Maggie Little: Data & Digital Ethics
Summary
TLDRIn this talk, Senior Research Scholar Maggie Little explores the ethical implications of the data and digital revolution, akin to the agricultural revolution 10,000 years ago. She discusses the massive amounts of data generated through new technologies and the ethical concerns surrounding privacy, surveillance, and data usage. Little also addresses the revolution in data analytics, including AI and machine learning, and the potential for bias and mistrust. She emphasizes the importance of designing, deploying, and governing these technologies responsibly to preserve privacy, advance justice, and maintain public trust.
Takeaways
- 🌐 The data and digital revolution is compared to the monumental shift from hunter-gatherers to agriculture 10,000 years ago, emphasizing its transformative impact on society.
- 📈 The revolution is powered by two main components: the data revolution and advancements in data analytics, particularly artificial intelligence (AI) and machine learning.
- 🔍 The data revolution involves massive amounts of data gathered through new technologies like drones with facial recognition and smart cities, as well as organic data from consumer digital activities.
- 🤖 AI and machine learning allow for the discovery of hidden patterns in data that traditional statistics might miss, offering new insights but also posing ethical challenges.
- 🔒 Privacy rights are a central ethical concern in the data revolution, with questions around the collection, storage, and secondary use of personal data.
- 🚨 Informational risks, such as the potential for data breaches and de-anonymization, are significant ethical issues that must be managed when handling sensitive data.
- 🏥 The potential for AI tools to improve public health, personalized medicine, and medical imaging is vast, but they must be designed and deployed with careful consideration of ethical implications.
- 🚫 The misuse of AI tools, including mission creep and commercialization, can lead to ethical concerns, especially if the tools are used for purposes beyond their intended design.
- 📊 Ethical biases in AI can occur when algorithms unintentionally reinforce existing prejudices or不公平ly impact vulnerable populations, highlighting the need for careful algorithm design and validation.
- 🌟 Public trust is crucial for the successful deployment of AI in healthcare and other sectors, and transparency, communication, and democratic accountability are key to maintaining this trust.
Q & A
What is the significance of the data or digital revolution compared to historical societal changes?
-The data or digital revolution is considered as momentous for human society as the agricultural revolution 10,000 years ago, which saw humans shift from being hunter-gatherers to settled agriculture.
What are the two main components of the current revolution in the 21st century?
-The two main components are the data revolution and the revolution in data analytics, both powered by the massive increase in computational power achieved in the last two decades.
How has the way we gather data changed due to new technologies?
-New technologies like drone planes with facial recognition and smart cities with sensors have enabled the gathering of massive amounts of data, which is part of the data revolution.
What is meant by 'organic data' in the context of the data revolution?
-Organic data refers to novel forms of data that are generated from everyday activities, such as social media posts, which can be scraped and analyzed for insights.
What is the role of artificial intelligence and machine learning in the data analytics revolution?
-Artificial intelligence and machine learning play a crucial role by enabling the analysis of large datasets to find patterns that traditional statistics might miss, thus enhancing our ability to extract information from data.
What are the potential benefits of leveraging massive amounts of data in public health?
-Leveraging massive amounts of data can aid in public health by generating hypotheses, tracking disease hot spots, and improving personalized medicine and medical imaging analysis.
What are the ethical concerns regarding the collection and storage of data?
-Ethical concerns include privacy rights, the question of who owns the data, the risk of data being used beyond its original intent, and the potential for misuse or commercialization of data.
Why is it important to consider informational risks when dealing with sensitive data?
-Informational risks are important because they involve the potential for sensitive information to be identified or inferred, even from anonymized data, through data aggregation or breaches, which can lead to privacy violations.
What is the 'mosaic effect' in the context of data ethics?
-The mosaic effect refers to the risk of de-anonymization where combining non-sensitive data from one database with sensitive data from another can lead to the identification of individuals and their sensitive information.
How can the deployment of AI tools in healthcare be ethically problematic?
-The deployment of AI tools can be ethically problematic due to issues like inaccurate predictions, biases in algorithms that disproportionately affect vulnerable populations, and the potential for misuse or mission creep where the tool is used for purposes beyond its original intent.
Why is public trust important when it comes to the use of AI in public health?
-Public trust is crucial because without it, people may be less likely to engage with health services or follow public health recommendations, which can have negative impacts on individual and community health.
Outlines
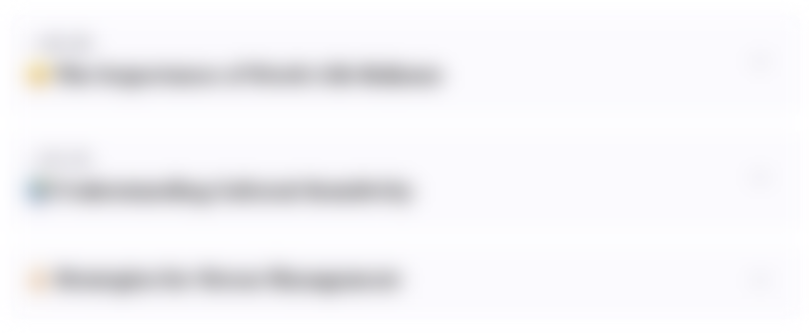
Esta sección está disponible solo para usuarios con suscripción. Por favor, mejora tu plan para acceder a esta parte.
Mejorar ahoraMindmap
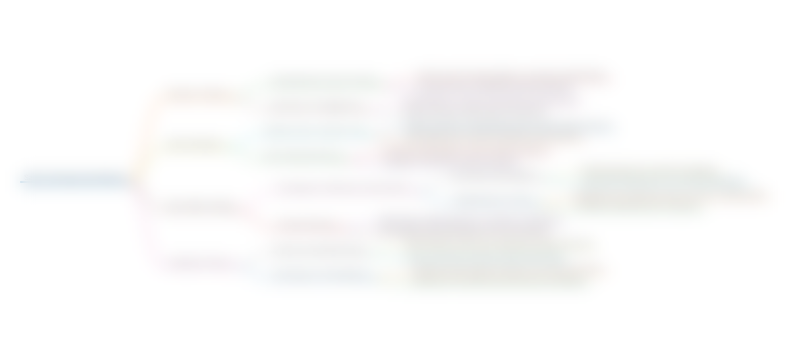
Esta sección está disponible solo para usuarios con suscripción. Por favor, mejora tu plan para acceder a esta parte.
Mejorar ahoraKeywords
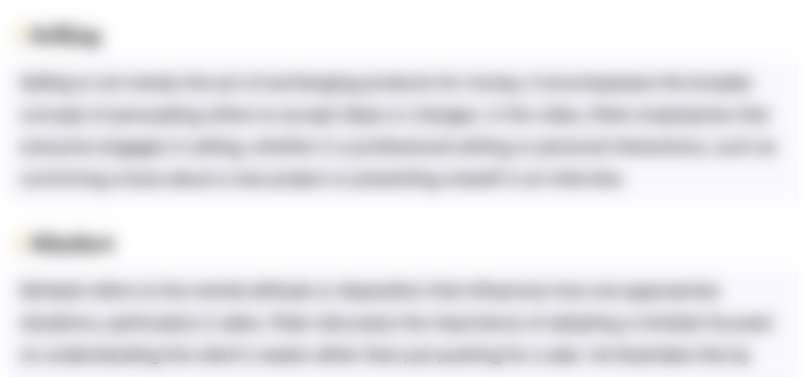
Esta sección está disponible solo para usuarios con suscripción. Por favor, mejora tu plan para acceder a esta parte.
Mejorar ahoraHighlights
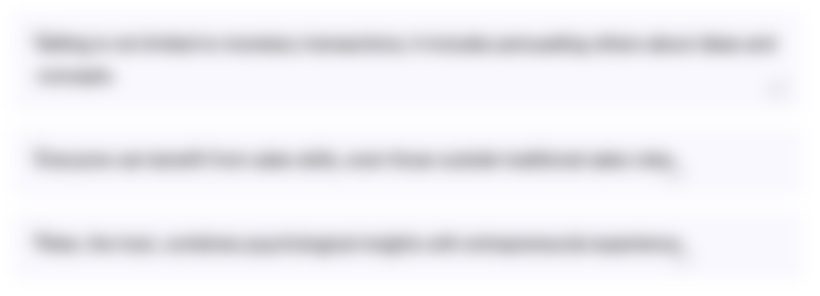
Esta sección está disponible solo para usuarios con suscripción. Por favor, mejora tu plan para acceder a esta parte.
Mejorar ahoraTranscripts
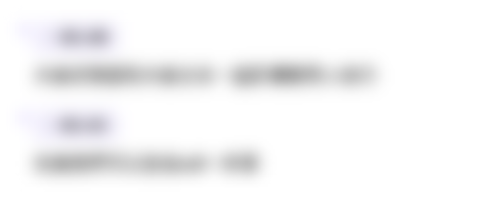
Esta sección está disponible solo para usuarios con suscripción. Por favor, mejora tu plan para acceder a esta parte.
Mejorar ahoraVer Más Videos Relacionados
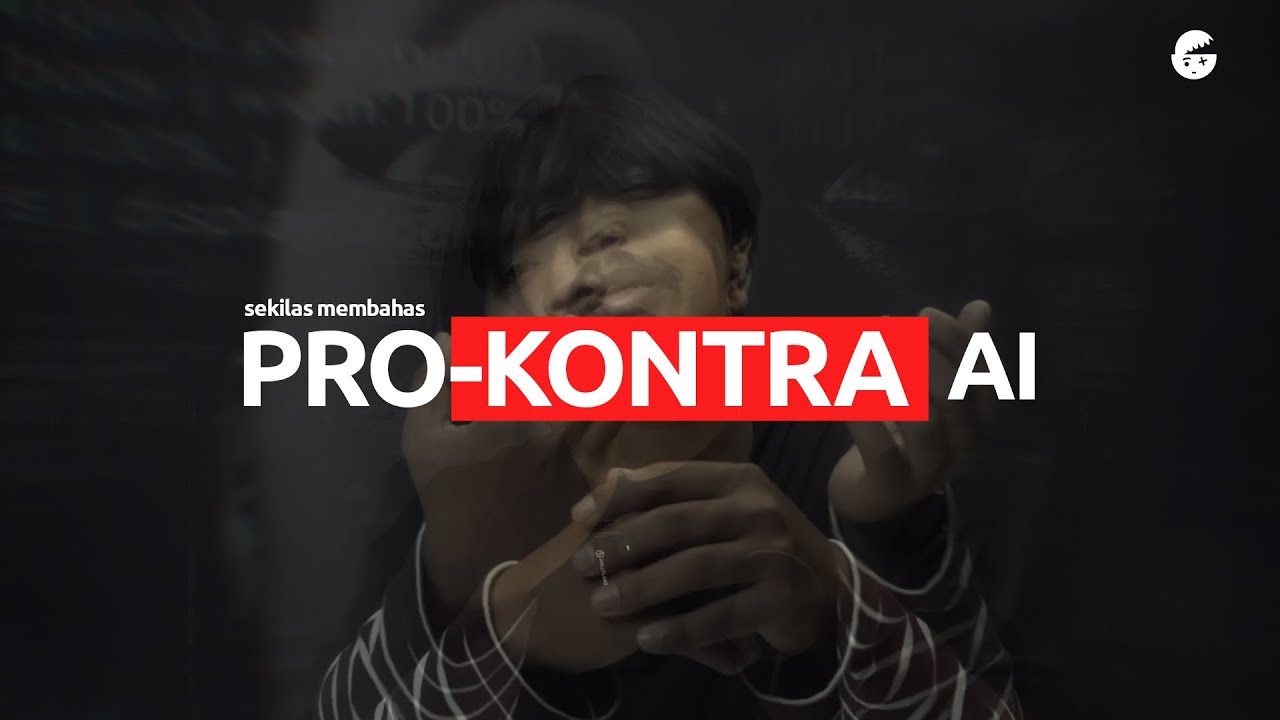
Sekilas Membahas, Pro Kontra AI, #artificialintelligence #ai #education

SMR Group C BUAS 2024
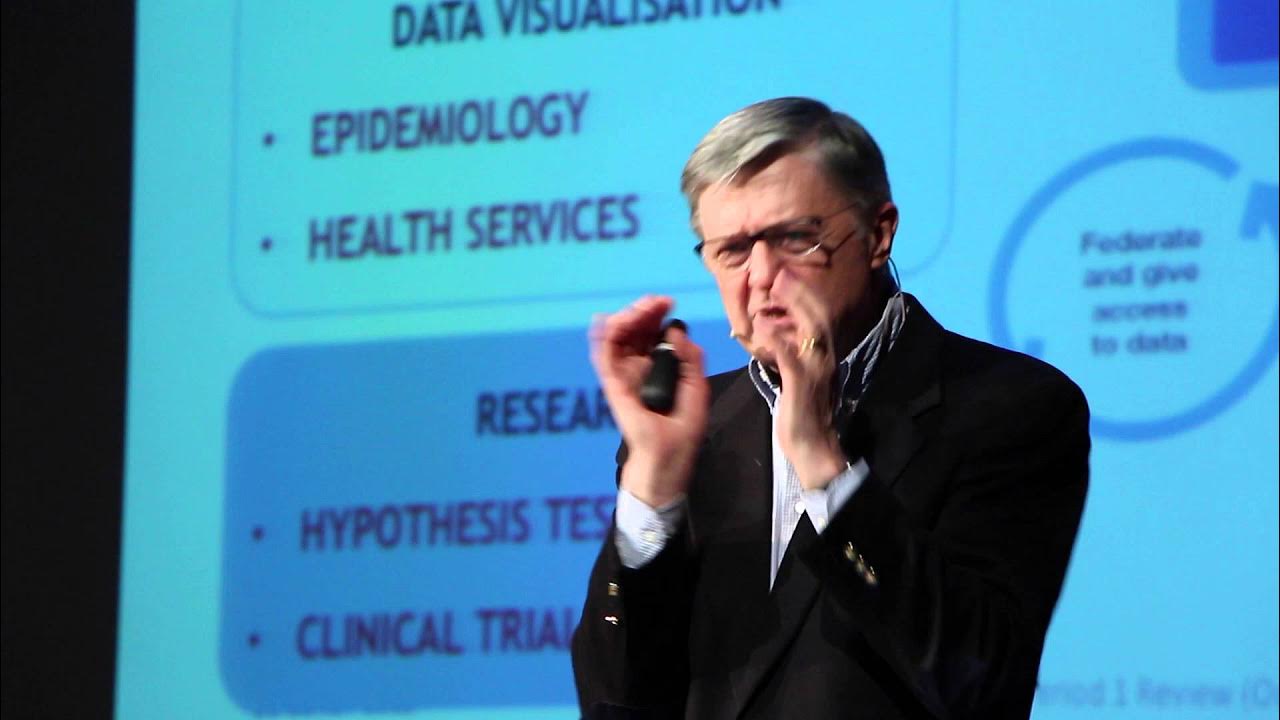
Future Medicine: Modern Informatics | Richard Frackowiack | TEDxYouth@Zurich
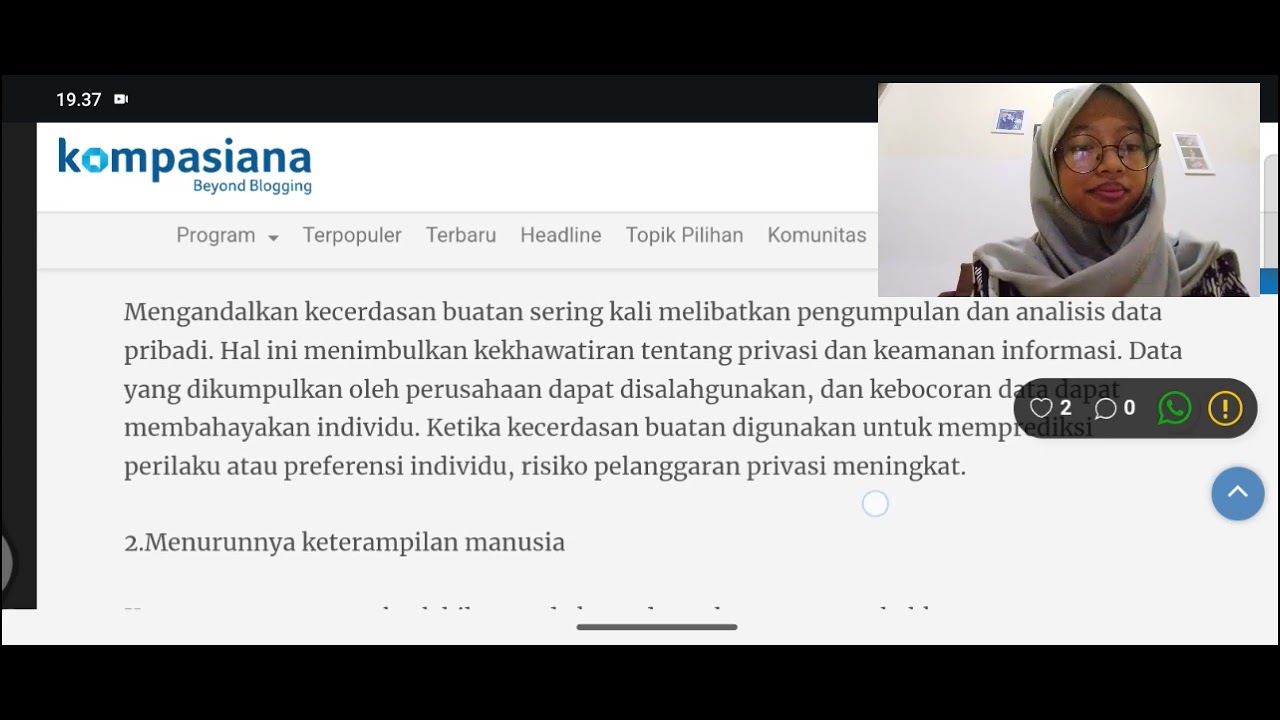
Ketergantungan pada AI: Menelusuri Batas Antara Manfaat dan Risiko

History of AI from 1950 to 2023
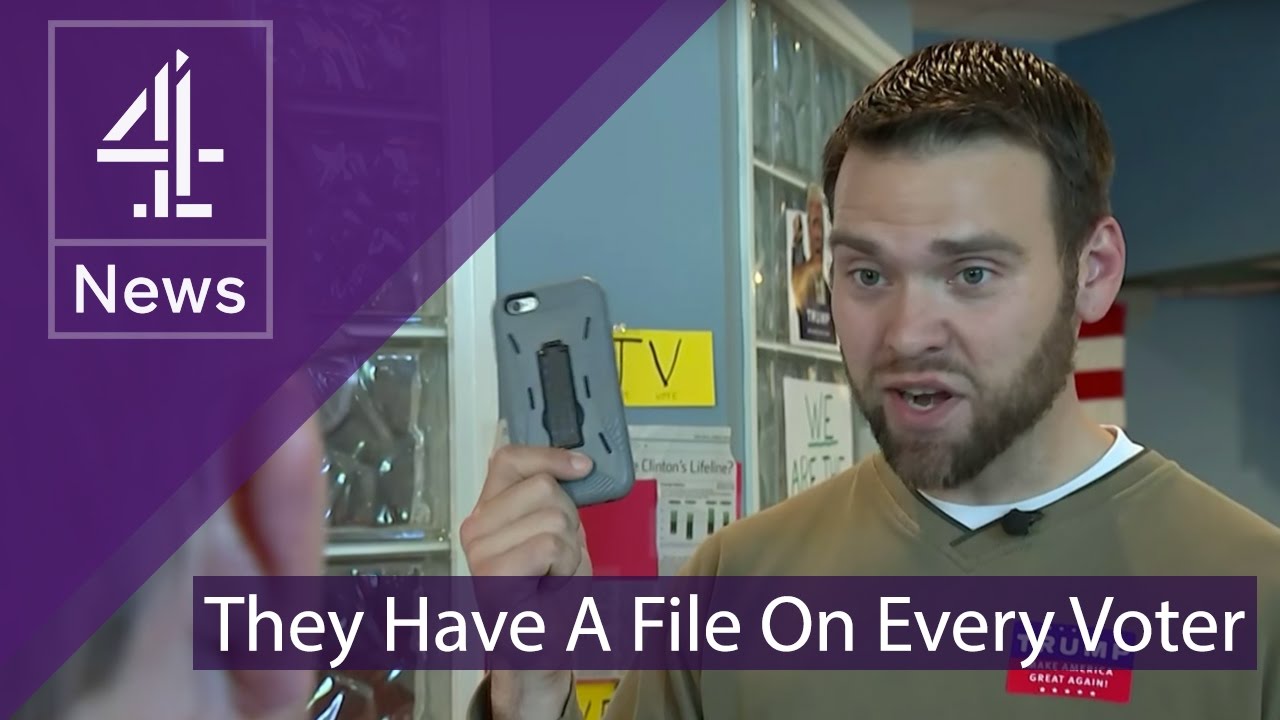
Cambridge Analytica: how big data shaped the US election
5.0 / 5 (0 votes)