24 hr AI conference: The AI in Audit Revolution (US)
Summary
TLDRIn this insightful discussion, Will Bible, the deputy leader of Audit, Transformation, Fraud, and Assurance Business, explores the impact of AI on the audit industry since 2015. With Brian Crowley and Ryan, they delve into the transformative power of AI, particularly large language models, in enhancing audit services, standardizing workflows, and managing the exponential growth of data. They address the challenges of deploying AI, including risk mitigation strategies, the importance of data quality, and the future skills required in a technology-driven audit profession.
Takeaways
- 😀 Digital transformation has been a key focus for the Audit, Transformation, Fraud, and Assurance Business since 2015, aiming to improve quality through standardized workflows and digitization.
- 🌐 The global deployment of the fully digital platform OMNIA is a significant milestone in the company's digital journey, enabling advanced data utilization and AI integration.
- 🔍 Data is identified as a crucial enabler for AI, and the standardization and structuring of data have been foundational to the deployment of AI capabilities in auditing.
- 📈 The rapid growth of data available to auditors presents challenges that AI is positioned to address, particularly in handling large volumes of transactions and identifying outliers.
- 📝 The script highlights the importance of written language in auditing, with AI capabilities like natural language processing being used to analyze and summarize vast amounts of textual data.
- 🤖 Generative AI is transforming the audit process by acting as a translator between human and machine, enhancing productivity, and democratizing enterprise knowledge.
- 🛠️ AI deployment in auditing involves a multidisciplinary approach, requiring collaboration across data science, IT, risk, legal, and quality to ensure effective and ethical use.
- 🔑 The importance of having a clear mission and value statement for AI is emphasized, to guide its design, development, and use, and to mitigate risks such as reputational damage and regulatory non-compliance.
- 🚀 The potential of generative AI in auditing is vast but still in its early stages, with opportunities for complex information extraction and enhancing human-machine interaction.
- 🛑 The need for a robust framework to evaluate AI performance, including metrics for accuracy, impact, and bias, is discussed, as is the importance of continuous monitoring and validation.
- 🔑 The script suggests that AI will not replace jobs but will change them, requiring a workforce that understands and can effectively utilize AI, emphasizing the need for ongoing upskilling and adaptability.
Q & A
What digital transformation journey has Will Bible's organization been on since 2015?
-Will Bible's organization has been on a digital transformation journey that involves rapidly innovating and deploying digital technologies to address specific pain points in the audit process. The focus has been on standardizing workflows and digitizing with the aim of improving overall quality.
What is the name of the globally deployed, fully digital platform mentioned in the script?
-The globally deployed, fully digital platform mentioned is called OMNIA.
What role does data play in the deployment of AI within Will Bible's organization?
-Data is a key enabler for deploying AI within the organization. The digital platform Omnia is critical for managing data and supporting AI capabilities.
What is Brian Crowley's perspective on the impact of AI on the audit business?
-Brian Crowley believes that the audit business, like others, is being significantly impacted by AI as clients digitize their operations, leading to an exponential growth in available data. This data growth presents a challenge that cannot be managed by human means alone, necessitating the use of AI.
What are some of the AI capabilities that Brian Crowley's group focuses on within audit?
-Brian Crowley's group focuses on AI capabilities such as anomaly detection using unsupervised learning to identify outliers in transactions, and natural language processing to handle written language in transaction descriptions, account names, and other forms of transactional evidence.
How does Ryan view the deployment of AI in the finance and reporting field?
-Ryan observes that AI is being deployed in low-risk areas initially, but its use is quickly expanding into other parts of the organization. He mentions specific AI applications in financial reporting, such as internal control for financial reporting, transaction monitoring, and anomaly detection.
What are some of the risks associated with deploying AI in critical business processes?
-Some of the risks include reputational risk, operational and financial risks, and regulatory compliance risks. There is also the challenge of disparate impact on protected groups and the evolving nature of AI regulation.
What is the trustworthy AI framework that Will Bible refers to?
-The trustworthy AI framework is a set of guidelines and principles that aim to ensure that AI is developed and used in a way that is ethical, transparent, and minimizes risk. It includes having a clear mission statement, value statement, and governance frameworks for AI deployment.
How does Brian Crowley see generative AI impacting the audit process?
-Brian Crowley sees generative AI as a tool that can significantly enhance productivity by acting as a translator between human and machine, making enterprise knowledge more accessible, and facilitating complex information extraction from documents.
What advice does Brian Crowley give for individuals looking to upskill in the area of generative AI?
-Brian Crowley advises individuals to learn about prompting and prompt engineering, which involves effectively interacting with AI models to provide instructions and achieve desired outcomes.
What are some lessons learned from deploying AI models in an enterprise context, according to Brian and Ryan?
-Some lessons learned include the importance of having high-quality data, the necessity of getting all stakeholders on board, the complexity of infrastructure, and the need for a clear mission statement. Additionally, it's crucial to consider where AI fits within the workflow and to be prepared for the uncertainty of AI outputs.
Outlines
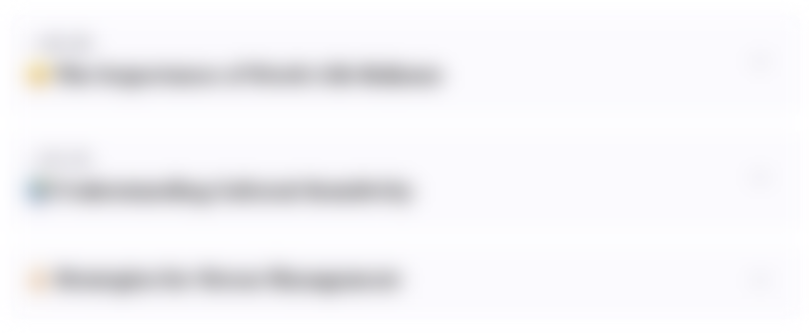
Dieser Bereich ist nur für Premium-Benutzer verfügbar. Bitte führen Sie ein Upgrade durch, um auf diesen Abschnitt zuzugreifen.
Upgrade durchführenMindmap
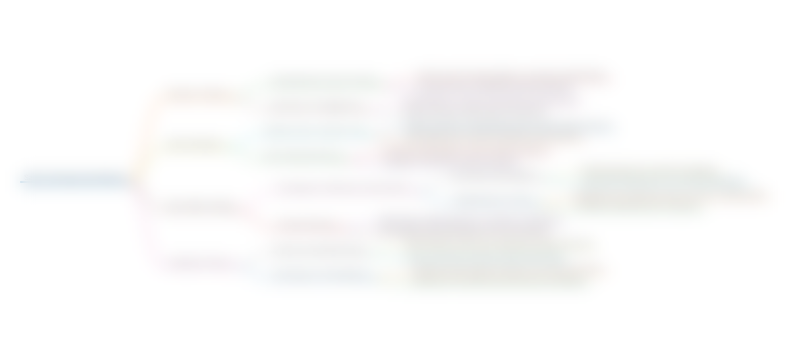
Dieser Bereich ist nur für Premium-Benutzer verfügbar. Bitte führen Sie ein Upgrade durch, um auf diesen Abschnitt zuzugreifen.
Upgrade durchführenKeywords
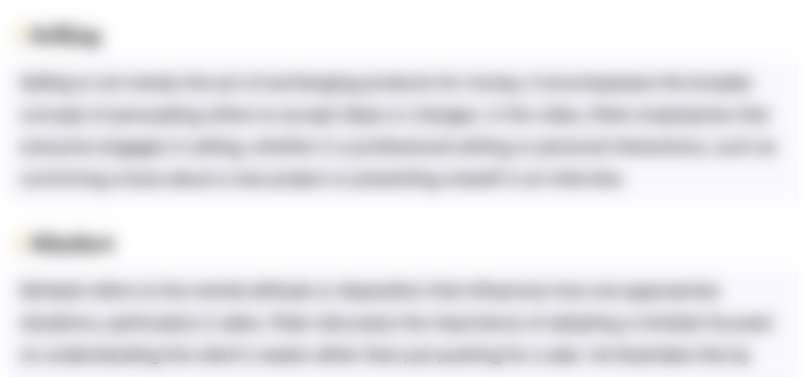
Dieser Bereich ist nur für Premium-Benutzer verfügbar. Bitte führen Sie ein Upgrade durch, um auf diesen Abschnitt zuzugreifen.
Upgrade durchführenHighlights
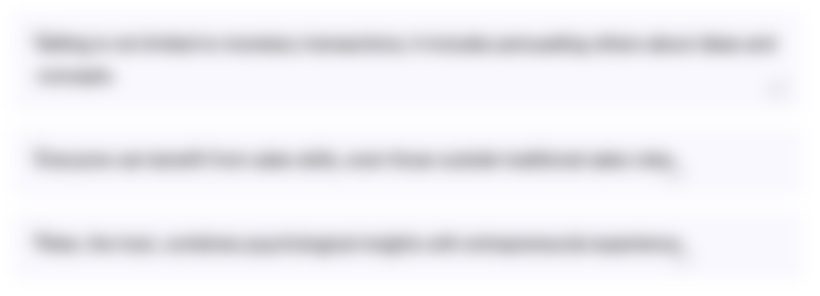
Dieser Bereich ist nur für Premium-Benutzer verfügbar. Bitte führen Sie ein Upgrade durch, um auf diesen Abschnitt zuzugreifen.
Upgrade durchführenTranscripts
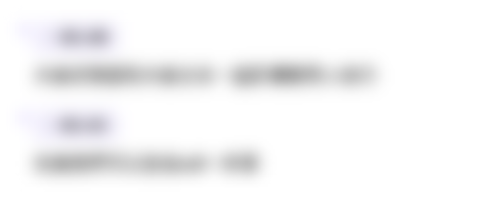
Dieser Bereich ist nur für Premium-Benutzer verfügbar. Bitte führen Sie ein Upgrade durch, um auf diesen Abschnitt zuzugreifen.
Upgrade durchführenWeitere ähnliche Videos ansehen

All Things Internal Audit AI Podcast: Generative AI Uses for Internal Audit

Powering the future of Audit through AI, Data, and Technology
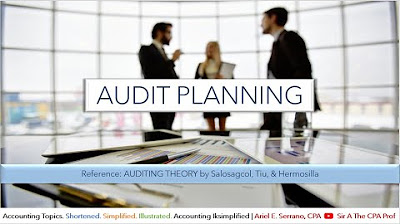
Audit Planning | Understanding the Entity and its Environment | Hermosilla, Tiu, Salosagcol
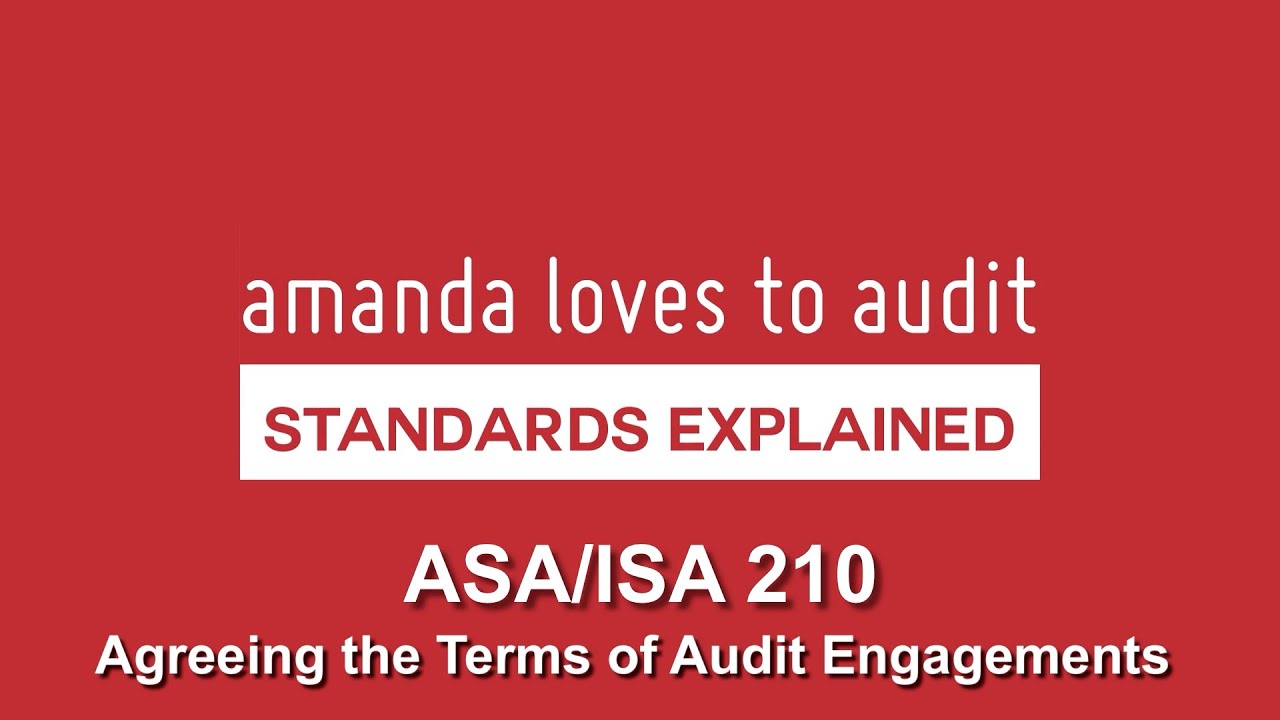
The CONTRACT between the Auditor & the Client | ISA/ASA 210
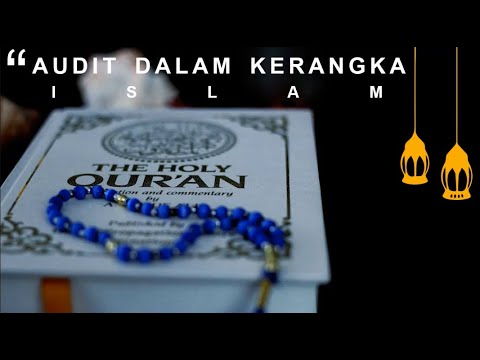
Audit dalam Kerangka Islam - Audit Lembaga Keuangan Syariah
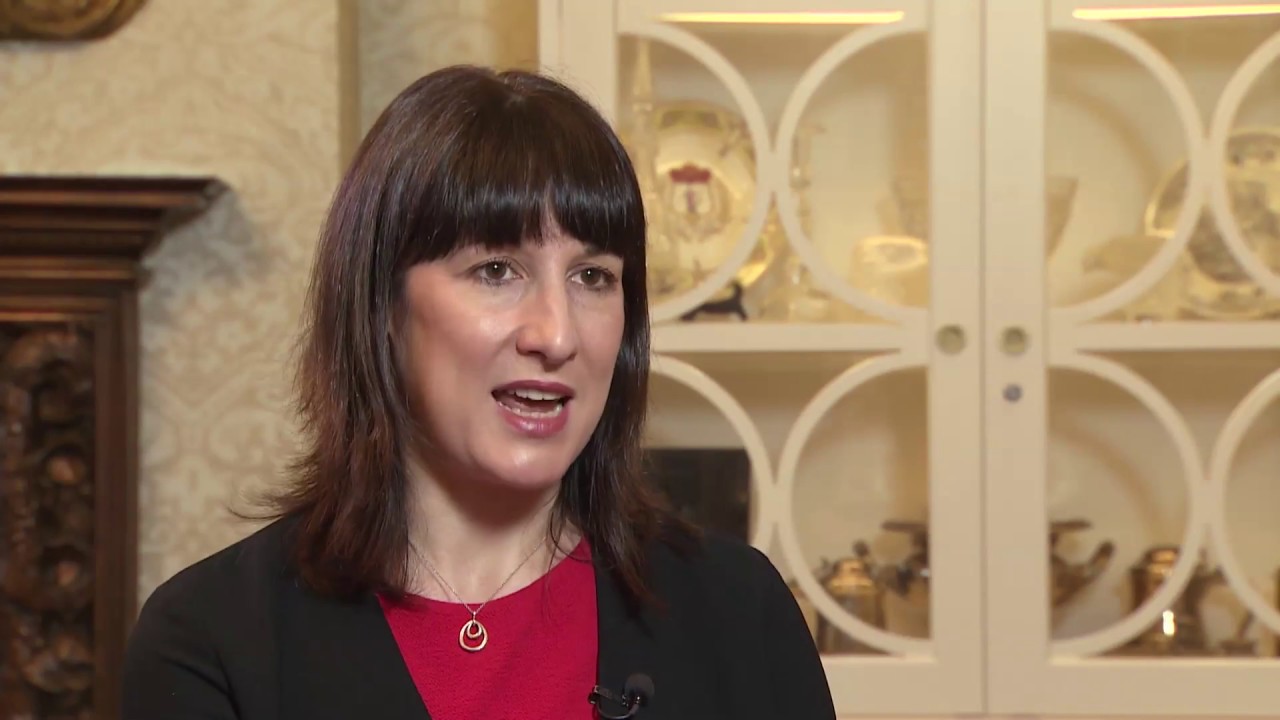
Rachel Reeves on fixing “broken” audit market
5.0 / 5 (0 votes)