How to Build an LLM from Scratch | An Overview
Summary
TLDRThe video provides an overview of key considerations when building a large language model from scratch in 2024, a now more feasible endeavor thanks to advances in AI. It steps through the process, from curating high-quality diverse training data, to designing an efficient Transformer architecture, to leveraging techniques like mixed precision to train at scale, to evaluating model performance on benchmarks. While still resource-intensive, building an LL.M may make sense for certain applications. The video concludes by noting base models are usually then customized via prompt engineering or fine-tuning.
Takeaways
- 😊 Building LLMs is gaining popularity due to increased interest after ChatGPT release
- 📈 Costs to train LLMs range from $100K (10B parameters) to $1.5M (100B parameters)
- 🗃️ High quality and diverse training data is critical for LLM performance
- ⚙️ Transformers with causal decoding are the most popular LLM architecture
- 👩💻 Many design choices exist when constructing LLM architectures
- 🚦 Parallelism, mixed precision, and optimizers boost LLM training efficiency
- 📊 Hyperparameters like batch size, learning rate, and dropout affect stability
- 📈 LLMs should balance model size, compute, and training data to prevent over/underfitting
- ✅ Benchmark datasets help evaluate capabilities on tasks like QA and common sense
- 🔄 Fine-tuning and prompt engineering can adapt pretrained LLMs for downstream uses
Q & A
What are the four main steps involved in building a large language model from scratch?
-The four main steps are: 1) Data curation 2) Model architecture 3) Training the model at scale 4) Evaluating the model.
What type of model architecture is commonly used for large language models?
-Transformers have emerged as the state-of-the-art architecture for large language models.
Why is data curation considered the most important step when building a large language model?
-Data curation is critical because the quality of the model is driven by the quality of the data. Large language models require large, high-quality training data sets.
What are some key considerations when preparing the training data?
-Some key data preparation steps include: quality filtering, deduplication, privacy redaction, and tokenization.
What are some common training techniques used to make it feasible to train large language models?
-Popular training techniques include mixed precision training, 3D parallelism, zero redundancy optimizers, checkpointing, weight decay, and gradient clipping.
How can you evaluate a text generation model on multiple choice benchmark tasks?
-You can create prompt templates with a few shot examples to guide the model to return one of the multiple choice tokens as its response.
What are some pros and cons of prompt engineering versus model fine-tuning?
-Prompt engineering avoids changing the original model but requires more effort to create effective prompts. Fine-tuning adapts the model for a specific use case but risks degrading performance on other tasks.
What are some examples of quality filtering approaches for training data?
-Classifier-based filtering using a text classification model, heuristic-based rules of thumb to filter text, or a combination of both approaches.
What considerations go into determining model size and training time?
-You generally want around 20 tokens per model parameter in the training data. And a 10x increase in model parameters requires around a 100x increase in computational operations.
Why might building a large language model from scratch not be necessary?
-Using an existing model with prompt engineering or fine-tuning is better suited for most use cases. Building from scratch has high costs and only makes sense in certain specialized cases.
Outlines
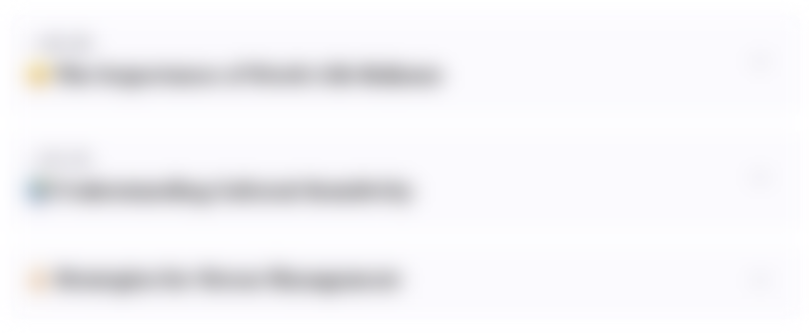
Dieser Bereich ist nur für Premium-Benutzer verfügbar. Bitte führen Sie ein Upgrade durch, um auf diesen Abschnitt zuzugreifen.
Upgrade durchführenMindmap
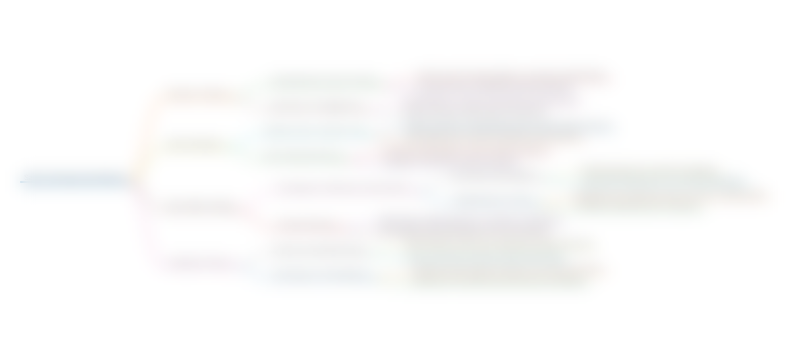
Dieser Bereich ist nur für Premium-Benutzer verfügbar. Bitte führen Sie ein Upgrade durch, um auf diesen Abschnitt zuzugreifen.
Upgrade durchführenKeywords
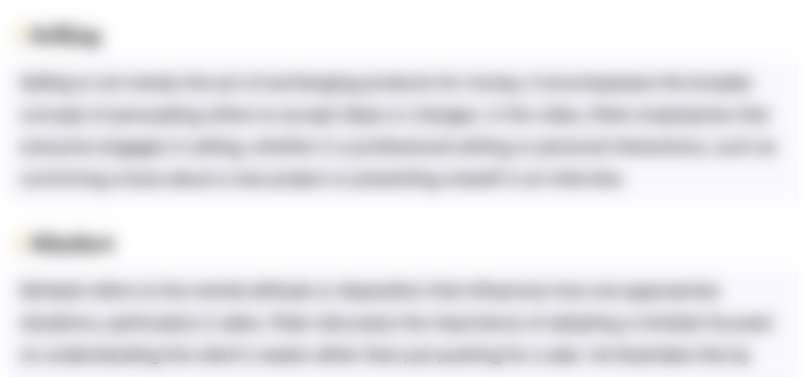
Dieser Bereich ist nur für Premium-Benutzer verfügbar. Bitte führen Sie ein Upgrade durch, um auf diesen Abschnitt zuzugreifen.
Upgrade durchführenHighlights
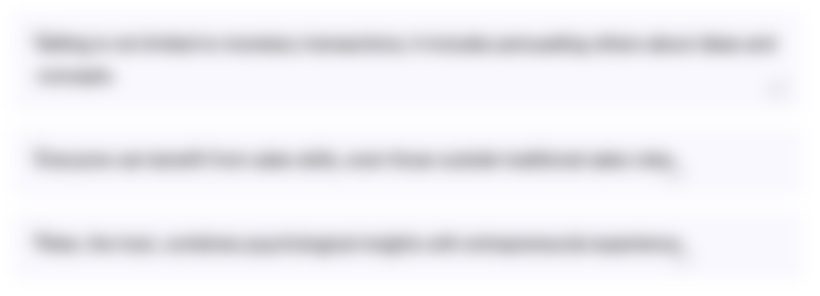
Dieser Bereich ist nur für Premium-Benutzer verfügbar. Bitte führen Sie ein Upgrade durch, um auf diesen Abschnitt zuzugreifen.
Upgrade durchführenTranscripts
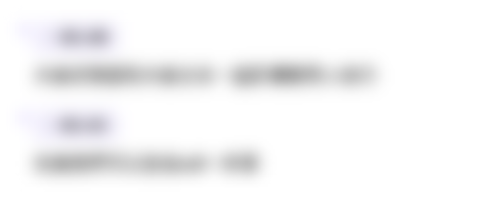
Dieser Bereich ist nur für Premium-Benutzer verfügbar. Bitte führen Sie ein Upgrade durch, um auf diesen Abschnitt zuzugreifen.
Upgrade durchführenWeitere ähnliche Videos ansehen
5.0 / 5 (0 votes)