Artificial intelligence in healthcare: opportunities and challenges | Navid Toosi Saidy | TEDxQUT
Summary
TLDRArtificial Intelligence (AI) is revolutionizing healthcare by personalizing treatment and enhancing hospital efficiency. AI models, trained on vast data sets, can predict patient outcomes and recommend tailored treatments. In cancer care, AI aids in diagnosing and identifying optimal therapies. However, current regulations aren't equipped for AI's dynamic learning capabilities, potentially hindering its full potential. New frameworks are needed to ensure AI's safe, effective integration into healthcare, promoting personalized health advice for all.
Takeaways
- 🤖 **AI as a Lifesaver**: Artificial intelligence has the potential to save lives and enhance healthcare for millions.
- 🏥 **Personalized Healthcare**: AI helps in personalizing care delivery, making hospitals more efficient, and improving healthcare access.
- 🧠 **Learning Models**: AI operates by training computer models on large datasets to make decisions or predict outcomes.
- 👨⚕️ **Better Decisions**: AI models can inform doctors about the most effective treatments for individual patients.
- 🧬 **Genetic Insights**: AI can analyze genetic information to aid in diagnosis and treatment, especially in complex cases like cancer.
- 🩺 **Cancer Care Innovation**: AI models are being developed to streamline cancer diagnosis and treatment planning.
- 🌐 **Global Impact**: The application of AI in healthcare is a global endeavor, aiming to improve outcomes for diverse populations.
- 📚 **Regulatory Challenges**: Existing regulatory frameworks are not designed for AI-based medical software and may limit its potential.
- 🔄 **Evolving AI**: AI software differs from traditional software as it learns and evolves, which current regulations do not fully accommodate.
- 🌟 **New Regulatory Frameworks**: Emerging proposals for regulation could unlock AI's full potential in healthcare if implemented correctly.
- 🌈 **Diverse Data Sets**: It's crucial that AI training data represents the entire human population to avoid bias and ensure accuracy.
Q & A
What is the potential of AI in healthcare according to the script?
-AI has the potential to save lives, personalize healthcare delivery, make hospitals more efficient, and improve access to healthcare by providing accurate decision-making tools.
How does AI work in the context described in the script?
-AI works by educating a computer model using complex and large data sets, allowing it to learn and make decisions or predict outcomes when presented with new data.
What role does AI play in personalized medicine?
-AI helps in personalized medicine by analyzing data from thousands of patients to determine the most effective treatment for an individual based on their unique conditions.
How is AI being used in cancer diagnosis as mentioned in the script?
-AI is used in cancer diagnosis by consolidating information from blood tests, X-ray images, and genetic information to provide accurate predictions of diagnosis, treatment options, and prognosis.
What is the significance of the AI tool developed in Brisbane for cancer patients?
-The AI tool developed in Brisbane can accurately identify the primary site of cancer, empowering doctors to provide specific treatments that are likely to work for the patient.
Why is the existing regulation framework a challenge for AI in healthcare?
-Existing regulation frameworks are not designed for AI software, which can learn and evolve over time, unlike traditional software. This can limit AI's potential to improve its functionality or accuracy.
What are the proposed solutions to the regulatory challenges faced by AI in healthcare?
-Proposed solutions include more transparent reporting mechanisms for developers to disclose how their models learn and evolve, combined with ongoing and real-time monitoring to ensure accuracy and adaptability.
Why is it important that the training data for AI algorithms is representative of the entire human population?
-It is important to prevent data bias and ensure that AI models are trained on diverse and robust data sets to accurately predict outcomes for all populations, not just specific demographics.
What is the potential issue with AI models trained on predominantly one demographic?
-AI models trained on predominantly one demographic may not perform well on other populations, leading to potential misdiagnoses or inaccurate predictions.
What functionality is urgently needed in AI models according to the script?
-AI models need a functionality that allows them to express uncertainty or provide a best guess based on a skewed training population when dealing with low confidence results.
How can new regulatory frameworks help implement AI in healthcare?
-New regulatory frameworks, developed in consultation with stakeholders, can ensure that AI tools are compliant, adaptive, and serve the entire population equally, thus improving healthcare delivery.
Outlines
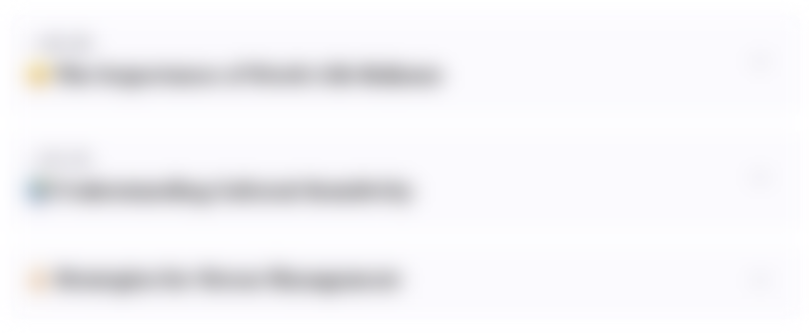
Esta sección está disponible solo para usuarios con suscripción. Por favor, mejora tu plan para acceder a esta parte.
Mejorar ahoraMindmap
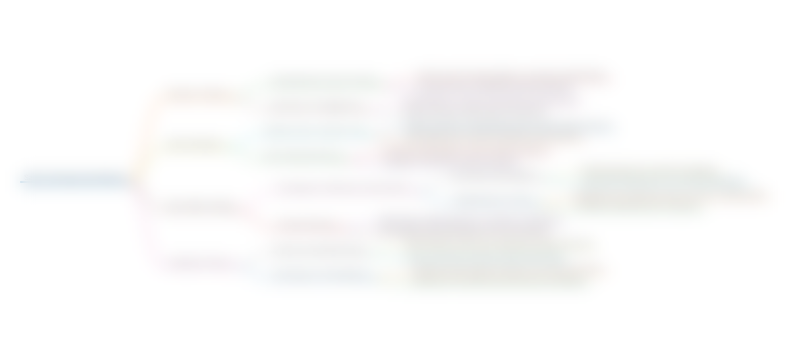
Esta sección está disponible solo para usuarios con suscripción. Por favor, mejora tu plan para acceder a esta parte.
Mejorar ahoraKeywords
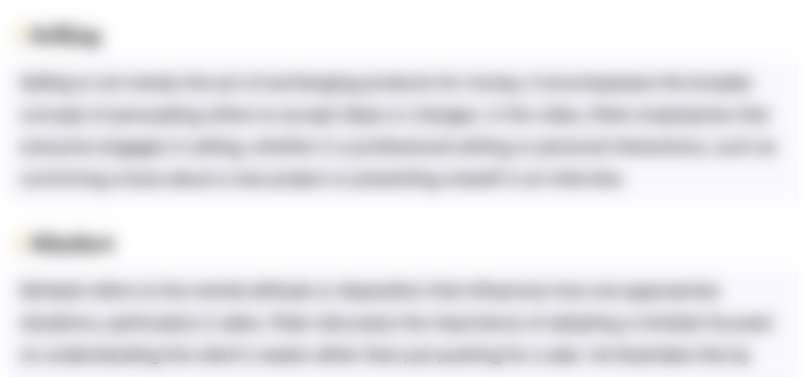
Esta sección está disponible solo para usuarios con suscripción. Por favor, mejora tu plan para acceder a esta parte.
Mejorar ahoraHighlights
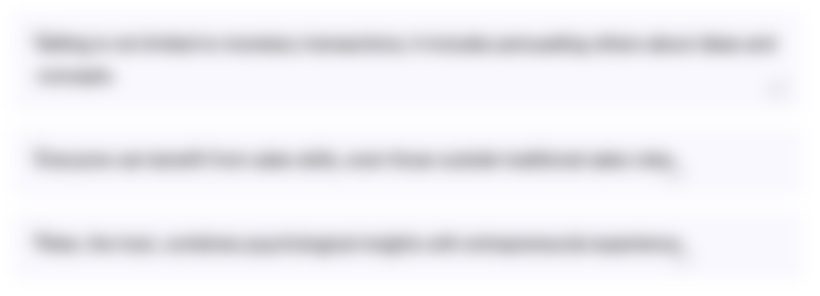
Esta sección está disponible solo para usuarios con suscripción. Por favor, mejora tu plan para acceder a esta parte.
Mejorar ahoraTranscripts
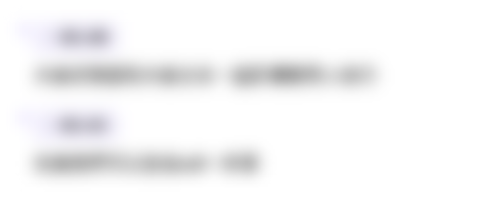
Esta sección está disponible solo para usuarios con suscripción. Por favor, mejora tu plan para acceder a esta parte.
Mejorar ahoraVer Más Videos Relacionados
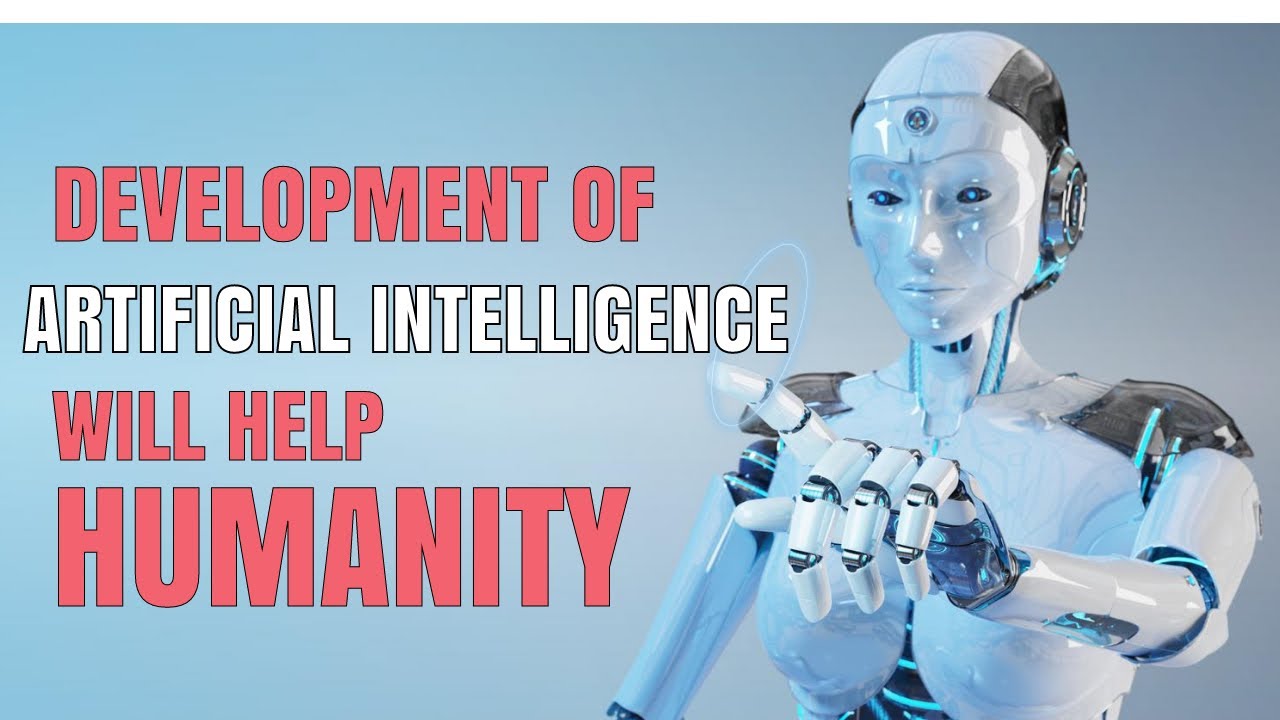
The Development of Artificial Intelligence Will Help Humanity
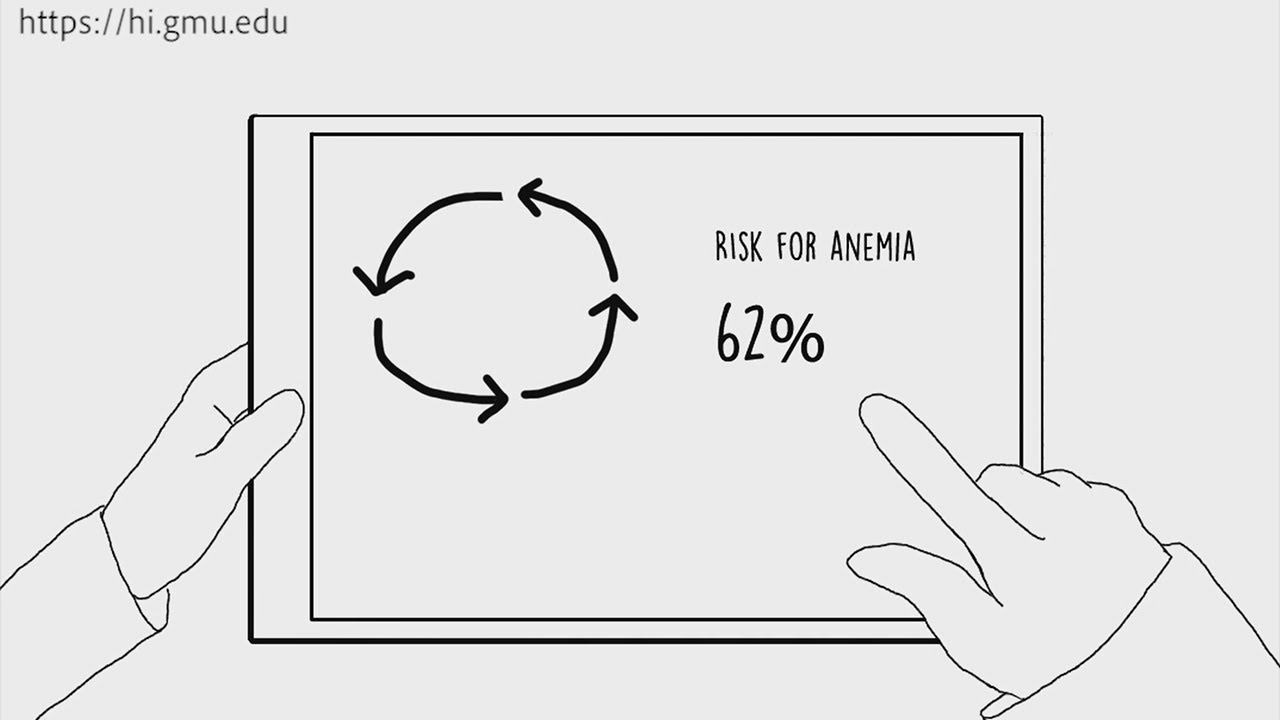
What is Health Informatics?
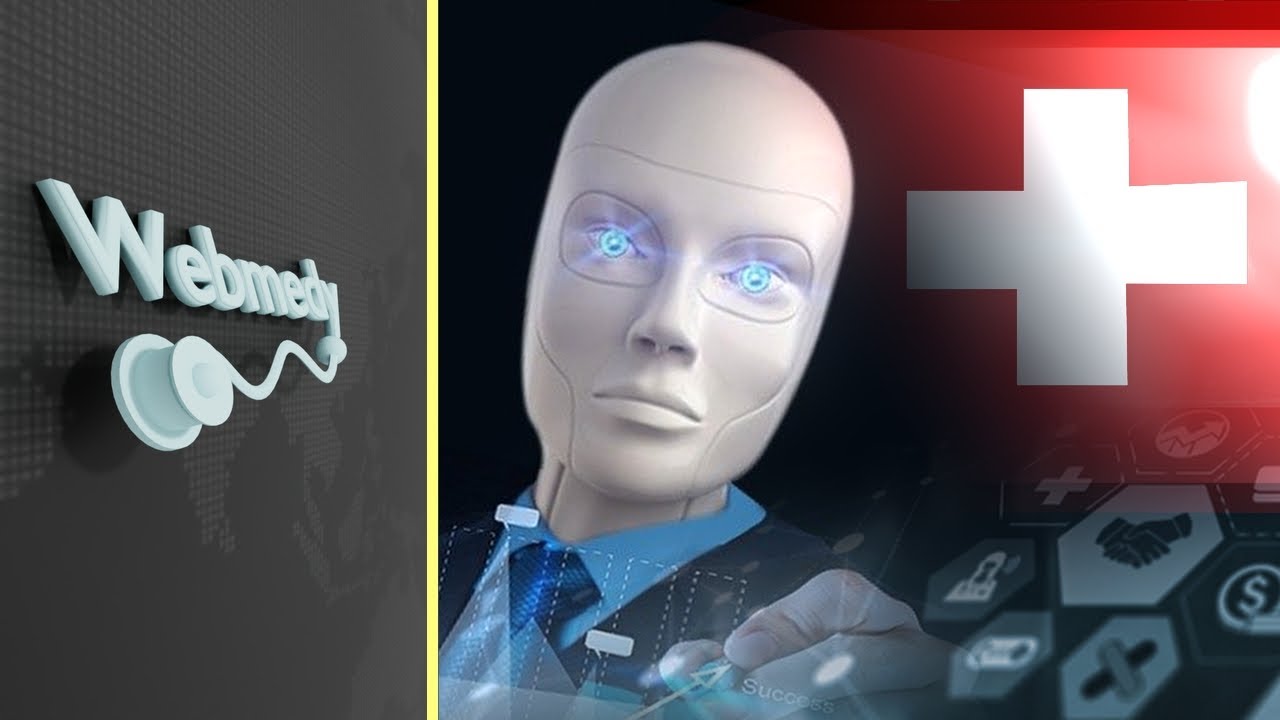
Kecerdasan Buatan dalam Perawatan Kesehatan | Masa Depan Kesehatan dengan AI
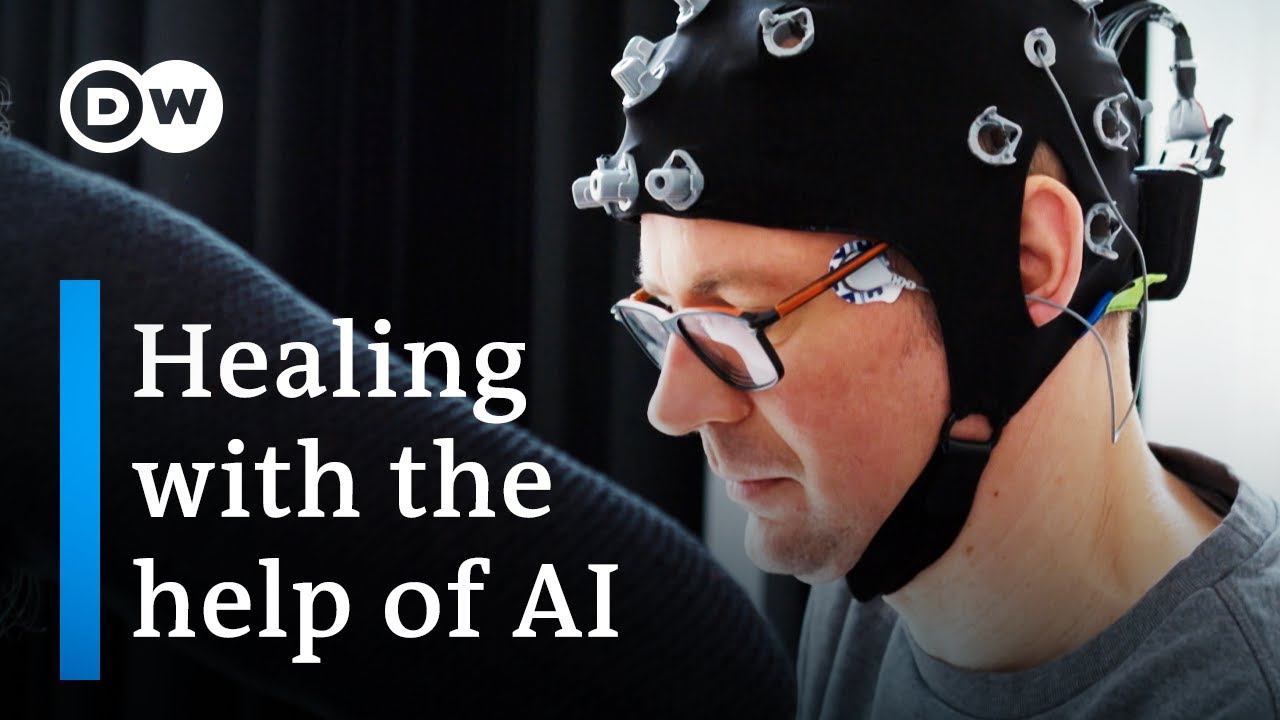
Doctors, apps and artificial intelligence - The future of medicine | DW Documentary
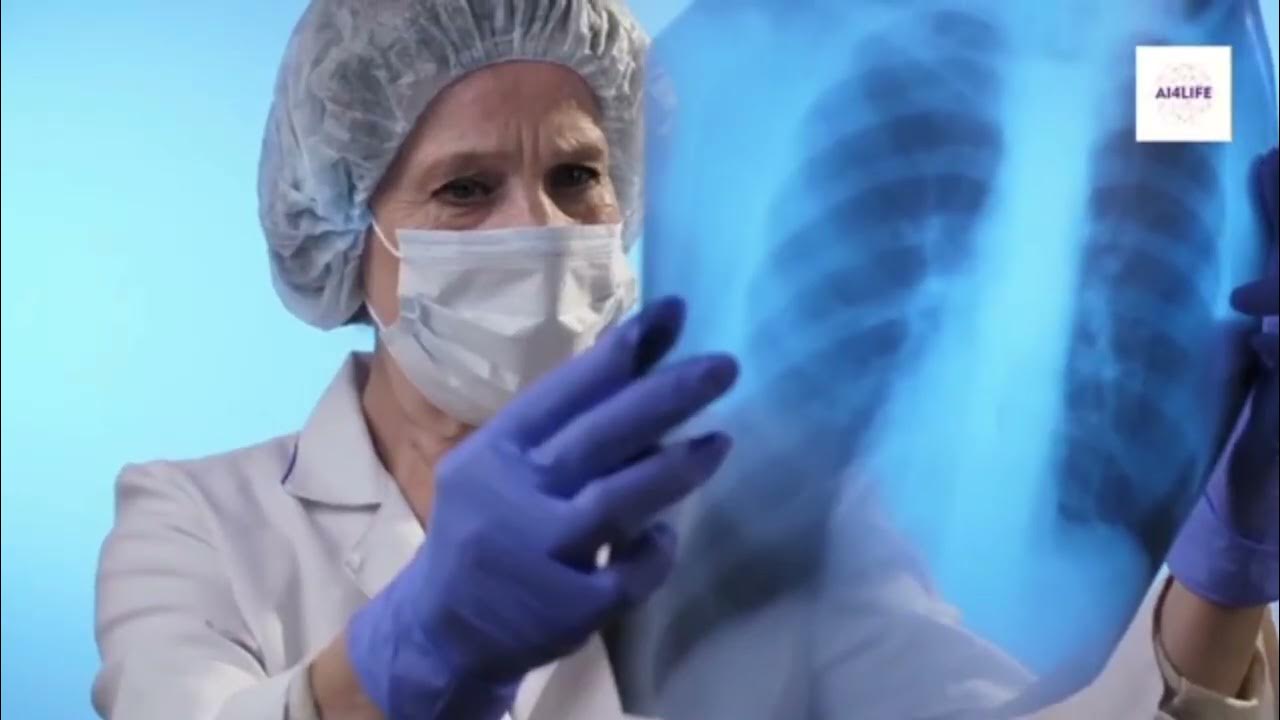
Perkembangan Teknologi Dalam Bidang Farmasi dan Kesehatan
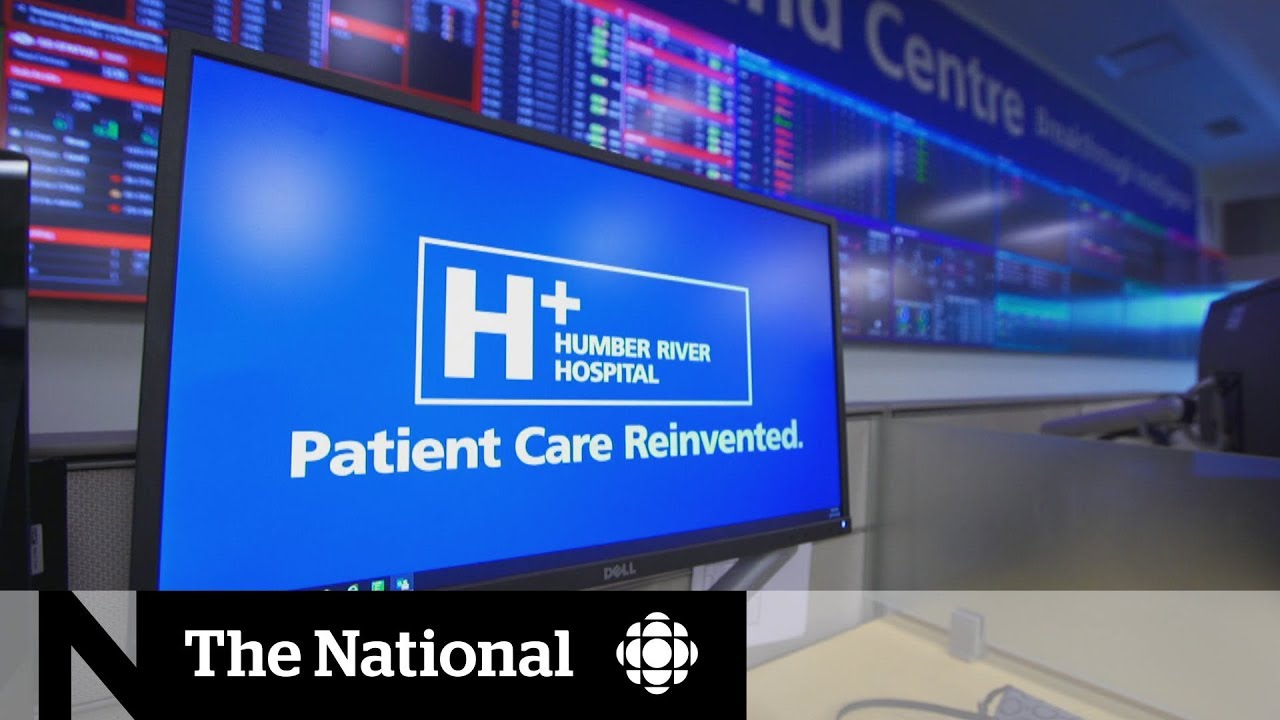
How AI could change the future of our health care
5.0 / 5 (0 votes)