Bayes theorem, the geometry of changing beliefs
Summary
TLDRThe video explains Bayes' theorem, a fundamental formula in probability and its wide-ranging applications in science, AI, and even treasure hunting. It starts with an illustrative example featuring Steve, a character used to demonstrate the biases in human judgment explored by psychologists Daniel Kahneman and Amos Tversky. Through this, the video emphasizes the importance of considering prior probabilities when evaluating evidence. The script breaks down the reasoning behind Bayes' theorem and encourages thinking in terms of proportions and visual representations to update beliefs based on evidence.
Takeaways
- 🧮 Bayes' theorem is a key concept in probability, widely used in scientific discovery, machine learning, AI, and even treasure hunting.
- 📊 The theorem helps update beliefs based on new evidence rather than making decisions in a vacuum.
- 📖 The example of Steve, a 'meek and tidy' individual, demonstrates how people often make judgments based on stereotypes, ignoring broader statistical information like the ratio of farmers to librarians.
- 📚 Kahneman and Tversky's research shows that people tend to irrationally rely on stereotypes instead of considering the overall probability of certain outcomes.
- 🔍 A key insight from Bayes' theorem is understanding how evidence should update prior beliefs. In the case of Steve, the prior is the proportion of farmers to librarians.
- ⚖️ The likelihood in Bayes' theorem refers to the probability of evidence given a hypothesis. The probability of a hypothesis is adjusted based on this likelihood.
- 📐 Thinking about probabilities as areas in a diagram helps visualize how the theorem works by restricting the space of possibilities.
- 👨🔬 Scientists and AI developers use Bayes' theorem to evaluate the probability of hypotheses given new data, making it fundamental in fields requiring decision-making based on evidence.
- 🧠 Bayes' theorem can also help frame how we think about updating personal beliefs and opinions in light of new information.
- 🎨 Using representative samples (like librarians and farmers) or visualizing possibilities as areas can make understanding probability and Bayes' theorem more intuitive.
Q & A
What is the main goal of the video?
-The main goal of the video is to help the viewer understand one of the most important formulas in probability: Bayes' theorem. The video aims to explain its significance, provide a deeper understanding of its components, and demonstrate when and how to use it.
Why is Bayes' theorem considered important?
-Bayes' theorem is central to scientific discovery, machine learning, artificial intelligence, and even real-world applications like treasure hunting. It helps in updating beliefs based on new evidence and is a powerful tool for quantifying uncertainty.
What is the story of Tommy Thompson used to illustrate in the video?
-The story of Tommy Thompson, who used Bayesian search tactics to uncover a shipwreck carrying $700 million worth of gold, is used to illustrate a practical, high-stakes application of Bayes' theorem in real-world problem-solving.
What does the example of 'Steve' in the video demonstrate?
-The example of 'Steve' demonstrates how people often make irrational judgments by focusing on stereotypes (such as Steve being more likely to be a librarian than a farmer) rather than using probabilistic reasoning that incorporates relevant facts like the ratio of farmers to librarians in the population.
What did Kahneman and Tversky’s research show about human judgment?
-Kahneman and Tversky's research showed that human judgments often contradict the laws of probability. In the example with Steve, people tend to ignore the prior probability (the ratio of farmers to librarians) and instead focus on stereotypes, leading to irrational conclusions.
How does Bayes' theorem help with probabilistic reasoning in the Steve example?
-Bayes' theorem helps by incorporating both the prior probability (the ratio of farmers to librarians) and the likelihood of Steve fitting the librarian or farmer stereotype. It shows that even if Steve fits the librarian stereotype more, the overwhelming number of farmers in the population makes it more likely that Steve is a farmer.
What are the key components of Bayes' theorem?
-The key components of Bayes' theorem are: the prior (the initial probability of a hypothesis before considering new evidence), the likelihood (the probability of the evidence given that the hypothesis is true), and the posterior (the updated probability of the hypothesis after considering the evidence).
How is Bayes' theorem useful in machine learning and AI?
-In machine learning and AI, Bayes' theorem is used to update models as new data becomes available. It allows systems to explicitly and numerically model their 'beliefs' and improve predictions based on evidence.
Why is it important to understand when to use Bayes' theorem?
-Understanding when to use Bayes' theorem is crucial because it allows you to systematically update your beliefs based on new evidence. It helps you avoid irrational conclusions and make informed decisions in uncertain situations.
What role does the concept of prior belief play in Bayes' theorem?
-The concept of prior belief in Bayes' theorem represents the initial probability of a hypothesis before considering new evidence. It is essential because Bayes' theorem updates this prior belief based on the likelihood of new evidence, rather than letting the evidence alone determine the outcome.
Outlines
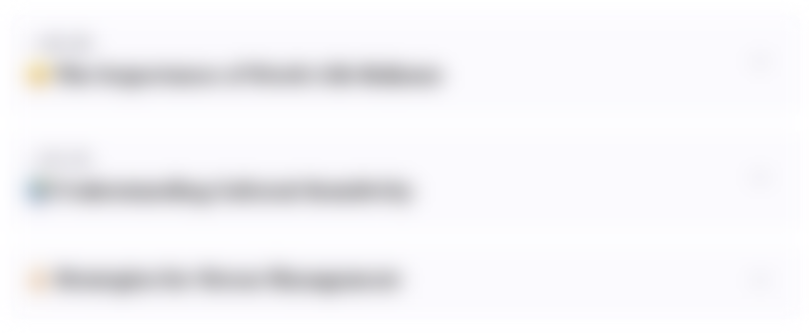
Esta sección está disponible solo para usuarios con suscripción. Por favor, mejora tu plan para acceder a esta parte.
Mejorar ahoraMindmap
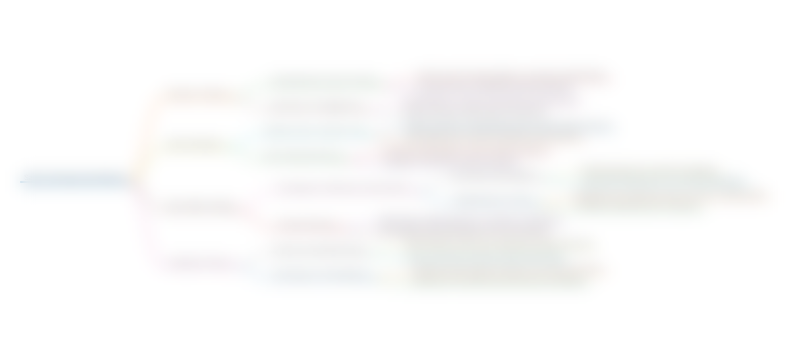
Esta sección está disponible solo para usuarios con suscripción. Por favor, mejora tu plan para acceder a esta parte.
Mejorar ahoraKeywords
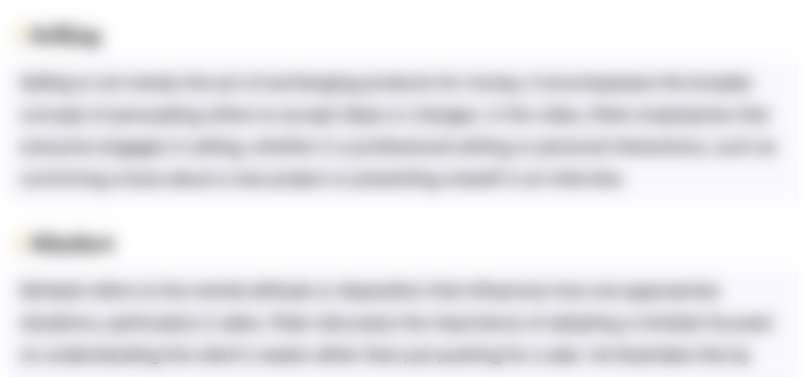
Esta sección está disponible solo para usuarios con suscripción. Por favor, mejora tu plan para acceder a esta parte.
Mejorar ahoraHighlights
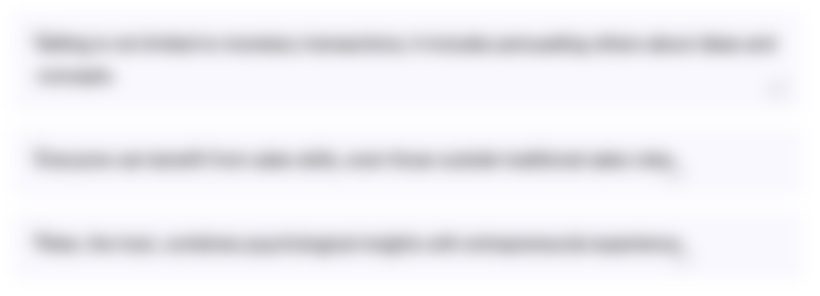
Esta sección está disponible solo para usuarios con suscripción. Por favor, mejora tu plan para acceder a esta parte.
Mejorar ahoraTranscripts
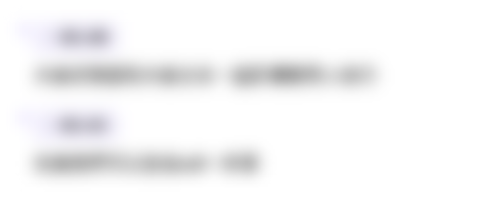
Esta sección está disponible solo para usuarios con suscripción. Por favor, mejora tu plan para acceder a esta parte.
Mejorar ahoraVer Más Videos Relacionados
5.0 / 5 (0 votes)