Are We Automating Racism?
Summary
TLDRDas Skript beleuchtet die Herausforderungen von künstlicher Intelligenz (KI) und Algorithmenbezug, insbesondere in Bezug auf rassistische Tendenzen. Es zeigt, wie KI-Systeme, die von menschlichen Entscheidungen und Vorurteilen beeinflusst sind, zu unerwarteten und unfairen Ergebnissen führen können. Das Video diskutiert Beispiele wie das Twitter-Bildzuschneiden, das rassistisch wirken könnte, und stellt die Notwendigkeit von Überwachung und ethischer Ausrichtung von KI-Technologien her. Es betont auch die Bedeutung der Fragestellung und der Verantwortung der Schöpfer von KI-Werkzeugen gegenüber der Gesellschaft.
Takeaways
- 🤖 Künstliche Intelligenz (KI) kann diskriminierend sein, selbst wenn sie neutrale Absichten hat und keine menschliche Vorurteile enthält.
- 📈 Datengetriebene Systeme werden zunehmend in unserem Leben eine größere Rolle spielen, aber ihre Fehlfunktionen betreffen nicht alle gleich.
- 🖼️ Algorithmen können aufgrund von Datenmangel an Vielfalt oder fehlerhaften Beispielen rassistisch wirken, selbst wenn das nicht beabsichtigt ist.
- 👀 KI-Systeme lernen von Beispielen, die von Menschen ausgewählt, gekennzeichnet und abgeleitet wurden.
- 🔍 Das Problem von KI-Bias ist groß und schwierig, aber es bedeutet nicht, dass wir auf maschinelles Lernen verzichten müssen.
- 📊 Eine der Vorteile von Computer-Bias im Vergleich zu menschlichem Bias ist, dass man ihn leicht messen und verfolgen kann.
- 🌐 Die Auswahl und Verwendung von Algorithmen hängt von Ressourcen und Macht ab und wer die Interessen durch ein prädiktives Modell vertreten werden soll.
- 📋 Modelle sollten dokumentiert werden, einschließlich ethischer Bedenken, wie der beabsichtigte Gebrauch, die Herkunft der Daten und Anweisungen zur Bewertung der Systemleistung.
- 🚫 Es ist wichtig, vorsichtig zu sein, wo und wie wir KI-Systeme einsetzen und welche menschliche Überwachung wir über diese Systeme haben.
- 🧐 Die Auswahl der Daten und das Labeling beeinflussen die KI-Modelle erheblich und können zu unerwünschten Ergebnissen führen, wenn nicht sorgfältig gemacht.
- 🤔 Die Frage, welche Technologien entwickelt und wie sie in unserer Welt eingesetzt werden, reduziert sich auf Machtdynamiken und die Interessen derjenigen, die die Fragen stellen.
- 📝 Die Verantwortung der Designer und Programmierer geht über die Rechenschaft gegenüber ihren Kunden hinaus und betrifft auch die Auswirkungen, die diese Werkzeuge in der Welt haben.
Q & A
Was ist das Hauptthema des Transcripts?
-Das Hauptthema des Transcripts ist die Diskussion über den algorithmischen Bias und die Auswirkungen von künstlicher Intelligenz (KI) auf die Gesellschaft, insbesondere in Bezug auf Diskriminierung und Vorurteile.
Wie kann ein Bildschirm- oder Fotocropping-Algorithmus diskriminierend wirken?
-Ein Bildschirm- oder Fotocropping-Algorithmus kann diskriminierend wirken, indem er bei der Auswahl des zu zeigenden Bildausschnitts zwischen verschiedenen Gesichtern eine Ungleichheit aufweist, z.B. indem er voreingenommen weiße Gesichter bevorzugt.
Was ist ein 'Saliency Prediction Model'?
-Ein 'Saliency Prediction Model' ist eine Art KI-Software, die darauf abzielt, die wichtigen Elemente in einem Bild zu identifizieren. Es basiert auf den Daten, die durch das Beobachten von menschlichen Augenbewegungen bei der Betrachtung von Bildern gesammelt wurden.
Wie kann die Auswahl von Daten, die zur Schulung eines Modells verwendet werden, zu Bias führen?
-Die Auswahl von Daten kann zu Bias führen, wenn sie nicht repräsentativ für die verschiedenen Bevölkerungsgruppen ist. Wenn beispielsweise ein Datensatz viele Katzenbilder enthält, aber nur wenige dunkelhäutige Gesichter, kann das Modell lernen, Katzen als wichtiger zu erkennen als bestimmte Menschen.
Was ist ein Beispiel für die Verwendung von KI in der Gesundheitsversorgung, die zu unerwünschten Ergebnissen führen kann?
-Im Text wird ein Beispiel genannt, bei dem ein Programm zur Identifizierung von Patienten mit chronischen Krankheiten zur Zusatzversorgung verwendet wurde. Das Programm basierte auf den Kosten der Patienten, was jedoch dazu führte, dass Patienten mit dunklerer Hautfarbe aufgrund von Kostenunterscheiden, die mit institutionalisierter Rassismus und mangelnder Versorgung zu tun hatten, nicht angemessen identifiziert wurden.
Wie können Modelle zur KI-Entwicklung verbessert werden, um Bias zu reduzieren?
-Modelle zur KI-Entwicklung können verbessert werden, indem sie mit einer Vielzahl von Daten trainiert werden, die eine breitere Repräsentanz der Bevölkerung widerspiegeln. Auch die Transparenz über die Datenherkunft und die Bezeichnung von Daten ist wichtig. Ein weiteres Werkzeug zur Verbesserung ist die sogenannte 'Modellkartei', die wichtige Informationen über das Modell und seine potenziellen ethischen Bedenken dokumentiert.
Was ist die Rolle von menschlicher Subjektivität in der KI-Entwicklung?
-Menschliche Subjektivität spielt eine große Rolle in der KI-Entwicklung, da Entscheidungen über die Auswahl und Beschriftung von Daten, die zur Schulung des Modells verwendet werden, von Menschen getroffen werden. Diese Entscheidungen können unbewusst Bias in den Algorithmen einfügen.
Wie kann die Verwendung von KI-Technologien in der Gesellschaft ethische und gesellschaftliche Herausforderungen hervorrufen?
-Die Verwendung von KI-Technologien kann ethische und gesellschaftliche Herausforderungen hervorrufen, da sie potenziell Diskriminierung und Ungerechtigkeit verfestigen können. Es ist wichtig, über die Auswirkungen von KI-Entscheidungen nachzudenken und sicherzustellen, dass sie nicht auf der Grundlage von Rasse, Geschlecht oder sozialem Status diskriminieren.
Was ist der Vorteil der Identifizierung von Bias in KI-Systemen gegenüber menschlichem Bias?
-Der Vorteil der Identifizierung von Bias in KI-Systemen ist, dass sie messbar und nachverfolgbar ist und es ermöglicht, an dem Modell zu arbeiten, um faire Ergebnisse zu erzielen, wenn man dazu motiviert ist.
Welche Rolle spielen Ressourcen und Macht bei der Entscheidung darüber, welche Technologien entwickelt und wie sie in unserer Welt eingesetzt werden?
-Ressourcen und Macht sind entscheidend, da sie bestimmen, wessen Interessen von einem prädiktiven Modell bedient werden und welche Fragen gestellt werden. Die Machtstrukturen legen fest, auf welchem Weg die Technologie eingesetzt wird und wer davon profitiert oder benachteiligt wird.
Was ist die Verantwortung von Designern und Programmierern in Bezug auf die Auswirkungen der von ihnen entwickelten Werkzeuge in der Welt?
-Designer und Programmierer müssen sich fragen, ob sie nur ihren Clients gegenüber verantwortlich sind oder auch gegenüber der gesamten politischen Gemeinschaft. Sie müssen sich bewusst sein, welche Auswirkungen ihre Tools haben können und ob sie bereit sind, für diese Auswirkungen zu haften.
Was ist der Unterschied zwischen dem Bias in einem Computer und dem Bias beim Menschen?
-Der Unterschied liegt darin, dass der Bias in einem Computer leichter gemessen und verfolgt werden kann, während der menschliche Bias oft komplexer zu identifizieren und zu quantifizieren ist.
Wie kann die Transparenz in Bezug auf die Entwicklung von KI-Modellen verbessert werden?
-Die Transparenz kann verbessert werden, indem Unternehmen interne Dokumentationskulturen wie 'Modellkarteien' einführen, die wichtige Informationen über die Funktionsweise des Modells, die Datenherkunft und die ethischen Bedenken liefern.
Outlines
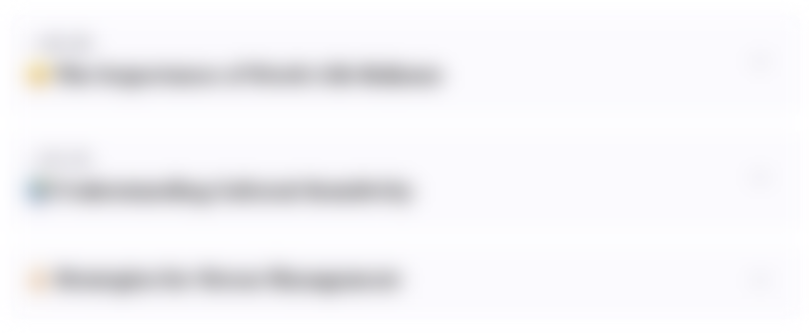
Dieser Bereich ist nur für Premium-Benutzer verfügbar. Bitte führen Sie ein Upgrade durch, um auf diesen Abschnitt zuzugreifen.
Upgrade durchführenMindmap
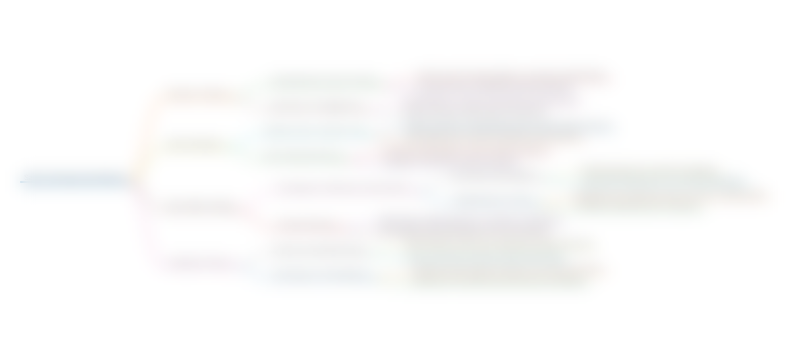
Dieser Bereich ist nur für Premium-Benutzer verfügbar. Bitte führen Sie ein Upgrade durch, um auf diesen Abschnitt zuzugreifen.
Upgrade durchführenKeywords
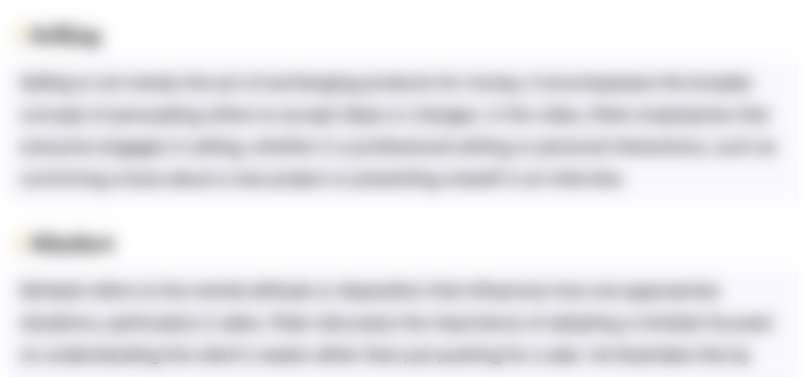
Dieser Bereich ist nur für Premium-Benutzer verfügbar. Bitte führen Sie ein Upgrade durch, um auf diesen Abschnitt zuzugreifen.
Upgrade durchführenHighlights
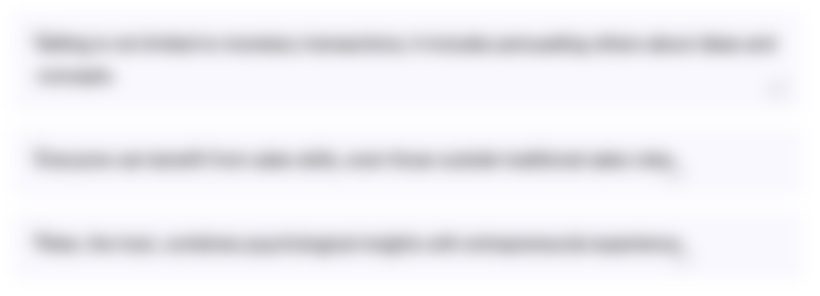
Dieser Bereich ist nur für Premium-Benutzer verfügbar. Bitte führen Sie ein Upgrade durch, um auf diesen Abschnitt zuzugreifen.
Upgrade durchführenTranscripts
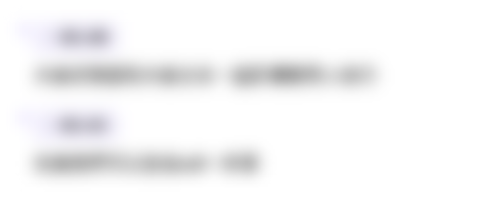
Dieser Bereich ist nur für Premium-Benutzer verfügbar. Bitte führen Sie ein Upgrade durch, um auf diesen Abschnitt zuzugreifen.
Upgrade durchführenWeitere ähnliche Videos ansehen
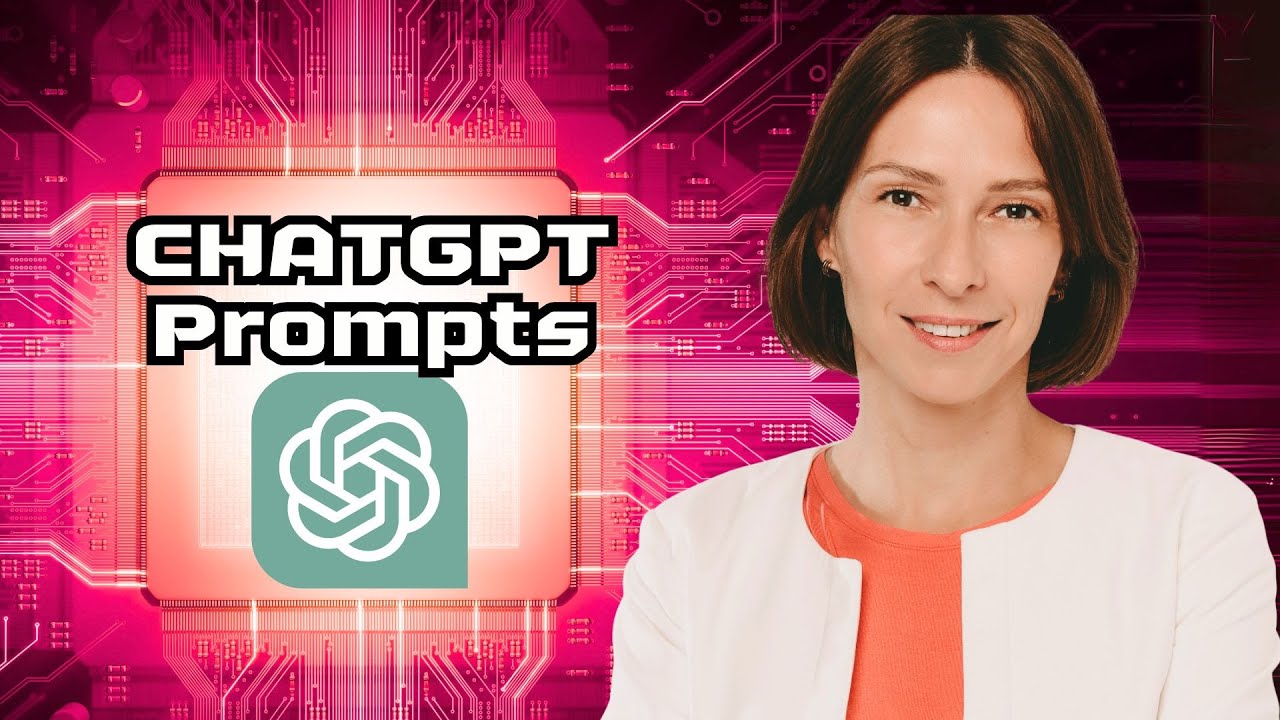
Wie erstelle ich gute Prompts für ChatGPT & Co?
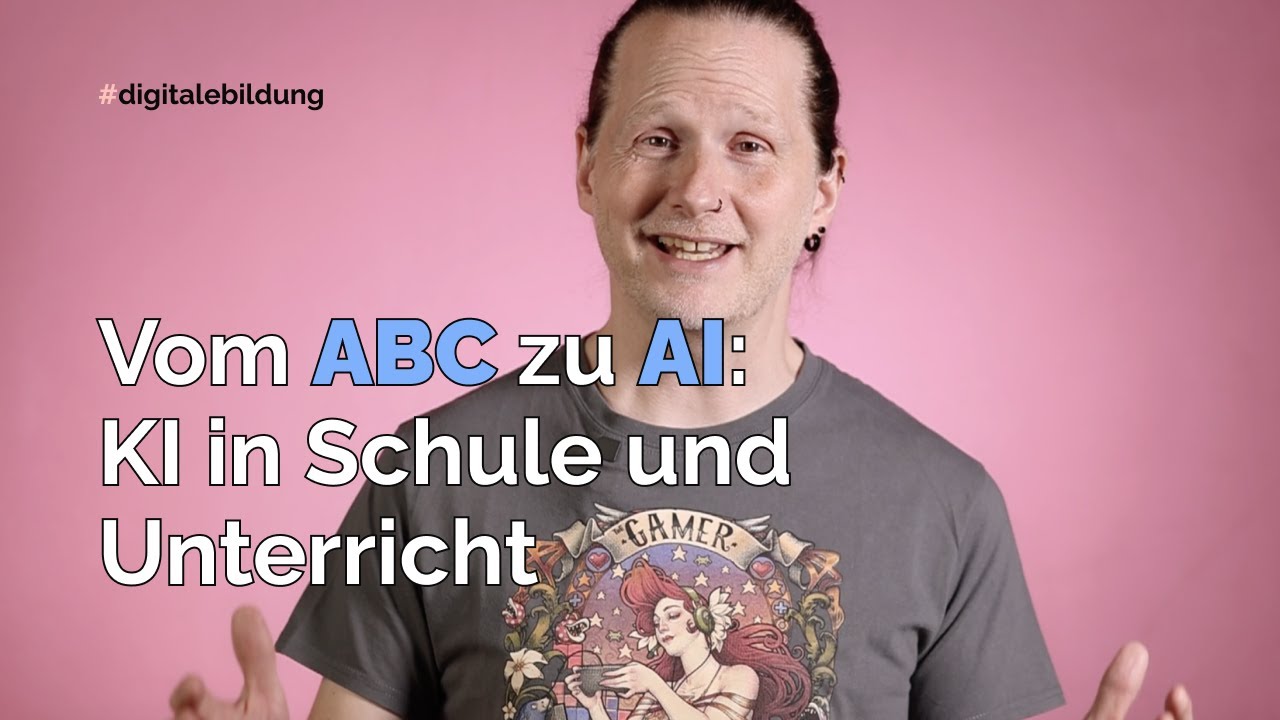
Vom ABC zu AI: KI in Schule und Unterricht
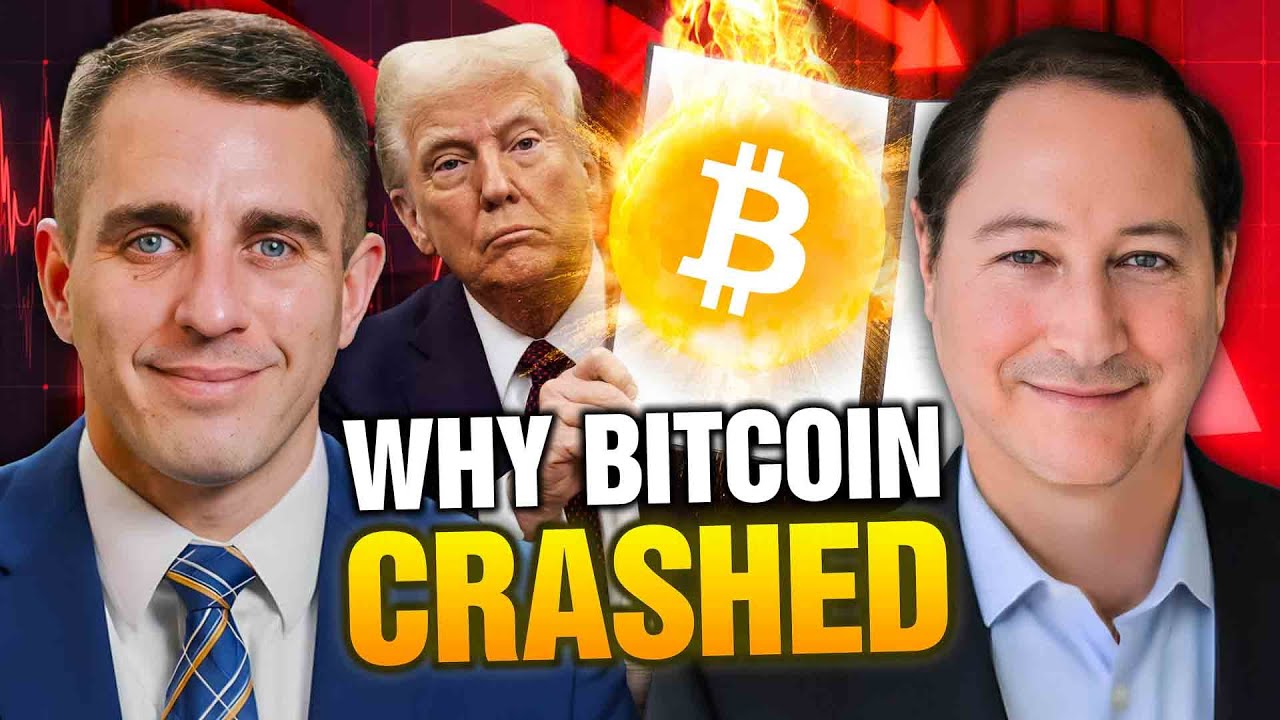
Why Bitcoin CRASHED After Trump Strategic Reserve News
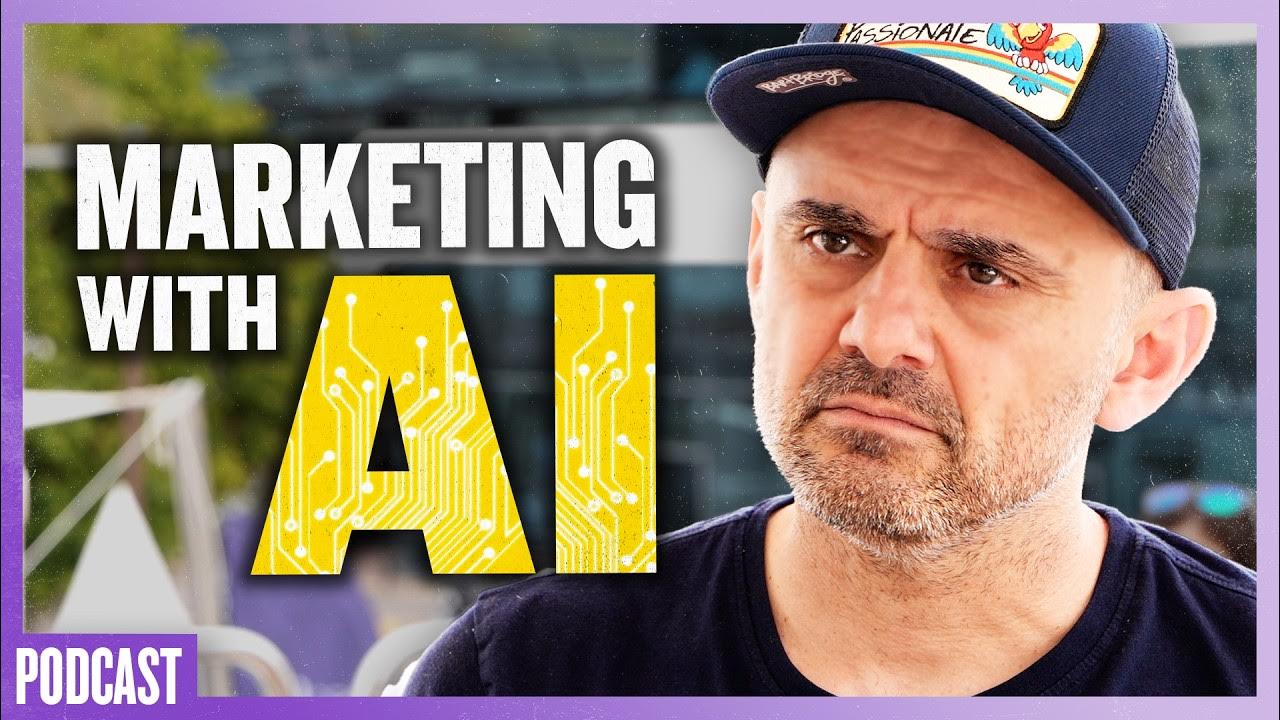
The Marketing Opportunities and Challenges With The Rise Of AI
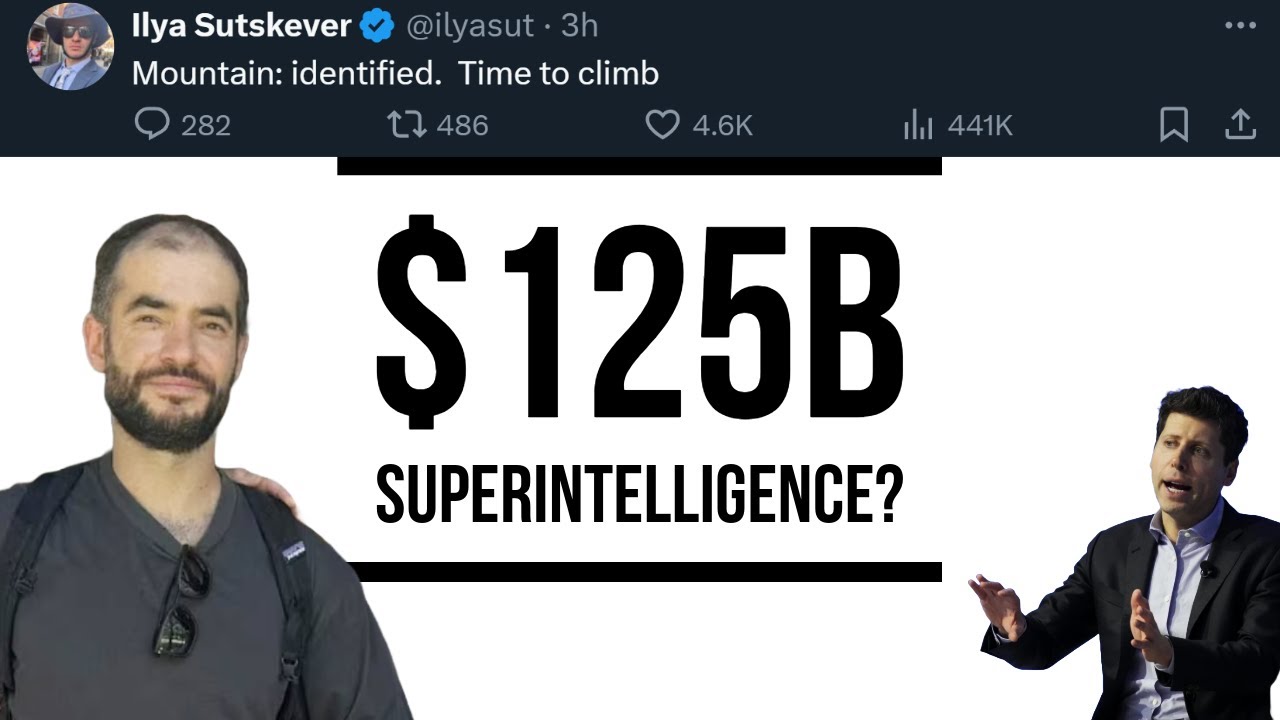
$125B for Superintelligence? 3 Models Coming, Sutskever's Secret SSI, & Data Centers (in space)...
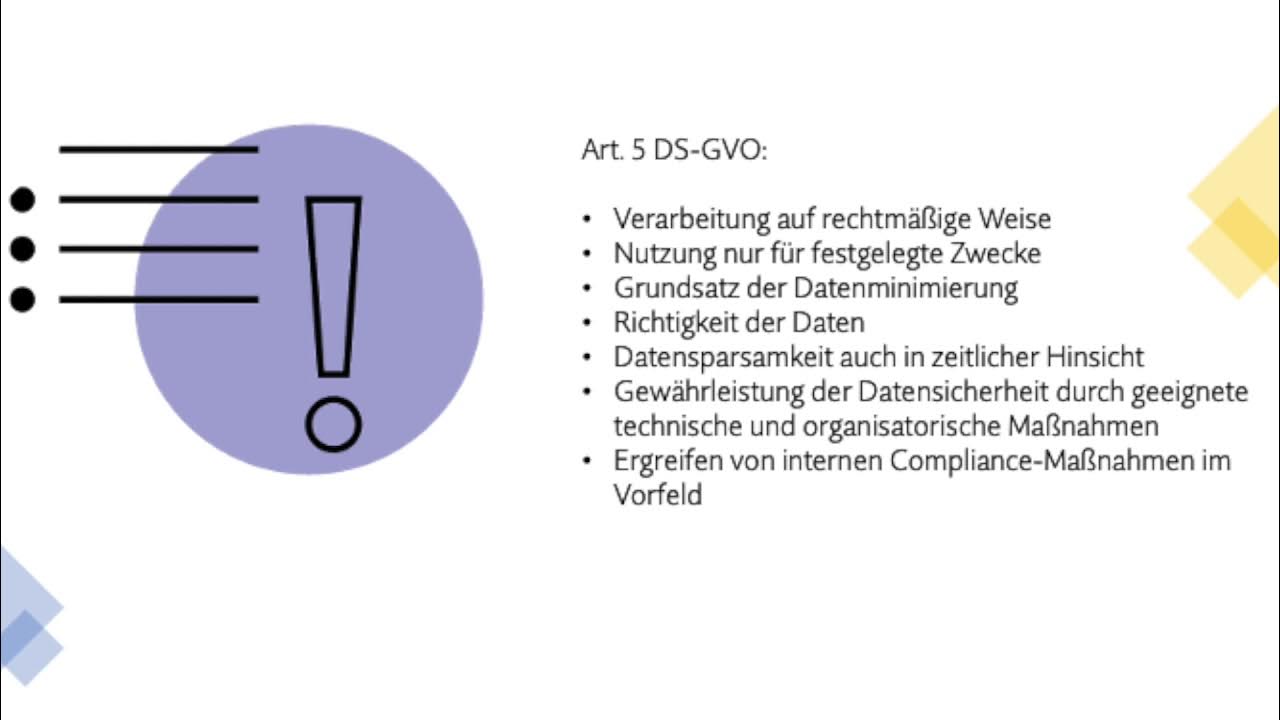
Lerneinheit2: Rechtliche Grundlagen zum Einsatz von Chatbots

KI und unsere Gesellschaft | Die Welt der KI entdecken 11
5.0 / 5 (0 votes)