Statistics in 10 minutes. Hypothesis testing, the p value, t-test, chi squared, ANOVA and more
Summary
TLDRThis script is an educational guide on hypothesis testing in statistics. It explains the concept of a null hypothesis and how statistical tests like the Z-test, T-test, ANOVA, and Chi-Square test work to determine if observed differences in a sample are statistically significant or due to chance. The speaker, Greg Martin, uses the example of a population of purple and yellow people to illustrate the process of testing hypotheses and interpreting P-values. He emphasizes the importance of understanding when to use each test and provides a cheat sheet for further reference.
Takeaways
- 🔍 Understand the concept of sampling: When a full population is too large to measure, a sample is taken to infer about the whole population.
- 🎨 Recognize the colors of the population: The example uses purple and yellow to represent different groups within the population.
- 📊 Learn about the null hypothesis: It is assumed that there is no difference in the population, which is what statistical tests try to disprove.
- ✅ Grasp the significance of the Z-test: It calculates the probability of observing a sample result if the null hypothesis is true.
- 📉 Understand P-values: They represent the likelihood of observing the sample results if the null hypothesis is true.
- 🚫 Rejection of the null hypothesis: If the P-value is smaller than the predetermined alpha level, the null hypothesis is rejected, indicating a statistically significant result.
- 📈 Learn about different statistical tests: The script discusses T-Test, ANOVA, Chi-Square, and Correlation tests, each suited for different types of data and research questions.
- 📚 Greg Martin's educational resource: The speaker provides a cheat sheet and encourages learning more about statistics from his website.
- 🔗 Accessing resources: Instructions are given on how to download a statistics cheat sheet from the website for further study.
- 📋 Apply statistical tests to real scenarios: Examples are given for each test to illustrate how they can be used to analyze data and draw conclusions.
Q & A
What is the purpose of taking a sample from a population in research?
-The purpose of taking a sample from a population is to estimate the characteristics of the entire population when it is impractical to measure every single individual.
What is the null hypothesis in the context of the purple and yellow people example?
-The null hypothesis in the example is that there is no difference in the population, meaning there are equal numbers of purple and yellow people.
How does the Z-test help in determining the likelihood of observing a sample with 90% purple people if the null hypothesis is true?
-The Z-test calculates the probability (P-value) of obtaining a result as extreme or more extreme than what was observed, assuming the null hypothesis is true. A small P-value indicates that it is unlikely to have observed such a sample by chance if the null hypothesis were true.
What is the significance of the P-value in statistical testing?
-The P-value indicates the probability of obtaining a result at least as extreme as the one observed, assuming the null hypothesis is true. A small P-value suggests that the observed difference is statistically significant and not likely due to chance.
What is the Alpha value in hypothesis testing, and how is it used?
-The Alpha value is a predetermined threshold used to determine the significance of the results. If the P-value is smaller than the Alpha value, the null hypothesis is rejected, indicating the results are statistically significant.
Can you explain the concept of hypothesis testing using the T-Test example provided in the script?
-In the T-Test example, the null hypothesis assumes no difference in the average weight between men and women in the population. The T-Test calculates the probability of observing the sample difference if this null hypothesis were true. A small P-value would lead to the rejection of the null hypothesis, suggesting a real difference exists.
What is the main difference between the T-Test and ANOVA?
-The main difference is that the T-Test compares the means of two groups, while ANOVA compares the means of three or more groups to determine if there are any statistically significant differences among them.
How does the Chi-Square test differ from the T-Test and ANOVA?
-The Chi-Square test is used with categorical variables, examining the relationship between two categorical variables to determine if there is a significant association between them, unlike the T-Test and ANOVA, which are used with numeric variables.
What is the purpose of the correlation test mentioned in the script?
-The correlation test is used to determine if there is a statistically significant relationship between two numeric variables, such as a correlation between age and weight.
How can one obtain the statistics cheat sheet mentioned by Greg Martin in the script?
-The statistics cheat sheet can be obtained by visiting Greg Martin's website, learnmore365.com, signing up for a free account, navigating to the free resources section, finding the statistics cheat sheet, and downloading it.
What is the advice Greg Martin gives at the end of the script regarding learning statistics?
-Greg Martin advises to get the basics right, understand the question being asked, and know how to use a particular test to answer that question, which will make understanding more complicated statistics easier.
Outlines
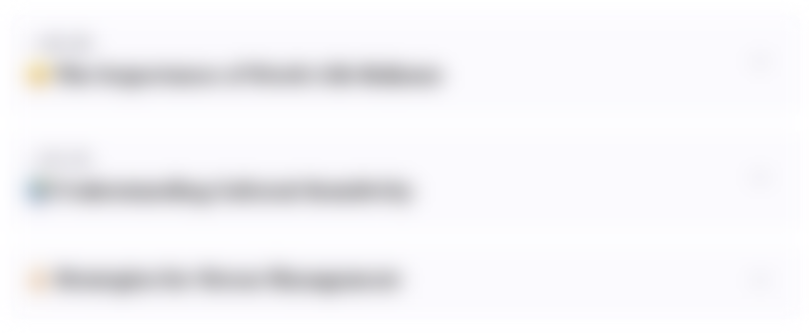
Esta sección está disponible solo para usuarios con suscripción. Por favor, mejora tu plan para acceder a esta parte.
Mejorar ahoraMindmap
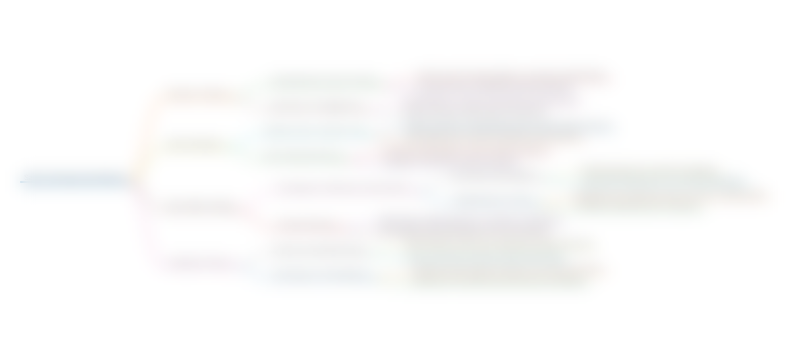
Esta sección está disponible solo para usuarios con suscripción. Por favor, mejora tu plan para acceder a esta parte.
Mejorar ahoraKeywords
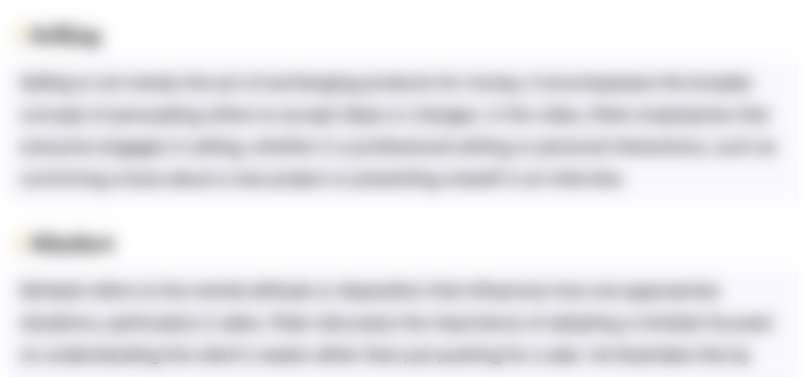
Esta sección está disponible solo para usuarios con suscripción. Por favor, mejora tu plan para acceder a esta parte.
Mejorar ahoraHighlights
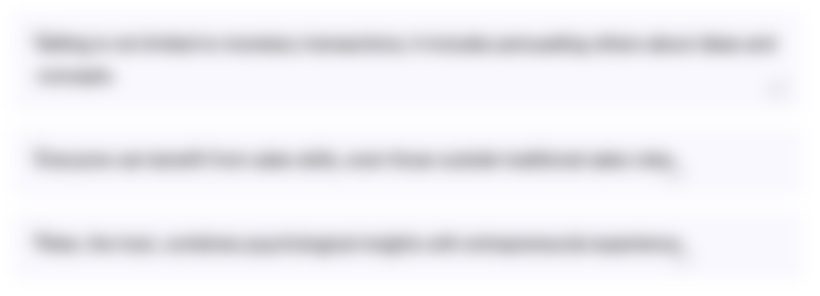
Esta sección está disponible solo para usuarios con suscripción. Por favor, mejora tu plan para acceder a esta parte.
Mejorar ahoraTranscripts
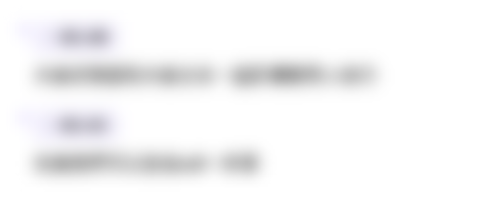
Esta sección está disponible solo para usuarios con suscripción. Por favor, mejora tu plan para acceder a esta parte.
Mejorar ahoraVer Más Videos Relacionados
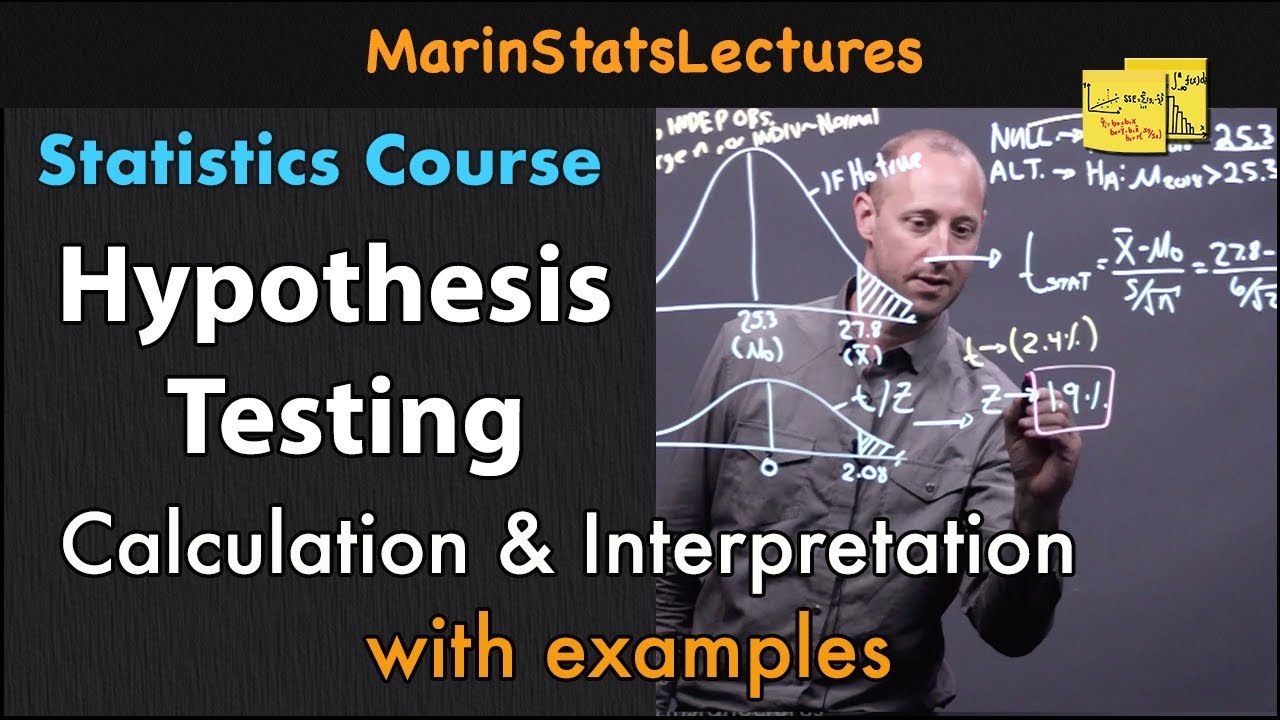
Hypothesis Testing: Calculations and Interpretations| Statistics Tutorial #13 | MarinStatsLectures

Test Statistics: Crash Course Statistics #26
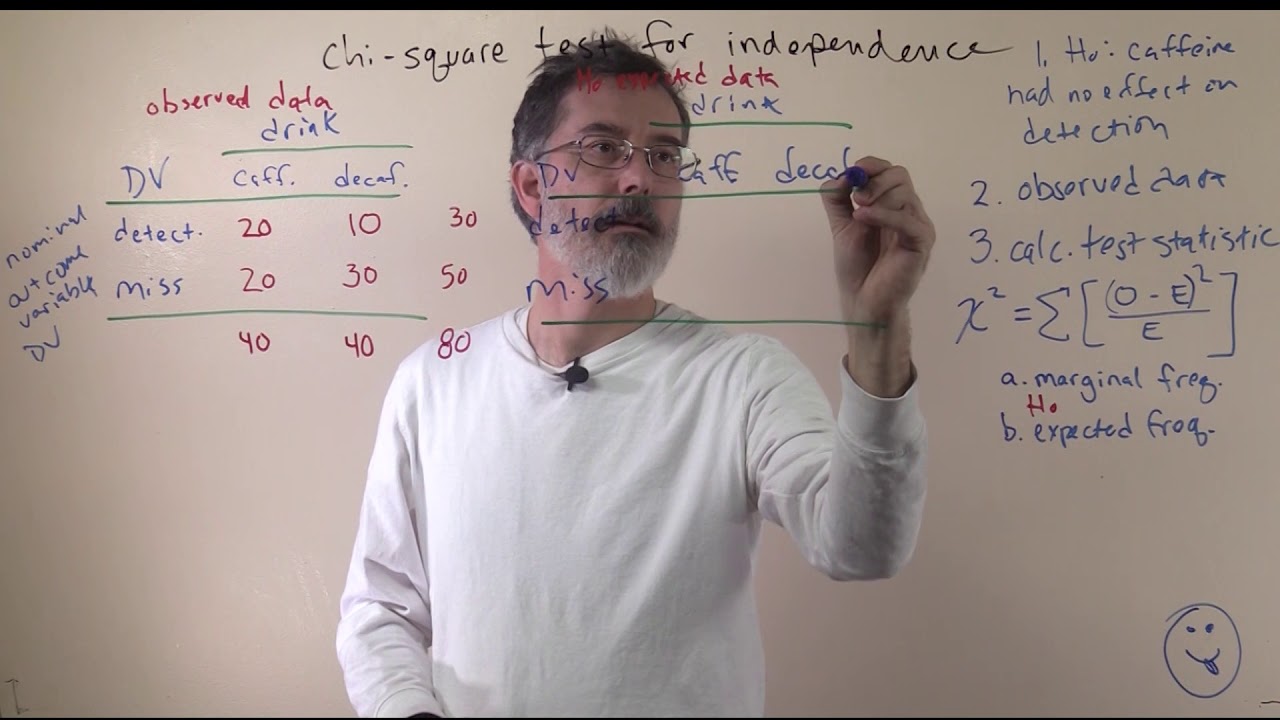
video 14.2. chi-square test of independence
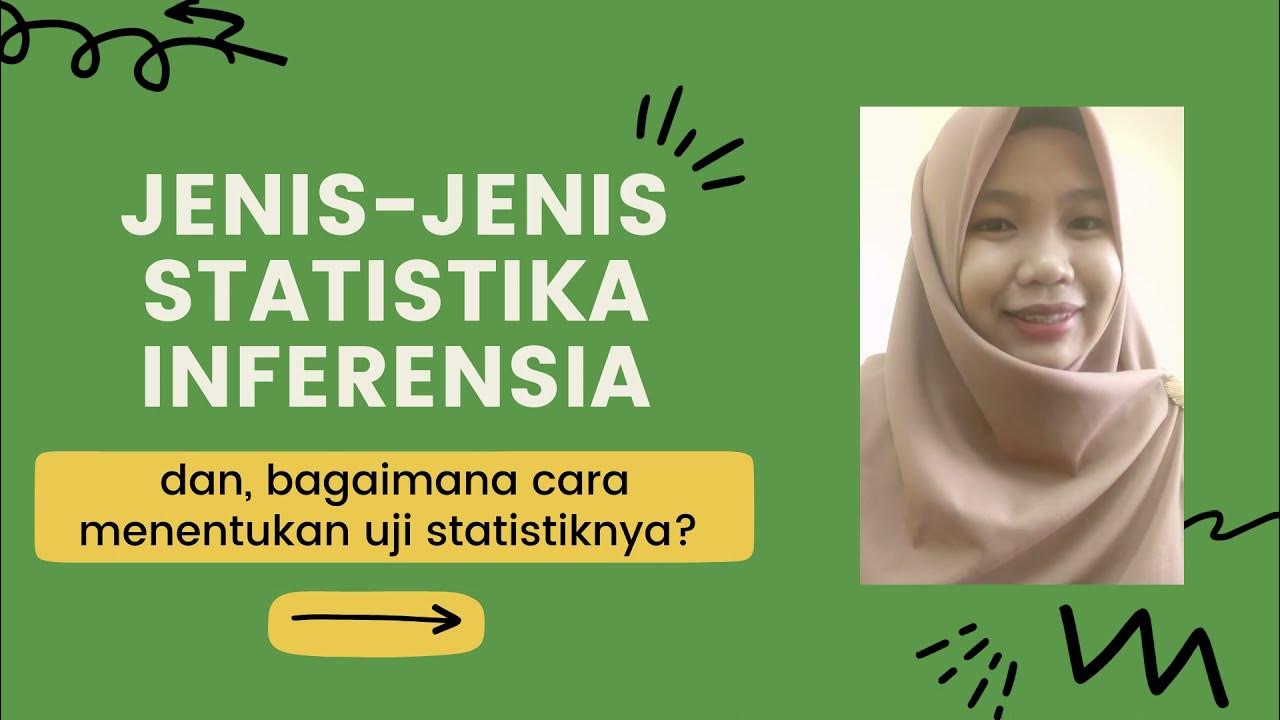
[PART 2] KONSEP DASAR STATISTIKA INFERENSIA
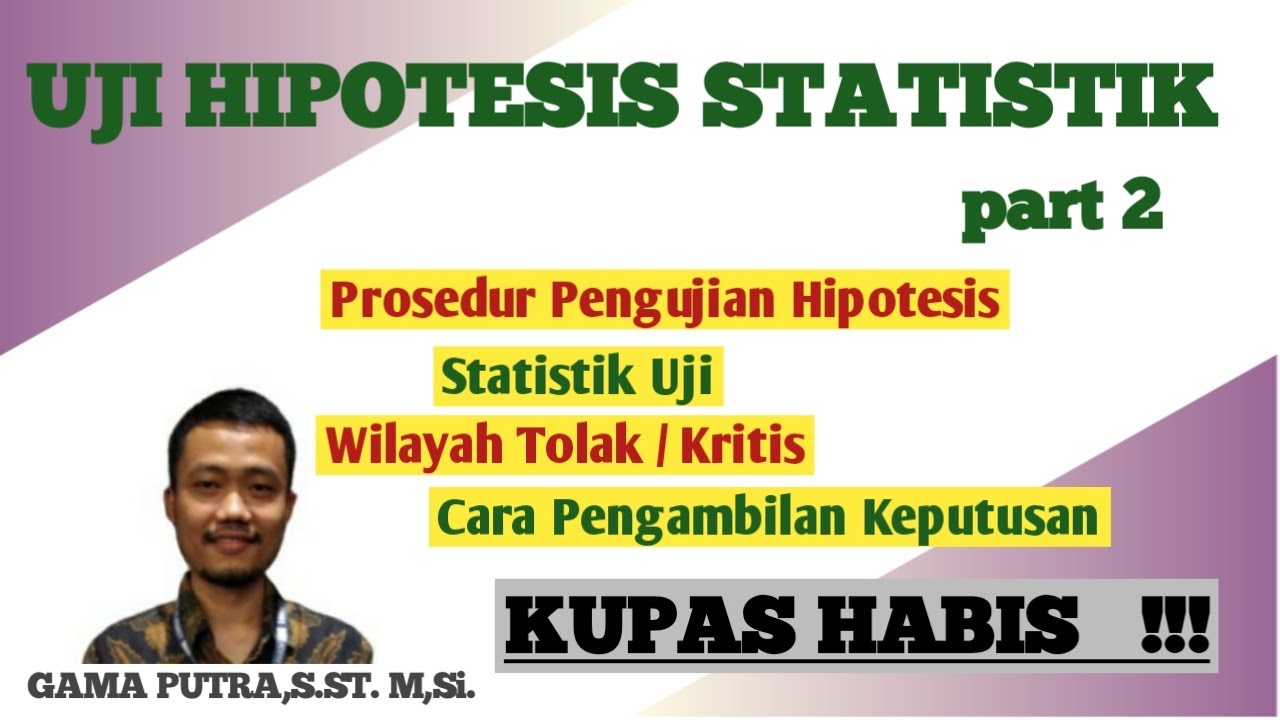
Uji Hipotesis part 2 (Prosedur Pengujian Hipotesis, Statistik Uji, Wilayah Tolak/ Kritis)
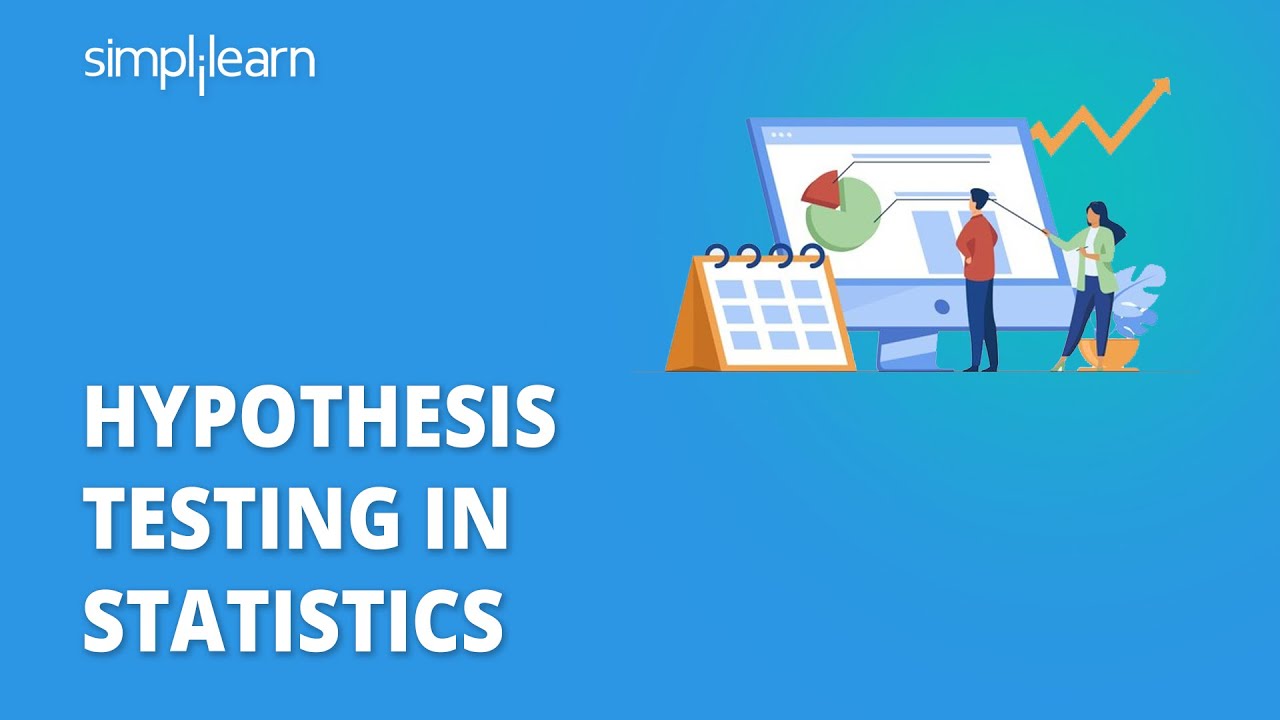
Hypothesis Testing In Statistics | Hypothesis Testing Explained With Example | Simplilearn
5.0 / 5 (0 votes)