How He Got $600,000 Data Engineer Job
Summary
TLDRIn this video, Zach Wilson shares his journey from a data analyst to a data engineer at major tech companies like Facebook, Netflix, and Airbnb. He discusses his salary progression from $80k to $600k, the unique work cultures at these companies, and the importance of data engineering skills like SQL, Python, and Apache Spark. Zach also addresses the impact of AI on data engineering roles and advocates for learning tools like Airflow and Databricks. He advises aspiring data engineers to focus on data modeling and machine learning for job security and provides resources to start a career in data engineering.
Takeaways
- 🚀 Zach Wilson's career started as a data analyst but evolved towards data engineering after realizing the rapid growth of data.
- 🐘 Zach was initially attracted to Hadoop, viewing it as a critical skill to learn early in his career.
- 👔 He found the corporate environment at Teradata too restrictive compared to the startup culture he preferred.
- 🌉 Zach's experience at Facebook (Meta) was significant for his growth as a data engineer, where he utilized tools like Hive and Dataflow.
- 📈 Netflix offered Zach a high salary upfront but also induced imposter syndrome due to the highly skilled team environment.
- 💼 Zach's salary progression from 80k to 600k is attributed to joining big tech companies and effectively negotiating his worth.
- 💼 He advises never to give the first number in a salary negotiation to avoid potentially lower offers.
- 💡 Zach emphasizes the importance of learning languages like SQL and Python, tools like Spark and Airflow, and skills in data modeling for aspiring data engineers.
- 🔮 The future of data engineering might see roles transformed or replaced by AI and low-code/no-code tools, but roles requiring nuanced expertise like machine learning will remain secure.
- 🌐 Zach misses the collaborative environment of corporate life but enjoys the autonomy and accountability of entrepreneurship.
- 🌟 For those looking to break into data engineering, Zach recommends his blog and the 'Data Engineer Handbook' GitHub repository for comprehensive resources.
Q & A
What was Zach Wilson's initial career role before becoming a data engineer?
-Zach Wilson initially started his career as a data analyst, specifically a 'Tableau guy', which involved working with data visualization tools.
Why did Zach Wilson decide to transition from data analysis to data engineering?
-Zach Wilson became bored with his data analyst role after mastering Tableau in about 9 months and decided to transition to data engineering after reading a statistic that 90% of the world's data had been created in the last 18 months, which made him realize the potential in the field.
Which company did Zach Wilson join after recognizing the growth in data and why?
-Zach Wilson joined a startup called Think Big Analytics after recognizing the significant growth in data, as he was drawn to the idea of working with big data technologies, particularly Hadoop.
How did Zach Wilson's career progress from Teradata to Facebook?
-Zach Wilson's career progressed from Teradata, where he worked for about 6-7 months in a very corporate environment, to Facebook after he left Teradata due to dissatisfaction with the corporate culture. He then moved to Washington DC for a brief period of software engineering before landing a data engineering role at Facebook in San Francisco.
What was the approximate salary progression for Zach Wilson from his first job to his role at Netflix?
-Zach Wilson's salary progressed from 80k at Teradata to 185k after joining Facebook, and then to 365k when he joined Netflix, where he was able to negotiate an increase to 550k within his first year.
What was the significant mistake Zach Wilson made during his salary negotiation at Netflix?
-Zach Wilson's significant mistake during his salary negotiation at Netflix was accepting the initial offer of 365k without doing proper research. He later found out that the median salary for his team was 500k, indicating he could have negotiated a higher starting salary.
What cultural differences did Zach Wilson experience between Facebook, Netflix, and Airbnb?
-Zach Wilson experienced a very collaborative culture at Facebook, which he felt was sometimes too collaborative. Netflix had a more mature and less intrusive work culture, with team members having established personal lives. Airbnb was not explicitly described, but he mentioned that both Google and Airbnb were known for their good work-life balance.
How did Zach Wilson overcome his imposter syndrome at Netflix?
-Zach Wilson overcame his imposter syndrome at Netflix after about a year, particularly after achieving a significant success with a database project, which made him feel that he truly belonged in the role.
What advice does Zach Wilson give for someone looking to get into data engineering?
-Zach Wilson advises that to get into data engineering, one should learn critical languages like SQL and Python, become familiar with tools such as Spark and Airflow, and understand data modeling. He also recommends his blog and the 'Data Engineer Handbook' GitHub repository as resources.
What is Zach Wilson's perspective on the impact of AI on data engineering roles?
-Zach Wilson believes that while AI will change some data engineering roles, especially those involving simple tasks, more nuanced roles involving machine learning or master data management are safe. He sees AI as a tool that can help solve problems faster but doesn't see it replacing the need for expert data engineers.
What is the 'Data mesh' architecture mentioned by Zach Wilson and why is it significant?
-The 'Data mesh' architecture is a new approach where business owners can manage and maintain their own data pipelines, potentially reducing the need for traditional data engineering roles. Zach Wilson believes it could become more successful with the advancement of tools like LLM (Large Language Models), although he has seen it fail in companies due to lack of proper tooling.
Outlines
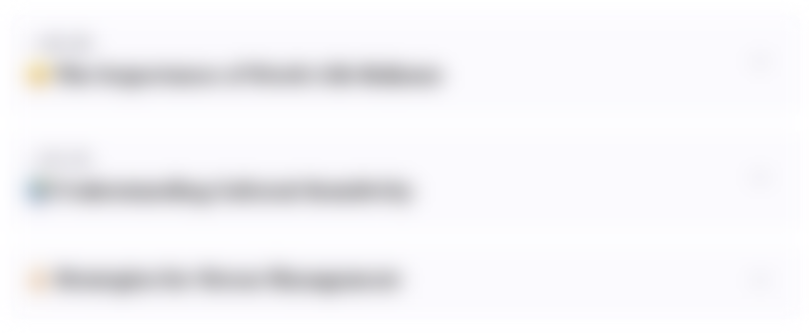
Esta sección está disponible solo para usuarios con suscripción. Por favor, mejora tu plan para acceder a esta parte.
Mejorar ahoraMindmap
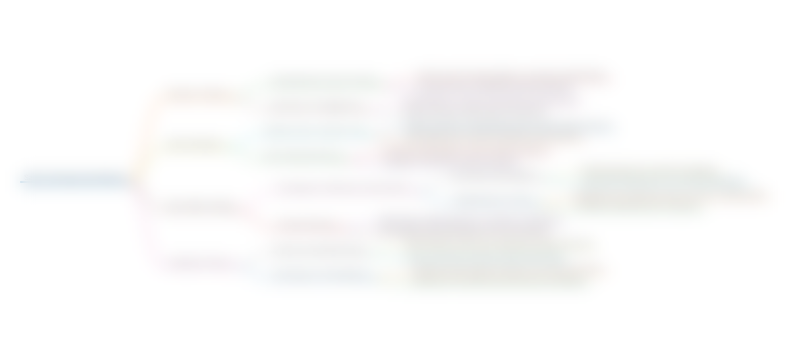
Esta sección está disponible solo para usuarios con suscripción. Por favor, mejora tu plan para acceder a esta parte.
Mejorar ahoraKeywords
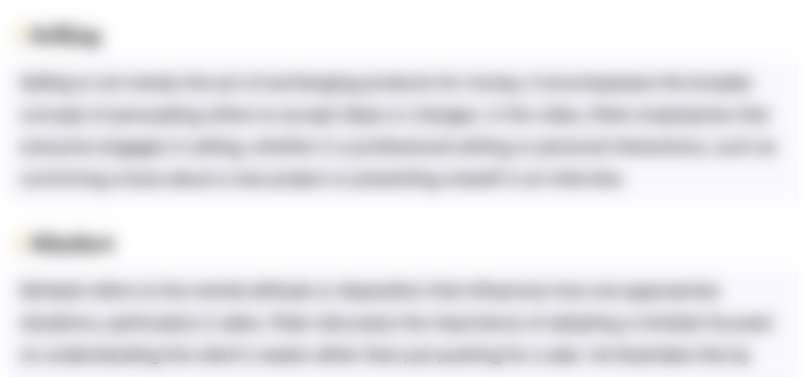
Esta sección está disponible solo para usuarios con suscripción. Por favor, mejora tu plan para acceder a esta parte.
Mejorar ahoraHighlights
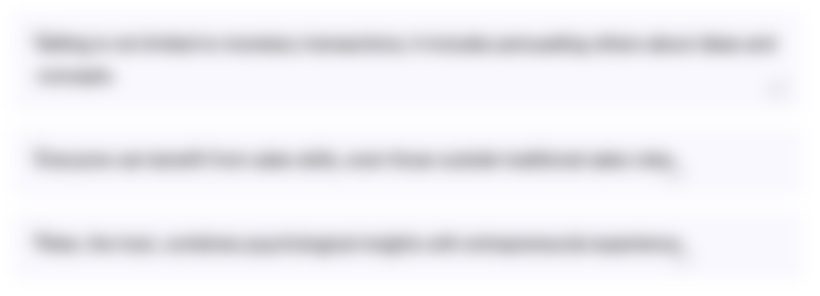
Esta sección está disponible solo para usuarios con suscripción. Por favor, mejora tu plan para acceder a esta parte.
Mejorar ahoraTranscripts
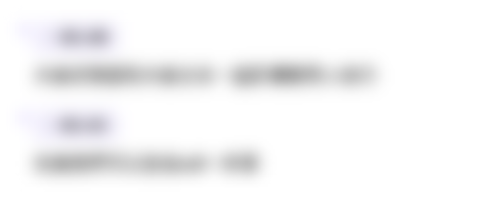
Esta sección está disponible solo para usuarios con suscripción. Por favor, mejora tu plan para acceder a esta parte.
Mejorar ahoraVer Más Videos Relacionados
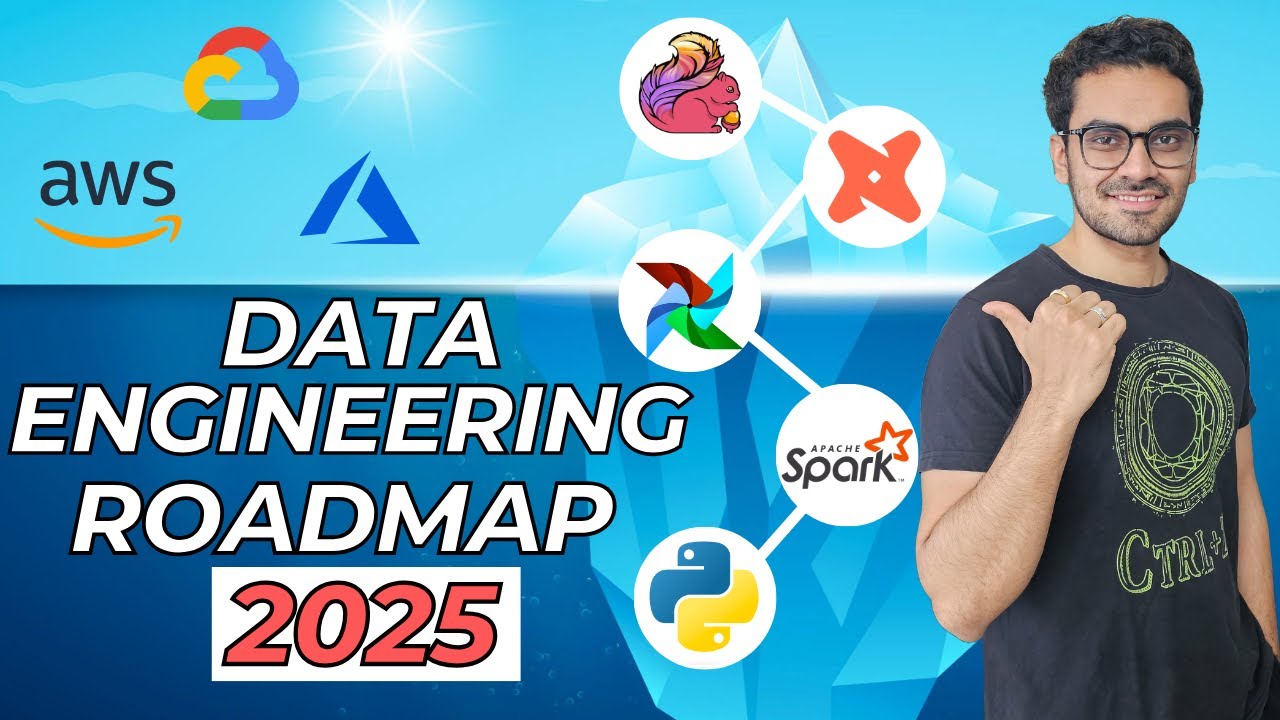
God Tier Data Engineering Roadmap - 2025 Edition
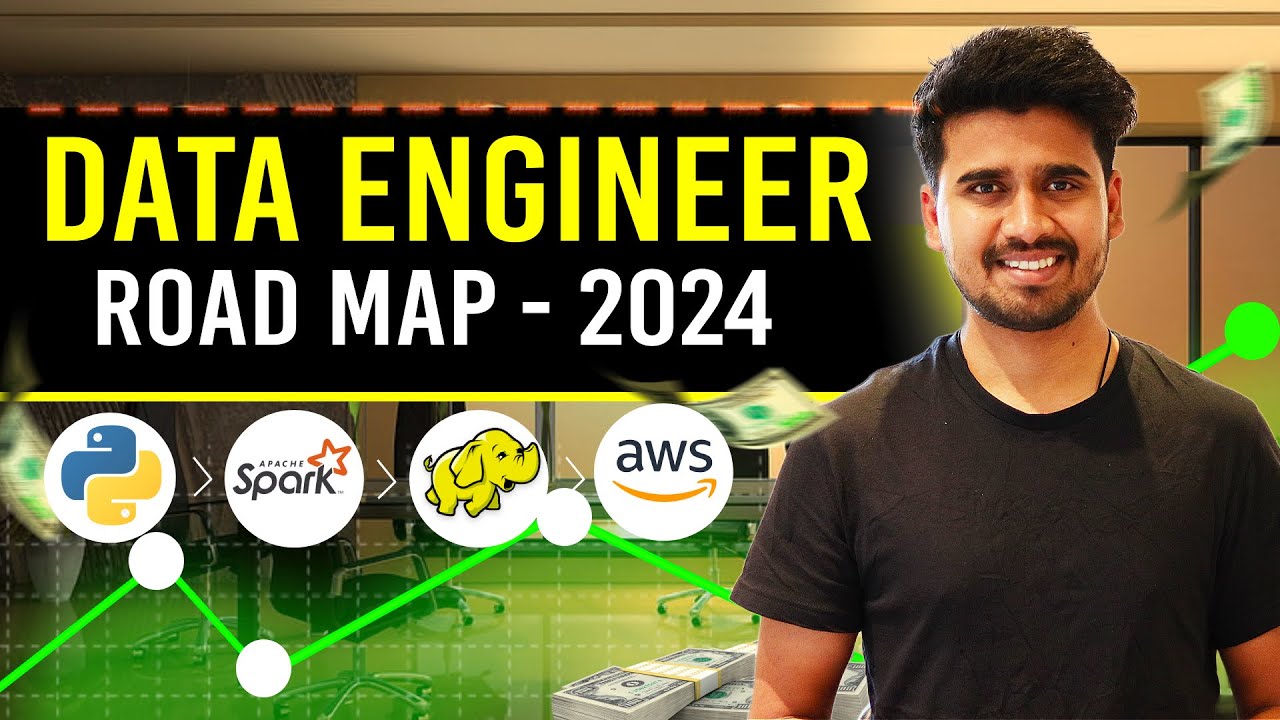
The Ultimate Big Data Engineering Roadmap: A Guide to Master Data Engineering in 2024
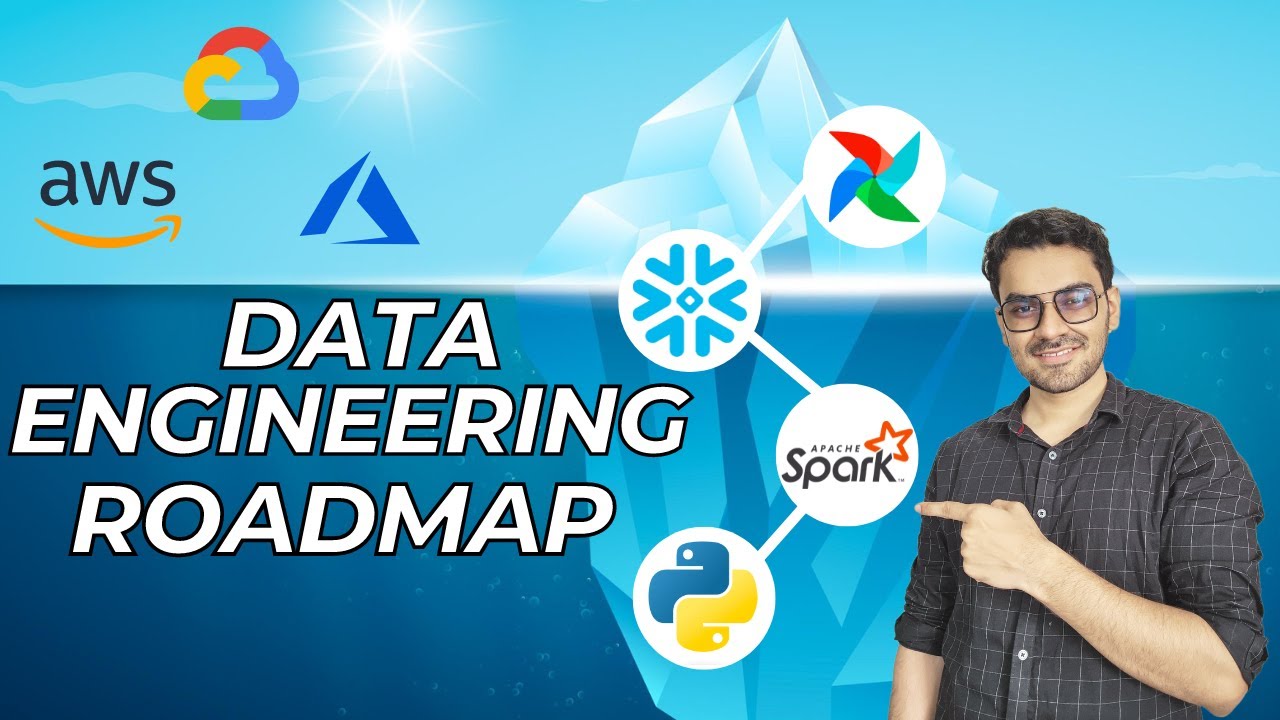
God Tier Data Engineering Roadmap (By a Google Data Engineer)
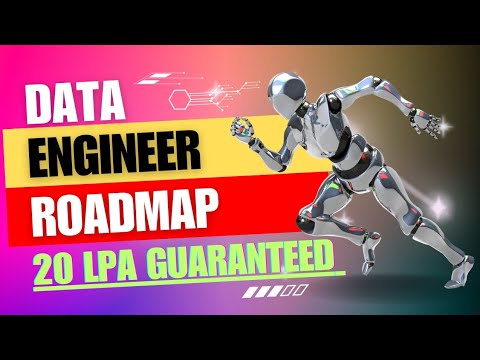
How to Become a Data Engineer in 2024? Data Engineer Complete Roadmap
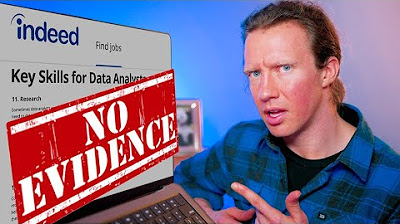
I analyzed 2,765,739 jobs to solve THIS
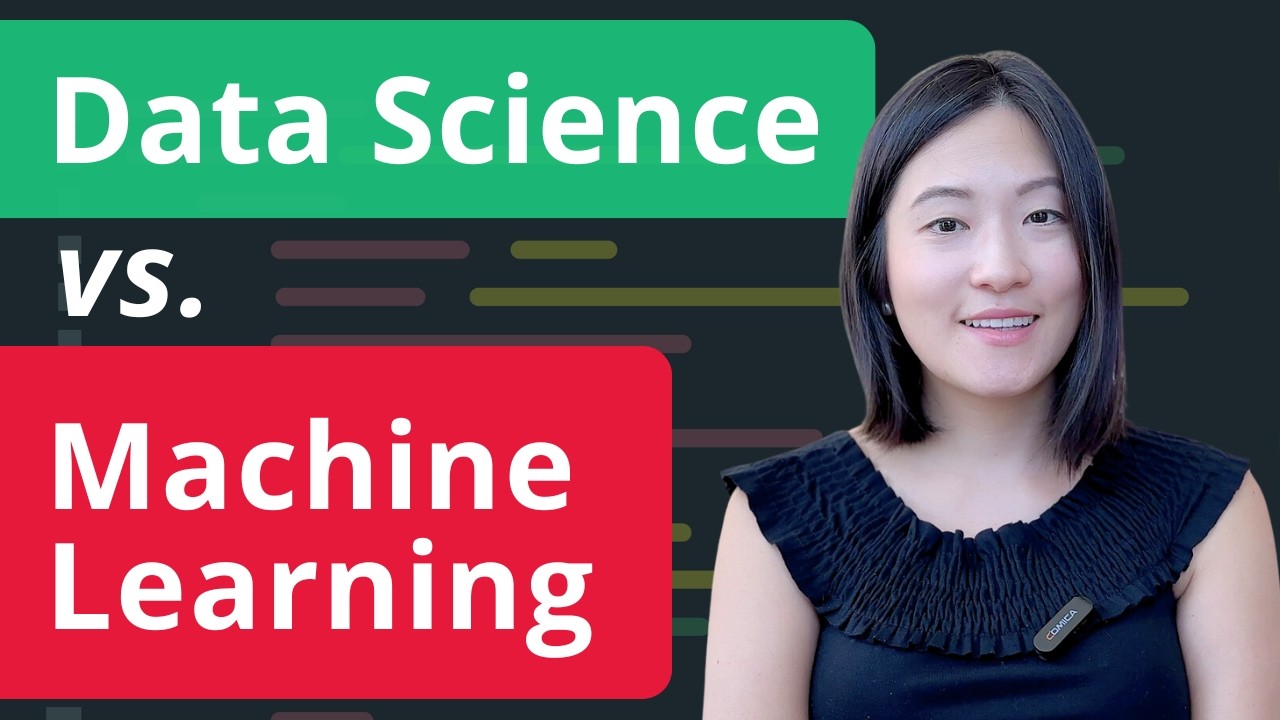
Data Science vs Machine Learning Engineer: Explained
5.0 / 5 (0 votes)