Will Quant Finance End Up Like Data Science
Summary
TLDRIn this video, Dimitri discusses the future of quantitative finance and data science, addressing the evolving educational requirements and industry standards. He critiques the degradation of data science into simple analytics, contrasting it with the more rigorous and structured field of quantitative finance. Dimitri predicts a continued demand for master's degrees in quantitative finance due to the complexity of model building and the high stakes involved, while data science may see a bifurcation into less technical roles and those requiring advanced degrees. He also touches on the cultural divide between data science and traditional statistics, advocating for a more integrated approach to utilizing statistical tools.
Takeaways
- 🔍 The future of Quantitative Finance is being shaped by evolving educational requirements, with a shift from needing a master's degree to potentially entering the field with an undergraduate degree.
- 📈 There's a growing debate on the qualifications needed for a Quant role, with some suggesting that the field is becoming more accessible to those without advanced degrees.
- 🎓 Data science is facing its own challenges with credentialing, as the field has seen a dilution in the definition of what constitutes a data scientist, with some roles now being filled by those with basic data analysis skills.
- 📊 The speaker criticizes the data science field for seeking shortcuts and quick solutions, rather than a deep understanding and rigorous approach to model building and analysis.
- 🏢 Companies are redefining job titles to reflect the changing nature of data-related roles, with titles like 'machine learning engineer' or 'AI specialist' becoming more common.
- 📉 The 2007-2008 financial crisis impacted the perception and requirements of Quant roles, leading to a decrease in the perceived need for advanced degrees in the field.
- 🧑💼 There's a bifurcation in Quant roles, with junior positions handling more basic data analytics and senior roles being filled by those with PhDs or advanced expertise.
- 💼 The speaker predicts that Quant Finance will maintain a higher educational bar compared to data science, due to the critical nature of the models and the financial risks involved.
- 🔧 The data science community is described as somewhat toxic and resistant to integrating traditional statistical methods, preferring to focus on newer techniques and tools.
- ⏳ The speaker anticipates that it may take 20 years or more for the data science field to mature and fully integrate traditional statistical methods into its practices.
Q & A
What is the main concern raised by the subscriber's question about the future of quantitative finance?
-The main concern is about the evolving requirements for entering the field of quantitative finance, particularly whether a master's degree will still be necessary in the future, as the field seems to be opening up to undergraduates.
What does the speaker think about the current state of data science?
-The speaker believes that data science has become a 'massive joke', with the field being degraded to the point where basic data analytics and the use of tools like Excel are being equated with data science.
Why does the speaker think that data science has been degraded?
-The speaker thinks data science has been degraded because many people are looking for shortcuts and quick solutions, leading to a dilution of the skills and knowledge required in the field.
What is the speaker's view on the role of data science in the job market?
-The speaker views data science as a field that is becoming more segmented, with less technical roles not requiring a master's degree and more technical roles starting to require advanced degrees again.
How does the speaker describe the evolution of quantitative finance in terms of job requirements?
-The speaker describes quantitative finance as a more mature field that has already gone through a phase of dilution in job requirements but has now stabilized, with a clear distinction between junior and senior quantitative roles.
What is the speaker's opinion on the necessity of a master's degree in quantitative finance?
-The speaker believes that a master's degree will continue to be a minimum requirement for rigorous roles in quantitative finance, as the field requires a high level of skill and knowledge.
How does the speaker compare data science to quantitative finance?
-The speaker compares data science to quantitative finance as a 'little brother', suggesting that data science is more general and less mature, while quantitative finance is more structured and defined.
What does the speaker think about the culture within the data science and machine learning community?
-The speaker views the culture within the data science and machine learning community as toxic, with a tendency to be anti-statistics and to focus on quick solutions rather than robust, reliable models.
What is the speaker's prediction for the future of data science and machine learning?
-The speaker predicts that data science and machine learning will eventually mature and merge back with traditional statistics, utilizing all the available tools effectively.
Why does the speaker believe that banks and firms are reluctant to hire undergraduates for quantitative roles?
-The speaker believes that banks and firms are reluctant to hire undergraduates because the quality of models produced by less experienced individuals is often not up to the standard required for the high-stakes decisions in quantitative finance.
Outlines
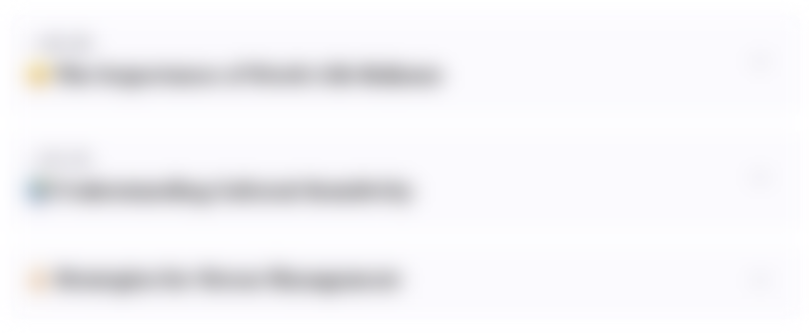
Esta sección está disponible solo para usuarios con suscripción. Por favor, mejora tu plan para acceder a esta parte.
Mejorar ahoraMindmap
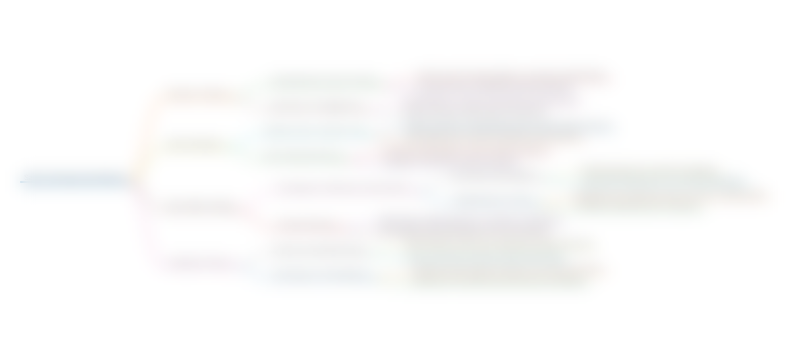
Esta sección está disponible solo para usuarios con suscripción. Por favor, mejora tu plan para acceder a esta parte.
Mejorar ahoraKeywords
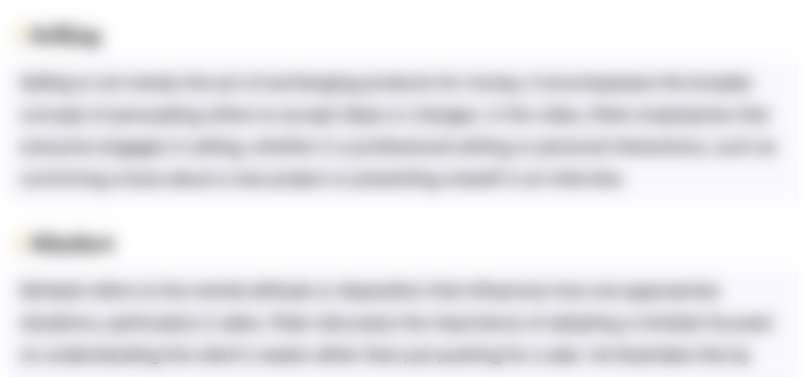
Esta sección está disponible solo para usuarios con suscripción. Por favor, mejora tu plan para acceder a esta parte.
Mejorar ahoraHighlights
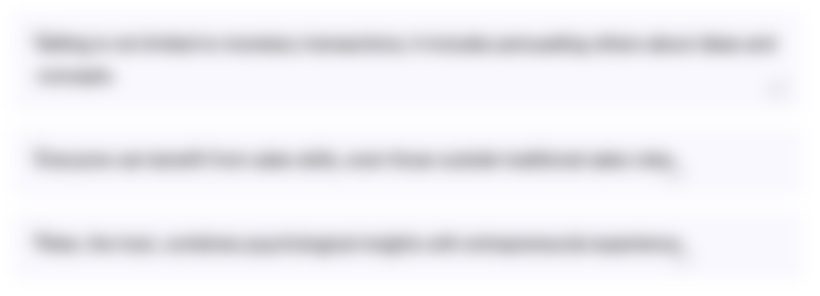
Esta sección está disponible solo para usuarios con suscripción. Por favor, mejora tu plan para acceder a esta parte.
Mejorar ahoraTranscripts
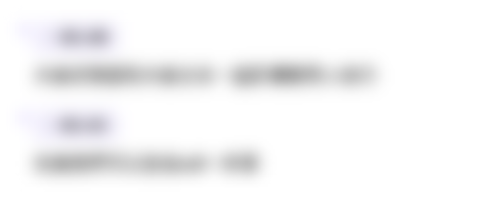
Esta sección está disponible solo para usuarios con suscripción. Por favor, mejora tu plan para acceder a esta parte.
Mejorar ahoraVer Más Videos Relacionados
5.0 / 5 (0 votes)