Statistical and Critical Thinking
Summary
TLDRThis video script emphasizes the importance of critical thinking in statistics, highlighting the need to complement textbook material with supplementary resources. It underscores the significance of active learning, such as writing definitions by hand and analyzing examples. The script warns against common pitfalls like confusing correlation with causation and the unreliability of self-reported data. It also addresses the impact of question order and phrasing on responses, the challenges of non-response and low response rates, and the potential bias in voluntary response samples. Additionally, it provides practical examples to illustrate the correct use of percentages and the distinction between statistical and practical significance.
Takeaways
- 📚 The video is a supplement to textbook material, not a replacement, emphasizing the importance of reading and understanding textbook content.
- ✍️ Writing out definitions and examples by hand is recommended for better memory retention and understanding.
- 🔍 Diagrams and charts should be copied by hand to help organize information and facilitate recall.
- 🧐 The video highlights potential pitfalls in statistical analysis, such as confusing correlation with causation.
- ⚠️ Correlation does not imply causation, a key concept to remember when interpreting statistical data.
- 🤔 The accuracy of self-reported data can be questionable, emphasizing the need for measured data over reported data.
- 🗳️ Loaded questions can lead to biased responses, so it's important to frame questions neutrally to avoid influencing outcomes.
- 🔄 The order of questions can impact responses, suggesting that the sequence in which options are presented matters.
- 🔒 Non-response and voluntary response samples can introduce bias, so it's crucial to consider who is responding and why.
- 📉 Low response rates can lead to unreliable data, so strategies to increase engagement and response are important.
- 🔢 Understanding the mechanics of percentages is crucial for accurate statistical analysis and interpretation.
Q & A
What is the primary purpose of the lecture videos mentioned in the transcript?
-The lecture videos are supplementary to the textbook material, meant to highlight important concepts, provide examples, and clarify potential confusion points, rather than replacing the textbook.
Why is it recommended to write out definitions by hand when studying from a textbook?
-Writing definitions by hand is believed to help the brain process and remember them more effectively.
What is a potential pitfall when dealing with correlation in statistics as discussed in the transcript?
-A potential pitfall is confusing correlation with causation. The transcript emphasizes that correlation does not imply causation, meaning that two variables being correlated does not necessarily mean one causes the other.
How can the way a question is worded impact the responses in a survey?
-The transcript points out that loaded questions, which are biased towards a certain answer, can sway responses. Additionally, the order in which options are presented can influence the choices people make.
What is a non-response in the context of a survey and why is it a concern?
-A non-response occurs when respondents ignore a question or a survey altogether, which can introduce bias into the data by excluding certain perspectives and potentially skewing the results.
Why might self-selected samples in a survey be unreliable?
-Self-selected samples can be unreliable because they are only from people who volunteer to respond, which may introduce bias if those who choose to respond have different characteristics than those who do not.
What strategies can be used to prevent low response rates in surveys?
-To prevent low response rates, surveys should present an engaging argument for their importance, be quick and easy to complete, and potentially offer a reward for participation.
What is the difference between statistical significance and practical significance as outlined in the transcript?
-Statistical significance is achieved when study results are unlikely to be due to random chance, often using a threshold of a 5% chance or less. Practical significance, on the other hand, refers to whether the findings make a meaningful enough difference to be considered useful or worth implementing.
How can percentages be misleading in statistical analysis as discussed in the transcript?
-Percentages can be misleading if they are not accurately representing the actual data or if they are presented without context, such as not considering the total number of subjects in a study.
Why is it important to understand the mechanics of converting between fractions, decimals, and percentages in statistics?
-Understanding these conversions is crucial for accurate data analysis and interpretation. It ensures that data is presented and understood correctly, avoiding misrepresentations that can lead to incorrect conclusions.
Outlines
📚 Textbook Study and Video Lectures
This paragraph emphasizes the importance of textbook study as a foundational step in learning statistical and critical thinking. It suggests writing out definitions by hand to aid memory, reading examples to reinforce understanding, and copying diagrams or charts to organize information. The role of video lectures is to supplement textbook material by highlighting key points and providing examples, rather than replacing the textbook. The paragraph also introduces the concept of pitfalls in statistics, such as the common mistake of confusing correlation with causation.
⚠️ Pitfalls in Data Analysis
The second paragraph delves into potential pitfalls in data analysis, particularly the misuse of correlation and causation. It uses the example of wet parking lots and rain to illustrate that correlation does not imply causation. The paragraph also warns against relying on self-reported data, which can be inaccurate, and stresses the importance of physical measurements for more reliable data. Additionally, it touches on the issue of loaded questions and the order of questions in surveys, which can influence respondents' answers.
🔍 Further Data Analysis Pitfalls
This paragraph continues the discussion on data analysis pitfalls, focusing on the dangers of non-response and voluntary response samples, which can introduce bias into the data. It also addresses the issue of low response rates and suggests strategies to prevent them, such as making surveys engaging, quick, and offering rewards for participation. The paragraph concludes with a warning about misleading percentages and the importance of understanding how percentages are used and calculated in statistical contexts.
👀 Observational Data and Bias
The fourth paragraph contrasts the reliability of observational data versus self-reported data, using the example of hand washing habits in public restrooms. It highlights the potential for bias in self-reported data due to social desirability and suggests that observing behavior directly can yield more accurate results. The paragraph also examines the potential for bias in research funding sources and the importance of considering the source of study funding when evaluating study outcomes.
📊 Statistical Significance vs. Practical Significance
This paragraph introduces the concepts of statistical significance and practical significance. It explains that statistical significance refers to the likelihood that study results are not due to chance, while practical significance pertains to whether the results are meaningful enough to be useful in real-world applications. The paragraph also discusses the importance of understanding the difference between these two types of significance and provides examples to illustrate the concepts.
🔢 Fractions, Decimals, and Percentages
The sixth paragraph focuses on the mathematical relationships between fractions, decimals, and percentages. It provides an example of converting a fraction to a decimal and then to a percentage, emphasizing the importance of understanding these conversions in statistical analysis. The paragraph also discusses the interpretation of percentages in the context of people or items, suggesting that results should be rounded to the nearest whole number when dealing with discrete entities.
❓ Suspicious Percentage Claims
The final paragraph addresses the potential for suspicious or incorrect percentage claims in research findings. It uses an example of a treatment's effectiveness in a small group to illustrate how percentages can be misused or misreported. The paragraph emphasizes the importance of understanding the mechanics of percentages to correctly interpret and question research findings.
Mindmap
Keywords
💡Statistical Thinking
💡Correlation
💡Causation
💡Pitfalls
💡Sample
💡Population
💡Voluntary Response Sample
💡Non-Response
💡Question Order Effect
💡Percentage
Highlights
Videos serve as supplementary material to textbooks, not replacements.
Importance of reading textbook definitions and examples thoroughly.
Writing out diagrams and charts by hand to aid memory and understanding.
Videos help highlight textbook information and provide examples.
Analyzing data while avoiding pitfalls such as confusing correlation with causation.
Correlation does not imply causation, exemplified by wet parking lots and rain.
Physical evidence is required to justify causality, not just statistical analysis.
Potential inaccuracy in self-reported data versus measured data.
Loaded questions can lead to biased responses.
Order of questions can influence responses, as shown in traffic versus industry pollution example.
Non-response and voluntary response samples can introduce bias.
Low response rates can create bias and affect the usefulness of data.
Strategies to prevent low response rates include engaging surveys, brevity, and rewards.
Misleading percentages can occur if not properly understood or calculated.
Understanding the difference between statistical significance and practical significance.
The flaw in assuming causation from correlation, as seen in the sour cream and motorcycle fatalities example.
Converting fractions to decimals and percentages with examples.
Interpreting percentages in context, such as rounding to nearest whole numbers for people.
Suspicion raised by a researcher's claim of a treatment working in 53% of a small group.
Transcripts
this video is about statistical and
critical thinking and these lecture
videos are meant to be supplementary to
the textbook material and so they're not
meant to replace
anything you might be able to get from
the textbook but just go alongside it to
help highlight some important things
give some examples and so let's be
also very clear that the first step we
do in any section or topic should be to
read the textbook now that involves
probably a few steps that is write out
all definitions by hand
when you write them with your hand your
brain does better processing remembering
them
and then secondly we need to read all
the examples and note or write any
questions or points of confusion often a
textbook will have all kinds of examples
in them we should be reading them to
understand and reinforce the definitions
or topics being discussed
in the section and then thirdly write
out
that is copy by hand any diagrams or
charts often these can help organize
information and so we have to have those
written out so that our brain remembers
them and knows where we can go back and
find them
and so that's always a step we should do
so this this kind of like taking notes
from the textbook again is the
the main task in any topic or section
and then these videos will help
highlight some of the information in the
textbook
so then secondly let's talk about some
important highlights in this topic or
section
now we're going to talk about analyzing
data
and specifically some potential pitfalls
that could occur okay pitfalls are like
dangers right
and in the case of statistics
we have to be very careful about how we
handle certain things such as
correlation
and
causation
so specifically here correlation
does
not
imply causation
okay so first of all these are a lot of
fill in the blanks kind of definitions
and examples that you'll find in these
video notes and the the note guided or
templates that you can use to fill in
the blank should be available so you can
actually follow along and fill in
yourself
but they do
purpose to highlight and emphasize some
of these definitions now correlation
is different than causation as noted
here in this first potential pitfall for
example
wet parking lots may correlate
that is have a connection with rain but
they don't cause the rain right if you
drive by and see a parking lot that is
totally wet
it may be reasonable to conclude that it
rained recently and there may be other
clues that could help
you know inform that conclusion but
just because the parking lot's wet
doesn't mean the parking lot caused the
rain it simply goes with the fact that
it may have range right
and so we have to be careful not to
confuse these it's
in statistics often important to find
correlations or things that have
connections
but we can't jump too much from that
to some big you know causal conclusion
here that may or may not be true and so
as our author notes here secondly
statements about causality that is
what's causing something else can be
justified by physical evidence
but not by statistical analysis and so
we just have to be very careful not to
lean a little too far over our skis on
the statistical side and
take from a correlation which may
actually occur
and conclude from it some
specific causal relationship they may
not actually
be impliable
secondly
we also have to be careful
so first was that correlation does not
cause or does not imply causation
secondly
we could have a potential pitfall if
sample data is
reported
reported instead of
measured
okay so one example of this would be if
you're trying to do some kind of
statistical analysis that involves
getting
people's weights so let's say you know
the weights of everyone in a classroom
or everyone in a building or something
well if you simply ask people to report
their weight
as you can probably imagine we all kind
of want to look and sound a little
better than we actually are so you may
get inaccurate numbers
people may round down or round up
depending on the scenario right
whereas if you were to measure it such
as have an organized study where every
participant is like standing on a scale
the same scale and it's being measured
by a third party independent you know
person
looking at the weight on the scale you'd
end up with a much more accurate
set of of measurements or data
and then thirdly we also have to be
careful of what could be called
loaded questions
okay and loaded questions are
ones which give away or kind of lean
in a certain direction
and our author gives us a really good
example of one so there was a
set of questions given where the first
one said
should the president have the line item
veto to eliminate waste
now 97 percent of respondents said yes
okay but then when the question was
phrased should the president have the
line item veto or not
only 57 said yes
so notice the difference between the way
these two questions were asked the first
one went a little further it was loaded
with some extra information right should
the president have line item video veto
to eliminate waste
okay well you're you're loading the
question with a specific
purpose or application that may sway
people more than the initial part of the
question right maybe people say should
they see this question and think well
yeah of course we should eliminate waste
so i'm going to say yes to that whereas
the second question
simply said should the president have
line item veto or not that's a totally
different way of looking at that same
kind of question but it's not loaded
with any specific application and you
can see there's a difference in
responses right that to the second one
only 57 yes so so maybe that was getting
more at the responses pertaining to the
line item veto uh ability as as opposed
to the you know desire to eliminate
waste
and next we should discuss or consider
the order
of questions
that are provided
so again our author gives us another
example the first question reads
would you say that traffic contributes
more or less to air pollution than
industry
well in response to that question 45
percent blames traffic
and notice that was the first
possible factor mentioned in the
question
whereas
only 27 percent
blamed industry and that was the last
thing or second thing mentioned and so
the order in which things are mentioned
in a question has the potential to
impact the way people respond to them
or you'll notice in the second
instance of this
this time when the question was asked
would you say that industry contributes
more or less to air pollution than
traffic well notice this time
the order was reversed so this time
industry was was mentioned first
and then
traffic was mentioned second and guess
what the actual responses were reversed
this time
24 blame traffic 57 blamed industry and
so it could be the case in in this
scenario this example that the order of
the items mentioned the question
actually impacted the responses and so
you know we have to be clear when
gathering and working with data and we
want to just be aware for these pitfalls
that could give us totally different
outcomes and analyses simply based on
the way a question may be worded
next we have to consider
non-response
if we have respondents ignoring a
question or ignoring a survey
then it's quite possible
that having these non-responses may
already filter
the data by what types of people respond
or not right if people are less likely
even to respond then we're not gathering
or capturing
their authentic response and that may
influence
the overall results in a study or
a data gathering process
and secondly recall as in the textbook
here something called voluntary response
sample or self selected sample
can be unreliable or biased for the
exact same reason so a self
self-selected sample would be something
where you're getting
feedback and it could be authentic
feedback
but it's only feedback from people who
volunteered
to respond to the question well the very
fact that they may have volunteered
could have been influenced by some other
characteristic that's going to skew the
data or give it some bias so we have to
be very careful about non-responses
which is another way of saying voluntary
response sample as mentioned by our
author
next we should be aware or be careful
with
low
response
rates
so again if you're trying to gather data
from people and you're requiring some
kind of response to communicate that
if you have a very low response rate
um then that's a fair reason to to
wonder whether the the the information
gathered it's going to be useful or not
and so
as noted by our author this can create
bias in the results but to help prevent
low response rates there are a couple or
a few strategies
that could be used so um first of all we
could serve a survey should present an
engaging argument for its importance um
surveys are ignored more often than
they're embraced likely very likely
and so if we can get an engaging or you
know context or motivation for the
survey that can actually help a lot
or secondly a survey should should not
be very time consuming right quick and
easy is what people like and that's a
more likely to get a response
than if it's some you know 30 page
or even just three page survey we want
to be
conducive to people getting it done
quickly
or thirdly
it's helpful to provide a reward for
completing a survey and this is often
done in studies
where
sometimes respondents may even get paid
for their feedback it just depends on
the scenario but these are things to
keep in mind uh when we're trying to
gather data that low response rates can
cause problems so we should be careful
with them uh and by the way what is
actually low or not could depend on the
context and so those are all
also just considerations
that we'll be going over as we encounter
these
next we should consider
misleading percentages
misleading percentages now
the mechanics of percentages is not
something uh
taught exclusively in this class so
likely at this point you've already
worked with percentages we'll review
some of it
with some examples here shortly but
percentages are important now note that
a percent
really means divided by a hundred this
is just a strict mathematical definition
four percent we have to be careful that
that's how it's being used in any
statistical context
and secondly let's recall that
converting between decimals percentages
and fractions
is important sometimes we'll have to
actually do that in statistics
and we might want to make sure it's done
correctly
now let's spend a little time going over
some examples now these examples will go
with the content of this topic or
section and
some of it may you know touch on things
that weren't explicitly highlighted in
this video lecture so far there may have
been things from the textbook that
you've already been reading or taking
notes on and so this will help you know
give you some more to think about and
process in these topics all right so
this is coming from our author and
our first question says in a survey of
1048 adults conducted by bradley
corporation
subjects were asked how often they wash
their hands when using a public restroom
and seventy percent of the respondents
said always
okay so we want two parts here for part
a identify the sample and the population
with the definitions of sample and
population given in the textbook i
assume you've already written them out
by hand in your notes and you know
thought through some of the examples
printed in the book
but we want to understand these clearly
so a sample would be
a potentially smaller amount of people
than a population it doesn't have to be
smaller but it typically is smaller than
the larger or full
population a sample like you may imagine
if you go to a restaurant or something
and you sample some food you're just
getting a small portion of maybe what
they offer right
so let's identify in this case what the
sample and population are well in this
case a survey was given to
1046 adults so the sample
in this case would be 1046.
that's how many adults were surveyed
right
but the population
would be
all
adults
or you know you could say any adults
right well obviously there are a lot
more adults than 1046
so
the sample is in this case much smaller
but it's supposed to just get a smaller
representative
slice of what the overall population
may be or maybe like
and then secondly
with this question why would better
results be obtained by observing
the hand washing instead of asking about
it well this goes up to one of our
potential pitfalls we noted above which
is the the reporting as opposed to
measured
so when people report that they wash
their hands
there's probably an inclination to say
you did because it's it's probably a
little more noble looking or or
respectable looking to say you wash your
hands right and so that may sway the
results where people are maybe more
inclined
to to say they did just because it looks
or sounds better so
why would better results be given by
observing because reporting
results
can skew them as opposed to observing as
we noted above
secondly determine whether a given
source has potential to create bias in a
statistical study
in an article
in journal of nutrition okay so this is
a real publication here noted that
chocolate is rich in flavonoids
the article notes quote regular
consumption of foods rich in flavonoids
may reduce the risk of coronary heart
disease end quote
the study received funding from mars
incorporated the candy company and the
chocolate manufacturers association
okay so think about this for a second
right
do these candy companies have an
interest in people thinking that their
candy might actually be nutritious well
of course right that may
make people more inclined to buy and eat
it well if those people got if those
candy companies funded
this study
and the study made that conclusion that
these flavonoids found in their candy
may actually be good for you
there could be reason to wonder if
there's some bias in these results right
because it's it's more likely that they
wanted those results and they they
funded the study
thirdly let's determine whether the
sampling method appears to be sound or
is flawed
okay so first in a survey of
1368 subjects the following question was
posted on the usa today website in your
view are nuclear plants safe
end quote the survey subjects were
internet users who chose to respond to
the question posted on the electronic
edition of usa today
so we're trying to determine does this
seem to be appear to be sound or flawed
well
if you understand and kind of imagine
yourself in this context
these were people who chose to respond
right
to this electronic question posted in
this specific place this electronic
edition of usa today and so this is what
you would call self-reporting or
voluntary response sample
and so this could be dangerous and it
could cause biased results as noted in
our pitfalls above
or part b in a survey of social media
usage the pew research center randomly
selected 2002 adults in the united
states
well since it was randomly selected
this kind of construction of this sample
actually would be more or more reliable
we could say it appears to be sound
next let's describe the difference
between statistical significance and
practical
significance now to be clear we are
going to study statistical significance
in a more rigorous way and definition
later on in the course this is just an
introductory you know section on the
topic of understanding statistical
thinking so
the definitions provided in the textbook
which you should have written out and by
hand in your notes
do give just kind of a general sense of
it and so statistical significance is
achieved when a study produces results
that are unlikely to occur due to random
chance okay now that's the general
meaning of it a commonly used criterion
is that five percent chance or less of
random occurrence is acceptable again
this is going to be studied in more
depth later on but the point is
statistically significant has a
mathematical meaning to it
and it involves the results get uh given
in the study
not likely being just a chance like oh
we just flipped a coin and that's how it
ended up right it's more likely that it
was not chance it was due to some other
actual factor
now practical significance occurs when a
treatment or finding makes enough of a
difference to justify its use
so for example an arduous diet that only
sheds a couple of pounds may not be
practically significant to people right
and so you could say well this diet
showed that it had statistical
significance to reduce you know weight
in people they lost two pounds or
something well okay even if it's
statistically significant practically
significant means people actually care
about the result enough to try it so
want to make sure and understand the
difference between those two things
next let's identify what's wrong with
the following quote
in recent years there has been a strong
correlation between per capita
consumption of sour cream and the
numbers of motorcycle riders killed in
non-collision accidents therefore
consumption of sour cream causes
motorcycle fatalities
okay hopefully this one's jumping out at
you loud and clear if not you can go up
and review one of our pitfalls called
correlation does not
imply causation that was one of the
things we noted above
and in this case it's exactly what's
happening right there's a correlation
apparently between sour cream
consumption and these fatal motorcycle
accidents so okay what is the actual
cause of that well the statistics can't
really tell us
but what we can say is that
the correlation or association between
two variables does not mean that one of
the variables is the cause of the other
correlation does not imply causation
next let's talk about or review some
work with fractions and decimals and
percentages here so we have an example
that says convert
559 out of 1165
to the following forms
we want to write it as a fraction a
decimal and a percentage well as a
fraction we can take the phrase out of
and use that kind of like a fraction bar
and so if it says 559 out of 1165 we can
say
559
is the numerator
and then the
1165 is the denominator so out of
basically means over in a fraction set
up right
now we can convert this to a decimal
simply by using a fraction as division
right that's what a fraction means and
so we could take this fraction 559
over or out of 1165
and in a calculator perhaps write that
as 559 divided by
1165
now we weren't given any specific
instructions for rounding in this
example sometimes you may see actual
instructions for it and we're actually
going to talk about certain rounding
standards and conventions more as we go
on in later sections so since it wasn't
mentioned i'm going to just say for this
one let's round to 100
and the decimal point form when we
actually crunch this fraction in a
calculator
it's a long decimal but if we round it
to a hundredth uh so we'll say
approximately
since it's rounded
uh point or zero point
four
eight
if rounded to a hundredth
uh you could you know round it to
different values
just based on what was uh requested now
we convert a decimal to a percentage by
understanding decimal place values right
two places to the right of a decimal
which is where our value stops here is
called the hundredths place
well that's exactly what a percent
means is out of 100 and so 0.48 or 0.48
is the definition of 48
and so again this is a rounded decimal
and so i'm that consequently the
percentage would also be rounded um
but nonetheless these are how these two
or three definitions relate fractions
decimals and percentages of course you
can go back and forth or different
orders from one of the other depending
on what you're given and where you're
trying to go with it but this is how
they're connected
similarly for number seven the question
says how would we calculate and
interpret quote 48
of 342 people end quote
well we have to convert sometimes um
english to mathematics so when you say
48
of
342
that's like saying
the decimal form of 48 which is
0.48
of
342 could mean times
342. of in this context would be
multiply and so you could crunch these
two numbers
and the result that we could get after
just multiplying 0.48 times 342 on a
calculator would be
164.16
okay so now that was the calculate part
but then the next part said and
interpret well if you're talking about
people which is the context of this this
number here
you can't represent or reasonably
describe 0.16
of a person
0.16 so
rather we just round up to the nearest
whole number when we're talking about
people or you know items that can't be
divided up so obviously animals would be
something similar too but so in this
case you would describe it or interpret
as 164
people just rounding to the nearest
whole number
and then
lastly here what is suspicious about a
researcher suggesting a treatment works
in 53
of a group of 20 subjects
well the more we work with percentages
the more comfortable i think we'll get
with them but in this case when you're
talking about a
a set of 20 subjects
let's remember first of all for a
percent
a percent is defined by a part
over or out of
a hole so part out of the whole thing
right and you can actually crunch these
numbers out to get a percentage
a percentage for it so
in this case
there were 20 subjects which means the
whole
was 20. so if i have 20 as my
denominator
then the part would be you know how many
respondents out of 20 i'm trying to
represent well in this case
we're suggesting that for those you know
for whom this treatment works that would
be the part we're talking about here
we're suggesting that somehow the part
number out of 20
should equal 53 or perhaps rounded to it
but the point is
it would be roughly 0.53 right well if
we start messing around with some some
percentages if i had
10 out of 20
that would be zero point or just point
five
zero 0.5
or 0.50 you can always add a zero onto
the right without changing the number of
a decimal okay so point 50 that would be
50 that's close to our target of 53
percent but it's too low so let's go one
higher well the next higher would be if
it worked for 11 out of 20 uh subjects
right you can't have 10 and a half so go
to 11 out of 20. well it turns out
that's
0.55
so interestingly we skipped right over
the reported result of 53 percent and
we're suggesting that the numbers can't
even give you that result
you can get a 50 percent or 55 percent
but not this 53
given so that's reason to be a little
suspicious about where the
53 or the 0.53
came from you know were they rounding
something else what's going on with
these percentages so understanding the
mechanics of percents helps us use them
and
interpret them and be wary of them
correctly
Ver Más Videos Relacionados
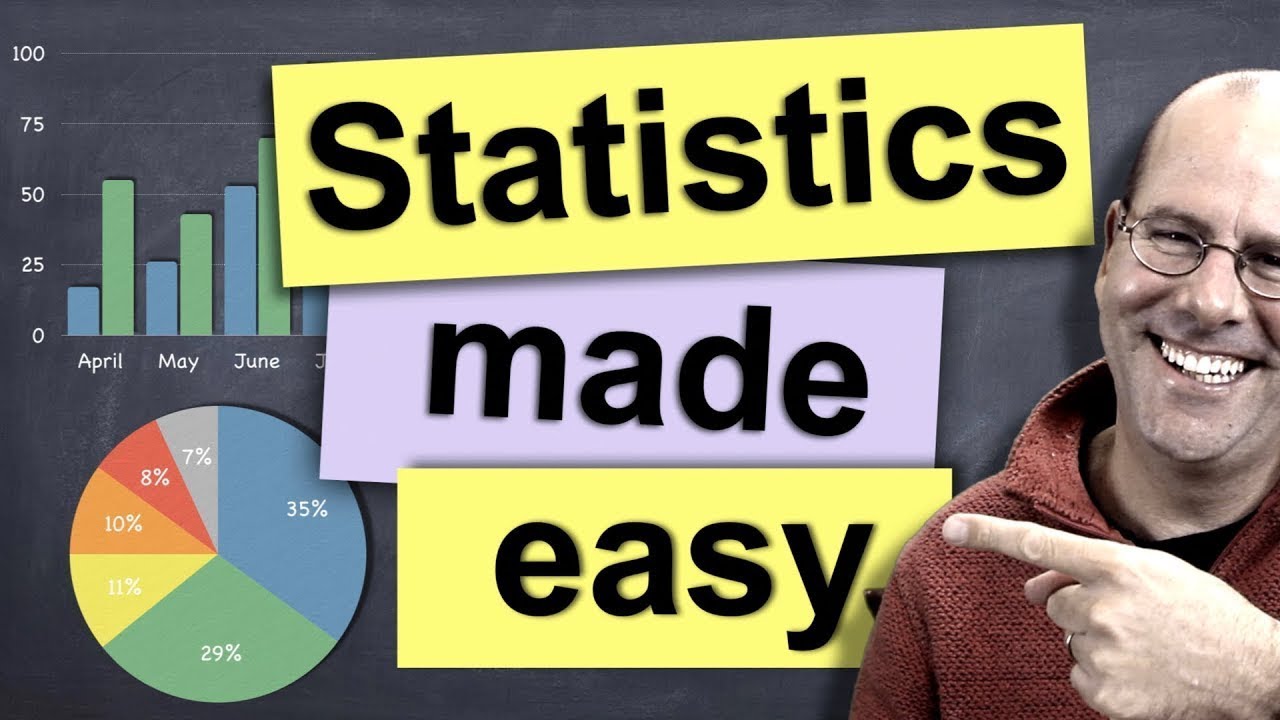
Statistics made easy ! ! ! Learn about the t-test, the chi square test, the p value and more
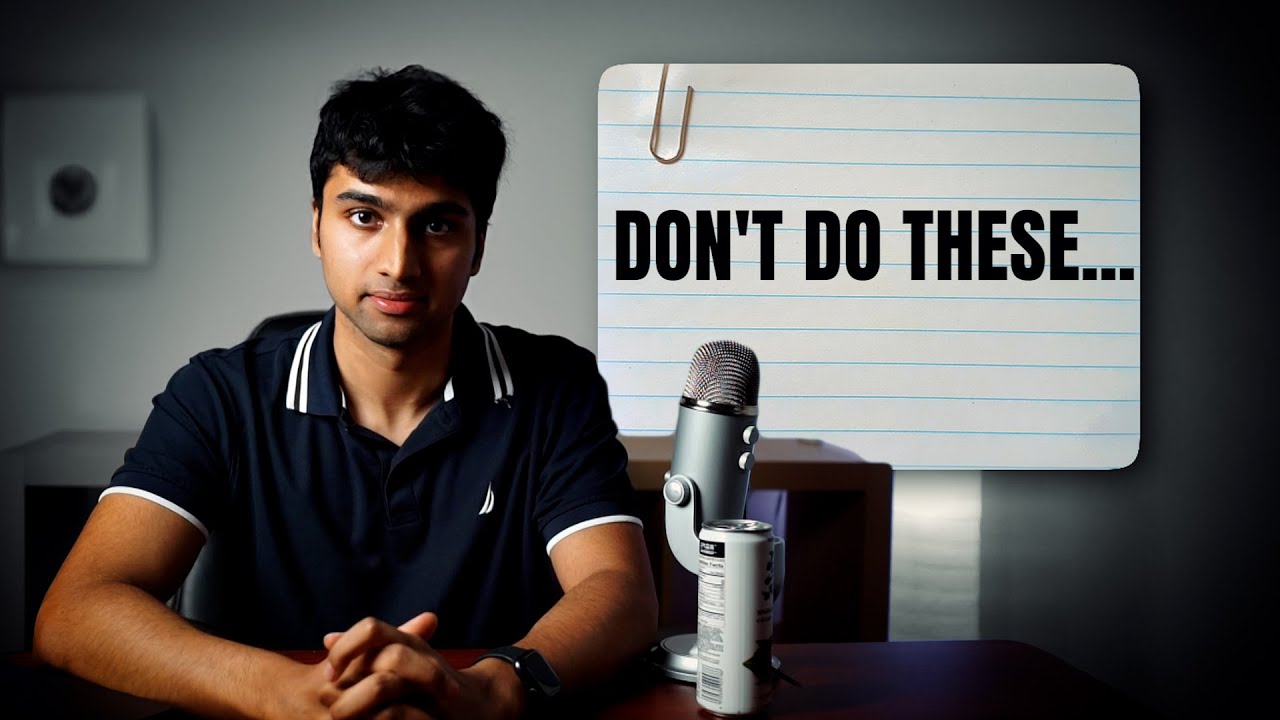
You NEED to AVOID these Mistakes as a Data Analyst | raw truth
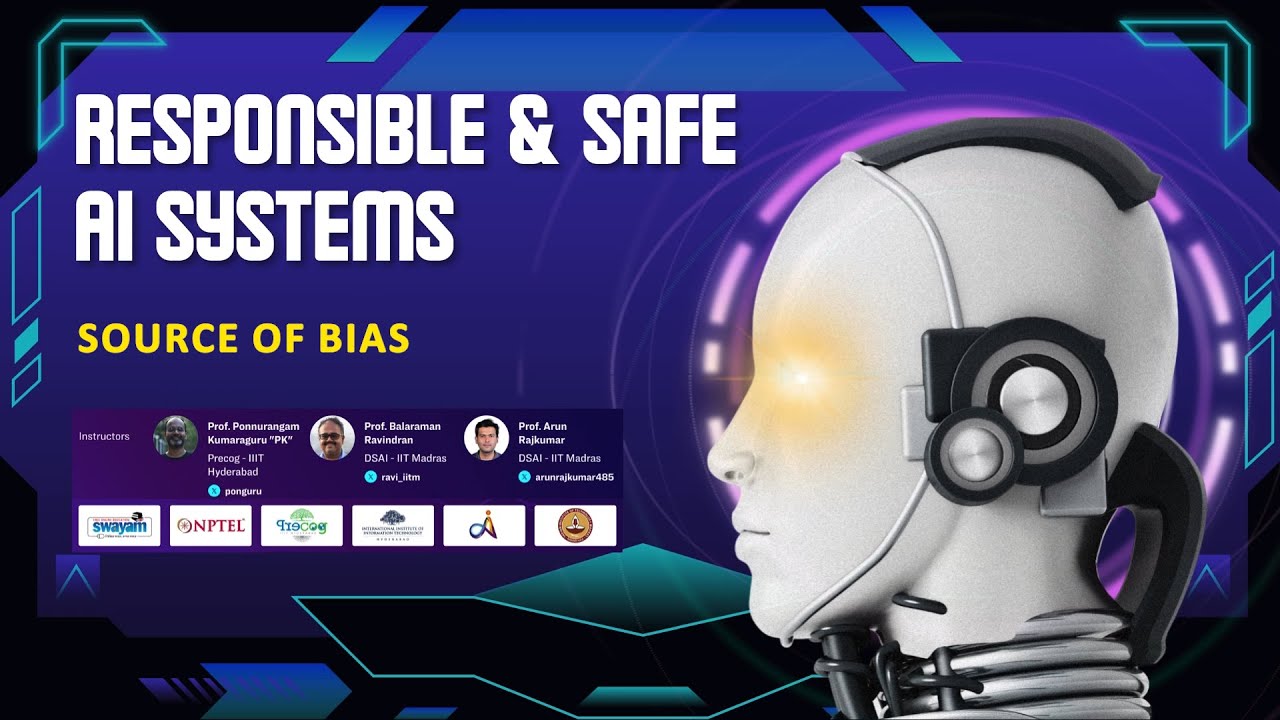
Source of Bias
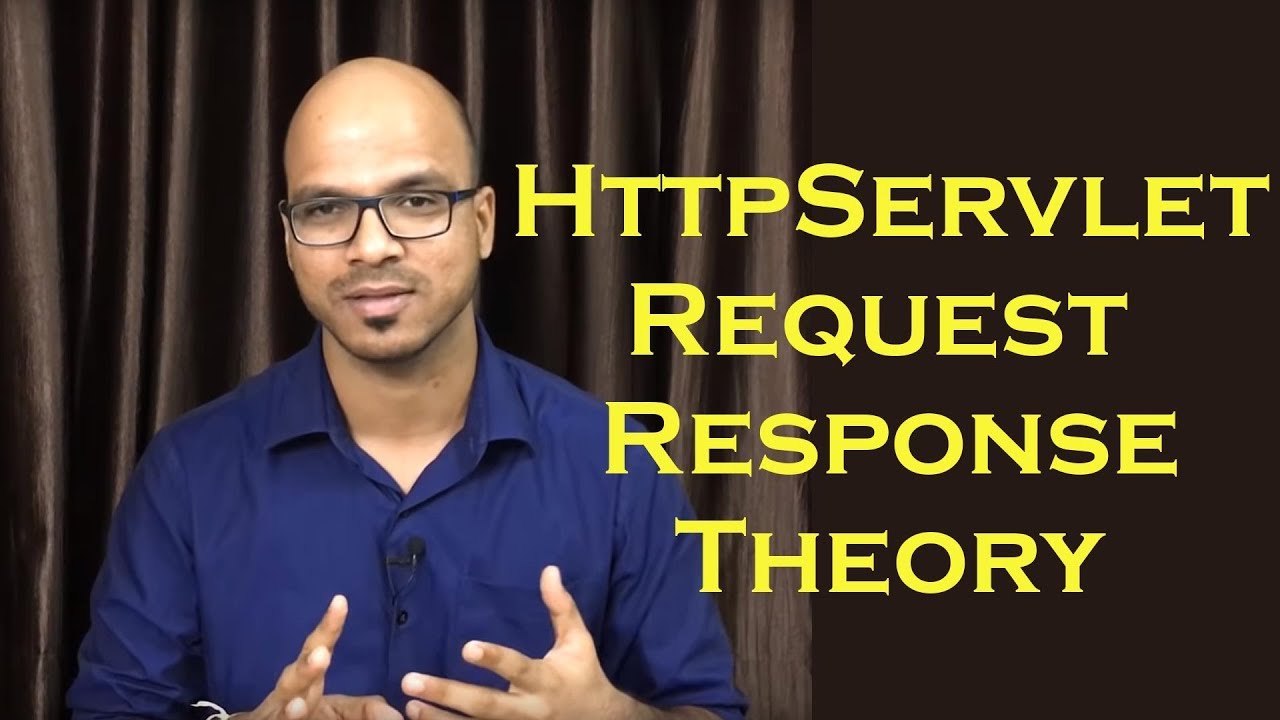
HttpServletRequest and HttpServletResponse Theory
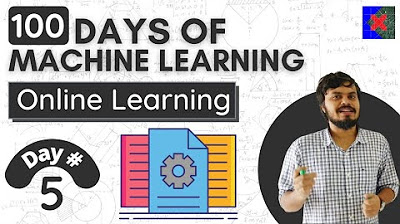
Online Machine Learning | Online Learning | Online Vs Offline Machine Learning
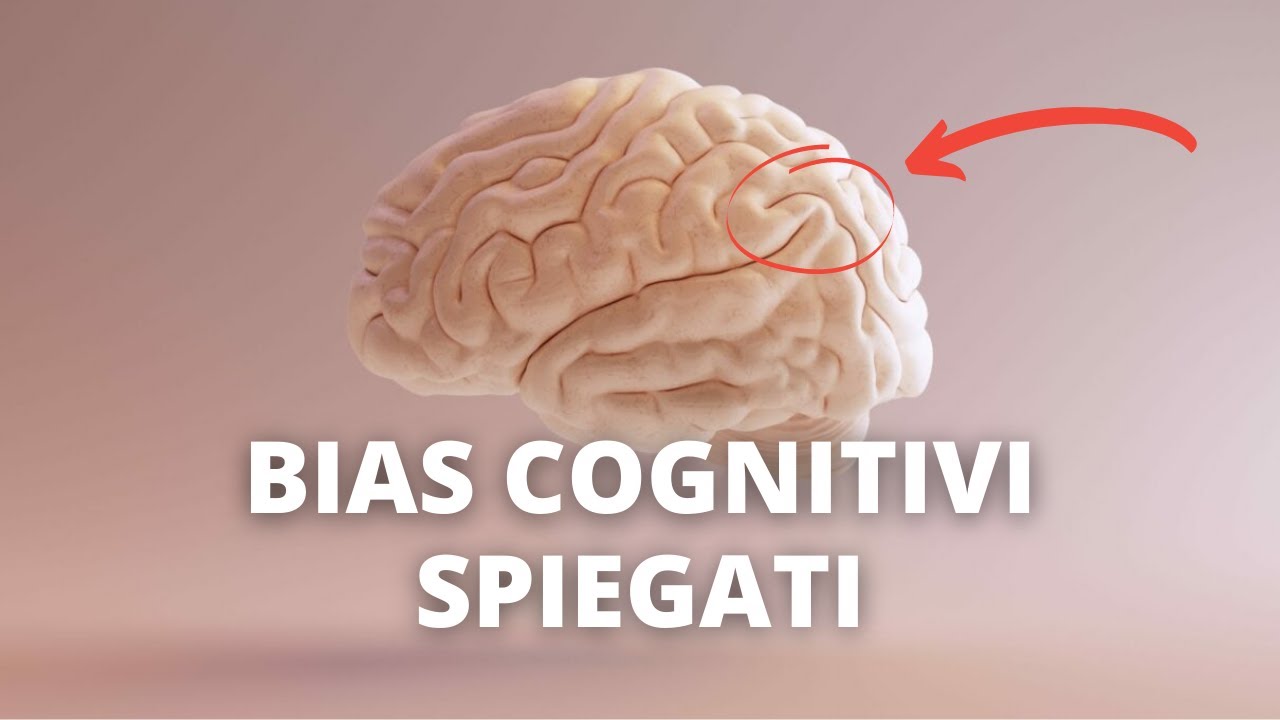
BIAS cognitivi: cosa sono (con esempi)
5.0 / 5 (0 votes)