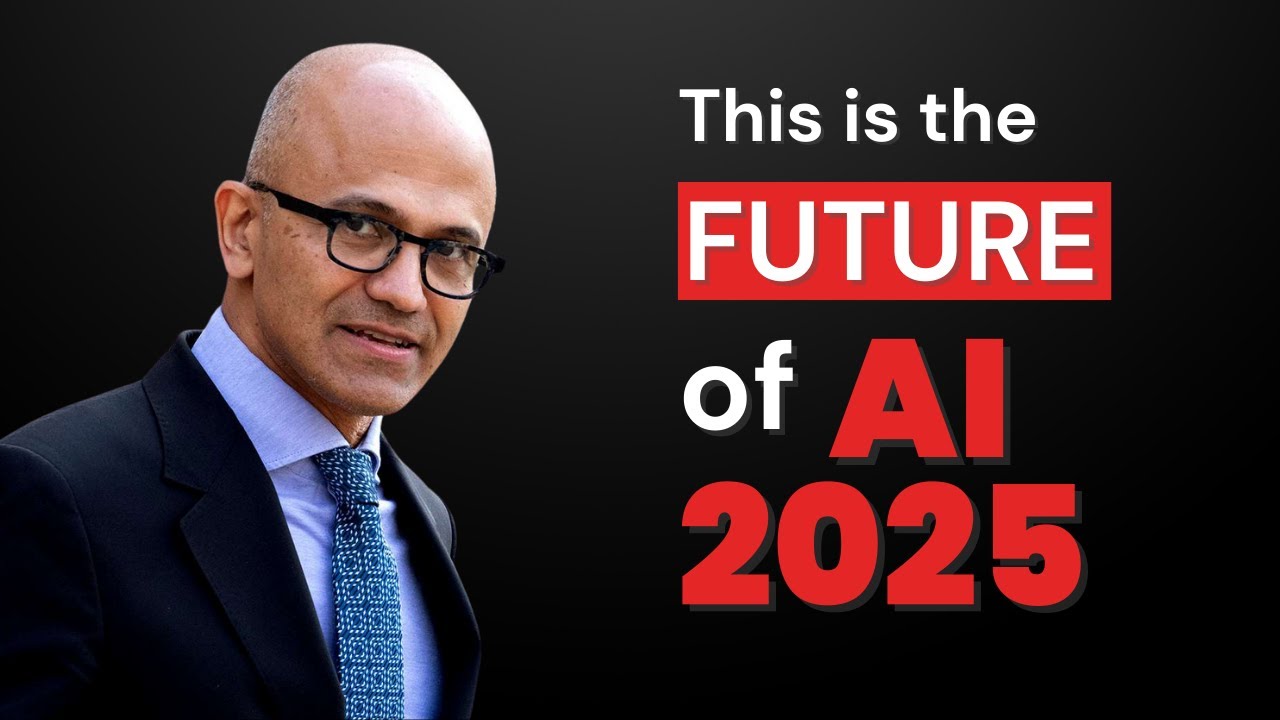
Microsoft And OpenAI Just Revealed The FUTURE Of AI
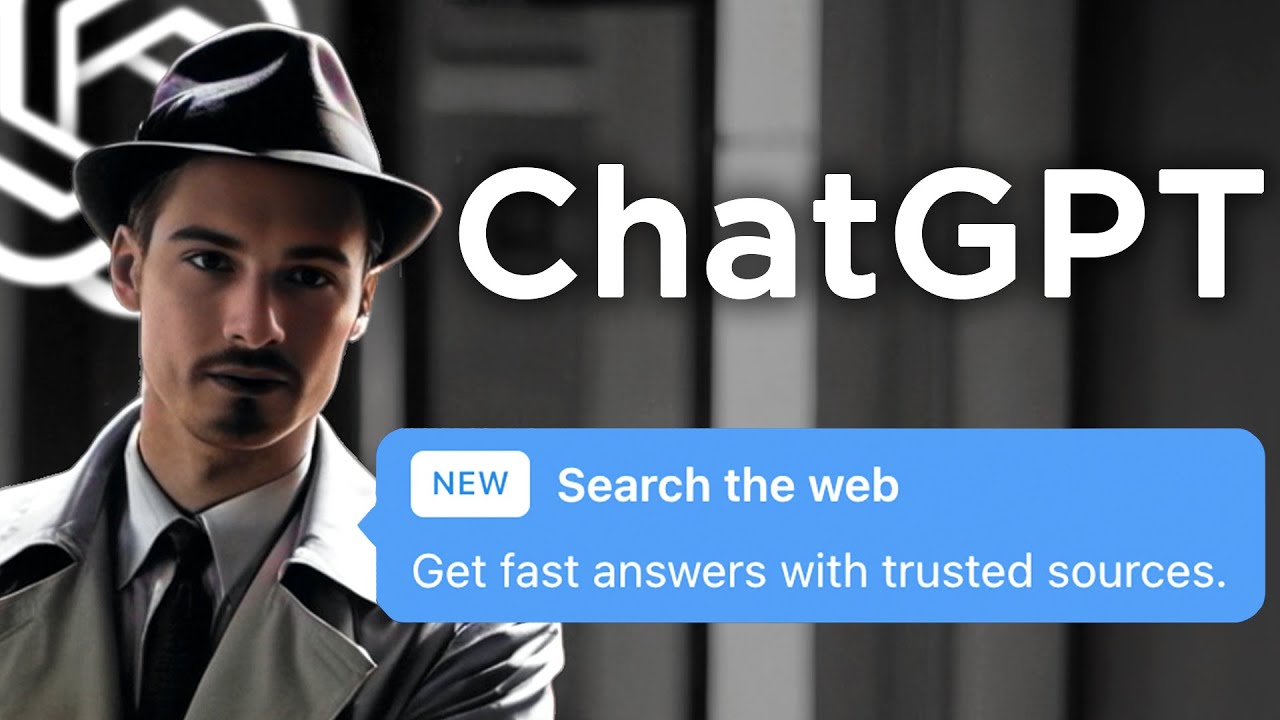
Biggest ChatGPT Upgrade, Google’s AI DJ & More AI Use Cases

松田語録:Googleの論文、Attention無限
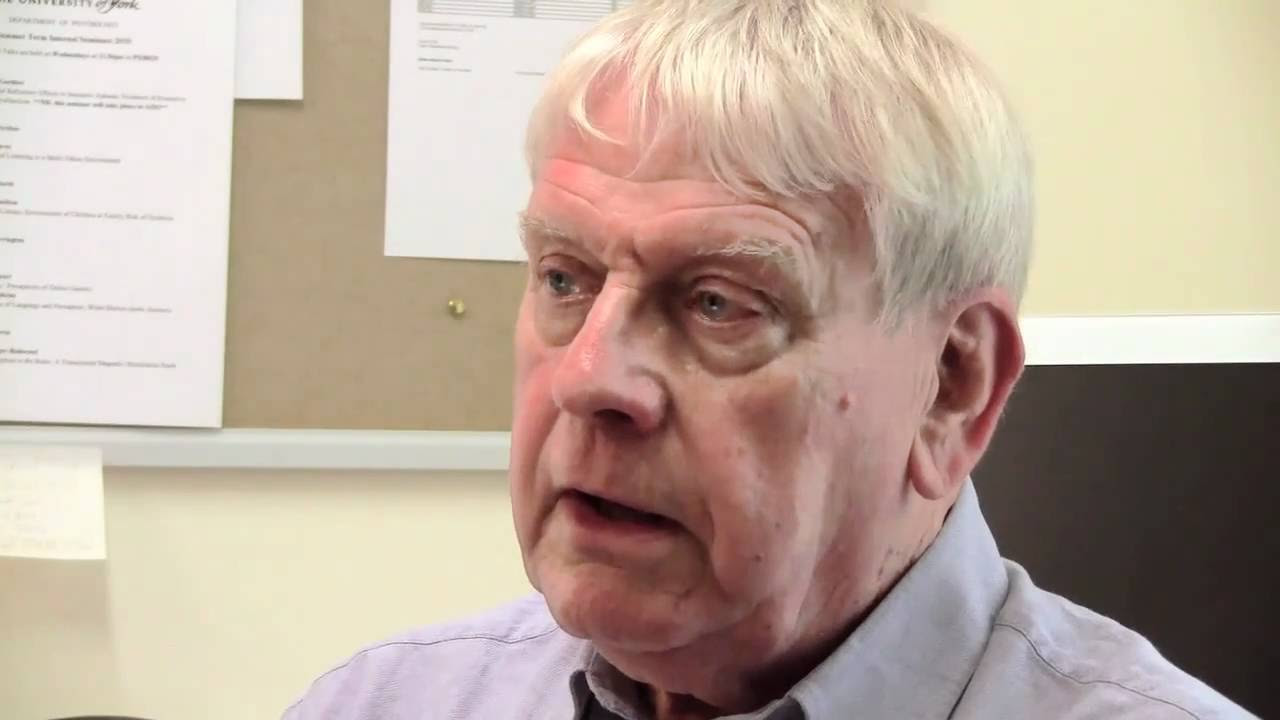
Alan Baddeley on the development of the working memory model
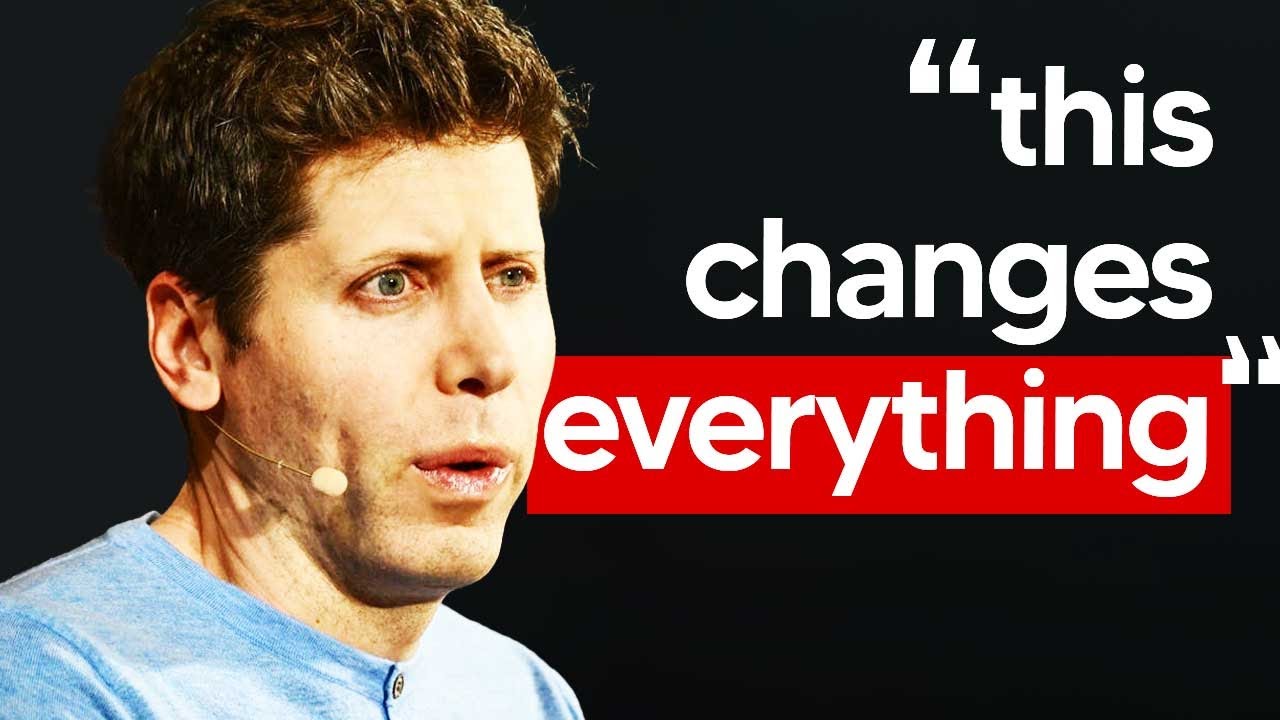
OpenAI And Microsoft Just Made A Game changing AI Breakthrough For 2025

Classification- Types of Learning and memory physiology | CNS physiology mbbs 1st year
5.0 / 5 (0 votes)