WTF is an AI PC?
Summary
TLDRDans cette vidéo, NV Patel, fondateur et PDG de Framework, présente les modèles de langage avancés et leur utilisation sur des ordinateurs portables. Il discute de la manière dont les modèles locaux comme Meta's Llama 3.1 peuvent offrir des réponses intelligentes sans avoir à envoyer de données en ligne. Le PDG explore également les avantages de la confidentialité et de la personnalisation des modèles, ainsi que les progrès rapides dans le domaine de l'IA et de l'apprentissage automatique.
Takeaways
- 🤖 NV Patel, fondateur et PDG de Framework, discute d'IA sur une vidéo YouTube.
- 🧠 Il existe beaucoup de bruit autour de l'IA, mais Patel se concentre sur les cas d'utilisation réels et fonctionnels.
- 💡 Patel démontre comment exécuter le modèle 'llama 3.1' de Meta localement sur un ordinateur portable Framework.
- 🔍 Le modèle 'llama 3.1' répond rapidement à des questions complexes, montrant l'avancée de l'IA sur matériel de consommation.
- 🆚 Les grands modèles de langage comme GPT sont propriétaires et coûteux, contrairement aux modèles plus petits et ouverts comme 'llama 3.1'.
- 🌐 Exécuter des modèles localement permet de garder le contrôle total des données et de personnaliser le modèle.
- 🏆 'llama 3.1' se classe dans le top 10 du Chatbot Arena, prouvant la compétitivité des modèles ouverts.
- 🔢 Les paramètres du modèle (poids internes) déterminent sa capacité à apprendre et à répondre.
- 💾 Il est possible de réduire la profondeur des bits des modèles pour les faire tenir dans une mémoire GPU limitée.
- 🖥️ Les GPU sont adaptés pour exécuter des modèles IA en raison de leur capacité de traitement de matrices et de bande passante mémoire élevée.
- 📝 AMA est un outil simple pour exécuter des modèles de langage sur une machine personnelle sans connaissances techniques approfondies.
Q & A
Qu'est-ce que NV Patel mentionne comme problème avec l'hype autour de l'IA?
-Il mentionne qu'il y a beaucoup de bruit et d'informations non essentielles ('noise and BS') autour des sujets à la mode, et qu'il préfère se concentrer sur ce qui est réel et fonctionne.
Quel est l'objectif de NV Patel lorsqu'il parle de l'IA?
-Il veut éviter les cas d'utilisation hype et parler de ce qui fonctionne réellement avec l'IA sur des matériels réels.
Pourquoi NV Patel n'est-il pas un expert en IA?
-Il se décrit comme un amateur, et son approche est donc de partager ce qu'il a appris jusqu'à présent plutôt que de parler avec l'autorité d'un expert.
Quel modèle d'IA a-t-il exécuté sur un ordinateur portable Framework?
-Il a exécuté le modèle 'llama 3.1' de Meta.
Quelle question a-t-il posée au modèle 'llama 3.1' lors de la démonstration?
-Il a demandé au modèle ce qui est important concernant le droit de réparation.
Quels sont les avantages de l'exécution d'un modèle IA localement?
-Les avantages incluent le contrôle total, la sécurité des données (elles ne quittent pas la machine), et la possibilité de modifier le modèle ou d'inspecter ce qui se passe en coulisses.
Quelle est la différence entre les modèles de langage géants comme ChatGPT et les modèles plus petits?
-Les grands modèles comme ChatGPT ont des centaines de milliards de paramètres et s'exécutent sur des serveurs coûteux, tandis que les petits modèles sont conçus pour s'exécuter sur des GPU de niveau consommateur.
Quels sont les modèles IA qui commencent à être compétitifs avec les plus grands modèles?
-Les modèles 'llama 3.1' de Meta sont mentionnés comme étant compétitifs avec les plus grands modèles tout en étant suffisamment petits pour s'exécuter sur un ordinateur portable.
Quel est le rôle des paramètres dans un modèle IA?
-Les paramètres sont les poids ou les éléments à l'intérieur du modèle, et plus il y en a, plus le modèle peut être intelligent, en gros.
Pourquoi les GPU sont-elles efficaces pour exécuter des modèles IA localement?
-Les GPU ont beaucoup de bande passante mémoire et de capacité de multiplication de matrices et de débit, ce qui est utile pour le machine learning, similaire à la façon dont elles sont utilisées pour les jeux vidéo.
Quel outil a utilisé NV Patel pour exécuter des modèles de langage sur son ordinateur portable?
-Il a utilisé un outil appelé 'ama', développé par un groupe appelé 'ama', pour exécuter des modèles de langage.
Quels sont les autres types de modèles IA que mentionne NV Patel?
-Il mentionne les modèles de vision, comme 'lava' et 'lava llama 3', qui peuvent analyser des images et interagir avec l'utilisateur en fonction de l'image.
Quel est l'intérêt de la génération d'images avec des modèles IA?
-La génération d'images est une autre application intéressante de l'IA où des modèles comme 'stable diffusion' ou 'flux' peuvent créer des images à partir de rien.
Outlines
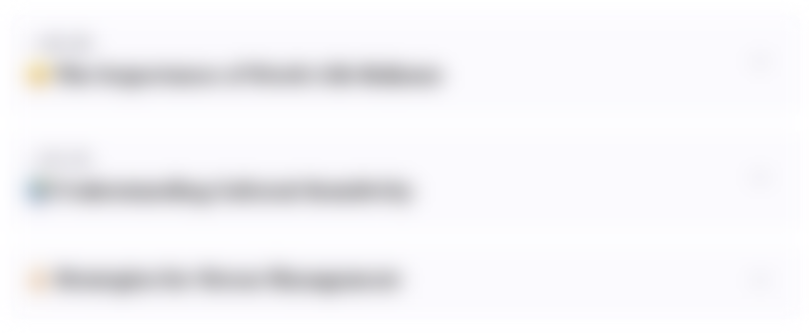
Dieser Bereich ist nur für Premium-Benutzer verfügbar. Bitte führen Sie ein Upgrade durch, um auf diesen Abschnitt zuzugreifen.
Upgrade durchführenMindmap
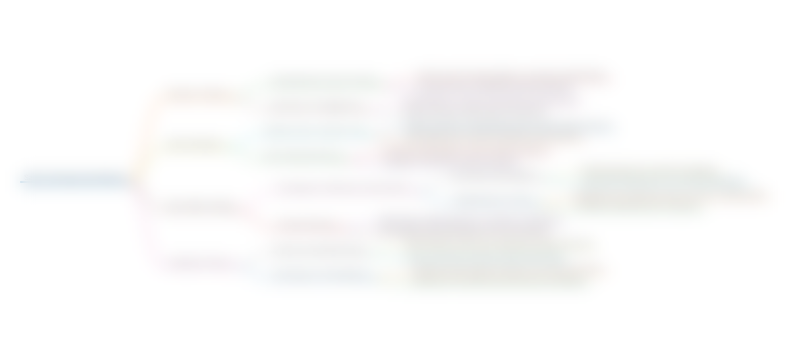
Dieser Bereich ist nur für Premium-Benutzer verfügbar. Bitte führen Sie ein Upgrade durch, um auf diesen Abschnitt zuzugreifen.
Upgrade durchführenKeywords
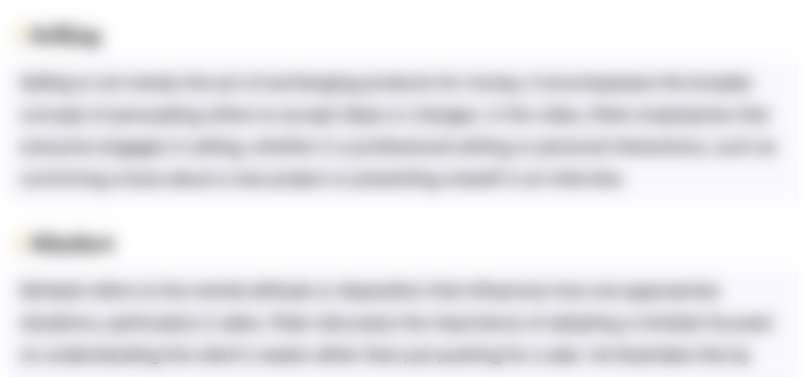
Dieser Bereich ist nur für Premium-Benutzer verfügbar. Bitte führen Sie ein Upgrade durch, um auf diesen Abschnitt zuzugreifen.
Upgrade durchführenHighlights
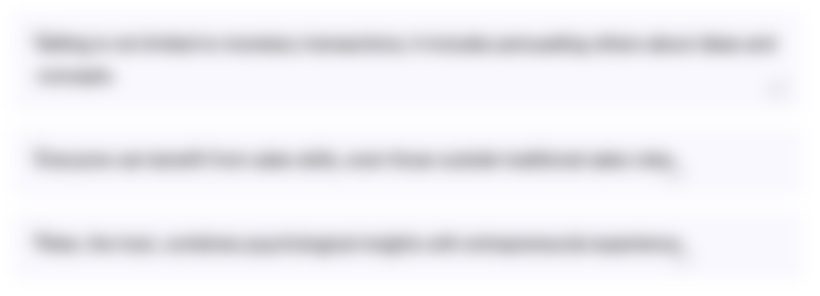
Dieser Bereich ist nur für Premium-Benutzer verfügbar. Bitte führen Sie ein Upgrade durch, um auf diesen Abschnitt zuzugreifen.
Upgrade durchführenTranscripts
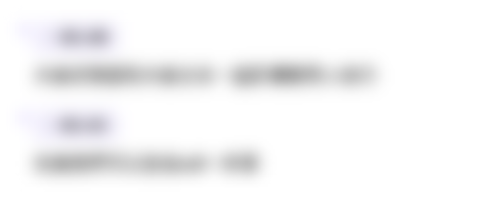
Dieser Bereich ist nur für Premium-Benutzer verfügbar. Bitte führen Sie ein Upgrade durch, um auf diesen Abschnitt zuzugreifen.
Upgrade durchführenWeitere ähnliche Videos ansehen
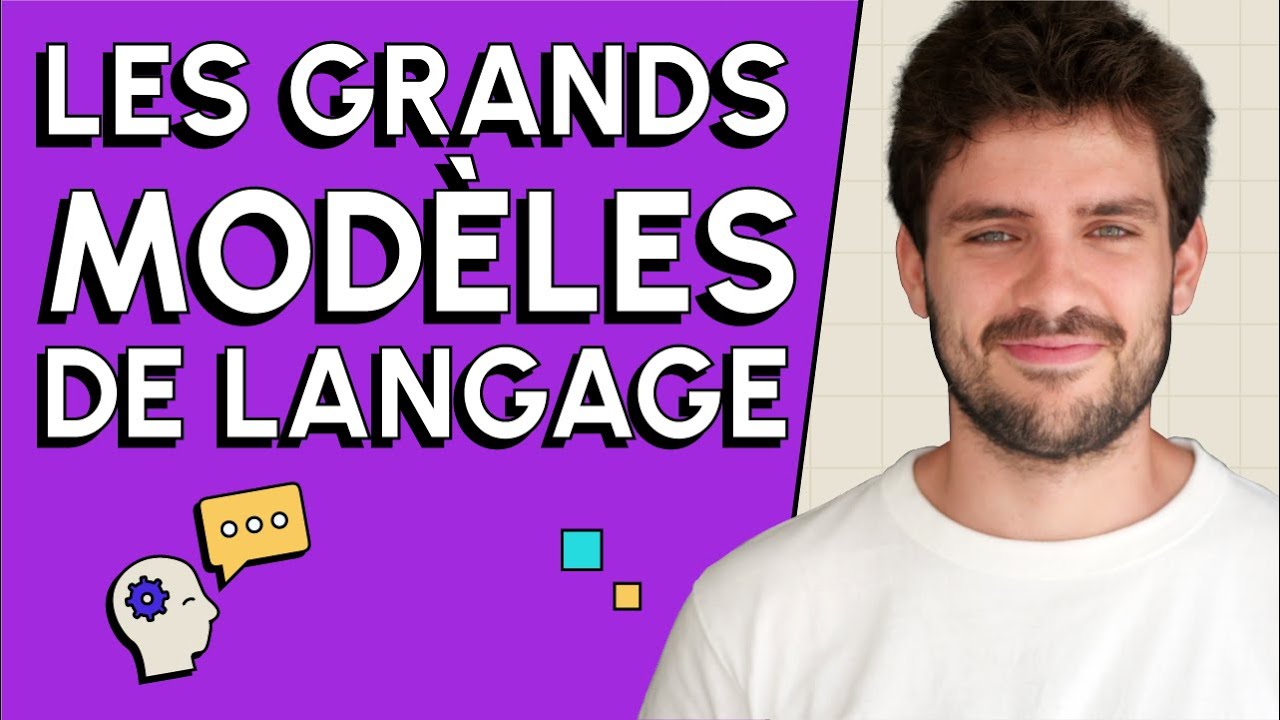
Que sont les Grands Modèles de langage (LLM) ?
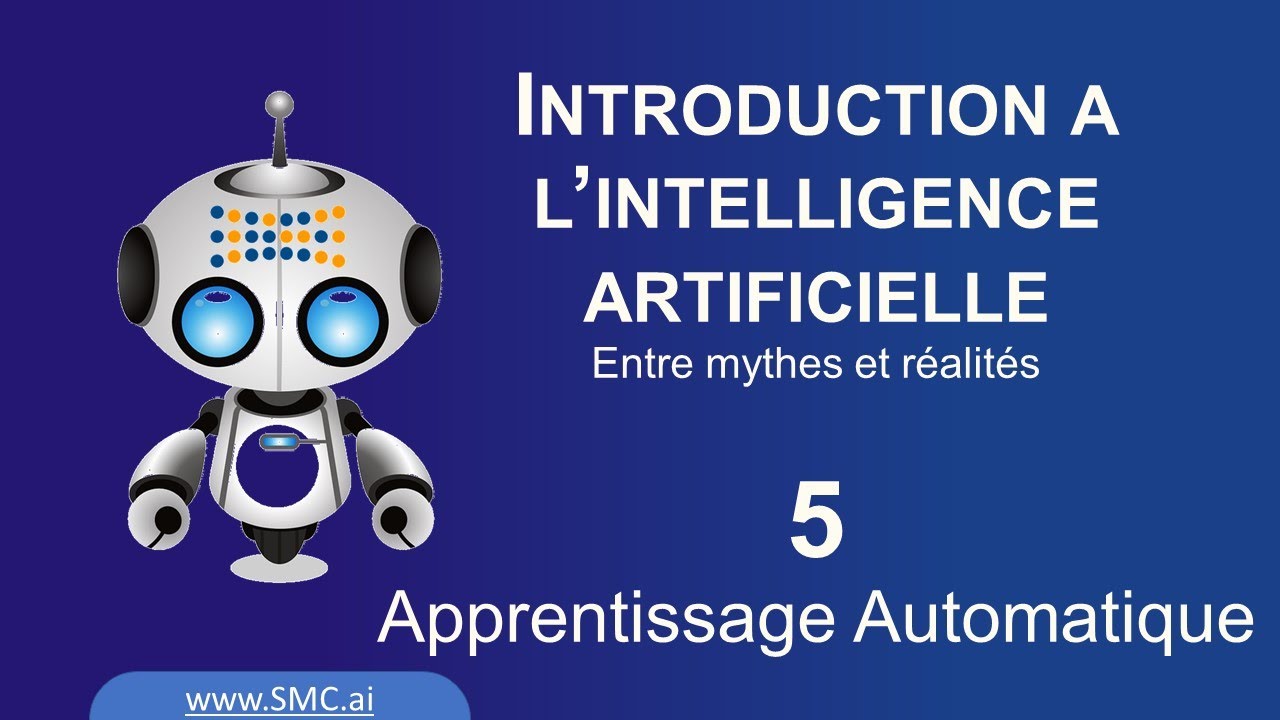
Introduction à l’intelligence artificielle - 5 - Apprentissage Automatique
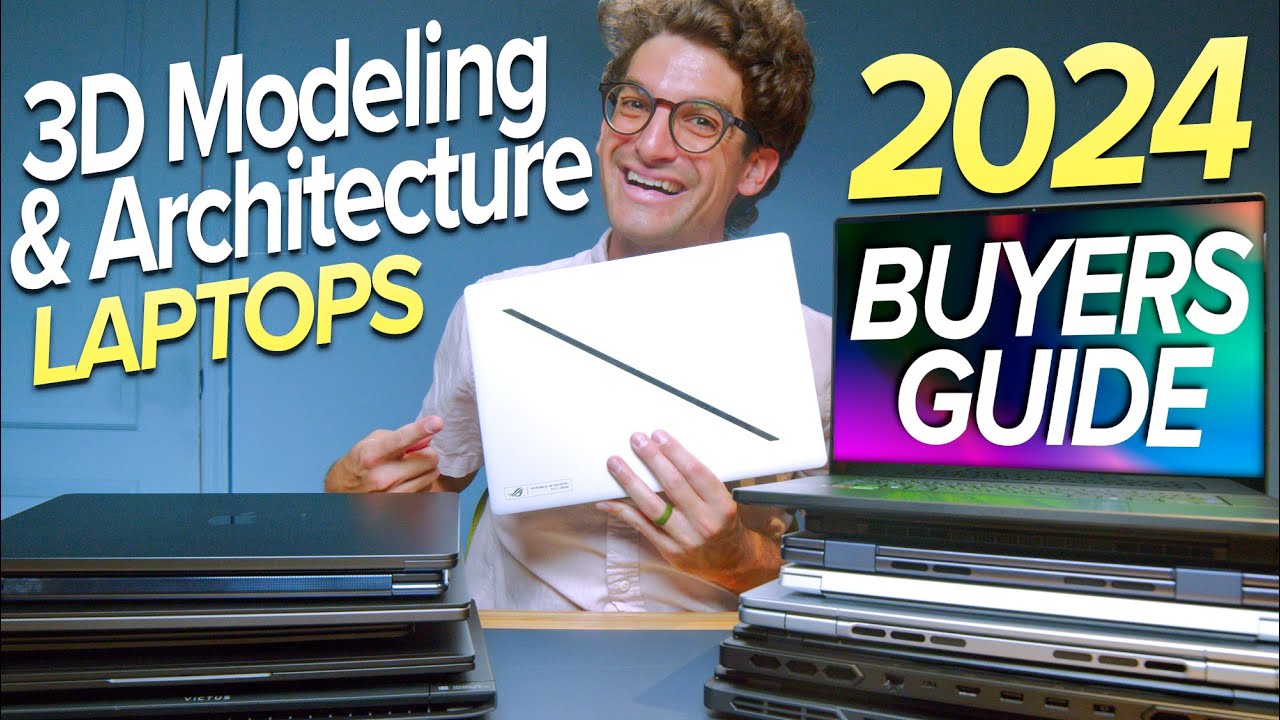
Best 3D Modeling & Architecture Laptops in 2024 | 3D Modeling Laptop Buyers Guide
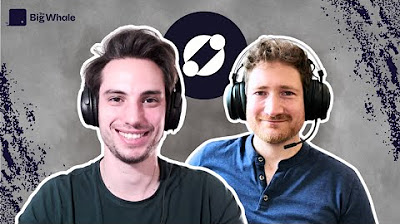
How DePIN can revolutionize the way AI works (With Jonathan Schemoul from Aleph.im)
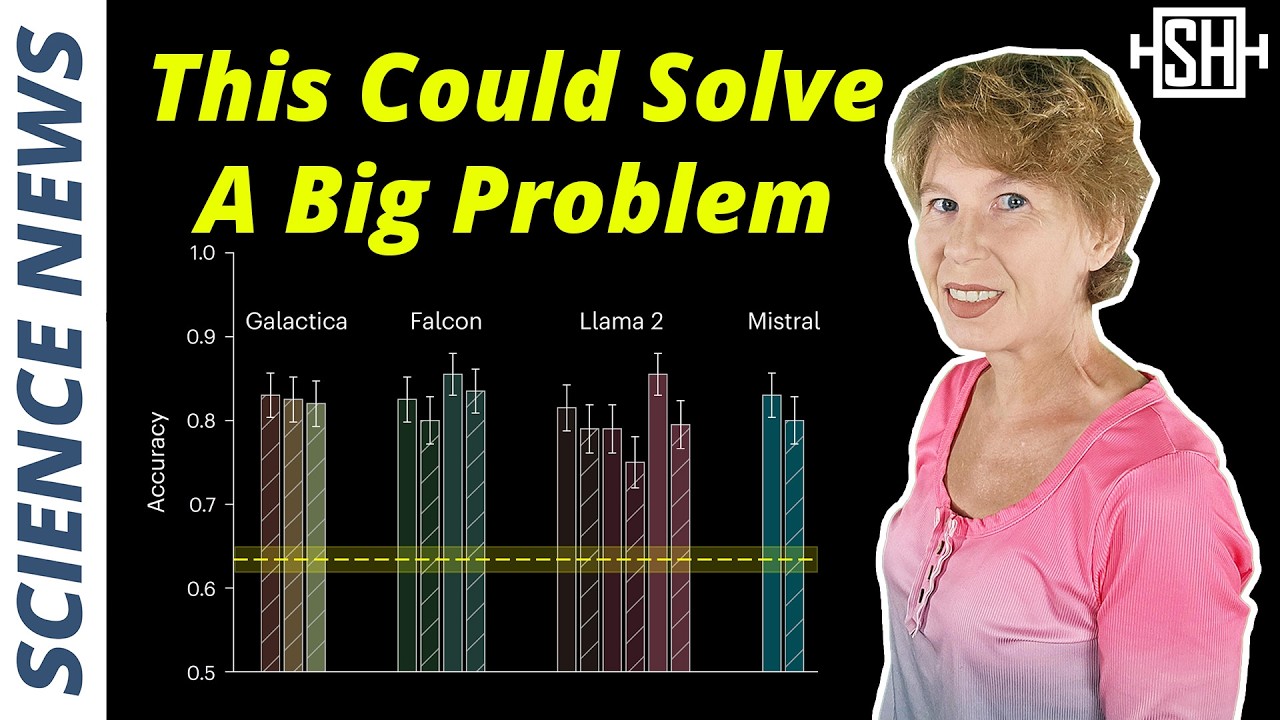
AIs Predict Research Results Without Doing Research

IA : Ce qu'on ne vous dit pas ...
5.0 / 5 (0 votes)