Finetuning Flux Dev on a 3090! (Local LoRA Training)
Summary
TLDRDans cette vidéo, l'orateur explique comment entraîner le modèle Flux Dev en utilisant l'outil AI d'OST. Il souligne l'importance d'avoir 24 Go de VRAM et partage des étapes détaillées sur la configuration du dossier de travail, la préparation du fichier YAML et l'intégration des dépendances. L'utilisateur apprend à configurer des hyperparamètres, des jeux de données et à générer des images de test. Le formateur explique également les cycles d'apprentissage du modèle, tout en donnant des conseils pour éviter un surapprentissage. En conclusion, il mentionne l'importance de la patience et de l'expérimentation dans l'entraînement des modèles.
Takeaways
- 💻 Assurez-vous d'avoir au moins 24 Go de VRAM, idéalement sur une carte graphique comme la 3090 Ti, pour faire fonctionner l'outil d'IA OST.
- 📂 Organisez vos fichiers dans des répertoires dédiés, comme un répertoire de travail 'work' avec un sous-dossier 'train' pour y regrouper tous les fichiers nécessaires.
- ⌨️ Suivez les étapes de configuration ligne par ligne pour installer les dépendances dans l'environnement de commande.
- 📑 Le fichier YAML est crucial pour la configuration des hyperparamètres, assurez-vous de copier et modifier l'exemple 'train_lora_flux_24g.yaml' pour adapter votre formation.
- 🖼️ Préparez correctement votre dataset avec des fichiers de texte et des images correspondants, et configurez la génération de fichiers tensor pour la sauvegarde régulière pendant l'entraînement.
- 🎨 Le modèle peut entraîner des styles différents, ici un style anime, avec des étapes régulières pour évaluer les images générées et ajuster les paramètres.
- ⚙️ L'outil permet l'utilisation de différentes tailles d'images grâce à la fonction de 'buckets', ce qui améliore l'efficacité du processus de formation.
- 🚀 Activez les options de 'shuffle tokens' et 'gradient checkpointing' pour optimiser l'entraînement sur les ressources VRAM limitées.
- 🔑 Configurez un fichier `.env` avec votre clé Hugging Face pour que le modèle puisse accéder aux ressources nécessaires pour la formation.
- ⏳ L'entraînement peut prendre environ 4 heures et vous pouvez observer des cycles d'apprentissage et de régression dans le processus, nécessitant plusieurs ajustements.
Q & A
Quelle est la configuration matérielle requise pour entraîner Flux Dev avec l'outil d'IA d'OST?
-Il est recommandé d'avoir une carte graphique avec 24 Go de VRAM, comme la 3090 Ti, pour que le processus d'entraînement fonctionne correctement sur une machine locale.
Pourquoi l'utilisateur doit-il configurer un fichier YAML?
-Le fichier YAML contient les hyperparamètres nécessaires à l'entraînement, comme le nom du modèle, les dossiers de sortie, et les paramètres de performance. Il est essentiel pour personnaliser et optimiser le processus d'entraînement.
Quels sont les principaux hyperparamètres à ajuster dans le fichier YAML?
-Les principaux hyperparamètres incluent le nom du fichier final, le dossier de sortie, les paramètres de la dimension (rank et dimension), la fréquence de sauvegarde des tensors, et la configuration des ensembles de données, entre autres.
Quel est l'intérêt d'utiliser des images de tailles différentes pendant l'entraînement?
-L'utilisation d'images de différentes tailles permet au modèle de s'entraîner avec des aspects variés, ce qui améliore sa flexibilité et sa capacité à générer des images dans plusieurs résolutions, rendant l'entraînement plus efficace.
Pourquoi l'utilisateur mentionne-t-il l'activation du mode 'gradient checkpointing'?
-L'activation du 'gradient checkpointing' permet de gérer les limitations de VRAM en économisant de la mémoire lors de l'entraînement, ce qui est utile pour les utilisateurs ayant des ressources limitées.
Pourquoi l'utilisateur saute-t-il certaines étapes d'échantillonnage au début de l'entraînement?
-L'utilisateur saute les échantillons initiaux pour éviter de perdre du temps et des ressources, car ces échantillons ne sont pas essentiels dans les premières étapes d'entraînement. Il préfère générer des échantillons à intervalles réguliers plus tard.
Comment l'utilisateur gère-t-il les espaces de stockage pendant l'entraînement?
-L'utilisateur configure le système pour conserver un nombre limité de fichiers de tensor (quatre dans cet exemple), en supprimant les plus anciens pour économiser de l'espace disque.
Pourquoi est-il important de configurer un token Hugging Face dans le processus?
-Le token Hugging Face est nécessaire pour accéder aux modèles et aux ressources externes hébergés sur Hugging Face, ce qui est crucial pour l'entraînement des modèles en utilisant les bibliothèques disponibles.
Quelles sont les étapes à suivre si l'on souhaite reprendre un entraînement interrompu?
-Il est possible de reprendre un entraînement interrompu en utilisant la fonctionnalité de reprise automatique à partir du dernier point de sauvegarde. Cette option peut être configurée dans le fichier YAML.
Quels sont les défis mentionnés lors de l'entraînement de modèles dans des cycles d'apprentissage?
-L'utilisateur observe que le modèle passe par des cycles d'apprentissage et de régression, où il apprend puis oublie certaines caractéristiques. Il recommande de ne pas arrêter l'entraînement trop tôt pour éviter une perte de nuances.
Outlines
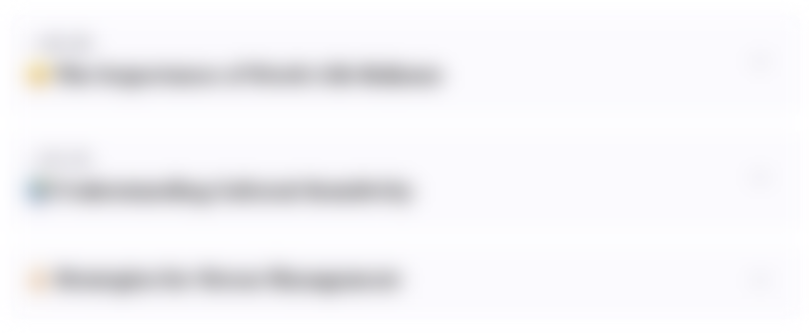
Dieser Bereich ist nur für Premium-Benutzer verfügbar. Bitte führen Sie ein Upgrade durch, um auf diesen Abschnitt zuzugreifen.
Upgrade durchführenMindmap
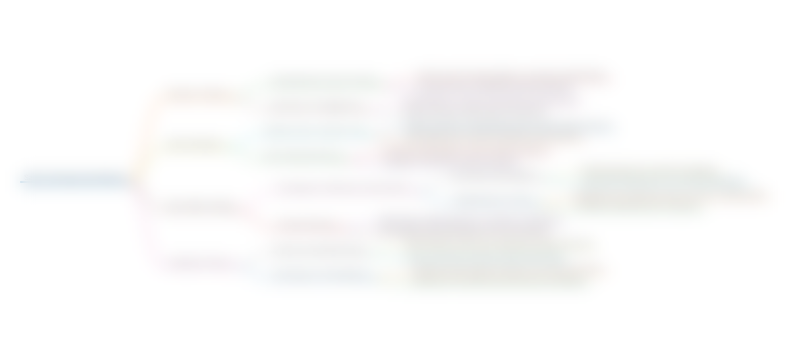
Dieser Bereich ist nur für Premium-Benutzer verfügbar. Bitte führen Sie ein Upgrade durch, um auf diesen Abschnitt zuzugreifen.
Upgrade durchführenKeywords
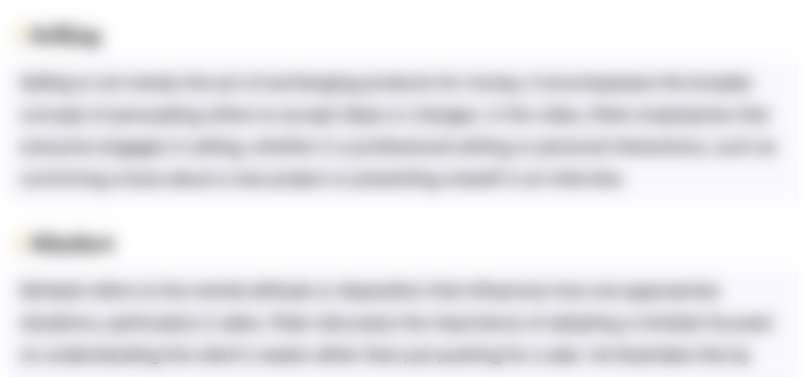
Dieser Bereich ist nur für Premium-Benutzer verfügbar. Bitte führen Sie ein Upgrade durch, um auf diesen Abschnitt zuzugreifen.
Upgrade durchführenHighlights
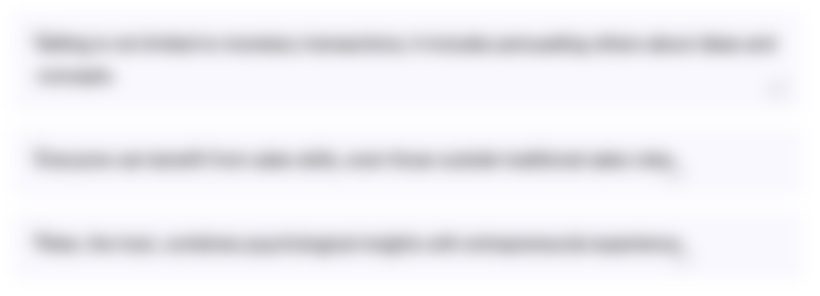
Dieser Bereich ist nur für Premium-Benutzer verfügbar. Bitte führen Sie ein Upgrade durch, um auf diesen Abschnitt zuzugreifen.
Upgrade durchführenTranscripts
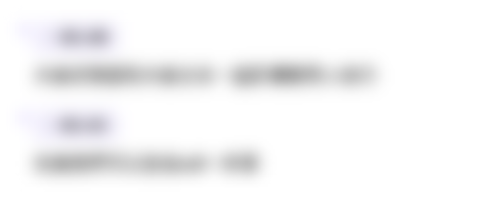
Dieser Bereich ist nur für Premium-Benutzer verfügbar. Bitte führen Sie ein Upgrade durch, um auf diesen Abschnitt zuzugreifen.
Upgrade durchführenWeitere ähnliche Videos ansehen

How To Create PERSONALIZED AI IMAGES With Flux - LoRA EXPLAINED
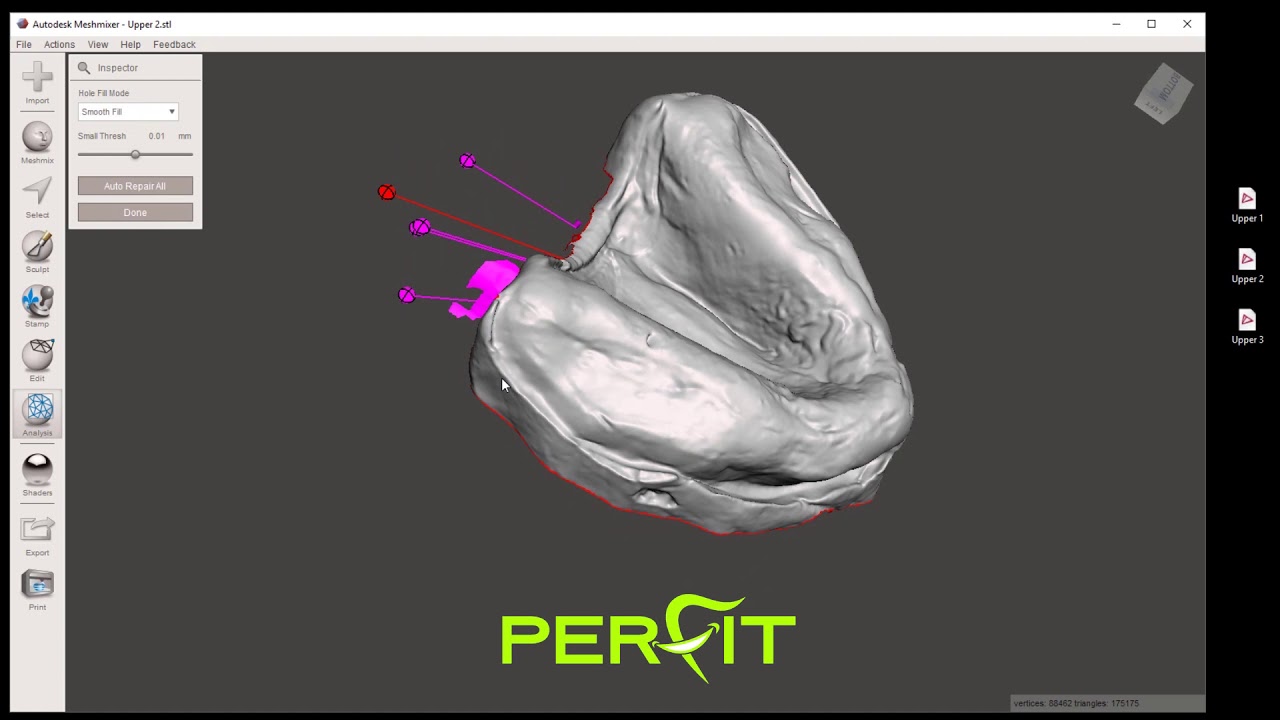
How to use the Analysis Inspector Tool
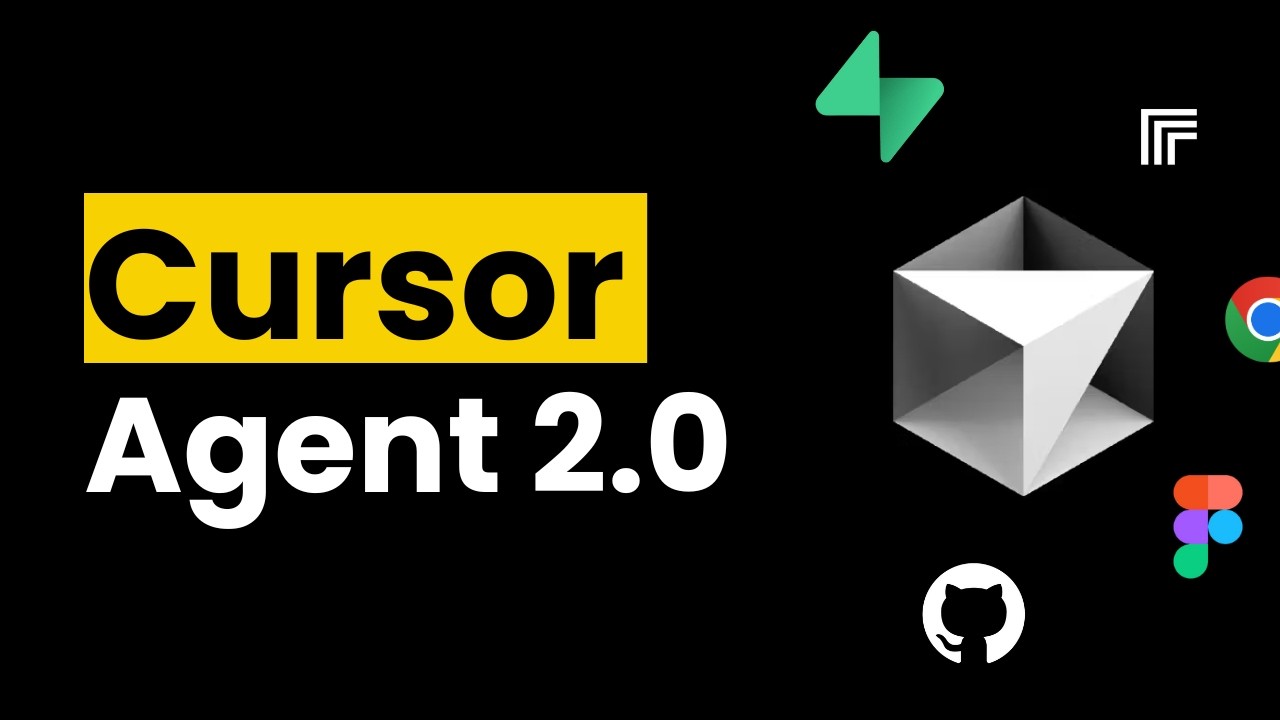
Those MCP totally 10x my Cursor workflow…
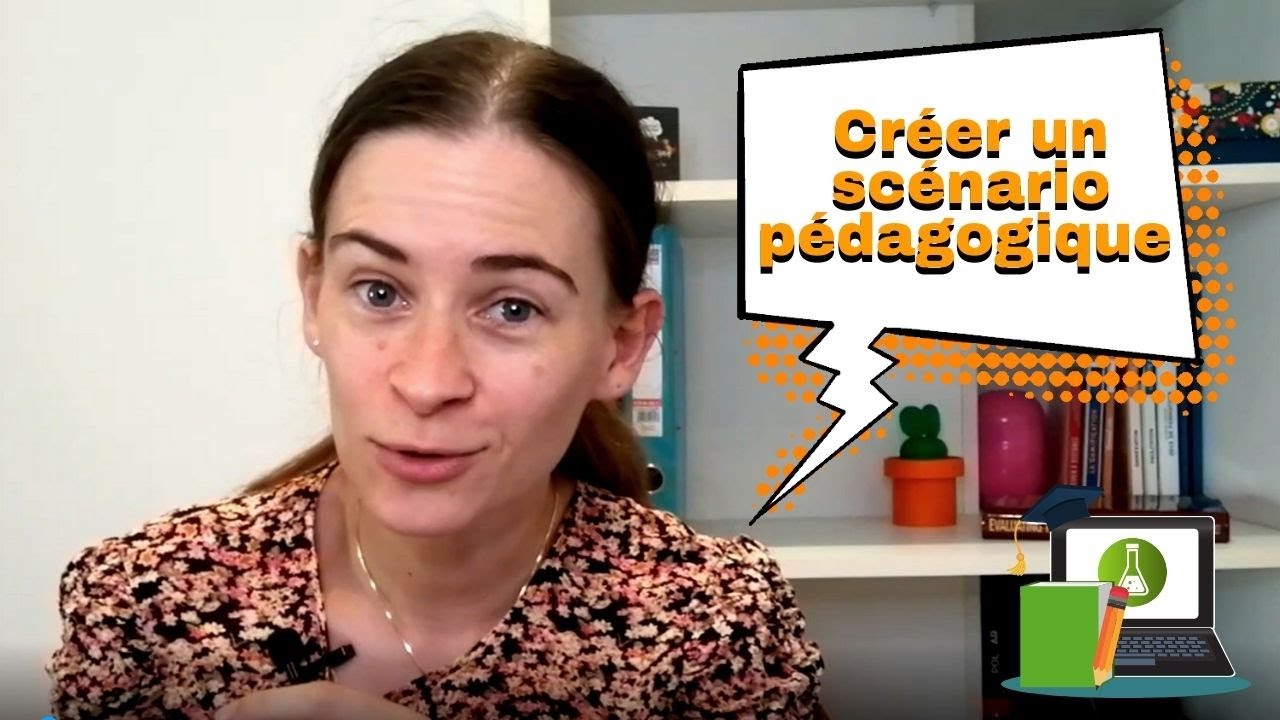
Créer un scénario pédagogique
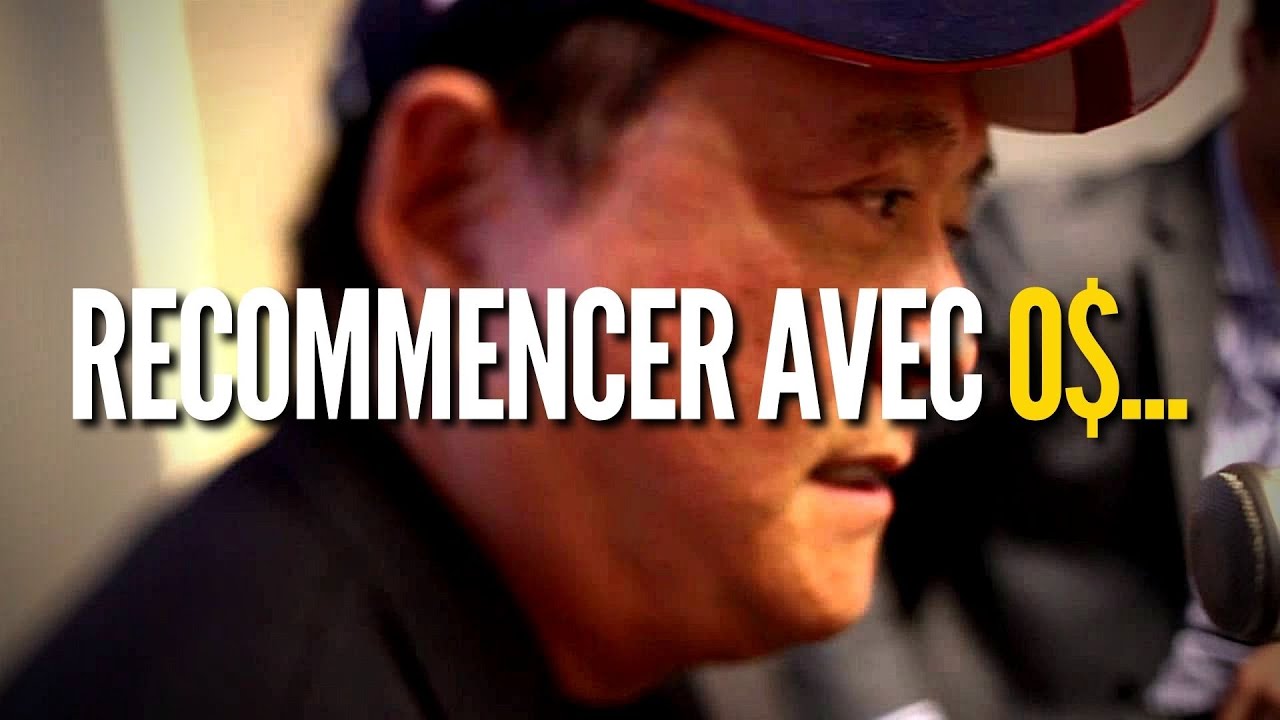
Si je devais RECOMMENCER À ZERO💲, voici MON PLAN PRÉCIS POUR DEVENIR RICHE - Robert KIYOSAKI

The Real Cost of Game Characters (And What to Charge)
5.0 / 5 (0 votes)