🚀 VivaTech 2024 : Keynote - More than a Model: The Gen AI Essentials for Business Innovation
Summary
TLDRこのビデオスクリプトでは、生成的AI(generative AI)の未来とその応用について語られています。スピーカーはAmazonでのインターンシップを通じてクラウドコンピューティングの基礎を築き、その後の20年間で技術業界で最も革新的な技術に関与してきたと語ります。生成的AIは医療、マーケティング、ゲームなど様々な業界で革新をもたらし、カスタマイズされた言語モデルやタスク自動化、セキュリティとガバナンスを備えたエンタープライズアプリケーションに組み込まれています。Amazon BedrockとAmazon Qは開発者とビジネスユーザーが生成的AIを活用し、業務の効率化と新しいアプリケーションの開発を促進するツールとして紹介されています。
Takeaways
- 🧑💼 スピーカーは、ジェネラティブAIを活用して新しい製品やサービスを創造することに情熱を燃やしているビルダーであり、夢を追う人々と共通しています。
- 🌐 クラウドコンピューティングの初期段階から、スピーカーはテクノロジー業界で最も革新的な技術に関与してきました。
- 📈 ジェネラティブAIの時代には、ビッグデータ、機械学習、そして大規模な言語モデルがプログラム可能となり、ビジネスのイノベーションを促進しています。
- 🛍️ マーケティング業界では、ジェネラティブAIが自動化された広告キャンペーンやハイパーパーソナライズドされた顧客体験を可能にしています。
- 🏥 医療業界では、ジェネラティブAIは医師を支援し、患者との会話を聞き取り、診療ノートを要約、画像診断方法を提案して診断の正確性を高めています。
- 🎮 ゲーム業界では、個々のプレイヤーの行動に基づいてキャラクター、ミッション、インタラクションの無限のバリエーションを持つ個々の体験を作成できます。
- 🔧 ジェネラティブAIは顧客体験の向上や従業員の生産性の向上を通じて、会話検索、テキスト要約、コード生成など、多くの業界で共通のテーマとユースケースを形成しています。
- 🛠️ モデルのカスタマイズ、タスクの自動化、セキュリティとガバナンスの強化が、ジェネラティブAIアプリケーションを構築する上で重要な要素です。
- 📚 データはビジネスや顧客を理解するための重要な差別化要因であり、RAG(Retrieve Augmented Generation)やファインチューニング、継続的プリトレーニングを通じてモデルにデータを活用できます。
- 🤖 開発者がAIモデルを利用し、タスクを自動化し、組織内の仕事を行うには、多くの手動プログラミングが必要なため、時間と専門知識を要するプロセスです。
- 🛡️ セキュリティとガバナンスは、ジェネラティブAIアプリケーションの展開において重要な課題であり、データプライバシーとエンタープライズグレードのAIの制御が必要です。
Q & A
ジェネラティブAIがどのような時代を迎えていると話されていますか?
-ジェネラティブAIは、現在、すべての業種がその力を借りて自己再発明を行っている時代を迎えています。AIは経営会議での議論の中心となっており、マーケティング、ヘルスケア、ゲームなどさまざまな分野でその能力を発揮しています。
ジェネラティブAIがビジネスに与える主な利点は何ですか?
-ジェネラティブAIはビジネスにとって、顧客体験の向上、従業員の生産性の向上、会話型検索、テキスト要約、コード生成支援、ビジネスオペレーションの最適化など、多くの利点をもたらします。
ジェネラティブAIを活用するためにはどのような技術が必要ですか?
-ジェネラティブAIを活用するためには、大規模言語モデルや他の基礎モデルへのアクセス、モデルのカスタマイズ能力、タスク自動化能力、セキュリティとガバナンスの組み込みなどが必要です。
Amazon Bedrockとはどのようなサービスですか?
-Amazon Bedrockは、ジェネラティブAIアプリケーションを構築し、拡大するためのフルマネージドサービスです。さまざまなAI企業から提供される基礎モデルへのアクセスを提供し、カスタムモデルのインポートも可能です。
モデルのカスタマイズに必要なデータ管理とはどのようなものですか?
-モデルのカスタマイズには、ビジネスや顧客に関するデータが不可欠です。データは、汎用的なジェネラティブAIモデルとビジネスに適ったアプリケーションを区別する要素であり、データ管理はデータの統制と統合を意味します。
タスク自動化とは何を意味していますか?
-タスク自動化とは、ビジネスや顧客のために日常業務を自動化する自律的なエージェントを作成することを意味します。これには、多くの手動プログラミングが必要な場合があり、開発者がこれらのタスクを実行するためのステップを定義し、ワークフローを調整する必要があります。
セキュリティとガバナンスがジェネラティブAIになぜ重要ですか?
-セキュリティとガバナンスは、ジェネラティブAIにおいては、モデルの出力に対する信頼性と責任を確立するために不可欠です。これには、データプライバシーの保護、モデルの品質管理、人間による判断の重要性などが含まれます。
Amazon Qはどのようなサービスですか?
-Amazon Qは、セキュリティとプライバシーを念頭に置いて開発された、最も高度なジェネラティブAIアシスタントです。開発者やビジネスユーザに効率をもたらし、タスクの自動化や情報へのアクセスを支援します。
mral AIはどのような企業ですか?
-mral AIは、AI分野において新しいプレイヤーとして登場し、独自のアプローチを提供することを目指す企業です。フランスを拠点としており、効率と多言語のサポートに重点を置いています。
mral AIが今後どのような方向性を貫くんですか?
-mral AIは、今後も多様なタスクに対応するためのモデルの多様化と最適化に注力し、さらにはコードの垂直化や多感覚モデルの開発にも取り組む予定です。
Outlines
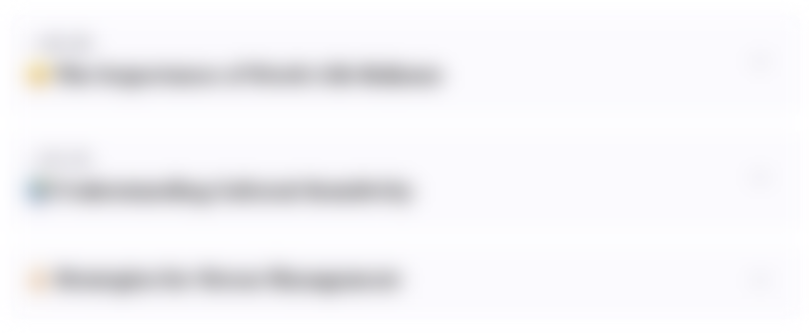
Dieser Bereich ist nur für Premium-Benutzer verfügbar. Bitte führen Sie ein Upgrade durch, um auf diesen Abschnitt zuzugreifen.
Upgrade durchführenMindmap
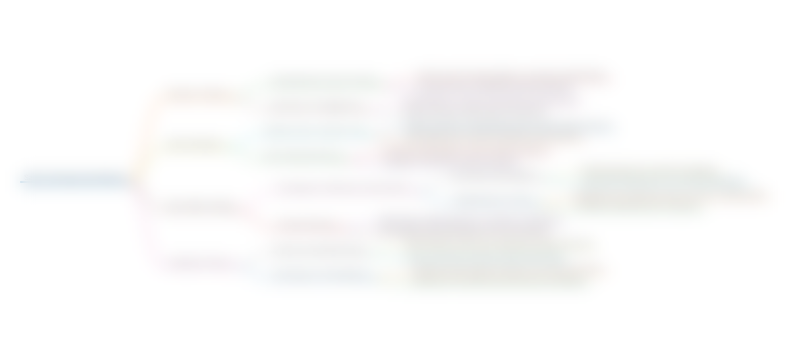
Dieser Bereich ist nur für Premium-Benutzer verfügbar. Bitte führen Sie ein Upgrade durch, um auf diesen Abschnitt zuzugreifen.
Upgrade durchführenKeywords
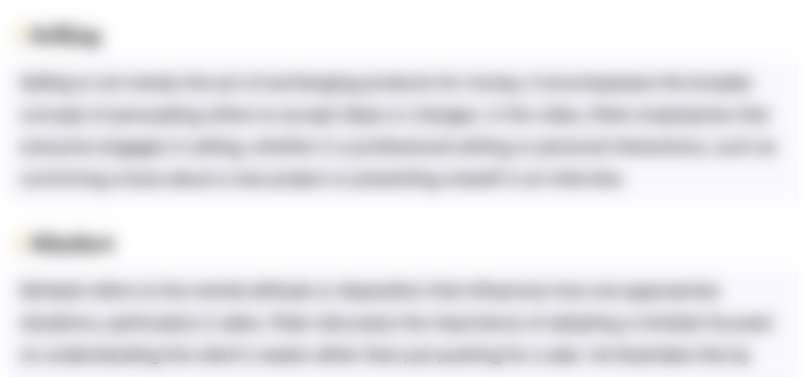
Dieser Bereich ist nur für Premium-Benutzer verfügbar. Bitte führen Sie ein Upgrade durch, um auf diesen Abschnitt zuzugreifen.
Upgrade durchführenHighlights
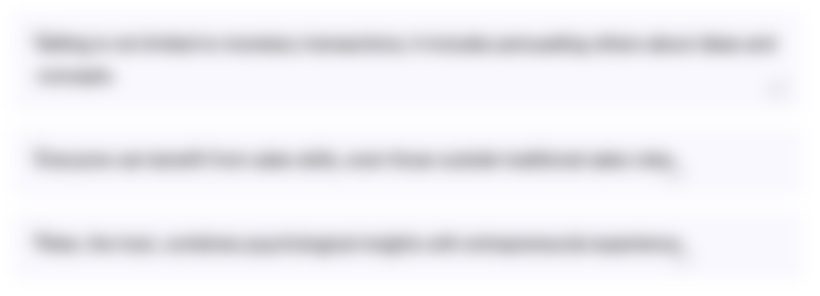
Dieser Bereich ist nur für Premium-Benutzer verfügbar. Bitte führen Sie ein Upgrade durch, um auf diesen Abschnitt zuzugreifen.
Upgrade durchführenTranscripts
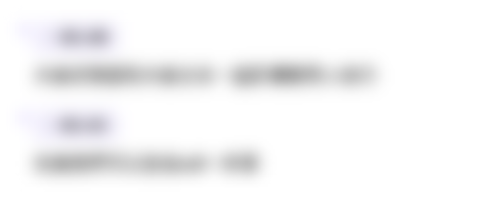
Dieser Bereich ist nur für Premium-Benutzer verfügbar. Bitte führen Sie ein Upgrade durch, um auf diesen Abschnitt zuzugreifen.
Upgrade durchführenWeitere ähnliche Videos ansehen
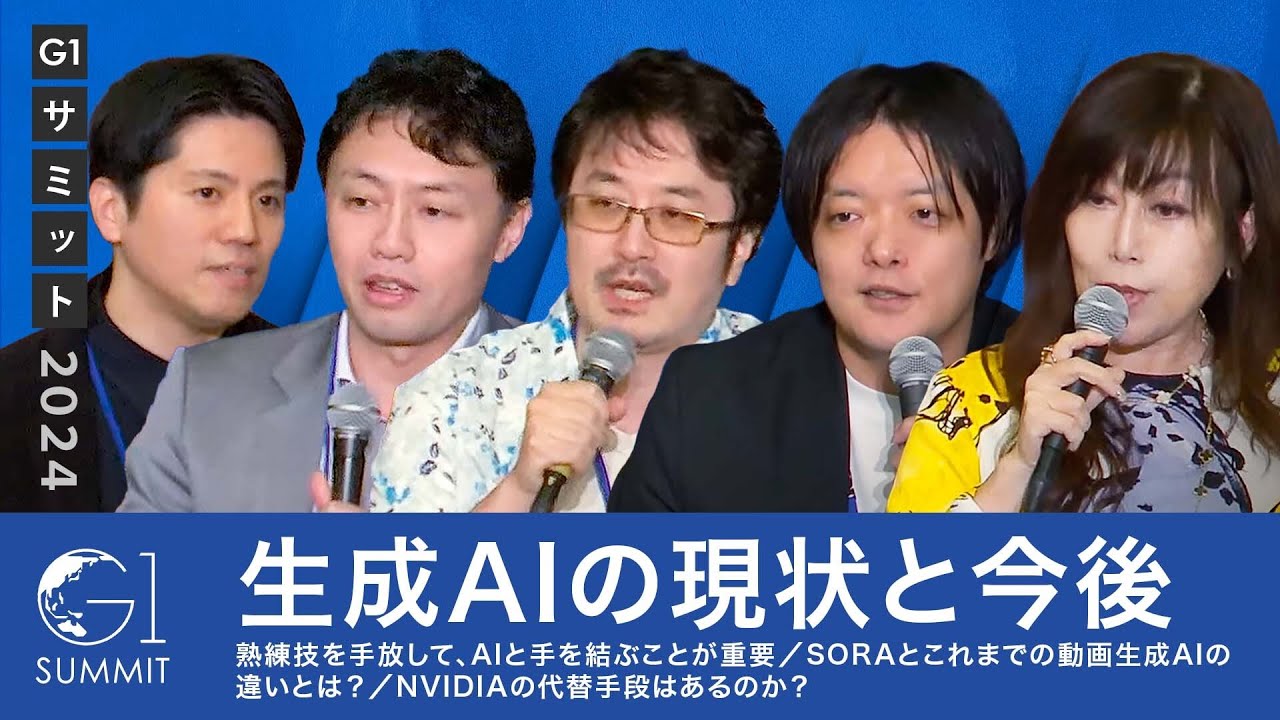
生成AIの現状と今後/熟練技を手放して、AIと手を結ぶことが重要/Soraとこれまでの動画生成AIの違いとは?/NVIDIAの代替手段はあるのか?【松尾豊×岩村水樹×上野山勝也×川上英良×関灘茂】
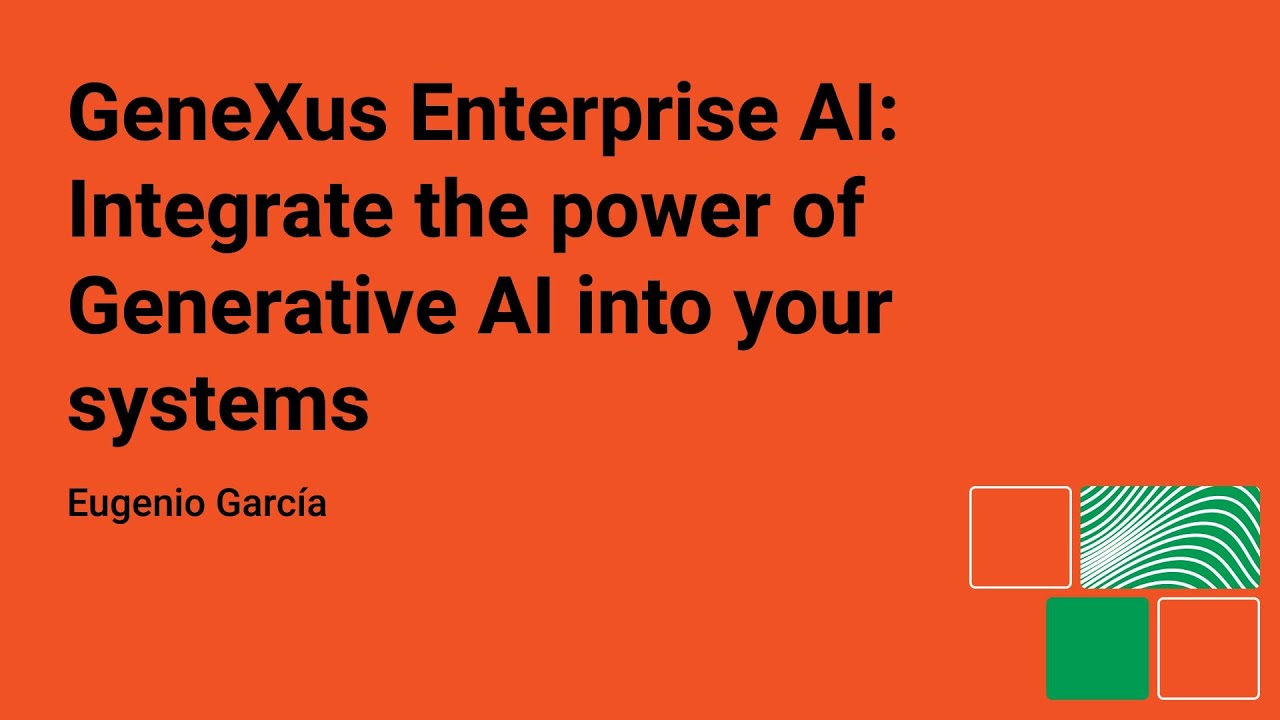
GeneXus Enterprise AI: Integrate the power of Generative AI into your systems
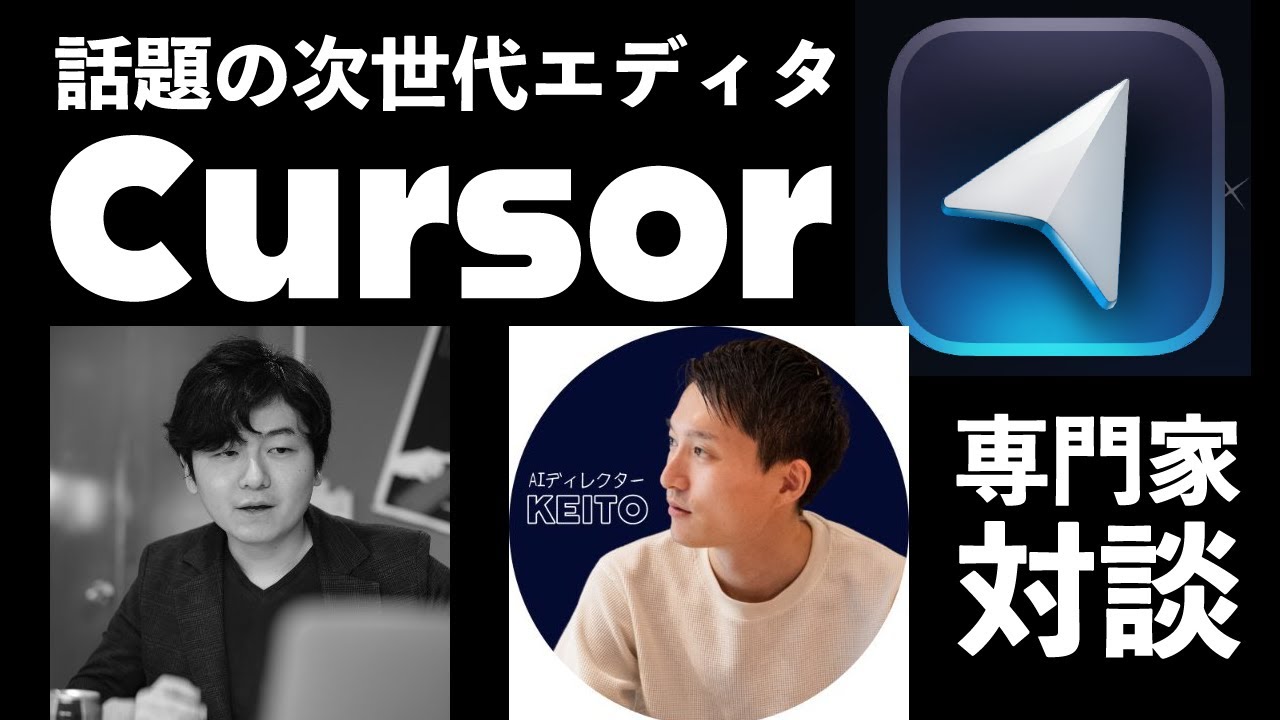
【IT関係者必見】話題の次世代AIエディタ「Cursor」について専門家に詳しく聞いてみた!
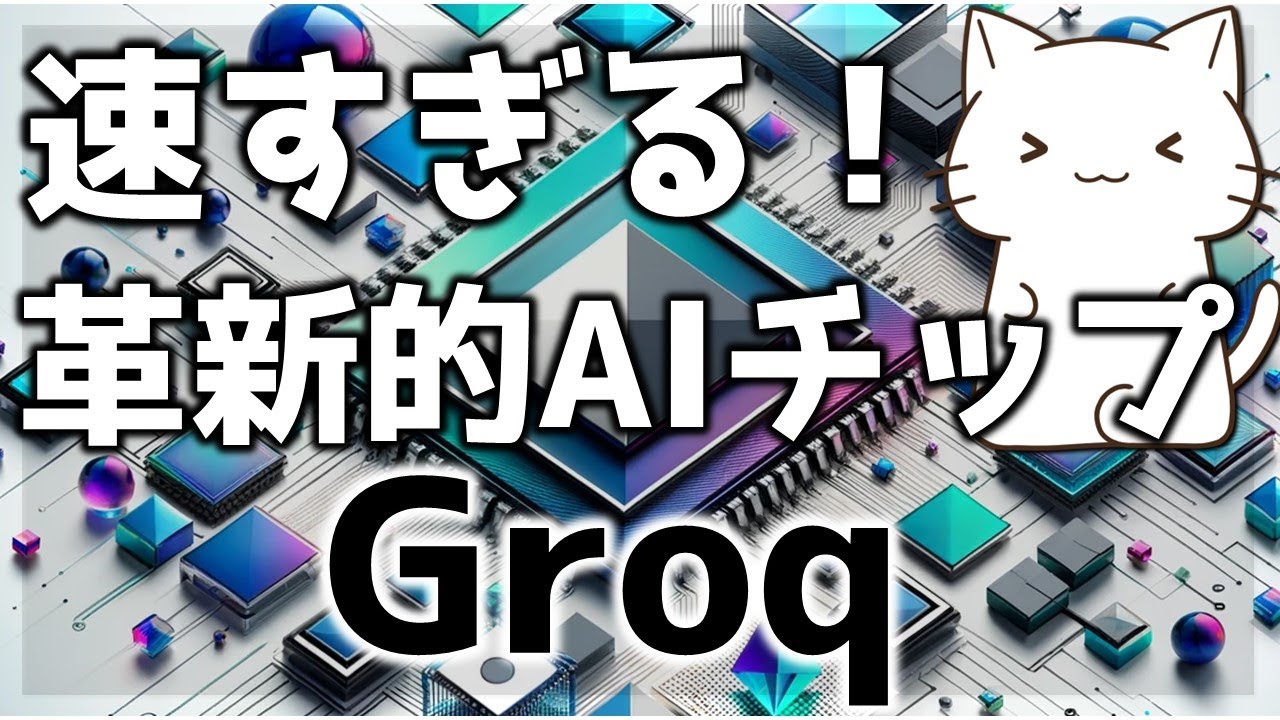
Nvidiaのライバル?革新的AIチップを開発するGroqについて解説してみた
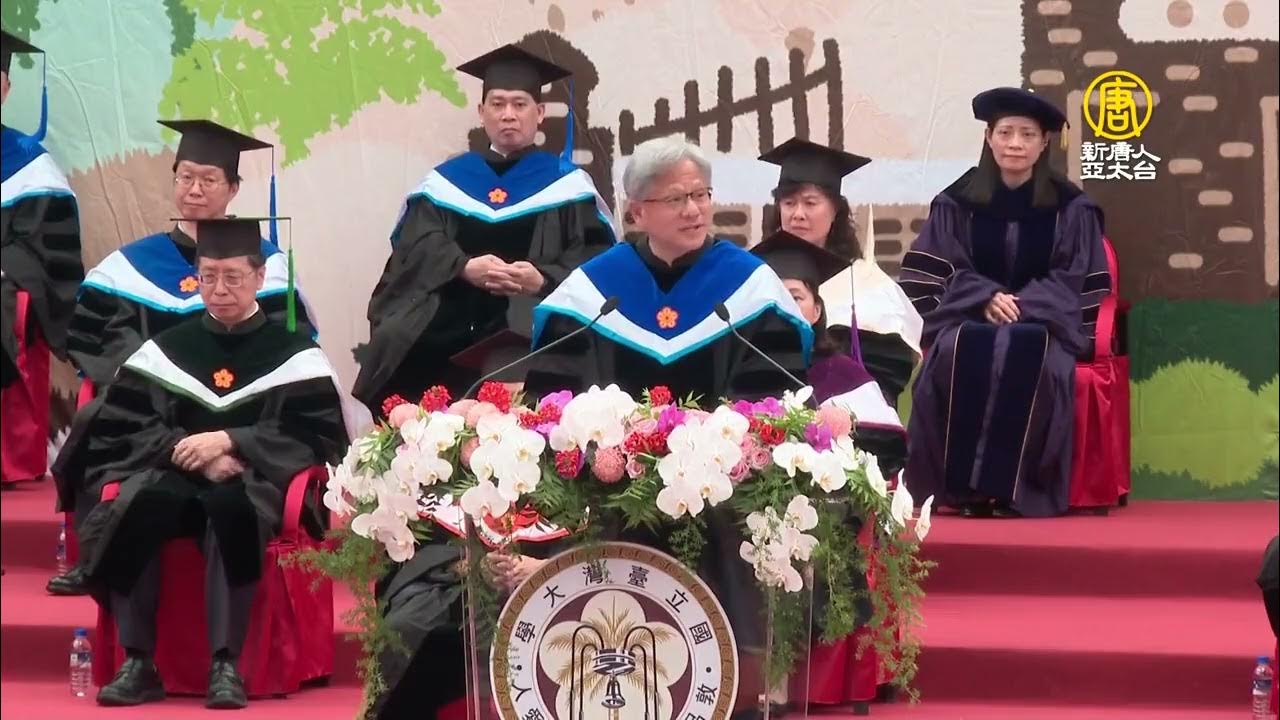
NVIDIA CEOのJensen Huangが国立台湾大学の卒業式(2023年5月27日)で行ったスピーチ、日本語字幕付き。

limited video summarize ing test
5.0 / 5 (0 votes)