New STUNNING Research Reveals AI In 2030...
Summary
TLDREpoch AI's report predicts significant advancements in AI, suggesting models like GPT-5 could generate over $2 billion in revenue within a year of release. The report highlights the potential for AI to automate a substantial portion of the $60 trillion global economy, with companies investing trillions in AI development. It also discusses the possibility of training runs increasing by 5,000 times by 2030, the exploration of synthetic data to overcome data scarcity, and the race for gigawatt-scale data centers. The video emphasizes the conservative yet impressive estimates for AI's future, indicating an 'incredible time' ahead in the field.
Takeaways
- 🔮 Epoch AI's report suggests that AI advancements could lead to models like GPT 5 generating over $2 billion in revenue within their first year of release.
- 🌟 The potential economic impact of AI is vast, with the possibility of automating a small portion of the $60 trillion annual economic output.
- 🚀 AI models are expected to develop agentic capabilities, allowing them to operate more independently and integrate seamlessly into existing workflows.
- 📈 The report forecasts a significant scale-up in AI models, with future models by 2030 potentially being 20,000 times more capable than current ones.
- 💹 There's a growing belief that investing trillions of dollars in AI development could be economically justified due to the enormous potential payoffs.
- 💼 Wall Street's skepticism about AI's profitability is contrasted with the long-term vision of AI's capability to revolutionize industries and generate substantial revenue.
- 💡 The report highlights the importance of AI's ability to automate tasks, with predictions that 100% of tasks could be automated by 2043.
- 🌐 Companies like Meta and Amazon are investing heavily in power and data centers, indicating a commitment to the future of AI and the infrastructure needed to support it.
- 🔋 The future of AI training involves larger models, with projections of training runs by 2030 being 5,000 times larger than those of llama 3.1, although power demand may not scale as much.
- 📊 The report discusses the potential for synthetic data to address data scarcity issues, with reinforcement learning being a method to improve the quality of AI-generated data.
Q & A
What is the main focus of Epoch AI's research initiative?
-Epoch AI's research initiative is focused on investigating trends in machine learning and forecasting the development of artificial intelligence.
What does the report by Epoch AI predict about the future of AI?
-The report predicts significant advancements in AI functionality, with newer models like GPT 5 potentially generating over $2 billion in revenue within their first year of release.
How does the report suggest AI models will integrate into existing workflows?
-The report suggests that AI models will seamlessly integrate into existing workflows, manipulate browser windows or virtual machines, and operate independently in the background.
What is the potential economic impact of AI models according to the report?
-The report suggests that if an AI model can automate a small portion of the $60 trillion economic output, generating $20 billion in economic value is plausible.
What does the report say about the scale-up from GPT 4 to GPT 6 models?
-The report indicates that GPT 4 to GPT 6 could represent a 10,000 times scale-up, and future models by 2030 could potentially be a 20,000 times scale-up.
How does the report address the concerns of Wall Street regarding AI investments?
-The report justifies the massive investments in AI by highlighting the potential for unprecedented economic growth and the possibility of capturing a fraction of the $60 trillion global labor compensation.
What is the significance of the 10x output prediction mentioned in the report?
-The 10x output prediction signifies that if AI automation replaces almost all human labor, economic growth could accelerate by tenfold or more over a few decades, increasing economic output significantly.
How does the report envision the future of training runs for AI models?
-The report envisions training runs for AI models to be longer, potentially lasting up to six months, and to be 5,000 times larger than those of llama 3.1 by 2030.
What are the potential constraints on AI development according to the report?
-The report identifies power and chip availability as the most binding constraints on AI development, with data scarcity being the most uncertain bottleneck.
How does the report address the issue of synthetic data and model collapse?
-The report discusses the use of reinforcement to improve the quality of AI-generated data, which can prevent model collapse and even lead to perfect performance in some cases.
Outlines
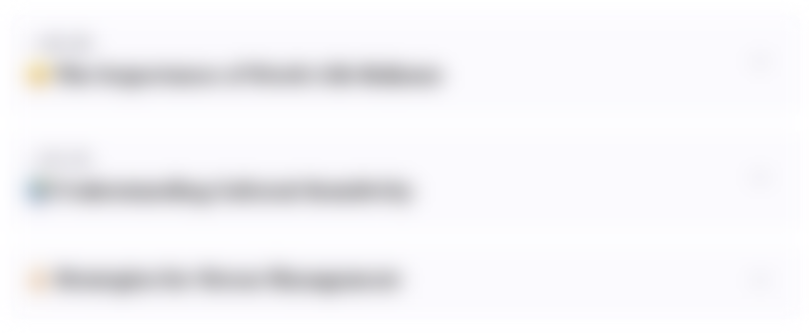
Dieser Bereich ist nur für Premium-Benutzer verfügbar. Bitte führen Sie ein Upgrade durch, um auf diesen Abschnitt zuzugreifen.
Upgrade durchführenMindmap
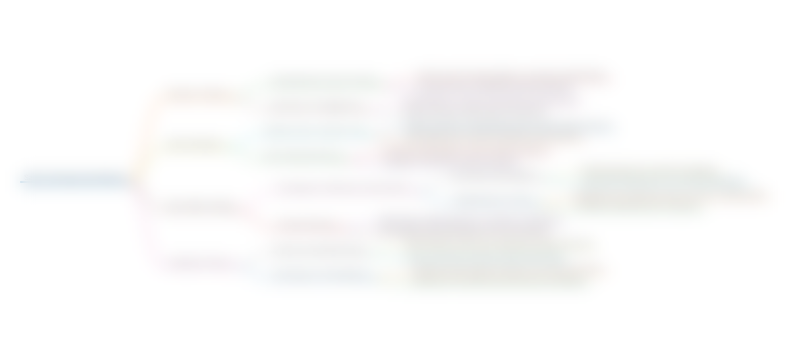
Dieser Bereich ist nur für Premium-Benutzer verfügbar. Bitte führen Sie ein Upgrade durch, um auf diesen Abschnitt zuzugreifen.
Upgrade durchführenKeywords
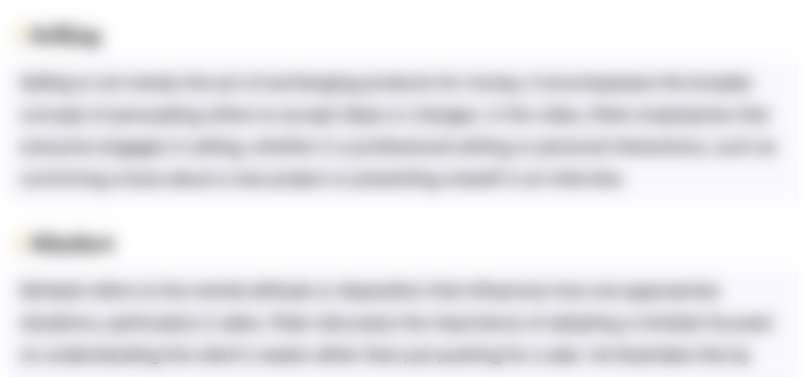
Dieser Bereich ist nur für Premium-Benutzer verfügbar. Bitte führen Sie ein Upgrade durch, um auf diesen Abschnitt zuzugreifen.
Upgrade durchführenHighlights
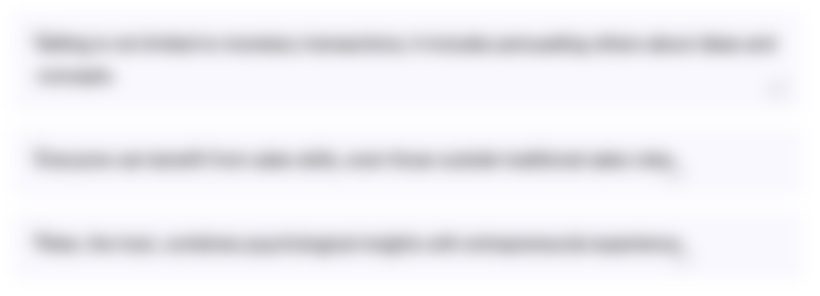
Dieser Bereich ist nur für Premium-Benutzer verfügbar. Bitte führen Sie ein Upgrade durch, um auf diesen Abschnitt zuzugreifen.
Upgrade durchführenTranscripts
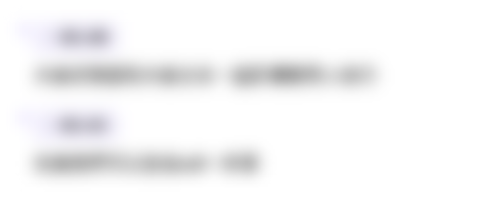
Dieser Bereich ist nur für Premium-Benutzer verfügbar. Bitte führen Sie ein Upgrade durch, um auf diesen Abschnitt zuzugreifen.
Upgrade durchführenWeitere ähnliche Videos ansehen
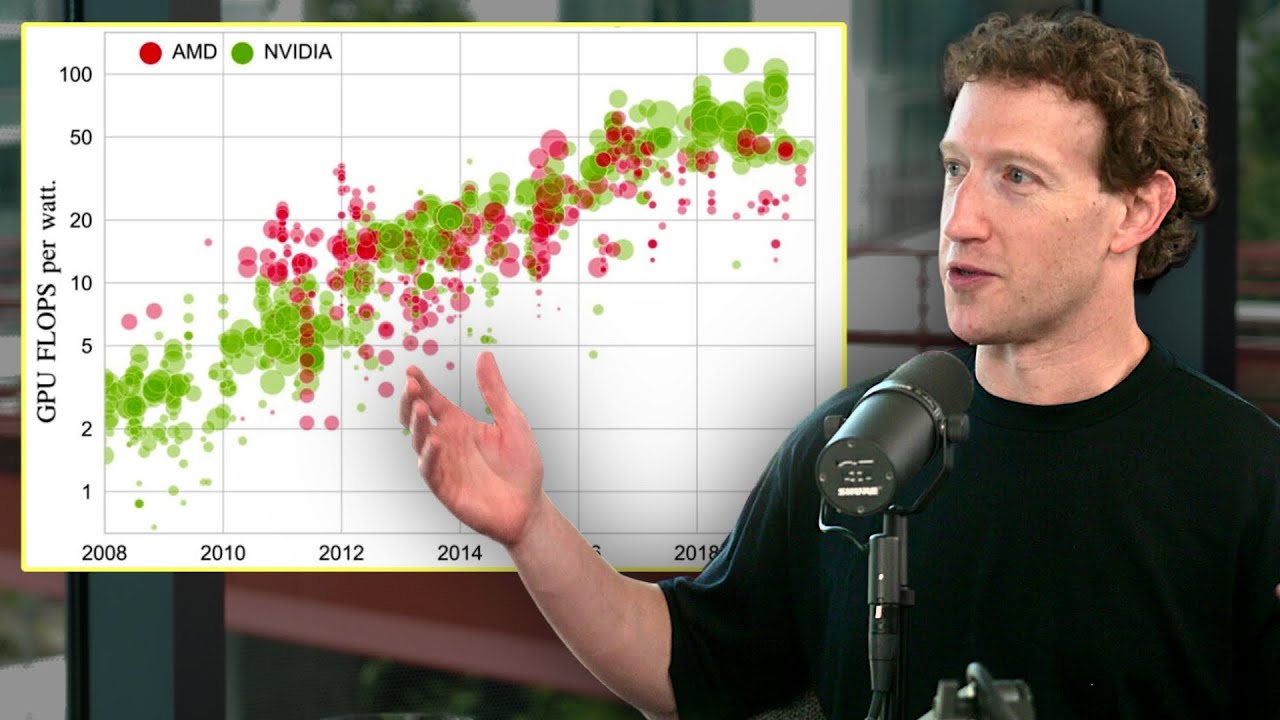
Energy, not compute, will be the #1 bottleneck to AI progress – Mark Zuckerberg

Every AI Existential Risk Explained
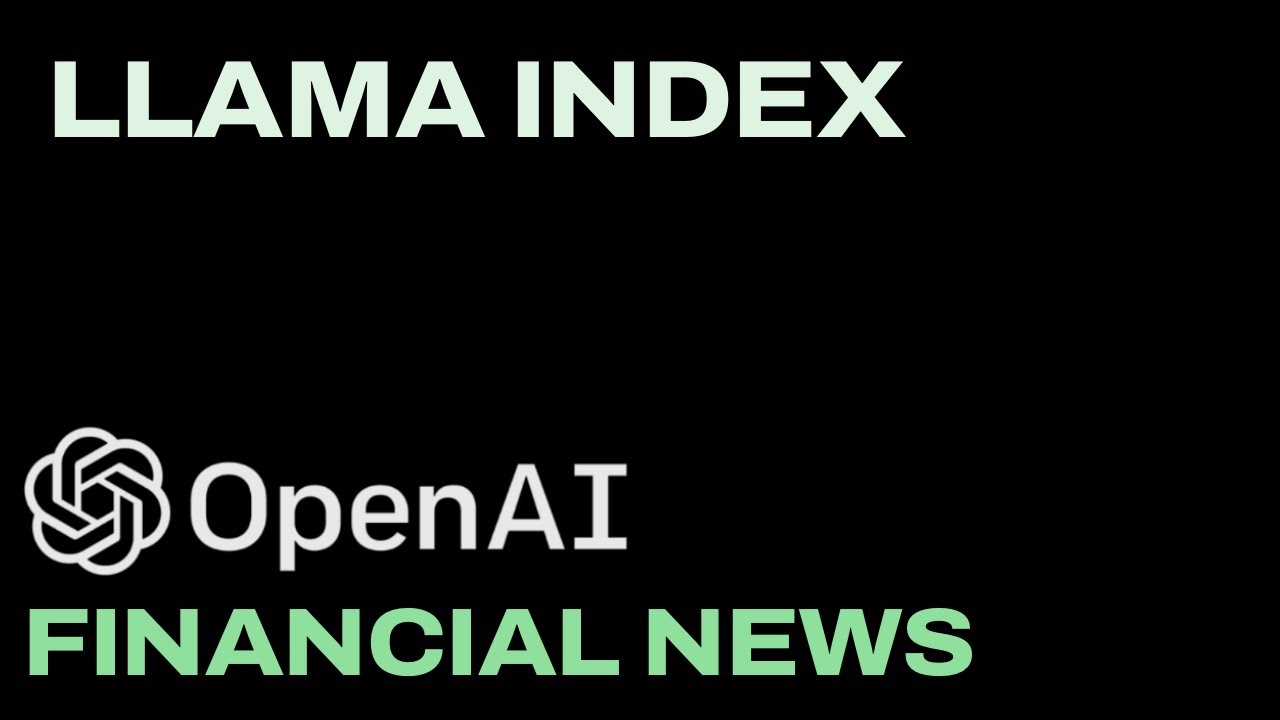
11. OpenAI and Llama Index - Financial News Analysis
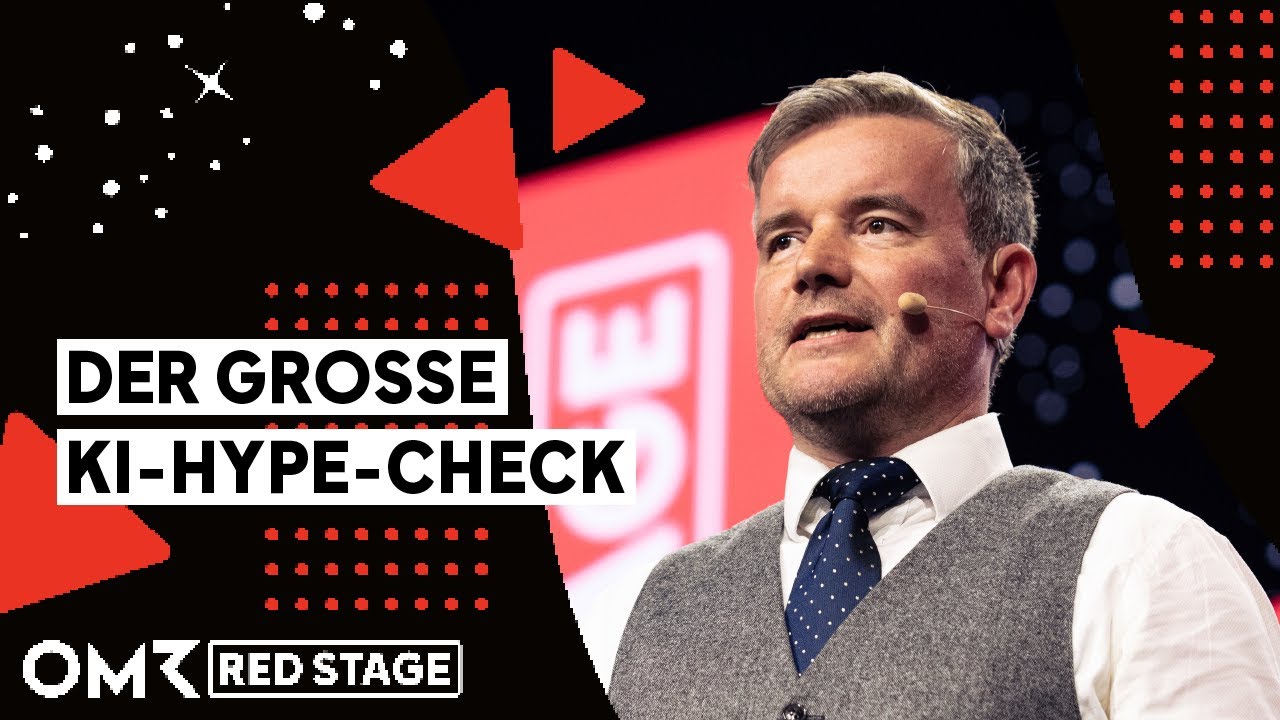
Wo wir in Sachen KI wirklich stehen und was uns erwartet: Deep Dive mit Philipp "Pip" Klöckner
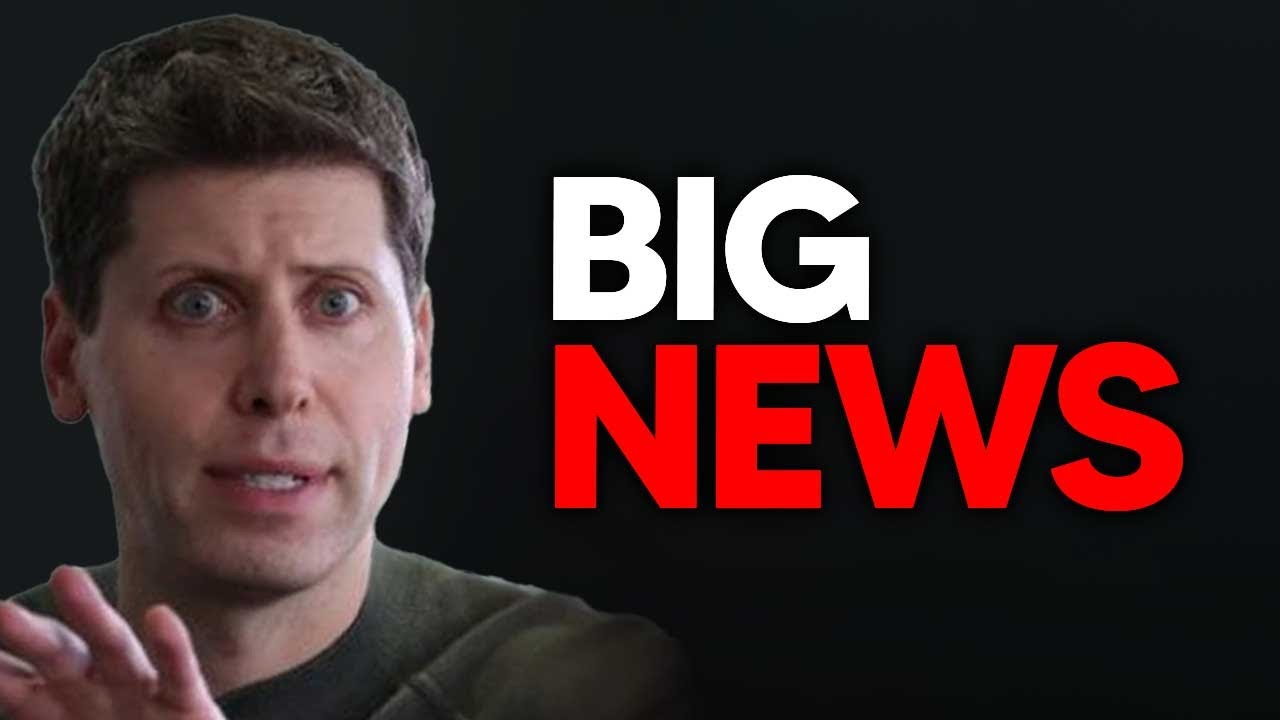
BIG AI NEWS: 10,000X Bigger Than GPT-4, AGI 2025, New Boston Dynamics Demo And More
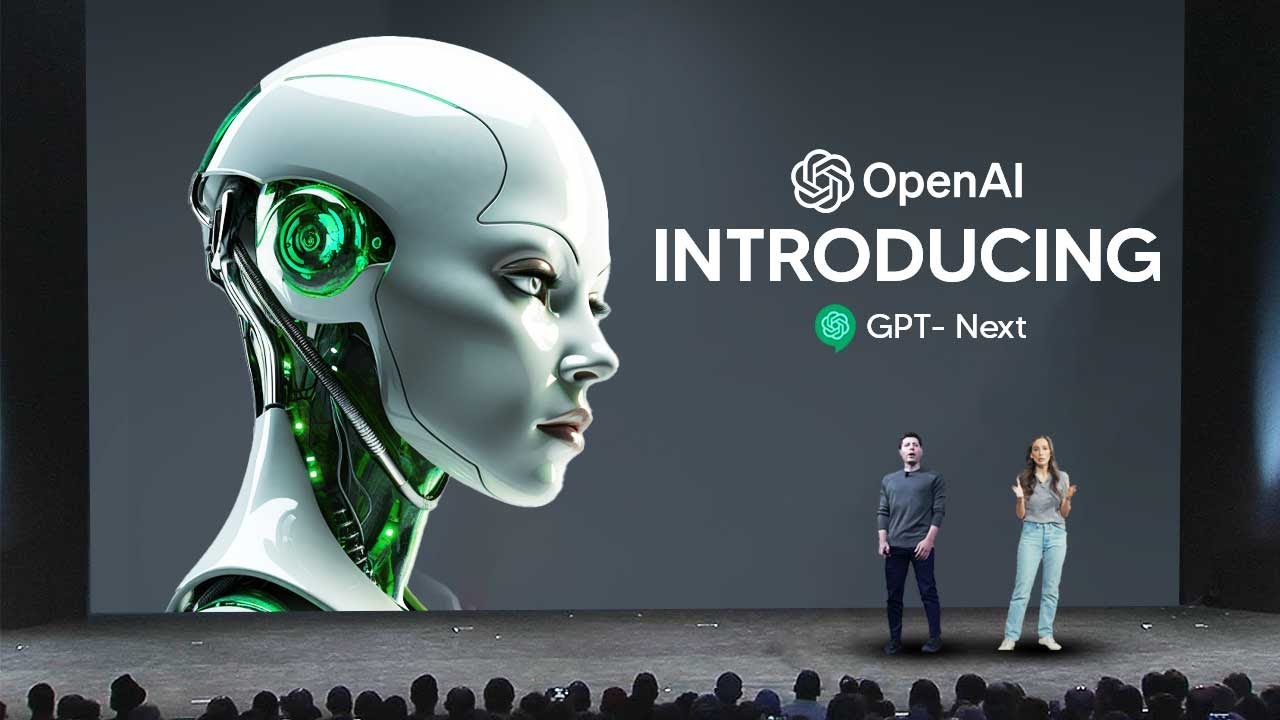
OpenAI'S "SECRET MODEL" Just LEAKED! (GPT-5 Release Date, Agents And More)
5.0 / 5 (0 votes)