What is Edge Computing for Data & AI, and Should You Be Interested?
Summary
TLDRBen Dunmeyer, a data analytics consultant at Thorogood, discusses the impact of Edge Computing on data and AI. He explains the concept of processing data near its source to reduce latency, enhancing real-time decision-making. Dunmeyer explores use cases like retail inventory tracking, workplace safety, and healthcare monitoring. He also delves into the practical challenges of implementing Edge Computing, such as hardware requirements, security, and aligning with existing data strategies. The talk concludes with thoughts on the evolving landscape of providers and the importance of integrating Edge Computing into long-term data strategies.
Takeaways
- 🌐 Edge Computing brings computation and storage capabilities closer to where data is generated, reducing reliance on central data hubs.
- 📈 It enables low latency or real-time data processing and analysis, which is crucial for applications requiring instantaneous decision-making.
- 🏭 The technology is particularly impactful in industries like manufacturing, healthcare, and retail, where real-time data can optimize operations and enhance safety.
- 🔧 Efficient use of physical assets is achieved by utilizing edge devices to perform computations and analyses during idle times, maximizing ROI on infrastructure.
- 🆕 The ability to combine new and diverse datasets collected through edge devices can lead to novel insights and improved decision-making across various sectors.
- 🛠️ Edge Computing allows for the filtering and prioritization of information, reducing the need to transfer all data back to a central hub and thus optimizing network usage.
- 🌟 It can operate effectively in environments with limited or unreliable network connectivity, making it suitable for remote locations or situations where network infrastructure is a constraint.
- 🛡️ Security and data sovereignty become critical considerations as sensitive data and intellectual property may be stored and processed on edge devices.
- 🔄 The technology's potential is heavily influenced by the capabilities of cloud platforms, which provide the infrastructure and tools necessary for edge computing implementations.
- 🔧 Successful integration of edge computing requires a strategic approach that considers technical capabilities, business objectives, and the physical realities of deploying and maintaining edge devices.
Q & A
What is the main focus of the discussion in the transcript?
-The main focus of the discussion is Edge Computing and its implications on data and AI, particularly looking at its potential impact on the data and AI landscape.
What is Edge Computing?
-Edge Computing refers to the ability to have both compute and storage within devices or where data is being generated, as opposed to relying solely on a central data hub or infrastructure.
What is the significance of low latency in Edge Computing?
-Low latency in Edge Computing is significant because it allows for real-time or near real-time data processing and analysis, which is crucial for applications that require instantaneous decision-making.
How does Edge Computing relate to AI applications?
-Edge Computing can enable AI applications by providing the necessary computation and storage capabilities close to where data is generated, allowing for faster and more efficient AI model training and inference.
What is the role of Thorogood in the context of this discussion?
-Thorogood is an independent Global Professional Services firm specializing in business intelligence and analytics strategies, solutions, and services. They offer a full range of data analytics services and work with various technologies to provide clients with solutions that best suit their needs.
What are some potential use cases for Edge Computing mentioned in the transcript?
-Some potential use cases for Edge Computing mentioned include real-time decision making, efficient usage of physical assets, combining new and diverse data sets, filtering information, and reducing dependency on environmental or physical limitations.
How does Edge Computing impact traditional business intelligence reporting?
-Edge Computing can impact traditional business intelligence reporting by allowing for real-time or near real-time analytics, which can provide more up-to-date insights and potentially lead to better decision-making.
What are the challenges associated with implementing Edge Computing?
-Challenges associated with implementing Edge Computing include physical practicalities such as procuring, installing, and maintaining the equipment, ensuring connectivity, addressing security concerns, and considering data sovereignty.
How does Edge Computing fit into an organization's overall data and AI strategy?
-Edge Computing should be considered as a facet of an organization's overall data and AI strategy, where it can be opportunistic for specific use cases or part of a wider rollout. It's important to align it with business and technical strategies and consider how it will work with existing systems.
What are some of the core capabilities needed to operate and manage Edge Computing?
-Core capabilities needed to operate and manage Edge Computing include operating and running systems, data systems, devops, data engineering, data science, machine learning, data collection, monitoring, and potentially mlops (machine learning operations).
How might the landscape of providers for Edge Computing look like in the future?
-The landscape of providers for Edge Computing may include the mainstays of the data and AI space, new players, conglomerates, or partnerships. It will be interesting to see how the hardware requirements and market potential shape the competition.
Outlines
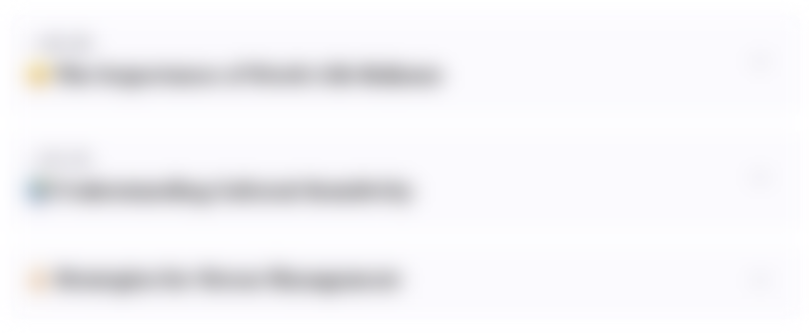
Dieser Bereich ist nur für Premium-Benutzer verfügbar. Bitte führen Sie ein Upgrade durch, um auf diesen Abschnitt zuzugreifen.
Upgrade durchführenMindmap
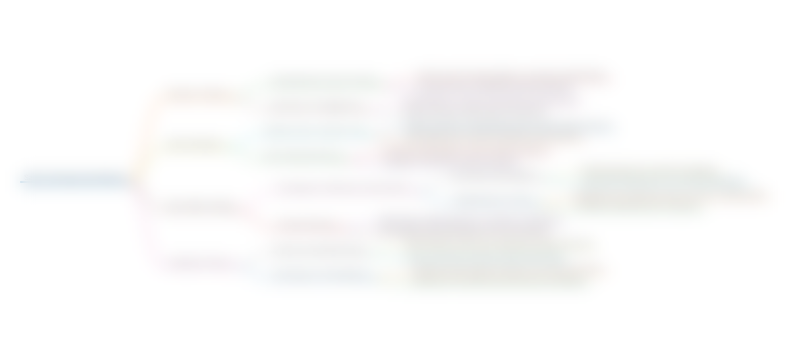
Dieser Bereich ist nur für Premium-Benutzer verfügbar. Bitte führen Sie ein Upgrade durch, um auf diesen Abschnitt zuzugreifen.
Upgrade durchführenKeywords
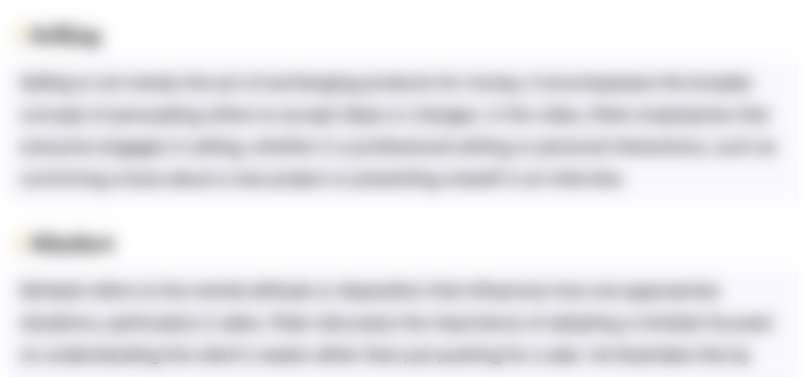
Dieser Bereich ist nur für Premium-Benutzer verfügbar. Bitte führen Sie ein Upgrade durch, um auf diesen Abschnitt zuzugreifen.
Upgrade durchführenHighlights
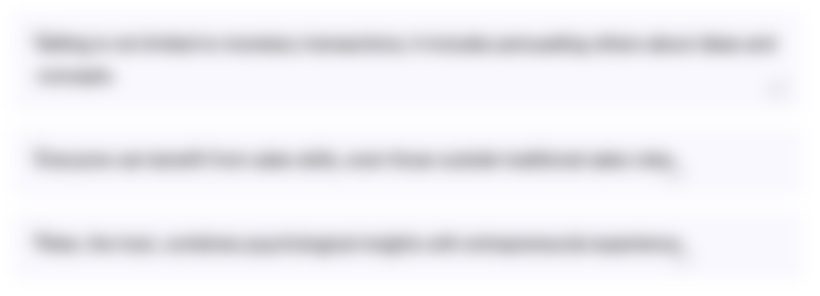
Dieser Bereich ist nur für Premium-Benutzer verfügbar. Bitte führen Sie ein Upgrade durch, um auf diesen Abschnitt zuzugreifen.
Upgrade durchführenTranscripts
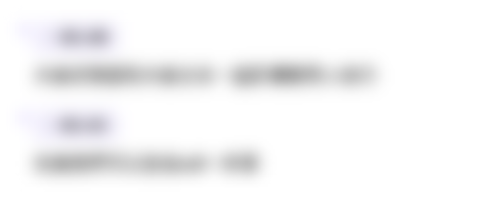
Dieser Bereich ist nur für Premium-Benutzer verfügbar. Bitte führen Sie ein Upgrade durch, um auf diesen Abschnitt zuzugreifen.
Upgrade durchführen5.0 / 5 (0 votes)