Genome-Wide Association Study - An Explanation for Beginners
Summary
TLDRIn this video, Nuna Crevallo explains the concept of a Genome-Wide Association Study (GWAS). She describes how GWAS identifies associations between genetic variations, known as single nucleotide polymorphisms (SNPs), and specific traits like BMI. Crevallo outlines the process, which involves large-scale data collection, genotyping, regression analysis, and the use of statistical methods to determine significant genetic associations. She also explains the importance of correcting for false positives and how the findings can lead to better understanding of genetic diseases and traits, potentially improving treatments and prevention.
Takeaways
- 🧬 Genome-wide association studies (GWAS) investigate associations between genetic variations and specific physical traits.
- 🧪 Single nucleotide polymorphisms (SNPs) are key genetic variations, where different nucleotides occur at the same position in the DNA.
- 👥 Humans share 99.9% of their genome, but the 0.1% of SNPs contribute to individual differences.
- 🌐 GWAS requires large sample sizes, typically from thousands to hundreds of thousands of people, to detect genetic associations.
- 🔄 The study focuses on a specific trait or phenotype, such as BMI, and analyzes genetic data across millions of SNPs.
- 📊 A regression analysis is performed to see if the number of SNP variants (alleles) is linked to the physical trait being studied.
- 📉 The strength of association is measured by p-values, which indicate the likelihood that an association is due to chance.
- 🔢 A Bonferroni correction is applied to adjust for false positives when testing millions of SNPs, using a stricter significance threshold.
- 🏙 A Manhattan plot visually represents significant associations, with SNPs positioned along chromosomes and their p-values shown.
- 🔍 Identifying SNPs associated with traits can lead to discoveries of important biological mechanisms and improve disease treatment.
Q & A
What is a Genome-Wide Association Study (GWAS)?
-A Genome-Wide Association Study (GWAS) is a method used to discover associations between variations in our genetic code and certain physical traits.
What are single nucleotide polymorphisms (SNPs)?
-SNPs are variations at a single position in a DNA sequence among individuals. They are a key element in understanding the genetic causes for human traits.
Why is it important to understand the genetic makeup of humans?
-Understanding the genetic makeup is important to determine the extent to which certain traits are genetic and to identify biological mechanisms that affect those traits.
How does a GWAS help in understanding traits like BMI?
-A GWAS helps in understanding traits like BMI by looking for variance in DNA that might impact it, such as genes that affect metabolism.
What is the significance of having a large sample size in a GWAS?
-A large sample size, ideally in the thousands or hundreds of thousands, is significant because it helps to minimize confounding genetic variation and increases the statistical power of the study.
What does genotyping involve in the context of a GWAS?
-In a GWAS, genotyping involves recording the nucleotides of each person at many known SNP locations, often over 2 million SNPs.
How is the association between genetic data and a phenotype computed?
-The association between genetic data and a phenotype is computed using a genome-wide association analysis program, which performs regression analysis for every SNP in the dataset.
What role does the software PLINK play in GWAS?
-PLINK is a commonly used software for GWAS that performs quality control filters on the genetic dataset and carries out the actual association analysis.
What is a p-value in the context of GWAS, and why is it important?
-A p-value measures the likelihood that the association found in the distribution of data points was due to random chance. It is important for determining the statistical significance of the association between a SNP and a phenotype.
What is a Bonferroni correction, and why is it used in GWAS?
-A Bonferroni correction is a statistical method used to adjust the threshold for significance when dealing with multiple comparisons, like in GWAS, to reduce the chance of false positives.
How are the results of a GWAS typically visualized?
-The results of a GWAS are typically visualized using a Manhattan plot, which plots each SNP's position along the chromosome and its associated p-value after a negative log transformation.
What is linkage disequilibrium, and how does it relate to GWAS?
-Linkage disequilibrium refers to the non-random association of alleles at different loci. In GWAS, significant SNPs often cluster together due to linkage disequilibrium, which can indicate the presence of important genes.
Outlines
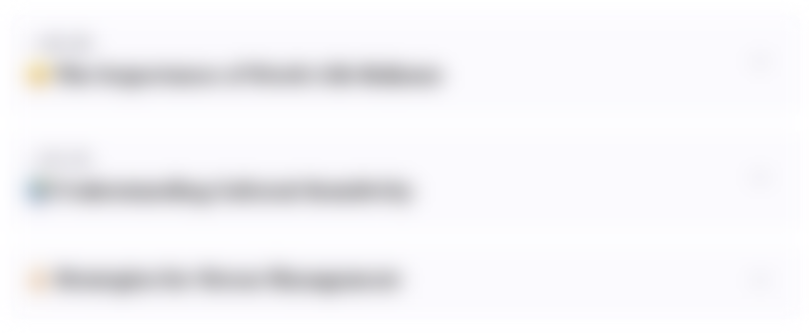
هذا القسم متوفر فقط للمشتركين. يرجى الترقية للوصول إلى هذه الميزة.
قم بالترقية الآنMindmap
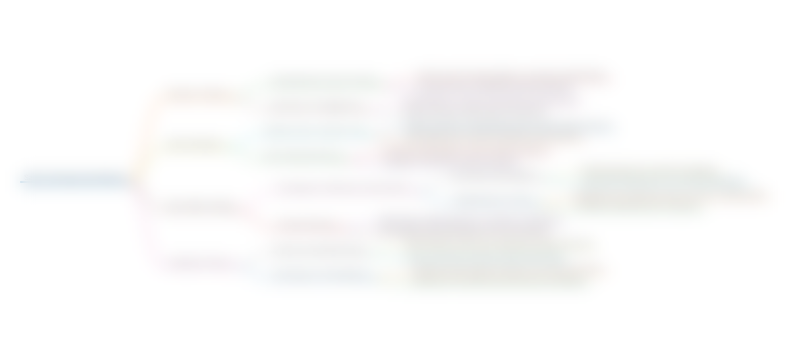
هذا القسم متوفر فقط للمشتركين. يرجى الترقية للوصول إلى هذه الميزة.
قم بالترقية الآنKeywords
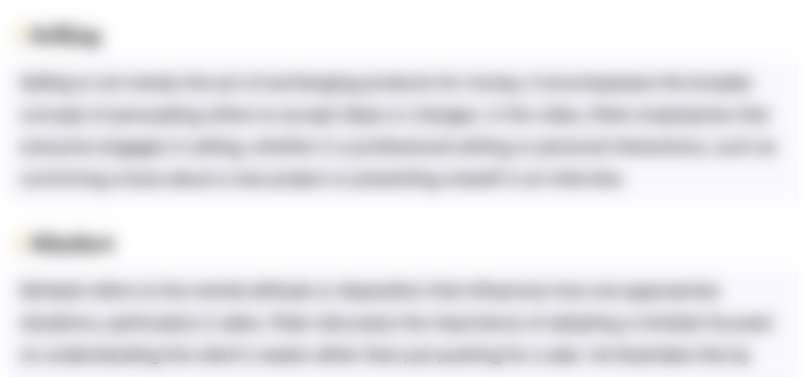
هذا القسم متوفر فقط للمشتركين. يرجى الترقية للوصول إلى هذه الميزة.
قم بالترقية الآنHighlights
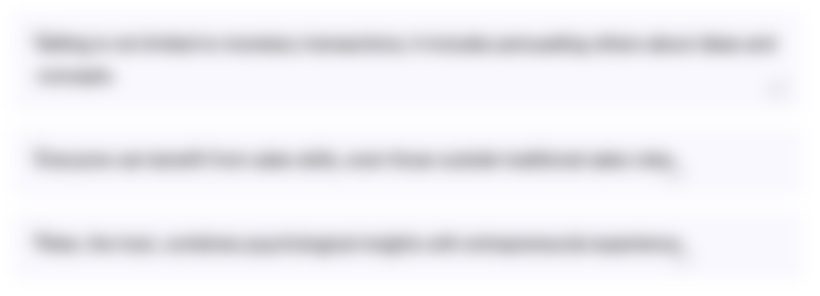
هذا القسم متوفر فقط للمشتركين. يرجى الترقية للوصول إلى هذه الميزة.
قم بالترقية الآنTranscripts
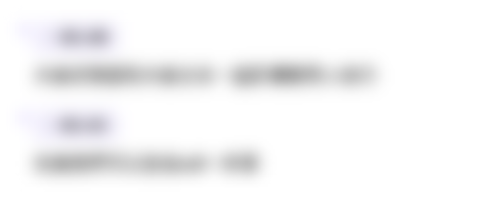
هذا القسم متوفر فقط للمشتركين. يرجى الترقية للوصول إلى هذه الميزة.
قم بالترقية الآنتصفح المزيد من مقاطع الفيديو ذات الصلة
5.0 / 5 (0 votes)