How I Made AI Assistants Do My Work For Me: CrewAI
Summary
TLDRIn this insightful video, the presenter explores the limitations of current AI language models, highlighting their reliance on fast, automatic thinking. By introducing innovative methods like 'tree of thought' prompting and custom AI agents, viewers learn how to enhance AI's problem-solving capabilities. The video also demonstrates practical applications, including creating a startup concept and generating content from real-time data sources like Reddit. Ultimately, it showcases the potential of AI in automating tasks while discussing the challenges and costs associated with using advanced models, encouraging experimentation and local model deployment.
Takeaways
- 😀 System 1 thinking is fast and automatic, while System 2 thinking is slow and rational, as described by Daniel Kahneman in 'Thinking, Fast and Slow.'
- 🤖 Current large language models (LLMs) operate primarily on System 1 thinking, lacking deep, rational processing capabilities.
- 🧠 Tree of Thought prompting allows LLMs to consider multiple perspectives for decision-making, simulating rational thinking.
- 🛠️ Custom agent systems like CreAI enable users to build AI agents that collaborate to solve complex problems.
- 👥 The tutorial illustrates how to set up a team of AI agents with distinct roles: a marketer, a technologist, and a business development expert.
- 📊 Each AI agent is assigned specific tasks, and the output from one agent serves as the input for the next, forming a sequential process.
- 🔍 Enhancing agents' intelligence involves integrating real-time data through built-in tools and custom scraping tools like a Reddit scraper.
- 📈 The quality of output can vary significantly based on the sources of information used by the agents.
- ⚙️ Local AI models are explored as alternatives to API calls, with varying levels of performance observed among different models.
- 💬 Viewers are encouraged to share their experiences with CreAI and other AI tools, fostering a community of knowledge sharing.
Q & A
What is the main focus of the video?
-The video discusses the limitations of current large language models (LLMs) in performing complex problem-solving, and introduces methods to enhance their capabilities by creating teams of AI agents.
What are the two types of thinking described in the video?
-The video describes two types of thinking: System 1, which is fast and automatic, and System 2, which is slow and requires conscious effort. Current LLMs primarily operate using System 1 thinking.
What is 'Tree of Thought' prompting?
-'Tree of Thought' prompting is a method that forces LLMs to consider an issue from multiple perspectives or experts before arriving at a conclusion, simulating System 2 thinking.
How does CreAI allow users to build custom AI agents?
-CreAI enables users, even non-programmers, to create custom agents that can collaborate on complex tasks by utilizing APIs or local models, making the process accessible and flexible.
What are the roles of the three AI agents in the startup example?
-In the startup example, the three AI agents are assigned the roles of a market researcher expert, a technologist, and a business development expert, each with specific tasks to contribute to the business plan.
What was the outcome of the business plan created by the AI agents?
-The business plan included ten bullet points, five business goals, a time schedule, and suggestions for using technologies like 3D printing, machine learning, and sustainable materials.
What tools can be added to make AI agents smarter?
-Users can enhance the intelligence of AI agents by integrating built-in tools that provide access to real-time data, such as text-to-speech capabilities, Google search results, and various APIs.
How does the video suggest improving the quality of information generated by AI agents?
-To improve the quality of information, the video suggests using better sources, such as local subreddits, and creating custom tools for scraping relevant data, thus ensuring the agents produce more accurate and relevant outputs.
What were some limitations of the local models tested?
-The local models faced challenges in understanding tasks effectively, often producing generic outputs and failing to grasp specific instructions, indicating variability in performance among different models.
What was the overall conclusion regarding the use of local models versus API models?
-The conclusion highlighted that while local models can avoid API costs and maintain privacy, they often struggle with task comprehension, and performance varies significantly across different models.
Outlines
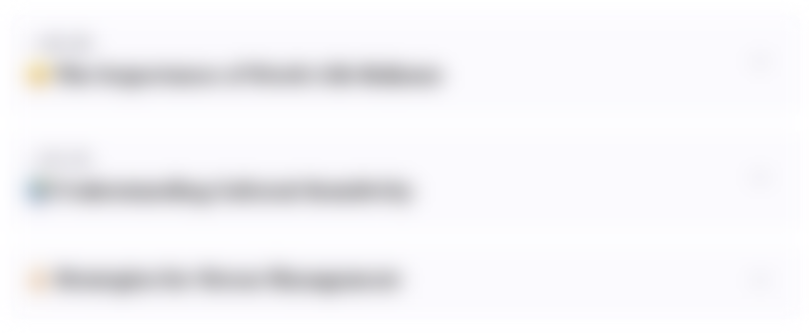
هذا القسم متوفر فقط للمشتركين. يرجى الترقية للوصول إلى هذه الميزة.
قم بالترقية الآنMindmap
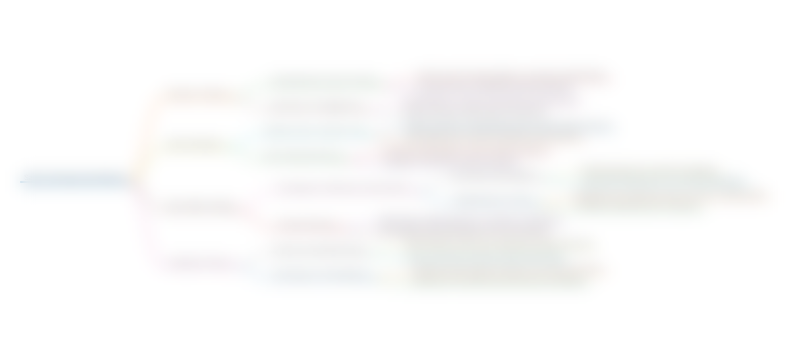
هذا القسم متوفر فقط للمشتركين. يرجى الترقية للوصول إلى هذه الميزة.
قم بالترقية الآنKeywords
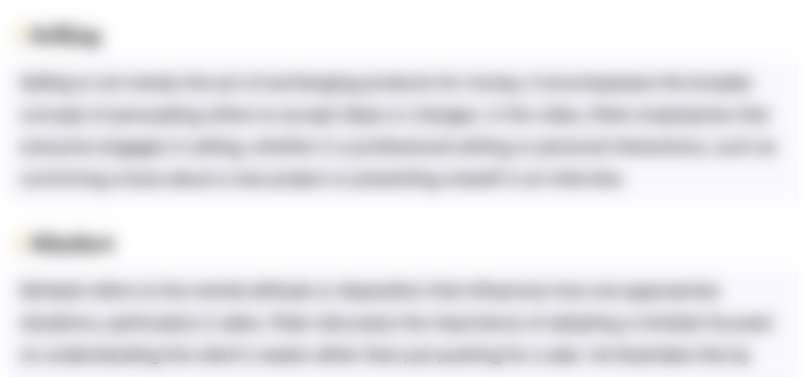
هذا القسم متوفر فقط للمشتركين. يرجى الترقية للوصول إلى هذه الميزة.
قم بالترقية الآنHighlights
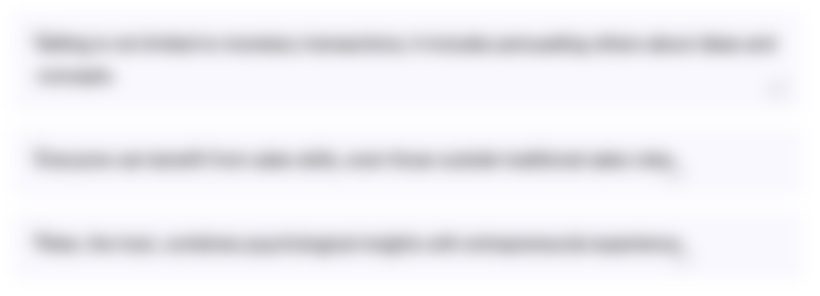
هذا القسم متوفر فقط للمشتركين. يرجى الترقية للوصول إلى هذه الميزة.
قم بالترقية الآنTranscripts
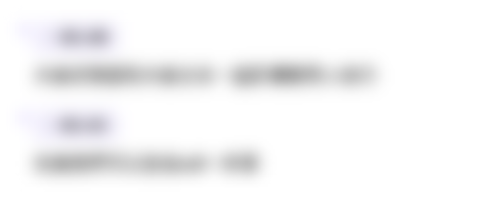
هذا القسم متوفر فقط للمشتركين. يرجى الترقية للوصول إلى هذه الميزة.
قم بالترقية الآنتصفح المزيد من مقاطع الفيديو ذات الصلة
5.0 / 5 (0 votes)