Bias in AI is a Problem
Summary
TLDRThe script discusses the issue of bias in AI systems, particularly in the context of hiring. It explains that if a company's historical data, which may contain biases like gender disparity in hiring or pay, is used to train a machine learning model, these biases will be perpetuated in the model's decisions. The script emphasizes the importance for companies to critically examine and address data bias to prevent reinforcing harmful patterns in AI and machine learning applications.
Takeaways
- 🧠 Bias in AI Systems: AI systems can develop biases that can lead to problematic outcomes.
- 📈 Historical Data Usage: A large company's past ten years of hiring data could be used to train a machine learning system for candidate selection.
- 🚫 Bias Continuation: If the original data contains biases, such as gender disparity in hiring or pay, these biases will be perpetuated by the machine learning model.
- 🔄 Reinforcement of Bias: Hiring decisions influenced by a biased machine learning algorithm can reinforce existing biases in the workplace.
- 🔍 Problem Identification: The script identifies the issue of bias in AI and machine learning as a serious problem that companies need to address.
- 📝 Data Scrutiny: Companies must carefully examine their data for biases to avoid training AI systems with skewed perspectives.
- 🤖 Machine Incapability: Machines cannot distinguish between genuine patterns and biases within data, necessitating human oversight in data analysis.
- 🧐 Importance of Pattern Recognition: The script highlights the difficulty in discerning between underlying patterns and biases in data, which is crucial for AI fairness.
- 🛠️ Addressing Bias: The need for companies to actively work on mitigating biases in their AI systems is emphasized.
- 🔑 Human Oversight: Human involvement is key in identifying and correcting biases in AI systems to ensure fairness and equity in decision-making.
Q & A
What is the main concern raised in the script about AI systems?
-The script raises the concern that AI systems can develop biases, which can be problematic and lead to unfair treatment in various applications such as hiring processes.
Why could using historical data to train a machine learning system be problematic?
-Using historical data to train a machine learning system can be problematic because if the original data contains biases, such as hiring more men than women or paying men higher salaries for the same jobs, these biases will be carried over to the machine learning model, affecting its future decisions.
What is the potential consequence of a biased machine learning model in hiring?
-The potential consequence is that the bias gets reinforced in the hiring process, leading to a perpetuation of unfair practices and discrimination.
What does the script suggest companies need to do to address bias in AI and machine learning?
-The script suggests that companies need to take a serious look at the bias in their data and address it to ensure fairness in AI and machine learning applications.
Why is it difficult for a machine to differentiate between an underlying pattern and a bias in the data?
-It is difficult because a machine lacks the contextual understanding and ethical judgment that humans possess, and it can only learn from the patterns presented in the data it is trained on.
How can biases in AI systems affect the fairness of future decisions?
-Biases in AI systems can affect the fairness of future decisions by favoring certain groups over others based on historical biases, rather than making decisions based on merit or fairness.
What is the importance of recognizing and addressing biases in AI systems?
-Recognizing and addressing biases in AI systems is crucial to ensure that the technology is used ethically and does not perpetuate or exacerbate existing inequalities.
Can you provide an example of how bias might manifest in a machine learning model trained on historical hiring data?
-An example could be a model that learns from data showing a higher proportion of men being hired for certain positions, leading it to prefer male candidates in the future, even when equally or more qualified female candidates apply.
What steps can be taken to mitigate biases when training machine learning models?
-Steps to mitigate biases include carefully selecting and auditing the training data, using diverse datasets, implementing fairness metrics, and continually monitoring and adjusting the model to ensure it does not perpetuate bias.
How can companies ensure that their AI systems are making unbiased decisions?
-Companies can ensure unbiased decisions by implementing bias detection and mitigation strategies, involving diverse teams in the development process, and regularly testing and refining their AI systems for fairness.
What role does transparency play in addressing biases in AI systems?
-Transparency is key in addressing biases as it allows for the examination of how decisions are made by AI systems, enabling the identification and correction of any biases present in the algorithms.
Outlines
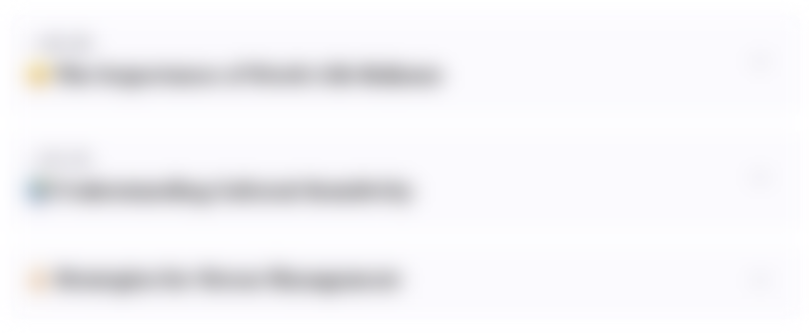
Dieser Bereich ist nur für Premium-Benutzer verfügbar. Bitte führen Sie ein Upgrade durch, um auf diesen Abschnitt zuzugreifen.
Upgrade durchführenMindmap
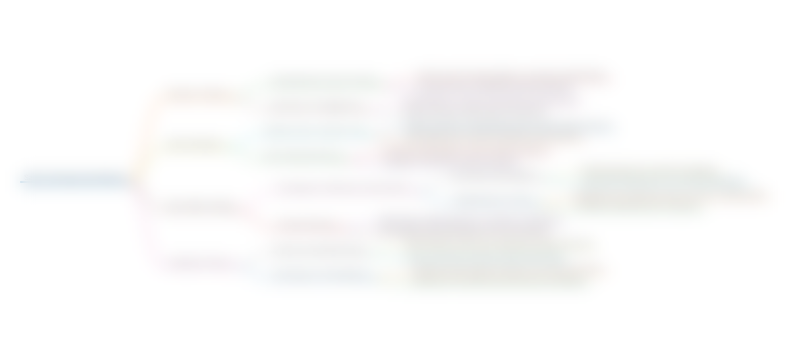
Dieser Bereich ist nur für Premium-Benutzer verfügbar. Bitte führen Sie ein Upgrade durch, um auf diesen Abschnitt zuzugreifen.
Upgrade durchführenKeywords
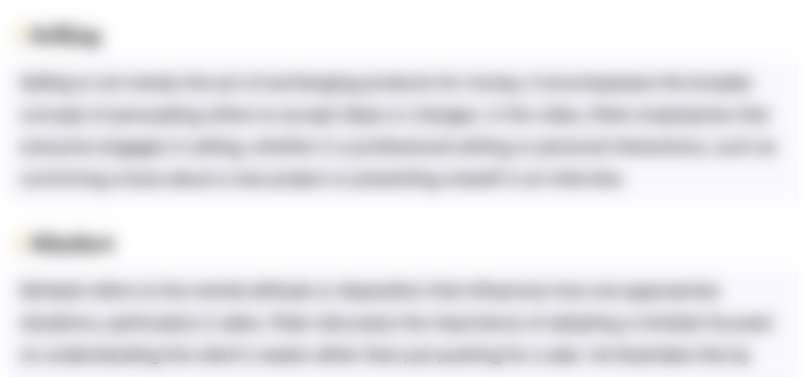
Dieser Bereich ist nur für Premium-Benutzer verfügbar. Bitte führen Sie ein Upgrade durch, um auf diesen Abschnitt zuzugreifen.
Upgrade durchführenHighlights
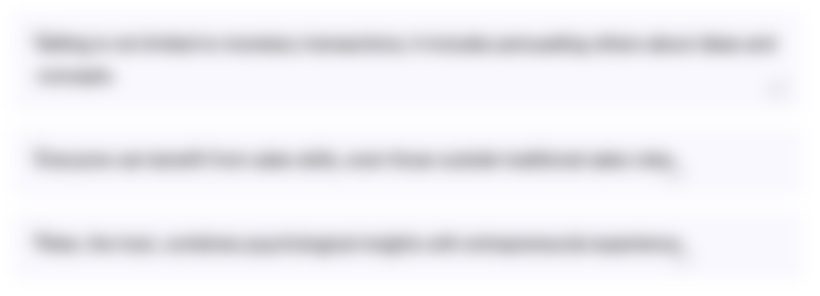
Dieser Bereich ist nur für Premium-Benutzer verfügbar. Bitte führen Sie ein Upgrade durch, um auf diesen Abschnitt zuzugreifen.
Upgrade durchführenTranscripts
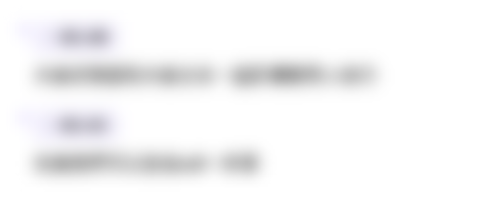
Dieser Bereich ist nur für Premium-Benutzer verfügbar. Bitte führen Sie ein Upgrade durch, um auf diesen Abschnitt zuzugreifen.
Upgrade durchführen5.0 / 5 (0 votes)