Has Generative AI Already Peaked? - Computerphile
Summary
TLDREl video discute la idea de que el uso de inteligencia artificial generativa para producir nuevas oraciones e imágenes, y su capacidad para entender imágenes y otros elementos, podría llevar a una inteligencia generalizada. Sin embargo, un nuevo artículo científico cuestiona esta teoría, argumentando que la cantidad de datos necesarios para lograr un rendimiento general de cero disparos en tareas nunca antes vistas sería astronómicamente grande, y posiblemente inalcanzable. El estudio examina el rendimiento de tareas secundarias, como la clasificación o recomendaciones, basadas en el uso de sistemas de empaquetado de CLIP, que usan grandes transformadores de visión y codificadores de texto. Los hallazgos sugieren que para problemas difíciles y conceptos poco representados en los conjuntos de datos, el modelo no será tan efectivo a menos que se cuente con una cantidad masiva de datos. Esto plantea un debate sobre la viabilidad de alcanzar una IA generalista a través del simple aumento de la cantidad de datos y modelos, y si en su lugar se requerirá una nueva estrategia o enfoque en la inteligencia artificial para mejorar el rendimiento en tareas complejas.
Takeaways
- 📈 La idea detrás de los modelos de inteligencia artificial generativa es que con suficientes pares de imágenes y texto, el modelo aprenderá a distilar lo que hay en una imagen en ese tipo de lenguaje.
- 🤖 Se ha argumentado que con la adición de más y más datos o modelos más grandes, eventualmente se alcanzará una inteligencia general o una IA extremadamente efectiva que funcione en todos los dominios.
- 🧪 Sin embargo, la ciencia no hipotetiza sobre lo que sucede, sino que justifica experimentalmente; por lo que cualquier afirmación de mejora continua debe ser comprobada empíricamente.
- 📉 Un reciente artículo sugiere que la cantidad de datos necesaria para lograr un rendimiento de cero disparos generales (tareas nunca antes vistas) es astronómicamente vasta y potencialmente imposible de alcanzar.
- 📚 Los modelos deClip embeddings utilizan un espacio compartido de embeddings para que las imágenes y el texto tengan una representación numérica similar, lo que se entrena a través de múltiples imágenes y textos.
- 🚀 Estas técnicas se han utilizado en tareas secundarias como la clasificación y recomendaciones, como en sistemas de recomendación de servicios de streaming.
- 🚧 El artículo demuestra que sin cantidades masivas de datos para respaldarlas, no es posible aplicar estas tareas secundarias de manera efectiva en problemas difíciles.
- 📉 Los hallazgos del artículo sugieren que el rendimiento en tareas de IA se vuelve logarítmico y se aplana con el aumento de los datos, lo que indica un posible punto de saturación.
- 🌳 La distribución de clases y conceptos dentro del conjunto de datos no es uniforme, lo que lleva a que algunos conceptos, como las especies de árboles específicas, estén muy subrepresentados.
- 🛠 Aunque los modelos grandes y la retroalimentación humana pueden mejorar el rendimiento, el artículo cuestiona si simplemente acumular más datos será suficiente para abordar tareas difíciles.
- ⚖️ El desafío es encontrar otras formas de abordar tareas difíciles que están subrepresentadas en los textos y búsquedas generales de Internet además de recolectar más datos.
- 🔮 Los avances futuros en IA dependerán de la capacidad de superar los límites actuales de los modelos de变压器 (Transformer) y de encontrar estrategias de aprendizaje automático más eficaces.
Q & A
¿Qué es un clip embedding y cómo se relaciona con la inteligencia artificial generativa?
-Un clip embedding es una representación numérica que encapsula el significado de una imagen y un texto, aprendida a partir de pares de imágenes y texto. Se utiliza en inteligencia artificial generativa para producir nuevas oraciones, imágenes, etc., y para entender la relación entre el lenguaje y las imágenes.
¿Por qué la idea detrás de los clip embeddings es que eventualmente se alcanzará una inteligencia general?
-La idea es que si se analizan suficientes pares de imágenes y texto, el modelo aprenderá a distilar la esencia de una imagen en un lenguaje similar. Con suficientes imágenes y texto, se espera que el modelo alcance un nivel de inteligencia general que le permita funcionar eficazmente en todos los dominios.
¿Qué argumenta la reciente investigación contra la posibilidad de una inteligencia general a través de la adición de más datos y modelos?
-La investigación sugiere que la cantidad de datos necesaria para lograr un rendimiento de cero disparos general (performance en nuevas tareas nunca vistas) es astronómicamente vasta, al punto de ser imposible de alcanzar con los recursos actuales.
¿Cómo se definen los conceptos en el estudio y cuál es su relación con la eficacia de las tareas downstream?
-Los conceptos se definen como ideas simples, como 'gato' o 'persona', o más complejas, como una especie específica de gato o una enfermedad. Se examinan 4,000 conceptos diferentes y se evalúa su prevalencia en conjuntos de datos, luego se prueba su rendimiento en tareas downstream como la clasificación cero disparos o sistemas de recomendación.
¿Qué hallazgos muestra la investigación en cuanto a la relación entre la cantidad de datos y el rendimiento en tareas downstream?
-La investigación muestra que la relación no es lineal ni exponencial, sino logarítmica, lo que significa que a medida que se agregan más datos, los incrementos en el rendimiento se vuelven menos significativos, hasta alcanzar un punto de platillo.
¿Por qué los sistemas de recomendación como Spotify o Netflix podrían beneficiarse de los clip embeddings?
-Porque los clip embeddings pueden generar un espacio compartido de representación para imágenes y texto. Utilizando esta representación, podrían recomendar programas basados en la similitud de sus embeddings con los programas que el usuario ha visto previamente.
¿Cómo afecta la distribución irregular de clases y conceptos en un conjunto de datos la capacidad de un modelo para realizar tareas difíciles?
-La distribución irregular conduce a una sobre-representación de ciertos conceptos y una sub-representación de otros, lo que hace que el modelo tenga un peor desempeño en las tareas relacionadas con los conceptos poco representados, al no haber suficientes datos para entrenar el modelo en ellos.
¿Qué sucede cuando un modelo de lenguaje grande es preguntado sobre un tema poco representado en su conjunto de entrenamiento?
-El modelo comienza a crear respuestas que son menos precisas y empieza a 'halucinar', es decir, a generar información que no está bien soportada por los datos de entrenamiento, lo que degrada su rendimiento.
¿Qué implicaciones tiene el hallazgo de que la adición de más datos y modelos no mejora significativamente el rendimiento para tareas difíciles?
-Implica que para mejorar el rendimiento en tareas difíciles, es necesario encontrar nuevas estrategias de aprendizaje automático o nuevas formas de representar los datos que superen los límites actuales de los modelos basados en Transformers.
¿Cuál es la sugerencia del hablante para mejorar el rendimiento en tareas difíciles que están sub-representadas en los conjuntos de datos?
-La sugerencia es que en lugar de simplemente recopilar más y más datos, se debe encontrar otras formas de abordar estas tareas difíciles, posiblemente utilizando técnicas de aprendizaje automático más avanzadas o estrategias de modelado de datos diferentes.
¿Por qué podría ser ineficiente continuar aumentando la cantidad de datos y el tamaño de los modelos para mejorar el rendimiento en tareas específicas?
-Puede ser ineficiente debido a que hay un punto de retorno decreciente donde el costo de adición de más datos y aumento del tamaño del modelo supera los beneficios en términos de mejora del rendimiento, especialmente cuando se trata de conceptos sub-representados en los conjuntos de datos actuales.
Outlines
🤖 Generative AI y su potencial en la inteligencia artificial
El primer párrafo discute la utilización de la inteligencia artificial generativa para crear oraciones y imágenes nuevas. Se explora la idea de que al analizar suficientes pares de imágenes y texto, el AI podría aprender a convertir lo que hay en una imagen en un lenguaje similar. Además, se cuestiona la creencia de que con la adición de más datos y modelos más grandes, la IA alcanzará una inteligencia generalizada. Se menciona un estudio reciente que argumenta lo contrario, es decir, que la cantidad de datos necesaria para lograr un rendimiento general de cero disparos es astronómicamente grande y posiblemente inalcanzable.
📈 Análisis de datos y conceptos clave en la IA
El segundo párrafo se enfoca en el análisis de datos y conceptos clave en la IA. Se definen conceptos simples y se examina su prevalencia en conjuntos de datos. Luego, se evalúa el rendimiento de tareas descendentes, como la clasificación de cero disparos o sistemas de recomendación, en función de la cantidad de datos disponibles para cada concepto. Se grafica la relación entre el número de ejemplos en el conjunto de entrenamiento y el rendimiento en la tarea, mostrando que el rendimiento tiende a nivelarse a pesar del aumento en la cantidad de datos, lo que sugiere un posible punto de inflexión en la mejora de la IA.
🌐 Dificultades y soluciones en la representación de datos en la IA
El tercer párrafo aborda las dificultades de representar ciertos objetos o conceptos en la IA debido a su bajo representatividad en los conjuntos de datos de entrenamiento. Se da ejemplos de cómo los modelos de IA pueden tener un rendimiento inferior al solicitarles tareas complejas que no están ampliamente representadas en los datos con los que fueron entrenados. Se argumenta que para mejorar el rendimiento en tareas difíciles, se requerirá encontrar nuevas formas de representar los datos o nuevas estrategias de aprendizaje automático. Además, se menciona el potencial de las empresas con más recursos para mejorar los modelos a través de la retroalimentación humana y otros métodos.
Mindmap
Keywords
💡clip embeddings
💡generative AI
💡Vision Transformer
💡text encoder
💡zero shot performance
💡data set
💡recommender system
💡classification
💡overfitting
💡representation learning
💡plateau
Highlights
Exploration of clip embeddings and their role in understanding the relationship between images and text.
Discussion on the potential of generative AI to produce new sentences and images.
The concept that analyzing pairs of images and text can lead to a distilled representation of an image's content in language.
Argument that with enough training data and a large network, AI could achieve general intelligence across domains.
The importance of experimental justification over hypothetical claims in scientific inquiry.
Recent paper arguing against the idea that simply adding more data and bigger models will solve complex AI tasks.
The paper suggests that achieving general zero-shot performance on new tasks requires an astronomical amount of data.
Introduction of clip embeddings, which use a shared embedded space for images and text to match their meanings.
Potential applications of clip embeddings in classification, image recall, and recommender systems.
The paper's findings that massive amounts of data are needed to effectively apply downstream tasks for difficult problems.
The challenge of classifying specific subcategories like breeds of cats or tree species due to insufficient data.
The paper's experiments on various concepts, models, and downstream tasks, showing a consistent trend.
Evidence suggesting a plateau in performance improvement despite increasing data and model sizes.
The need for alternative strategies beyond Transformers for better performance on underrepresented tasks.
The paper's analysis of the prevalence of different concepts in datasets and their impact on downstream task performance.
The issue of class imbalance within datasets, leading to varied performance on different tasks.
The potential for companies with more resources to improve models through better data and human feedback.
The anticipation of future developments in AI and whether performance will plateau or continue to improve.
Sponsorship message and invitation to participate in programs run by Jane Street, with a link to their website.
Transcripts
so we looked at clip embeddings right
and we've talked a lot about using
generative AI to produce new sentences
to produce new images and so on and so
to understand images all these kind of
different things and the idea was that
if we look at enough pairs of images and
text we will learn to distill what it is
in an image into that kind of language
so the idea is you have an image you
have some texts and you can find a
representation where they're both the
same the argument has gone that it's
only a matter of time before we have so
many images that we train on and so and
such a big Network and all this kind of
business that we get this kind of
general intelligence or we get some kind
of extremely effective AI that works
across all domains right that's the
implication right the argument is and
you see a lot in the sort of tech sector
from the from some of these sort of um
big tech companies who to be fair want
to sell products right that if you just
keep adding more and more data or bigger
and bigger models or a combination of
both ultimately you will move Beyond
just recognizing cats and you'll be able
to do anything right that's the idea you
show enough cats and dogs and eventually
the elephant just is
implied as someone who works in science
we don't hypothesize about what happens
we experimentally justify it right so I
would say if you're going to if you're
going to say to me that the only upward
trajectory is is going you know the only
trajectory is up it's going to be
amazing I would say go on and prove it
and do it right and then we'll see we'll
sit here for a couple of years and we'll
see what happens but in the meantime
let's look at this paper right which
came out just recently this
paper is saying that that is not true
right this paper is saying that the
amount of data you will need to get that
kind of General zero shot performance
that is to say performance on new tasks
that you've never
seen is going to be astronomically vast
to the point where we cannot do it right
that's the idea so it basically is
arguing against the idea that we can
just add more data and more models and
we we'll solve it right now this is only
one p
and of course you know your mileage may
vary if you have a bigger GPU than these
people and so on but I think that this
is actual numbers right which is what I
like because I want to see tables of
data that show a trend actually
happening or not happening I think
that's much more interesting than
someone's blog post that says I think
this is going what's going to happen so
let's talk about what this paper does
and why it's interesting we have clip
embeddings right so we have an image we
have a big Vision Transformer and we
have a big text encoder which is another
Transformer bit like the sort of you
would see in a large language model
right which takes text strings my text
string today and we have some shared
embedded space and that embedded space
is just a numerical fingerprint for the
meaning in these two items and they're
trained remember across many many images
such that when you put the same image
and the text that describes that image
in you get something in the middle that
matches and the idea then is you can use
that for other tasks like you can use
that for classification you can use it
for image recall if you use a streaming
service like Spotify or Netflix right
they have this thing called a recom
recommended system a recommended system
is where you've watched this program
this program this program what should
you watch next right and you you might
have noticed that your mileage may vary
on how effective that is but actually I
think they're pretty impressive what
they have to do but you could use this
for a recommender system because you
could say basically what programs have I
got that embed into the same space of
all the things I just watched and and
recommend them that way right so there
are Downstream tasks like classification
and recommendations that we could use
based on a system like this what this
paper is showing is that you cannot
apply these effectively these Downstream
tasks for difficult problems without
massive amounts of data to back it up
right and so and the idea that you can
apply you know this kind of
classification on hard things so not
just cats and dogs but specific cats and
specific dogs or subspecies of tree
right or difficult problems where the
the answer is more difficult than just
the broad category that there isn't
enough data on those things to train
these models and way I've got one of
those apps that tells you what specific
species a tree is so is it not just
similar to that no because they're just
doing classification right or some other
problem they're not using this kind of
generative giant AI right the argument
has been why do that silly little
problem where you can do a general
problem and solve all your problems
right and the response is because it
didn't work right that's that's that's
that's why we're doing it um so there
are pros and cons for both right I'm not
going to say that no generative AI is
useful or no or these these models are
incredibly effective for what they do
but I'm perhaps suggesting that it may
not be reasonable to expect them to do
very difficult medical diagnosis because
you haven't got the data set to back
that up right so how does this paper do
this well what they do is they def they
Define these Core Concepts right so some
of the concepts are going to be simple
ones like a cat or a person some of them
are going to be slightly more difficult
like a specific species of cat or a
specific disease in an image or
something like this and they they come
up about
4,000 different concepts right and these
are simple text Concepts right these are
not complicated philosophical ideas
right I don't know how well it embeds
those and and what they do is they look
at the prevalence of these Concepts in
these data sets and then they sh they
they test how well the downstream task
of let's say one zero shot
classification or recall recommended
systems works on all of these different
concepts and they plot that against the
amount of data that they had for that
specific concept right so let's draw a
graph and that will help me make it more
clear right so let's imagine we have a
graph here like this and this is the
number of
examples in our training set of a
specific concept right so let's say a
cat a dog something more difficult and
this is the performance on the actual
task of let's say recommend a system or
recall of an object or the ability to
actually classify as a cat right
remember we talked about how you could
use this for zero shck classification by
just seeing if it embeds to the same
place as a picture of a cat the text a
picture of a cat that kind of process so
this is performance right the best case
scenario if you want to have an all
powerful AI that can solve all the
world's problems is that this line goes
very steeply upwards right this is the
exciting case it goes like like this
right that's the exciting case this is
the kind of AI explosion argument that
basically says we're on the Custer
something that's about to happen
whatever that may be where the scale is
going to be such that this can just do
anything right okay then there the
perhaps slightly more reasonable should
we say pragmatic interpretation which is
like just call it balanced right which
is but there a sort of linear movement
right so the idea is that we have to add
a lot of examples but we are going to
get a decent performance Boost from it
right so we just keep adding examples
we'll keep getting better and that's
going to be great and remember that if
we ended up up here we have something
that could take any image and tell you
exactly what's in it under any
circumstance right that's that's kind of
what we're aiming for and similarly for
large language models this would be
something that could write with
Incredible accuracy on lots of different
topics or for image generation it would
be something that could take your prompt
and generate a photorealistic image of
that with almost no coercion at all
that's kind of the goal this paper has
done a lot of experiments on a lot of
these Concepts across a lot of models
across a lot of Downstream tasks and
let's call this the evidence what you're
going to call it pessimistic now it is
pessimistic also right it's logarithmic
so it basically goes like this right
flattens out it flattens out now this is
just one paper right it doesn't
necessarily mean that it will always
flatten out but the argument is I think
that and it's not an argument they
necessarily make in in the paper but you
know the paper's very reasonable I'm
being a bit more Cavalier with my
wording the suggestion is that you can
keep adding more examples you can keep
making your models bigger but we are
soon about to hit a plateau where we
don't get any better and it's costing
you millions and millions of dollars to
train this at what point do you go well
that's probably about as good as we're
going to get with technology right and
then the argument goes we need something
else we need something in the
Transformer or some other way of
representing data or some other machine
learning strategy or some other strategy
that's better than this in the long term
if we want to have this line G up here
or this line gar up here that's that's
kind of the argument and so this is
essentially
evidence I would argue against the kind
of
explosion you know possibility of but
just you just add a bit more data and we
were on the cusp of something we might
come back here in a couple of years you
know if you're still allow me on
computer file after this absolute
embarrassment of of these claims that I
made um and we say okay actually the
performan has improve improved massively
right or we might say we've doubled the
number of data sets to 10 billion images
and we've got 1% more right on the on on
the classification to which is good but
is it worth it I don't know this is a
really interesting paper because it's
very very fough right if there's a lot
of evidence there's a lot of Curves and
they all look exactly the same it
doesn't doesn't matter what method you
use it doesn't matter what data set you
train on it doesn't matter what your
Downstream task is the vast majority of
them show this kind of problem and the
other problem is that we don't have a a
nice even distribution of classes and
Concepts within our data set so for
example cats you can imagine are over um
emphasized or over represented over
represented yeah over represented in the
data set by an order of magnitude right
whereas specific planes or specific
trees are incredibly under represented
because you just have tree right so I
mean trees are probably going to be less
represented than cats anyway but then
specific species of tree very very
underrepresented which is why when you
ask one of these models what kind of cat
is this or what kind of tree is this it
performs worse than when you ask it what
animal is this because it's a much
easier problem and you see the same
thing in image generation if you ask it
to draw a picture of something really
obvious like a castle where that comes
up a lot in the training set it can draw
you a Fant fantastic castle in the style
of Monet and it can do all this other
stuff but if you ask it to draw some
obscure artifact from a video game
that's barely even made it into the
training set suddenly it's starting to
draw something a little bit less quality
and the same with large language models
this paper isn't about large language
models but the same process you can see
actually already happening if you talk
to something like chap GPT when you ask
it about a really important topic from
physics or something like this it will
usually give you a pretty good
explanation of that thing because that
in the training set but the question is
what happens when you ask it about
something more difficult right when you
ask it to write that code which is
actually quite difficult to write and it
starts to make things up it starts to
hallucinate and it starts to be less
accurate and that is essentially the
performance degrading because it's under
represented in the training set the
argument I think is at least it's the
argument that I'm starting to come
around to thinking if you want
performance on hard tasks tasks that are
under represented on just general
internet text and searches we have to
find some other way of doing it than
just is collecting more and more data
right particularly because it's
incredibly inefficient to do this right
on the other hand we they you know these
companies will they've got a lot more
gpus than me right they're going to
train on on bigger and bigger corpuses
better quality data they're going to use
human feedback to better train their
language models and things so they may
find ways to improve this you know up
this way a little bit as we go forward
but it's going to be really interesting
see what happens because you know will
it Plateau out will we see trap GPT 7
or8 or 9 be roughly the same as chat
dpt4 or will we see another
state-of-the-art performance boost every
time I'm kind of trending this way but
you know it'll be excited to see if it
goes this way take a look at this puzzle
devised by today's episode sponsor Jane
straight it's called bug bite inspired
by debugging code that world we're all
too familiar with where solving one
problem might lead to a whole chain of
others we'll link to the puzzle in the
video description let me know how you to
get on and speaking of Jane Street we're
also going to link to some programs that
they're running at the moment these
events are all expenses paid and give a
little taste of the tech and problem
solving used at trading firms like Jane
Street are you curious are you Problem
Solver are you into computers I think
maybe you are if so well you may well be
eligible to apply for one of these
programs check out the links below or
visit the Jane Street website and follow
the these links there are some deadlines
coming up for ones you might want to
look at and there are always more on the
horizon our thanks to Jane Street for
running great programs like this and
also supporting our Channel and don't
forget to check out that bug bite puzzle
Ver Más Videos Relacionados
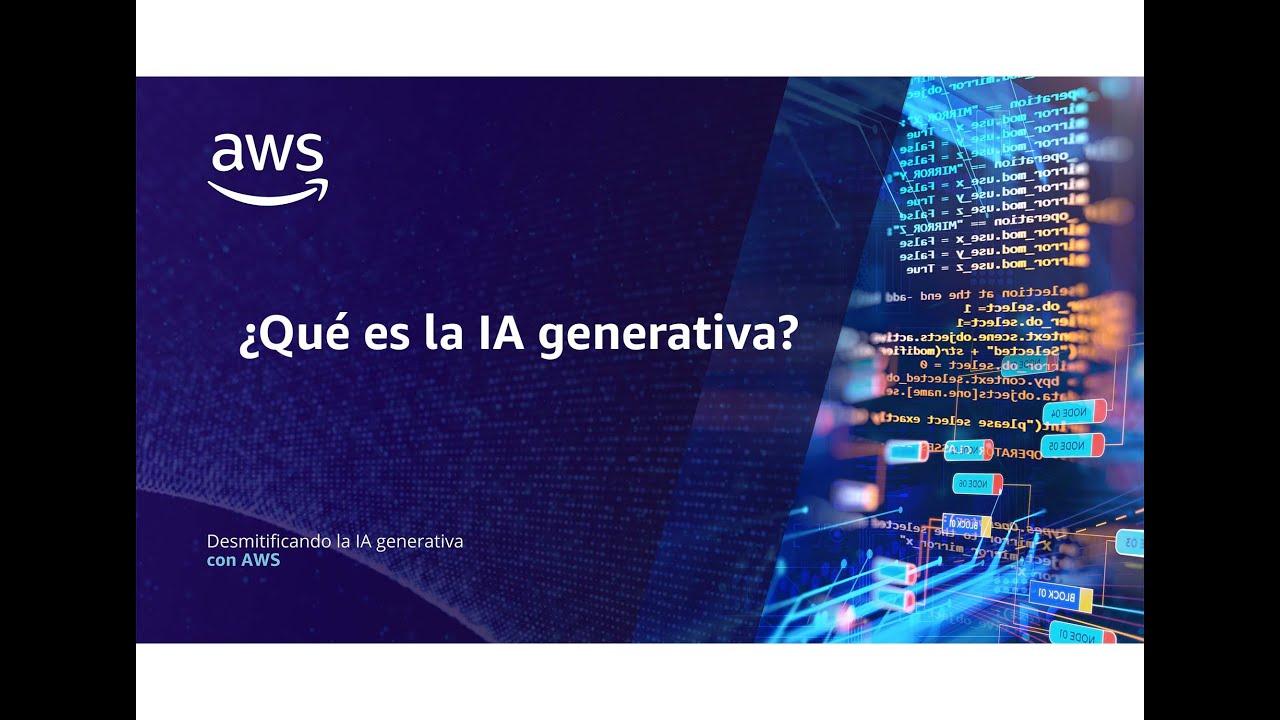
¿Qué es la IA generativa? | Desmitificando la IA generativa con AWS
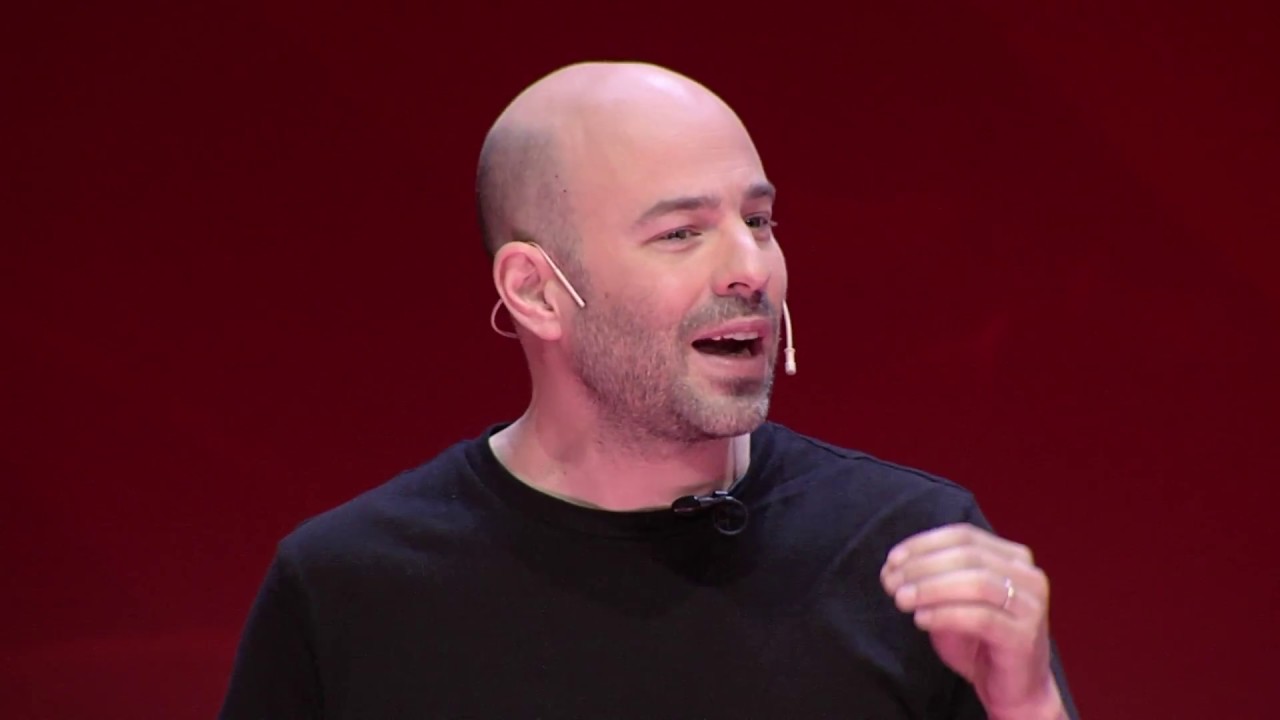
Inteligencia Artificial: ¿Amiga o Enemiga? | Diego Fernández Slezak | TEDxRiodelaPlata
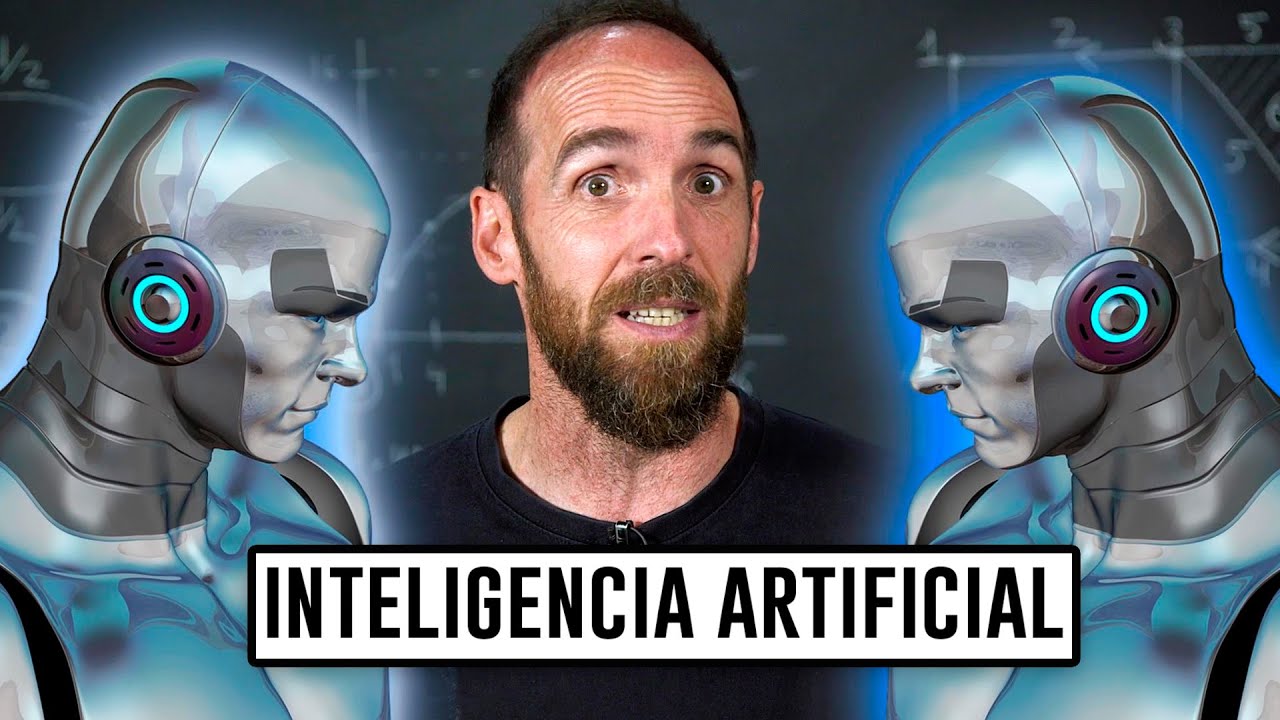
¿Qué es y cómo funciona la INTELIGENCIA ARTIFICIAL?
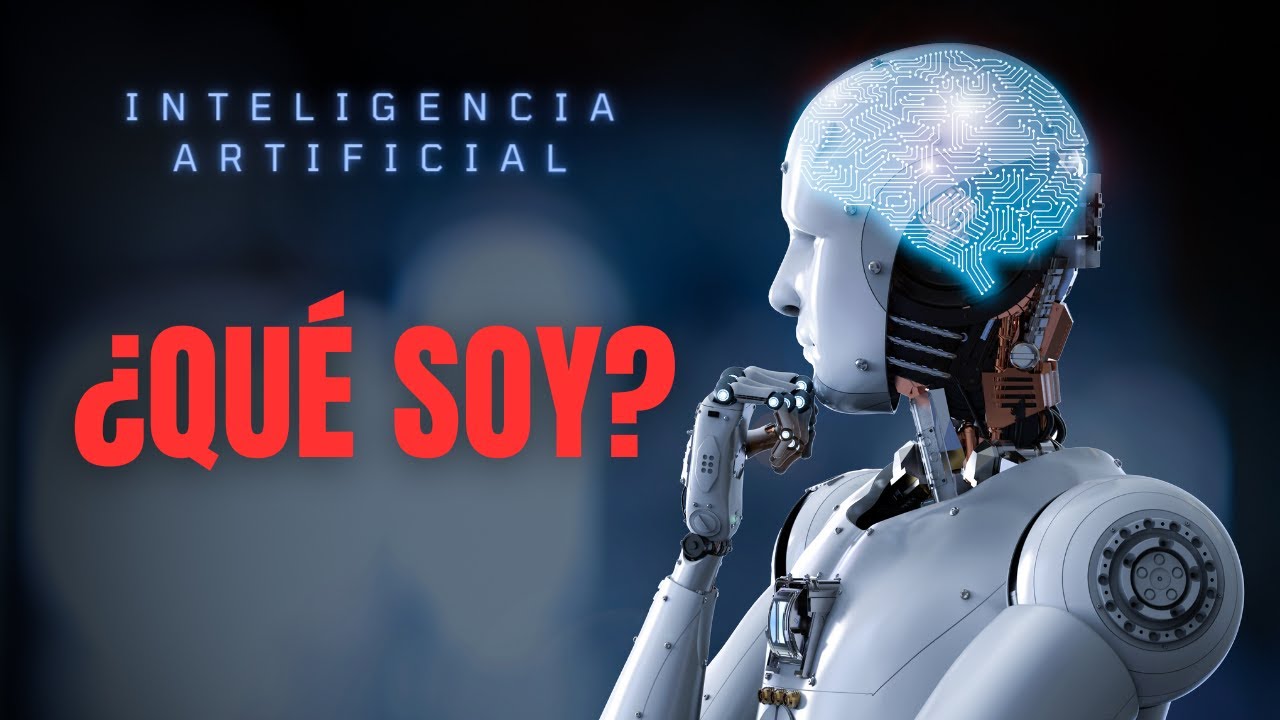
Qué es y como funciona la INTELIGENCIA ARTIFICIAL
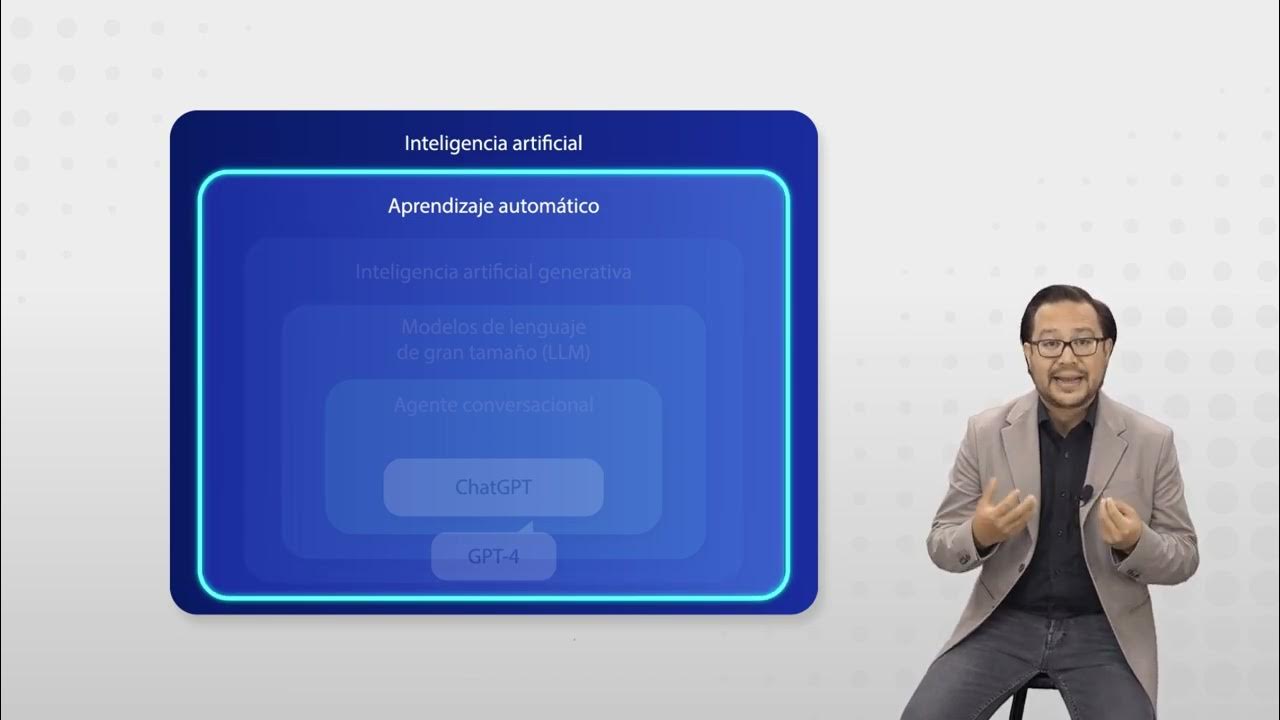
Qué es Inteligencia Artificial Generativa?
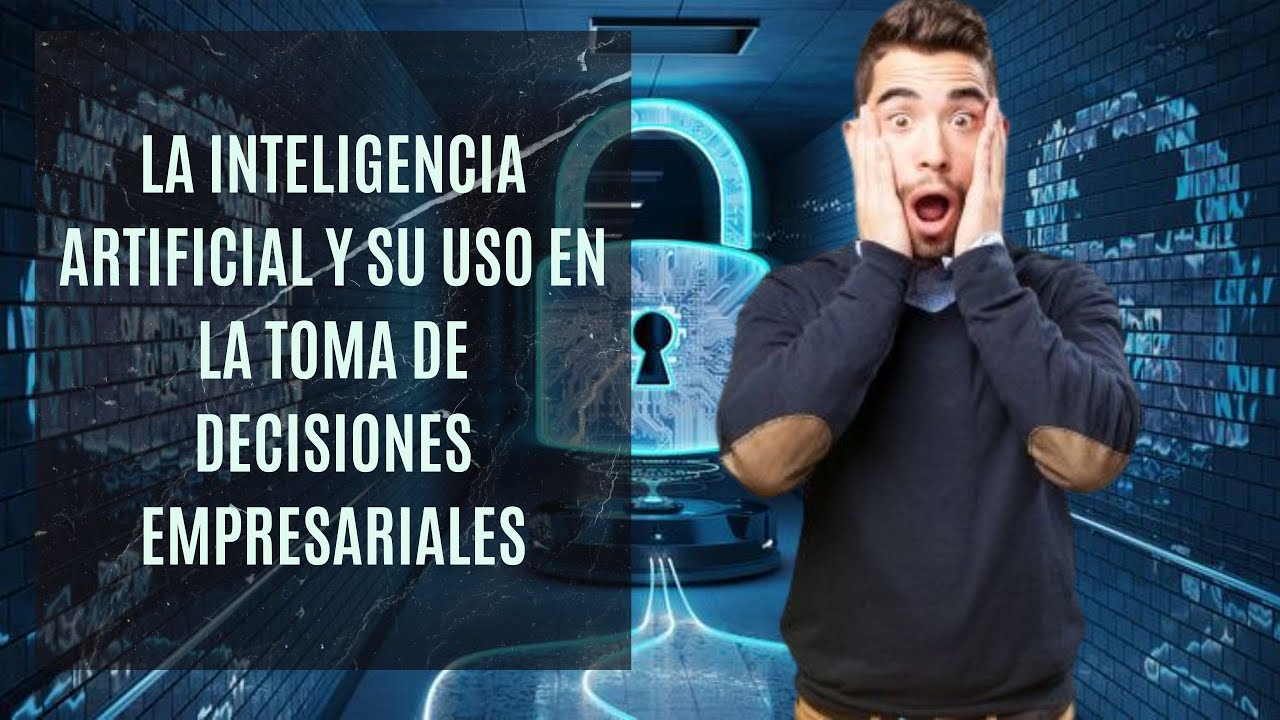
La inteligencia artificial y su uso en la toma de decisiones empresariales
5.0 / 5 (0 votes)