Data Federation with Unity Catalog
Summary
TLDRIn this video, Pearl UU, a Technical Marketing Engineer at Databricks, discusses how the Databricks Data Intelligence Platform simplifies the discovery, querying, and governance of distributed data across multiple sources. She addresses the challenges posed by fragmented data and demonstrates how Databricks' Lakehouse Federation allows organizations to securely access and manage their data, regardless of its location, without the need for data ingestion. The video also includes a demo on creating connections and managing data across different databases using Databricks' Unity Catalog.
Takeaways
- 🌐 Data is often scattered across multiple systems, making it hard to discover and access, which can hinder informed decision-making and innovation.
- 🔍 The Databricks Data Intelligence Platform aims to simplify the discovery, querying, and governance of data, regardless of its location.
- 🚀 Organizations face challenges with data integration due to the time and resources required to move data to a single platform, which can slow down execution.
- 🔒 Fragmented governance can lead to weak compliance and increased risk of inappropriate data access or leakage, affecting collaboration and data democratization.
- 🏰 Lakehouse Federation is introduced as a solution to address the pain points of a data mesh framework, allowing for easier exposure, querying, and governance of siloed data systems.
- 🔑 With Lakehouse Federation, users can automatically classify and discover all data types in one place, enabling secure access and exploration for everyone in the organization.
- 🛠️ The platform accelerates ad hoc analysis and prototyping across all data and analytics use cases without the need for data ingestion.
- 📊 Advanced query planning and caching across sources ensure optimal query performance, even when combining data from multiple platforms with a single query.
- 🛡️ A unified permission model allows for setting and applying access rules and safeguarding data across sources, including row and column-level security and tag-based policies.
- 🔄 Data lineage and auditability are built-in, helping to track data usage and meet compliance requirements, with the ability to visualize upstream and downstream relationships.
- 📝 The demonstration showcases how to discover, govern, and query data spread across PostgreSQL, MySQL, and Delta Lake in a unified and easy manner using Databricks tools.
Q & A
What is the main challenge with data scattered across multiple systems?
-The main challenge is that it makes data discovery and access difficult, leading to incomplete data and insights, which hinders informed decision-making and innovation.
Why does data integration take time and resources, slowing down execution?
-Data integration is time-consuming because it requires moving data from external sources to a chosen platform, and some data might not be worth the effort due to the time it takes before landing in a unified location.
What are the risks associated with fragmented governance?
-Fragmented governance leads to weak compliance, duplication of efforts, and an increased risk of not being able to monitor and guard against inappropriate access or data leakage, which hinders collaboration and data democratization.
How does the Lakehouse Federation approach address the pain points of a data mesh?
-Lakehouse Federation simplifies the exposure, querying, and governance of siloed data systems as an extension of the Lakehouse, enabling automatic classification, discovery, and secure access to all data, regardless of its location.
What does it mean to enable everyone in an organization to securely access and explore all data available?
-It means that all users within an organization can access and explore data from various sources in a unified manner, with no need for data ingestion, and with advanced query planning and caching for optimal performance.
How does the Lakehouse Federation ensure optimal query performance across multiple platforms?
-It uses a single engine for advanced query planning across sources and caching, ensuring that even when combining data from multiple platforms with a single query, the performance is optimized.
What is the significance of having a single permission model for data governance?
-A single permission model allows for setting and applying access rules consistently across different data sources, enabling row and column level security, tag-based policies, centralized auditing, and compliance requirements with built-in data lineage and auditability.
How does the script demonstrate the process of creating a connection to an external database system?
-The script shows the process by creating a connection to a PostgreSQL database using a function and then creating a foreign catalog that mirrors the PostgreSQL database in the unified catalog for querying and managing user access.
What is the purpose of granting access to users or groups of users to use a connection?
-Granting access promotes democratic data processing in a seamless and efficient way, allowing appropriate teams to respond to data changes quickly.
How does the script illustrate the importance of data lineage for understanding data relationships?
-The script uses a lineage graph to show how tables were created, starting with federating into a PostgreSQL database directly from Databricks, and subsequently creating Delta tables, illustrating the lineage between different tables and their sources.
What are the prerequisites for creating a foreign catalog in the Databricks environment?
-To create a foreign catalog, one must have the 'create catalog' permission on the metastore and be either the owner of the connection or have the 'create foreign catalog' privilege on the connection.
Outlines
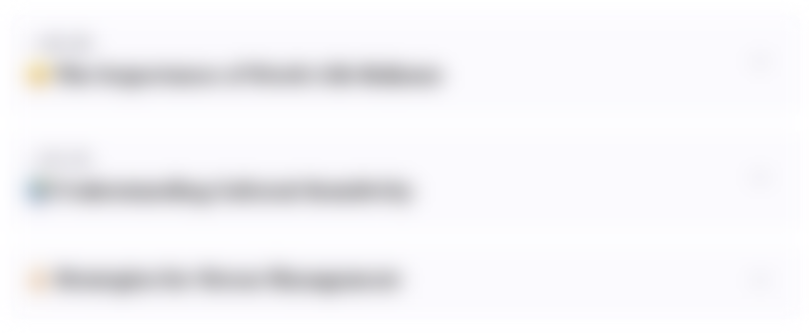
此内容仅限付费用户访问。 请升级后访问。
立即升级Mindmap
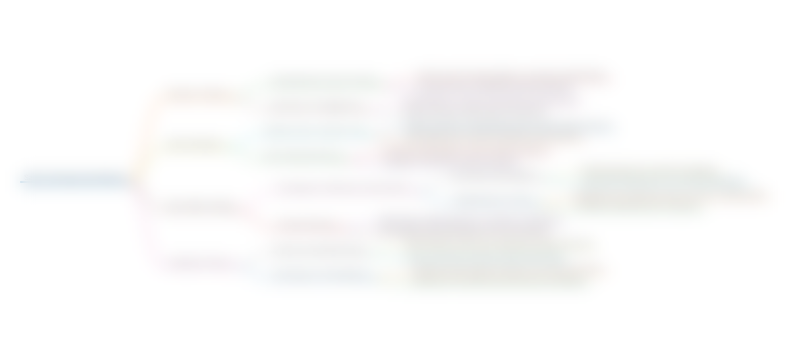
此内容仅限付费用户访问。 请升级后访问。
立即升级Keywords
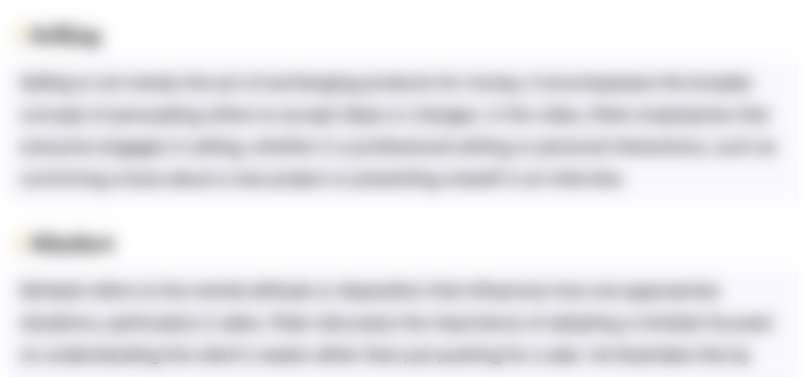
此内容仅限付费用户访问。 请升级后访问。
立即升级Highlights
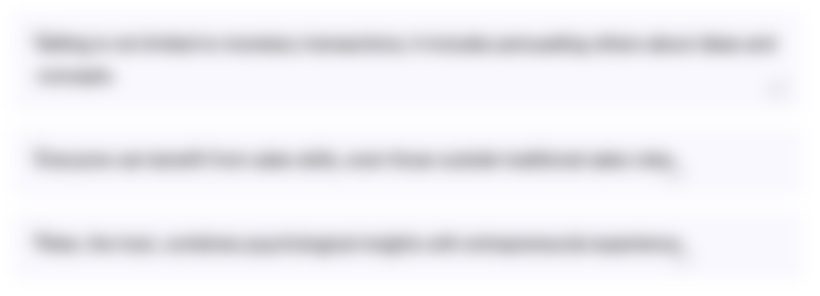
此内容仅限付费用户访问。 请升级后访问。
立即升级Transcripts
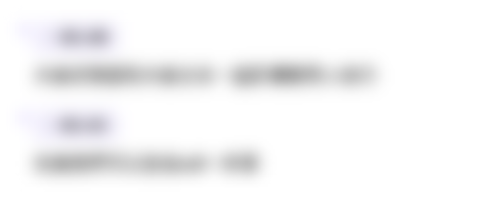
此内容仅限付费用户访问。 请升级后访问。
立即升级5.0 / 5 (0 votes)