Model Evaluation using Visualization #datascience #datascience #technology #subscribeformore
Summary
TLDRThis video script delves into model evaluation through visualization techniques. It emphasizes the utility of regression plots for estimating variable relationships, highlighting correlation strength and direction. The script guides on plotting these using Seaborn's 'regplot' and 'residplot' functions, illustrating how residual plots can reveal linearity or non-linearity in data. It also touches on distribution plots for visualizing models with multiple variables, showcasing how predicted and actual values compare, and how using multiple features can enhance model accuracy.
Takeaways
- 📈 Regression plots are essential for visualizing the relationship between two variables, indicating the correlation strength and direction.
- 📊 The horizontal axis in a regression plot represents the independent variable, while the vertical axis represents the dependent variable.
- 📝 Each point in a regression plot corresponds to a different target, with the fitted line showing the predicted values.
- 🖋️ Seaborn's 'regplot' function is a simple method to create regression plots, requiring the column names for independent and dependent variables.
- 🔍 Residual plots help examine the error between predicted and actual values, providing insights into the model's fit.
- 📉 A residual plot with zero mean and evenly distributed values around the x-axis suggests a well-fitted linear model.
- 🌀 Curvature in a residual plot indicates that a non-linear function might be more appropriate for the data.
- 📊 Seaborn's 'residplot' function is used to create residual plots, showing the relationship between predicted and actual values.
- 📈 Distribution plots are useful for visualizing models with multiple independent variables, comparing predicted and actual values.
- 📊 In a distribution plot, the vertical axis is scaled to normalize the area under the distribution, useful for continuous values.
- 💡 The script illustrates the use of different visualization techniques to evaluate and understand the performance of regression models.
Q & A
What is the purpose of using regression plots in model evaluation?
-Regression plots are used to estimate the relationship between two variables, determine the strength of the correlation, and identify the direction of the relationship (positive or negative).
What do the horizontal and vertical axes represent in a regression plot?
-The horizontal axis represents the independent variable, while the vertical axis represents the dependent variable.
How is a regression plot created using the Seaborn library in Python?
-First, import the Seaborn library. Then use the 'regplot' function, specifying the 'x' parameter for the independent variable, the 'y' parameter for the dependent variable, and the 'data' parameter for the dataframe containing the data.
What does a residual plot represent?
-A residual plot represents the error between the actual value and the predicted value. It is plotted with the independent variable on the horizontal axis and the residuals (errors) on the vertical axis.
What does it indicate if the residuals are distributed evenly around the x-axis with similar variance?
-If the residuals are evenly distributed around the x-axis with similar variance and zero mean, it suggests that a linear model is appropriate for the data.
What might it indicate if there is curvature in the residual plot?
-Curvature in the residual plot indicates that the linear assumption may be incorrect, and a non-linear model might be more appropriate.
How can Seaborn be used to create a residual plot?
-First, import the Seaborn library. Then use the 'residplot' function, specifying the independent variable series as the first parameter and the dependent variable series as the second parameter.
What is the purpose of a distribution plot in model evaluation?
-A distribution plot is used to compare the predicted values to the actual values, helping visualize the accuracy of the model's predictions across a range of values.
How are continuous target values handled when creating a distribution plot using Pandas?
-Since a histogram is for discrete values, Pandas converts continuous target values to a distribution, scaling the vertical axis to make the area under the distribution equal to one.
What does a distribution plot with the predicted and actual values reveal about the model's performance?
-The distribution plot shows how close the predicted values are to the actual values. For example, in the provided script, predicted values for prices ranging from $10,000 to $20,000 are closer to the actual target values compared to the predicted values for prices ranging from $40,000 to $50,000.
Outlines
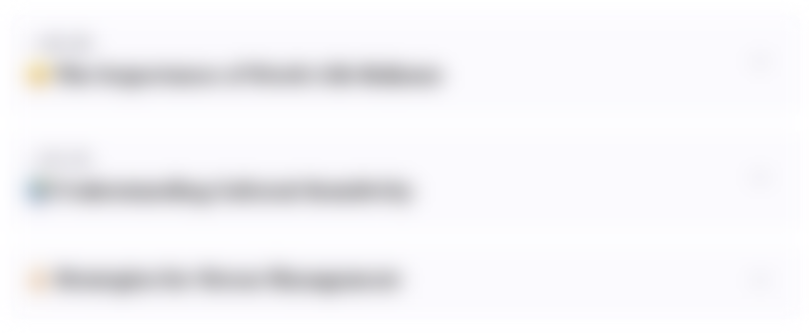
Dieser Bereich ist nur für Premium-Benutzer verfügbar. Bitte führen Sie ein Upgrade durch, um auf diesen Abschnitt zuzugreifen.
Upgrade durchführenMindmap
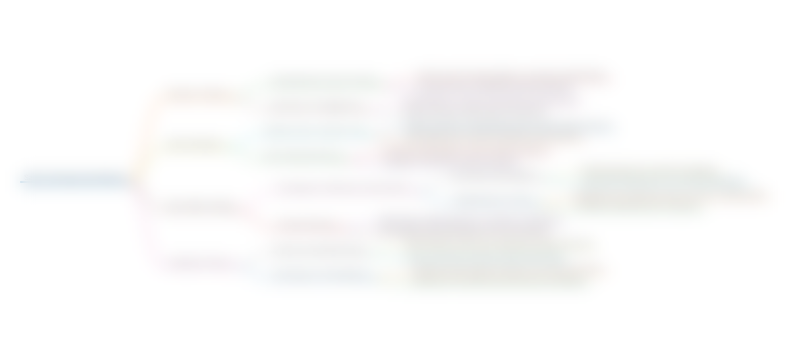
Dieser Bereich ist nur für Premium-Benutzer verfügbar. Bitte führen Sie ein Upgrade durch, um auf diesen Abschnitt zuzugreifen.
Upgrade durchführenKeywords
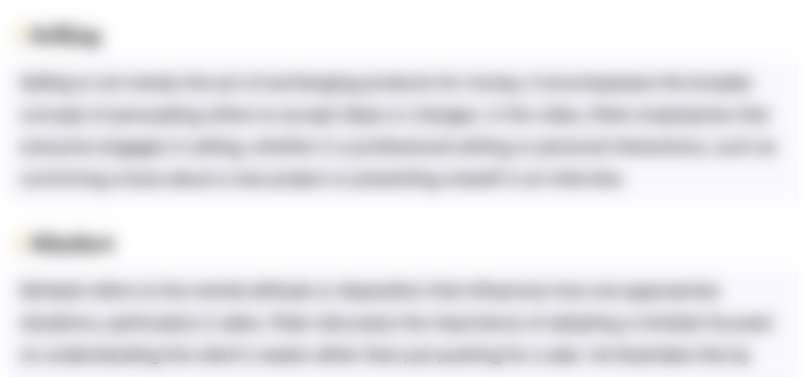
Dieser Bereich ist nur für Premium-Benutzer verfügbar. Bitte führen Sie ein Upgrade durch, um auf diesen Abschnitt zuzugreifen.
Upgrade durchführenHighlights
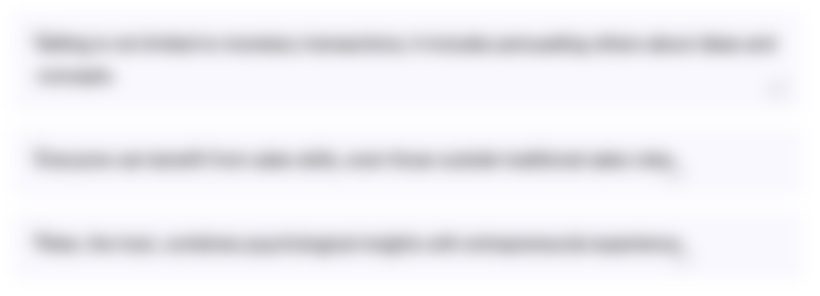
Dieser Bereich ist nur für Premium-Benutzer verfügbar. Bitte führen Sie ein Upgrade durch, um auf diesen Abschnitt zuzugreifen.
Upgrade durchführenTranscripts
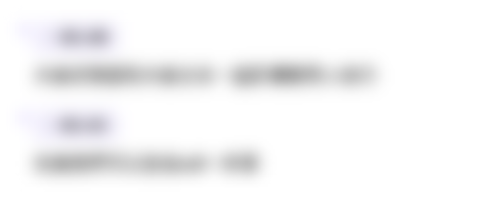
Dieser Bereich ist nur für Premium-Benutzer verfügbar. Bitte führen Sie ein Upgrade durch, um auf diesen Abschnitt zuzugreifen.
Upgrade durchführenWeitere ähnliche Videos ansehen

Prediksi Penyakit Serangan Jantung | Machine Learning Project 11
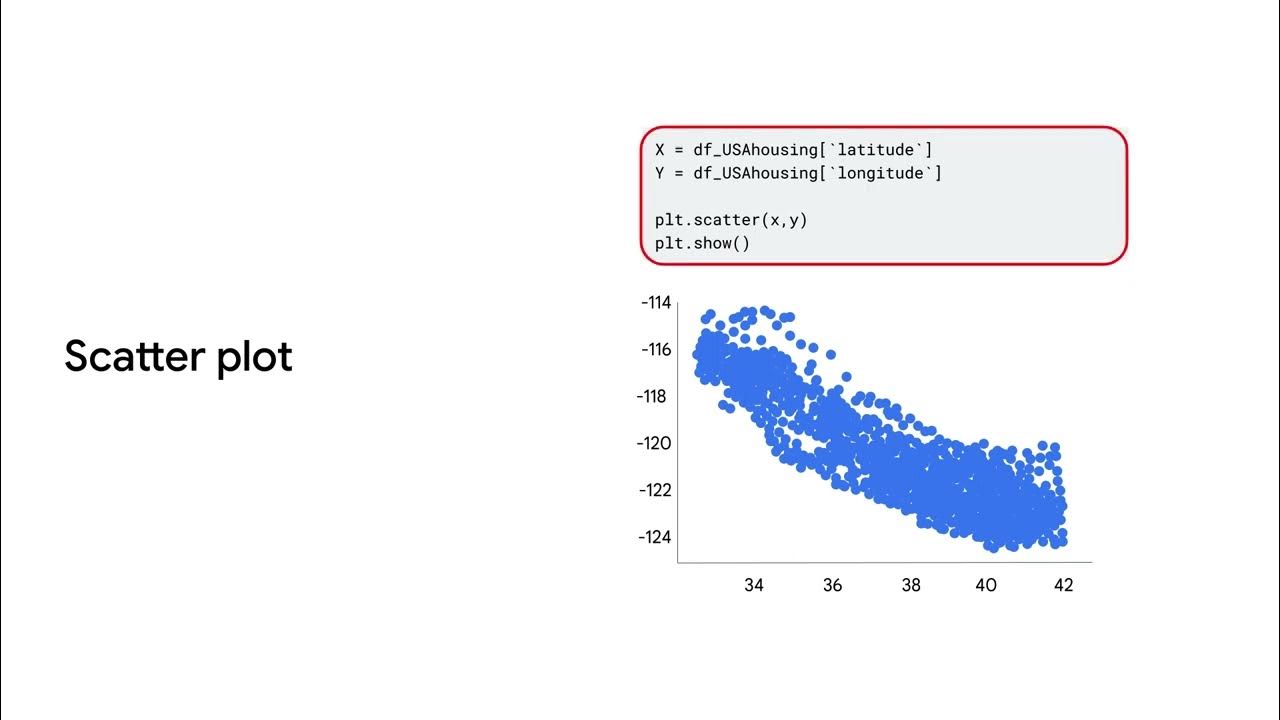
Data analysis and visualization
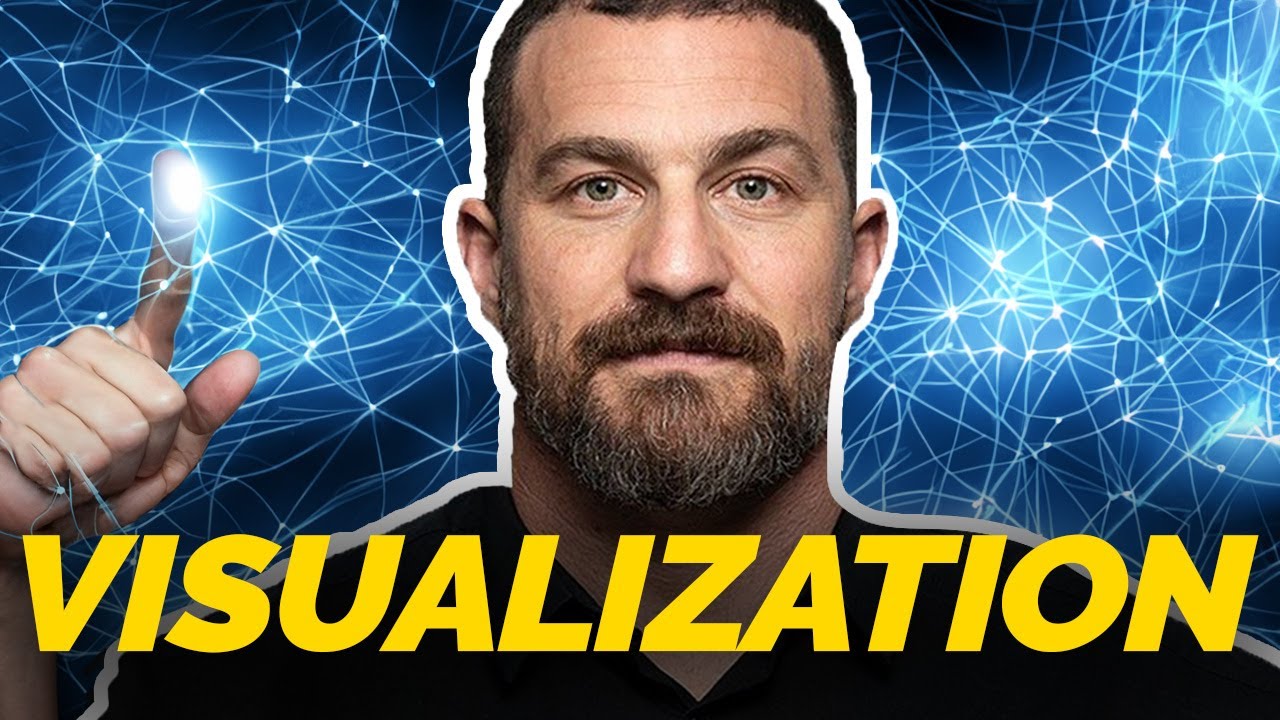
The Neurobiology of Visualization & HOW TO DO IT RIGHT | Andrew Huberman
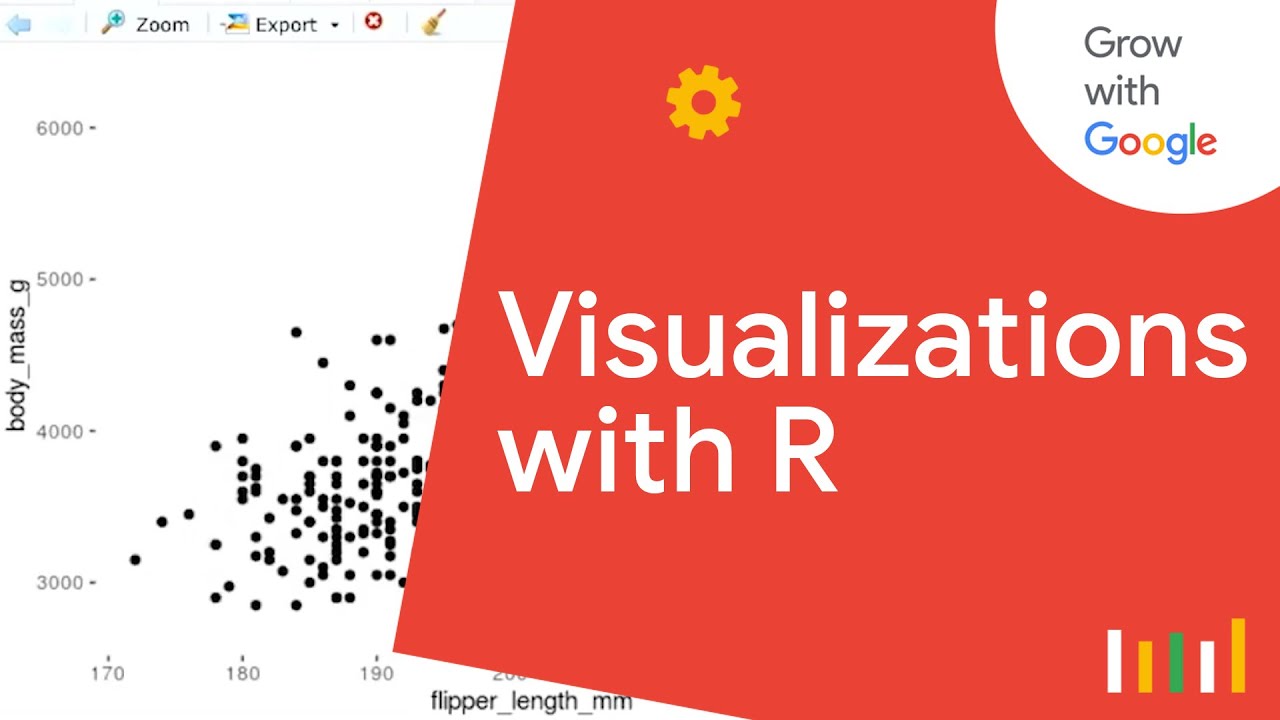
Intro to Data Visualization with R & ggplot2 | Google Data Analytics Certificate
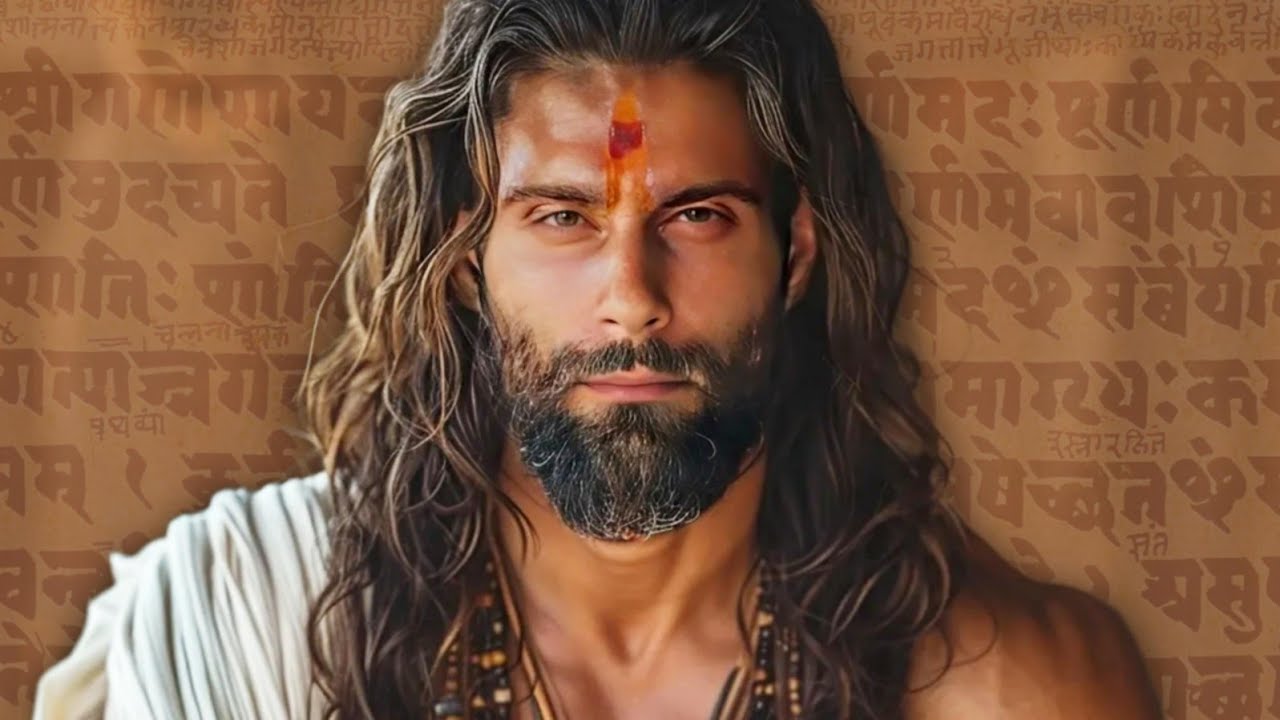
This MEDITATION Technique Is More Powerful Than All Books Combined
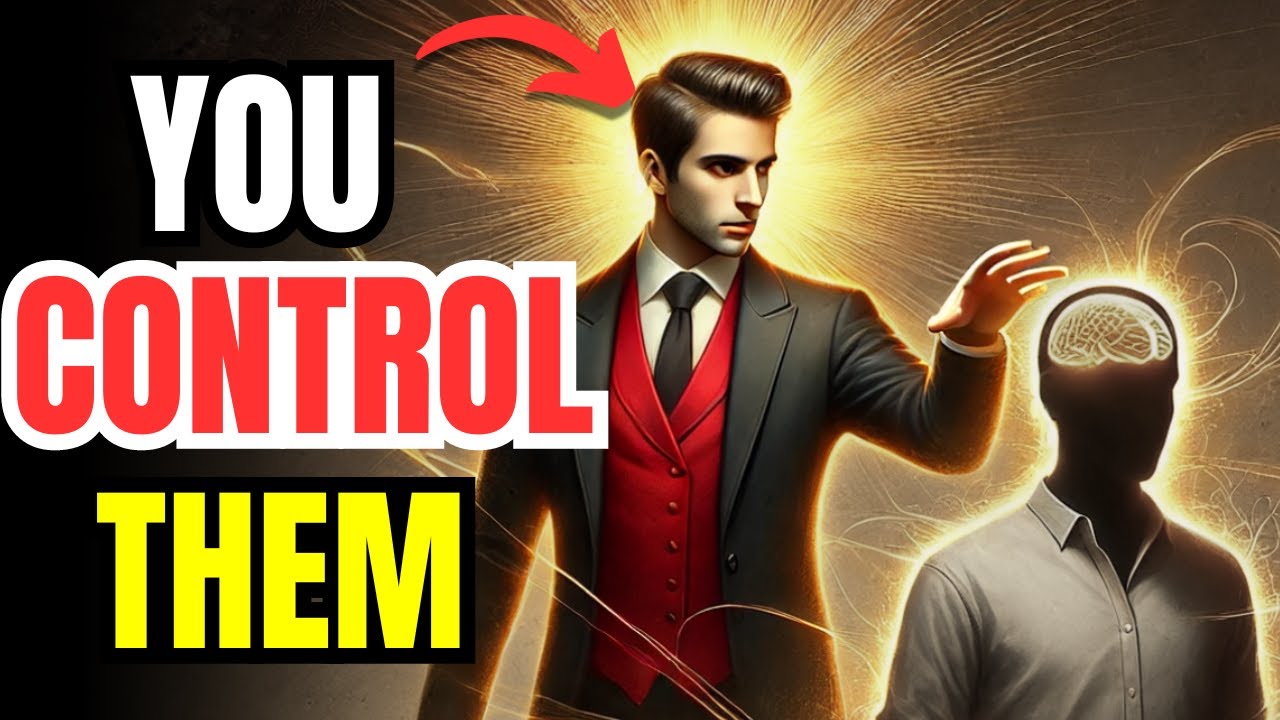
CHOSEN ONES, YOUR THOUGHTS CAN CONTROL OTHERS😎! | THIS IS HOW....
5.0 / 5 (0 votes)