MRI Physics Fourier Transform for MRI Kucharczyk
Summary
TLDRThis lecture focuses on MRI data processing and display, supplementing a previous talk on MRI physics. It explains how the Fourier transform is used to analyze the complex waveforms received by the MRI receiver coil, breaking them down into simpler sine waves of distinct frequencies and amplitudes. The presentation visually demonstrates how these waveforms can be represented in both the temporal and spatial domains, ultimately simplifying the data into a two-dimensional or three-dimensional k-space. This method allows for a comprehensive display of the MRI data, enabling a detailed understanding of the object scanned.
Takeaways
- 🧠 This talk serves as a supplement to a lecture on MRI physics, focusing on the processing and display of MRI data.
- 🔄 The Fourier transform is the primary mathematical tool used for processing MRI data, which involves complex waveforms of multiple frequencies and amplitudes.
- 📡 The MR receiver coil is responsible for receiving signals from the object being scanned, which are then processed.
- 🌊 The raw data from the coil is a complicated waveform that can be simplified using the Fourier transform into discrete frequencies and amplitudes.
- 📊 The presentation uses a simplified pictorial demonstration to explain how the Fourier transform processes and displays MRI data.
- 🌀 By adding waves of different frequencies and amplitudes, a complex waveform is created, which can be decomposed into simpler components using the Fourier transform.
- 📈 The data can be displayed in various ways, including as an amplitude versus frequency graph, making it easier to understand the composition of the waveform.
- 🔵 The amplitude and frequency of the signal can be represented as dots on a graph, which simplifies the visualization of complex data.
- 📐 The concept of the Fourier transform can be applied to both temporal (time-based) and spatial (distance-based) domains.
- 📊 The final display of MRI data is often in the form of a graph where each dot represents a frequency and amplitude at a specific spatial frequency, known as k-space.
- 🔗 The Fourier transform allows for the transformation of complex data into a sum of simple sine waves, which is essential for understanding the data obtained from MRI scans.
Q & A
What is the main purpose of the supplement to the lecture on MRI physics?
-The supplement is created to address recurrent questions that have come up during the original lecture, providing a more detailed discussion and a recorded video for individual use at their convenience.
What is the role of the MR receiver coil in the MRI system?
-The MR receiver coil is responsible for receiving the signal from the object being scanned. It captures an induced voltage consisting of multiple frequencies and amplitudes.
What mathematical tool is commonly used to process the raw data received by the MR receiver coil?
-The Fourier transform is the mathematical tool most commonly used for processing the raw data received by the MR receiver coil.
How does the Fourier transform help in processing MRI data?
-The Fourier transform allows for the decomposition of a complicated waveform, which consists of multiple frequencies and different amplitudes, into a sum of simple sine waves of discrete frequencies.
What is the significance of the amplitude and frequency in the context of MRI data?
-In MRI data, the amplitude represents the strength of the signal at a particular frequency, while the frequency indicates the number of times a signal repeats over a period of time or distance.
How is the complex waveform resulting from the addition of multiple sine waves typically displayed?
-The complex waveform resulting from the addition of multiple sine waves can be displayed as an amplitude versus frequency graph, where amplitude is represented by the height of blue columns on the frequency axis.
What is the difference between the temporal domain and the spatial domain in MRI data?
-The temporal domain refers to variations over a unit of time, while the spatial domain refers to variations over a unit of distance. The Fourier transform can be applied to both domains to analyze the data.
How is spatial information that varies in two dimensions represented in MRI data?
-Spatial information that varies in two dimensions is represented by considering both the x and y directions, with each having a specific frequency and amplitude, which can be displayed as dots in a two-dimensional Fourier space or k-space.
What is the term used for the pictorial representation of numerical values of frequencies and their amplitudes obtained from the MRI scanner?
-The pictorial representation of numerical values of frequencies and their amplitudes obtained from the MRI scanner is referred to as two-dimensional Fourier space or simply two-dimensional k-space.
How can the Fourier transform be extrapolated to three dimensions in MRI data?
-The Fourier transform can be extrapolated to three dimensions by collecting frequency data in the z direction, resulting in 3D Fourier space or 3D k-space, with the third dimension represented by kz in addition to kx and ky.
What is the typical representation of MRI data after applying the Fourier transform?
-The typical representation of MRI data after applying the Fourier transform is a graph where the intensity of each dot represents the amplitude of each spatial frequency at a particular point, and the position of each dot represents the spatial frequency in each direction.
Outlines
🧠 MRI Data Processing and Display
This paragraph introduces the purpose of the video script, which is to supplement a lecture on MRI physics by addressing recurrent questions in greater detail. It explains the role of the MRI receiver coil in capturing signals from the object being scanned. The raw data received is a complex waveform consisting of multiple frequencies and amplitudes. The Fourier Transform is highlighted as the key mathematical tool used in MRI imaging to process this information. The script uses a simplified pictorial demonstration to explain how the Fourier Transform is applied to process and display MRI data. It starts with a basic sine wave example, showing how different waves with varying frequencies and amplitudes can be combined to form a complex waveform. The Fourier Transform allows this complex waveform to be decomposed into its constituent frequencies and amplitudes, which can be more easily analyzed and understood.
🌐 Fourier Transform in Spatial Domain
This paragraph delves into the application of the Fourier Transform in the spatial domain, contrasting it with the temporal domain. It uses an example of a line varying in whiteness over a unit of distance to illustrate how the Fourier Transform can be applied to analyze variations in functions over distance. The concept of frequency and amplitude is extended to the spatial domain, where the line's color variation is represented as a wave with a specific frequency and amplitude. The Fourier Transform is then used to simplify this data into a dot plot, where the position of the dot represents the frequency, and its brightness represents the amplitude. This method is extended to two-dimensional data, where variations in both the x and y directions are considered, leading to a more complex dot plot that represents the spatial frequencies and their amplitudes in both dimensions.
📊 Understanding MRI Data through K-Space
The final paragraph summarizes the concepts discussed in the script, focusing on the importance of frequency and the Fourier Transform in analyzing MRI data. It explains how the induced voltage in an MRI receiver coil is a complex waveform that can be decomposed into simple sine waves of discrete frequencies using the Fourier Transform. The conventional display of MRI data is described as a graph where the intensity of each dot represents the amplitude of each spatial frequency at a particular point in time, and the position of each dot represents the spatial frequency in each direction. The script introduces the concept of two-dimensional Fourier space or k-space, which is a pictorial representation of the numerical values of frequencies and their amplitudes obtained from the MRI scanner. The idea is extended to three dimensions, creating 3D Fourier space or 3D k-space. The paragraph concludes by emphasizing that a single graph can accurately display all the data obtained from every position in the object being scanned over the entire measurement time, providing a comprehensive view of the MRI data.
Mindmap
Keywords
💡MRI
💡Fourier Transform
💡Receiver Coil
💡Frequency
💡Amplitude
💡Waveform
💡Spatial Domain
💡Temporal Domain
💡k-space
💡Sine Wave
Highlights
The talk supplements a lecture on MRI physics, focusing on processing and display of MRI data.
The MR receiver coil receives signals from the object being scanned, inducing a complex voltage waveform.
The Fourier transform is the primary mathematical tool for processing MRI data, used for almost all MR imaging.
A simplified pictorial demonstration of the Fourier transform is presented to explain its role in MRI data processing.
The waveform's frequency and amplitude are key parameters described in the talk.
Adding waves of different frequencies and amplitudes results in a complex waveform.
The Fourier transform allows for the decomposition of a complex waveform into its constituent frequencies and amplitudes.
Data can be displayed as an amplitude versus frequency graph, simplifying the interpretation of complex waveforms.
The relationship between a complex waveform and its frequency components is illustrated through the Fourier transform.
The Fourier transform is also applicable to the spatial domain, analyzing variations over distance.
A line varying in whiteness over a unit of distance is used to demonstrate the spatial domain analysis.
The concept of frequency in the spatial domain is introduced, with the line having a frequency of one cycle per meter.
The Fourier transform can be applied to two-dimensional data, such as variations in both the x and y directions.
The talk explains how to represent two-dimensional spatial information using a combination of frequencies and amplitudes.
The concept of two-dimensional Fourier space or k-space is introduced to represent spatial frequencies.
The extension of the Fourier transform to three dimensions is discussed, creating 3D Fourier space or 3D k-space.
Frequency is defined as the number of repetitions over a period of time or distance.
The Fourier transform decomposes a complicated waveform into simple sine waves of discrete frequencies.
MRI data is typically displayed as a graph where the intensity of each dot represents the amplitude of a spatial frequency.
The position of each dot in the graph represents the spatial frequency in each direction.
A single graph can show all data obtained from every position in the object being scanned over the entire measurement time.
The typical representation of MRI data is a single picture, summarizing the entire scan's data.
Transcripts
this talk is on the processing
and display of mri data it is intended
to be a supplement
to a lecture that i have frequently
given with professor don plewis
on the basics of mri physics which we
call spin gymnastics
this supplement is created because of
questions that that have come to us on a
recurrent basis
on certain parts of the original
talk therefore
we felt it would be worthwhile to go
over
certain of these questions in greater
detail
and then to record the talk
as a video and have it available for
each individual's use
at the individual's convenience
this short presentation
will discuss how mri data
is processed and displayed
the mr receiver coil is the part of the
mri system
that receives signal from the object
being scanned
the data that is received by the coil is
an induced voltage in the coil
consisting of multiple frequencies
and amplitudes it has a very complicated
waveform in its raw state
the fourier transform is the
mathematical tool most commonly used for
processing this type
of information and is used for almost
all
mr imaging this presentation is a
simplified pictorial demonstration of
the fourier transform
and how the fourier transform is used to
process
and display mri data
let's look at a wave as it evolves
over time we'll start with something
very simple
we look at this sine wave it goes
through one
full cycle over one second
and we have assigned an arbitrary scale
to the vertical axis
we see it has an amplitude of
three units above the baseline so we
would describe
this wave as having a frequency of one
cycle per second an
amplitude of three units which we could
represent
in textual form as we see in the upper
left
or simply as a pair of numbers a one
in a three let's look at a couple
other waves of different frequencies and
different amplitudes
the next one has a frequency of three
cycles
over one second and it has an amplitude
of one
the next wave has a frequency of eight
cycles per second
and an amplitude of two units
if we then add all these waves together
over each fraction of a second
we would display it as the graph
on the bottom it looks like a pretty
complicated waveform
it would be difficult to tell what the
component frequencies and the component
amplitudes are of the frequencies that
contribute to this wave
because the this wave is composed of
multiple different frequencies in the
amplitude varies
another way of displaying the the same
data
is as follows if we look
in the upper row that same
data could be displayed as an amplitude
versus frequency graph where the
amplitude is displayed as a blue column
of three units tall
and placed on the frequency axis that
represents
one cycle per second similarly we could
do this
with the other graphs
as shown in the next row down
this is a frequency of three with an
amplitude
of one unit the next one
uh demonstrates that the frequency
is eight cycles per second and has an
amplitude
of two units and if we now add all
this data together it's a much simpler
way of
looking at the data we see that it's
composed of three discrete
frequencies each of different amplitudes
so if we're going the reverse direction
using
the waveforms in the leftmost column it
would be difficult to
disassemble the complicated waveform
into its individual components
amplitudes but if we look at the middle
column
it's a relatively simple task the
relationship between the leftmost column
and the middle column is the fourier
transform
and then we can take this one step
further
and if we look at the bottom row rather
than
displaying the amplitudes
as the height of blue columns
we could simply represent them as dots
on a single linear axis where the
either the size or the brightness of the
dot represents the amplitude at the
particular frequency
remembering of course that these graphs
are a pictorial representation of
certain numbers
so if we take a second look at the
bottom right
we see that that complicated waveform
consists
of frequencies of one cycle per second
with an amplitude of three
three cycles per second with an
amplitude of one
and eight cycles per second of
amplitude 2.
the same type of analysis
can be carried out for things
or functions that vary over a unit of
distance
rather than over a unit of time and this
is called the spatial domain
rather than the temporal domain
so in this example i have drawn
a relatively thick line across the
top left of the image that
varies in whiteness going from left to
right
it starts as a neutral gray it becomes
white then becomes gray then becomes
black
and becomes gray again so it's gone over
one full cycle in color variation over
a unit of distance of one meter
so in the spatial domain it would be
said that this line has a frequency of
one cycle per meter
and if we assigned numbers to the gray
scale
where gray is zero and white is three
we would say that the white part
of this line has an amplitude of three
above the baseline grayscale
and we could represent it as a wave
over a distance of one meter where the
amplitude of the wave varies from zero
representing gray
up to three representing white back to
zero representing gray
minus three representing black and back
to zero
representing gray
if we then did the same type of fourier
transform
on this line we could represent it the
same way
we could represent it as having an
amplitude of 3 units
in this case units of whiteness at a
frequency
of one cycle over a meter
and then we could collapse this data
down further
into a dot if we wish to so display the
information
as a single dot on the frequency
axis at the position of one cycle per
meter
and the brightness of the dot would have
an amplitude of three
so this works very well for something
that varies
in one dimension how about if it varies
in two dimensions
well if we try to represent that data
in the bottom left hand image that
square
that single white dot of amplitude 3
would only represent
the function
the variation in signal intensity
in the x direction and if it has no
variation in the white in the y
direction it would be adequate
but what if something also varied in the
y direction
let's look at the situation
if we have spatial information
that varies in two directions
on the bottom we have the example we
have previously seen
where we have spatial information that
varies over one
cycle per meter in the x direction and
we've represented it
as a single white dot of amplitude three
on the x frequency direction
equal to one cycle per meter
if we now have a second
a square where the variation
in signal intensity is in the vertical
or y direction
and we change the frequency to
three cycles of variation over
one meter of distance in that vertical
or y direction
we'd have we would say it has three
cycles per meter
in the y direction and we've changed the
amplitude
to have an amplitude of 2 rather than
amplitude of 3.
if we put these two things together we
would get an image like that
but we could decompose that and
represent it as a frequency
in the x direction as already shown of
one with amplitude three and a frequency
in the y direction of
frequency 3 and amplitude 2.
if we did this for every possible
frequency
say up to 128 or 256 different
frequencies
each of different amplitudes we would
end up displaying
these dots in
the x and y directions and it would look
like this
and what this is is a pictorial
representation
of amplitudes which is represented by
the intensity of each point
of each spatial frequency in each of the
x
in y directions which is determined by
the position of the point
the axes when we're dealing with spatial
frequencies
are conventionally labeled as k sub x
and k sub y but
it's important not to lose
site of the fact that each dot in this
single graph represents
a frequency in the x direction a
frequency in the right direction
and an amplitude of those frequencies
so what we saw at the last part of the
last slide
was this picture this is a pictorial
representation
of the numerical values of the
frequencies and their amplitudes that
come out of the mri scanner into the mri
receiver coil and it is
referred to as t two dimensional fourier
space
or simply as two-dimensional k space
uh the same
uh can be extrapolated to three
dimensions if you collect data
a frequency data in the z direction so
the third dimension becomes
kz in addition to kx and ky
and in this case we have 3d fourier
space
or 3d k space
this brings us to the summary frequency
is the number of times that something is
repeated over a period of time
or over a distance in space
the fourier transform is a mathematical
tool that allows a complicated waveform
consisting of multiple frequencies and
different amplitudes
to be decomposed into a sum of simple
sine waves of discrete frequencies
the induced voltage in an mri receiver
is this type of complicated waveform
so it lends itself well for fourier
analysis the conventional way of
displaying this mri data is a graph
where the intensity of each dot
represents the amplitude
of each spatial frequency at a
particular point in time
the position of each dot represents the
spatial frequency
in each direction and units of cycles
per unit length
to conclude a single graph can
accurately
show all the data obtained from every
position
in the object being scanned over the
entire measurement time
it can be displayed as a single picture
and the typical representation
is shown at the bottom right
that brings us to the end of this short
lecture thank you for your attention
تصفح المزيد من مقاطع الفيديو ذات الصلة
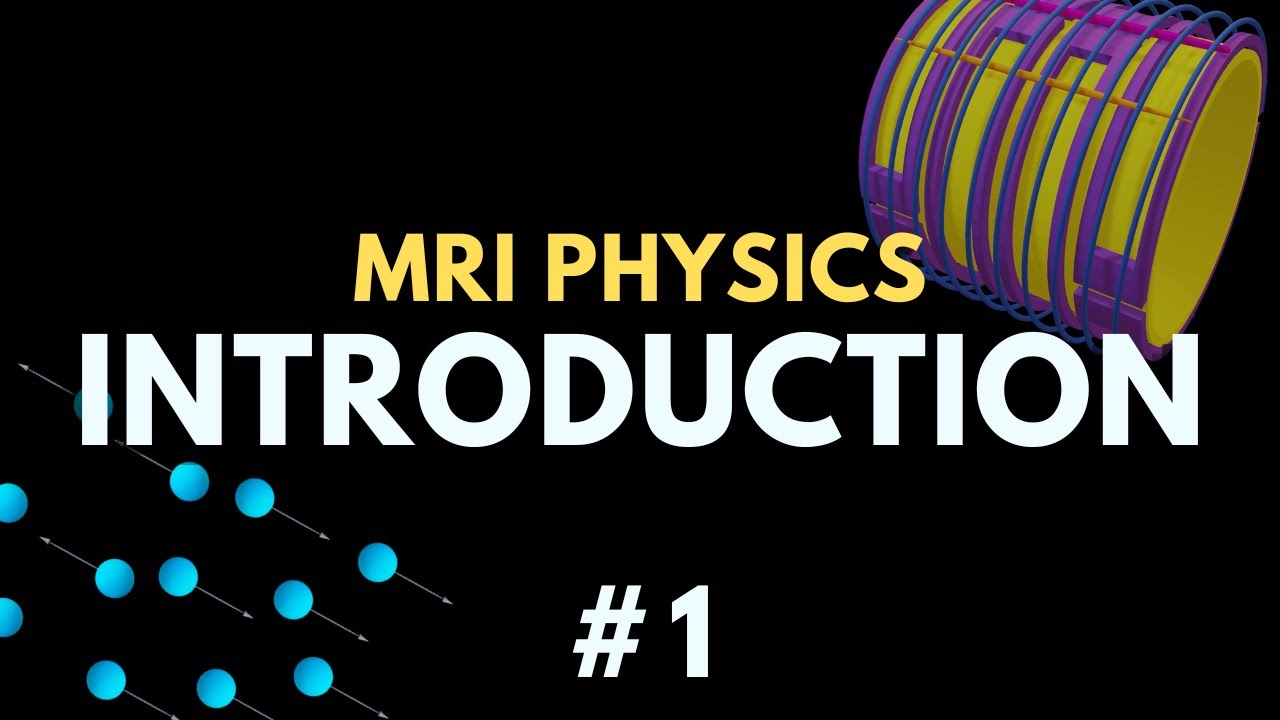
MRI physics overview | MRI Physics Course | Radiology Physics Course #1
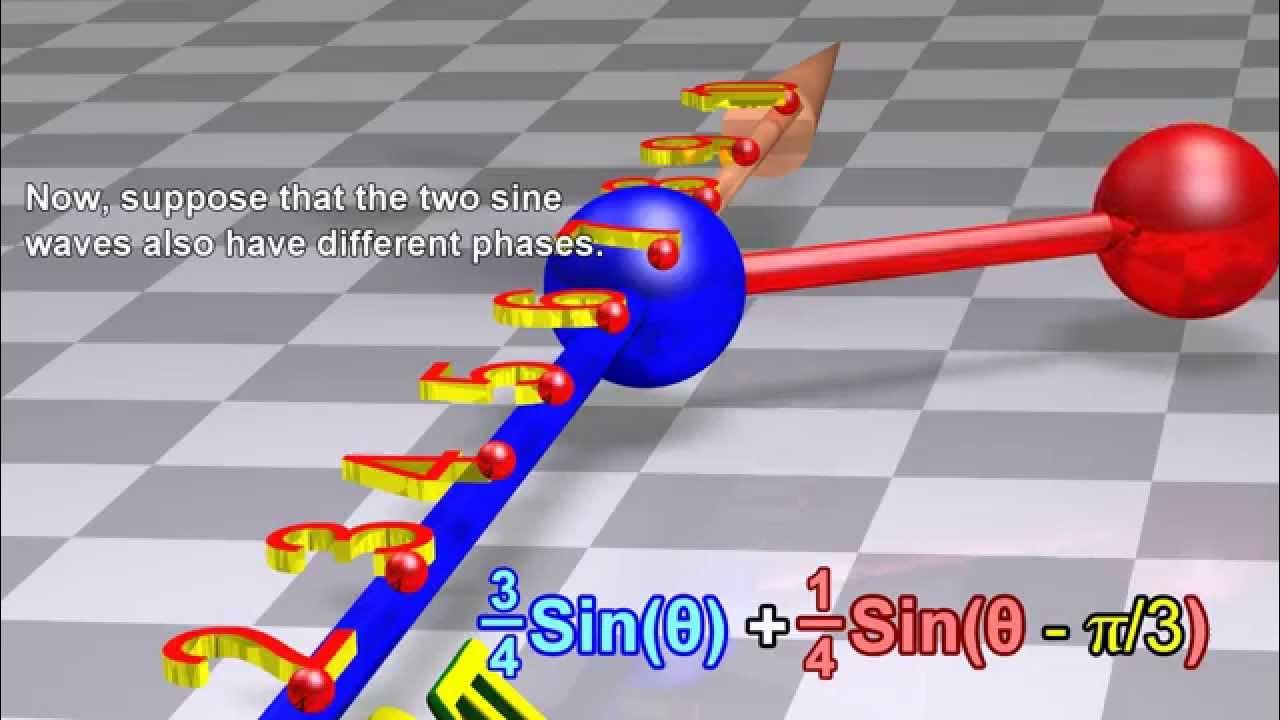
Fourier Transform, Fourier Series, and frequency spectrum
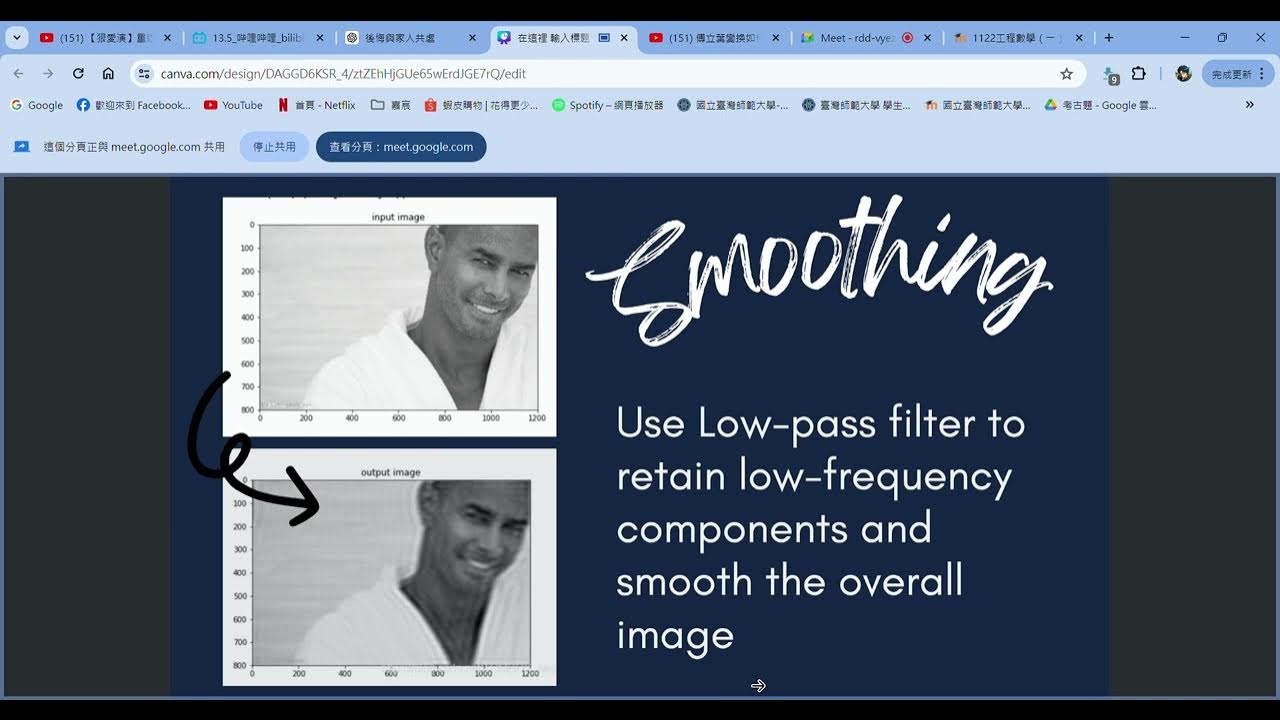
工程數學 傅立葉轉換報告
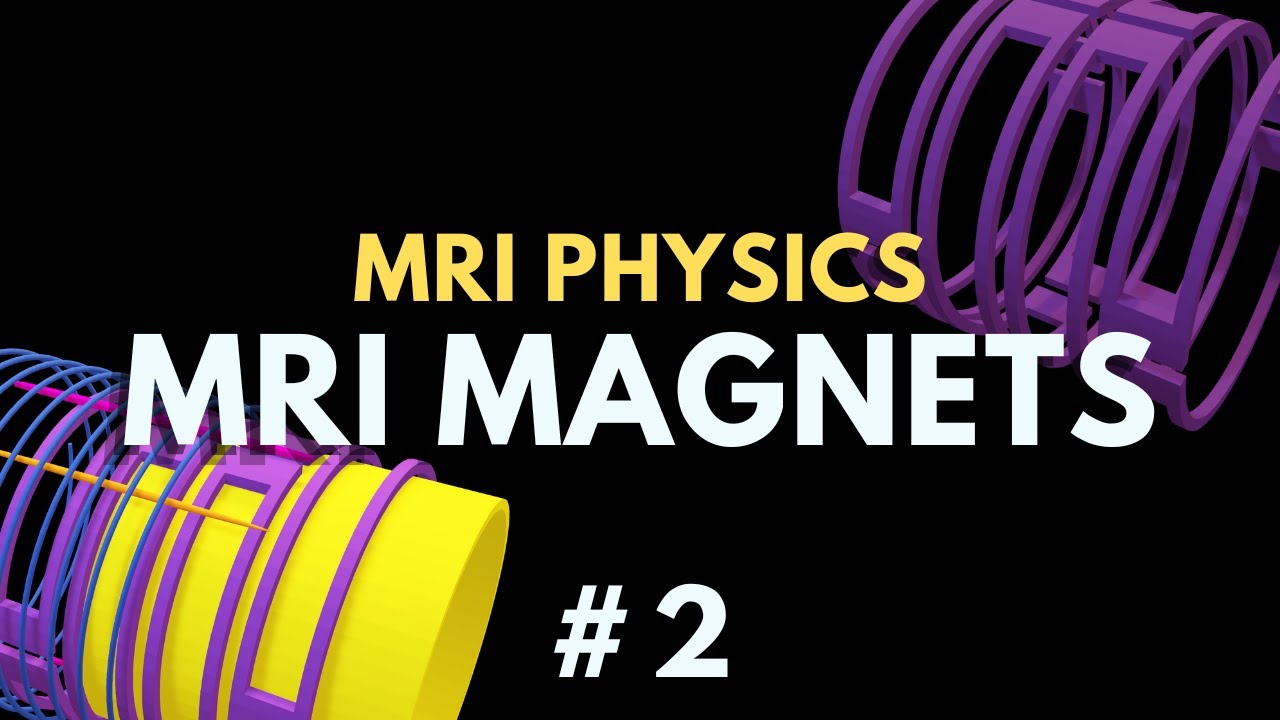
MRI Machine - Main, Gradient and RF Coils/ Magnets | MRI Physics Course | Radiology Physics Course#2

How does an MRI machine work?
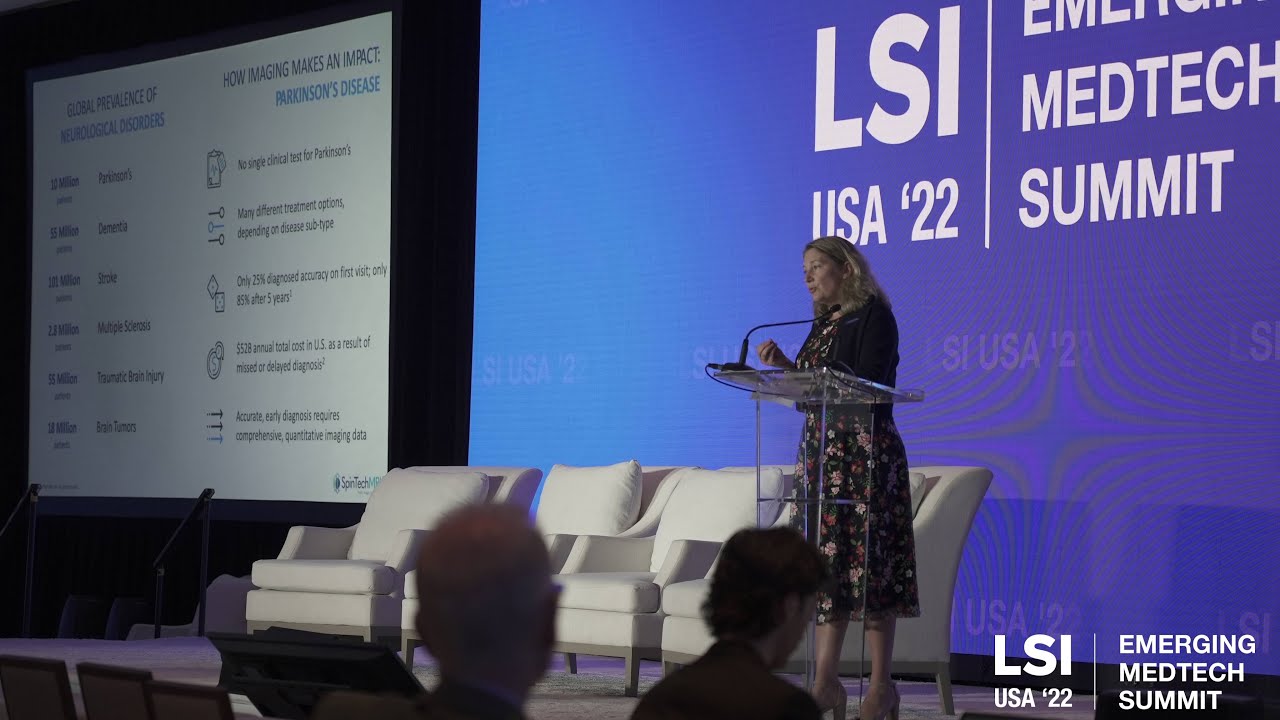
Karen Holzberger, President & CEO, SpinTech MRI | LSI USA '22 Emerging Medtech Summit
5.0 / 5 (0 votes)