Lecture 10.1 - Binomial distribution - Bernoulli distribution
Summary
TLDRThe script discusses the concept of Bernoulli trials, a type of experiment with two possible outcomes, success and failure, each with its probability. It explains how these trials are used to model binary outcomes in various scenarios, such as coin tosses, dice rolls, and opinion polls. The script delves into the parameters of the Bernoulli distribution, including its expectation (E[X] = p) and variance (Var(X) = p(1-p)), highlighting the maximum uncertainty when the probability of success equals the probability of failure. The importance of understanding the Bernoulli random variable and its parameter p in the context of binary experiments is emphasized.
Takeaways
- 📚 The script discusses the concept of Bernoulli trials and their applications in various scenarios, emphasizing the importance of understanding the parameters and outcomes of these trials.
- 🎲 A Bernoulli trial is defined as an experiment with two possible outcomes, typically labeled as 'success' (1) and 'failure' (0), with the probability of success being denoted by 'p'.
- 🧩 The script explains that the Bernoulli distribution is characterized by its parameter 'p', which represents the probability of success in a single trial.
- 📉 The variance of a Bernoulli random variable is given by p(1-p), indicating that the maximum variance occurs when p equals 0.5, which corresponds to the highest level of uncertainty.
- 🔍 The expectation (expected value) of a Bernoulli random variable is E[X] = p, which is a measure of the center of the distribution.
- 📈 The script illustrates the calculation of expected value and variance for Bernoulli trials, providing the formulas E[X] = ∑xi * P(X = xi) and Var(X) = p - p^2.
- 🌐 The concept of Bernoulli trials is applied to real-world examples such as coin tosses, dice rolls, opinion polls, elections, and pharmaceutical trials to demonstrate their practical relevance.
- 🤔 The script encourages the audience to consider what constitutes a 'success' or 'failure' in the context of different experiments, highlighting the subjective nature of these labels.
- 📊 The script introduces the idea of probability mass function or probability distribution for Bernoulli random variables, which assigns probabilities to all possible outcomes.
- 🔑 The parameter 'p' is key to understanding the Bernoulli distribution, as it dictates both the expected value and the variance of the variable.
- 🌟 The maximum variance in a Bernoulli trial, which indicates the highest uncertainty, occurs when the probability of success and failure is equal (p = 0.5).
Q & A
What is the main focus of the script regarding learning in the context of Bayesian statistics?
-The script focuses on understanding the Bayesian approach to probability distributions, specifically how they naturally develop and the impact of the parameters of the Bayesian distribution on its shape.
What are the two outcomes typically associated with a Bernoulli trial?
-A Bernoulli trial is associated with two outcomes: 'success' and 'failure', which are often assigned the values 1 and 0, respectively.
How is the term 'success' defined in the context of a Bernoulli trial?
-In a Bernoulli trial, 'success' is defined as the occurrence of a specific event, such as getting a head in a coin toss, and is assigned the value of 1.
What is the significance of the parameter 'p' in a Bernoulli distribution?
-The parameter 'p' in a Bernoulli distribution represents the probability of success. It is crucial as it determines the expected value and variance of the distribution.
How is the expected value (E[X]) of a Bernoulli random variable calculated?
-The expected value (E[X]) of a Bernoulli random variable is calculated using the formula E[X] = 0 * (1-p) + 1 * p, which simplifies to p.
What is the formula for calculating the variance (Var(X)) of a Bernoulli random variable?
-The variance (Var(X)) of a Bernoulli random variable is calculated using the formula Var(X) = p * (1 - p).
At what value of 'p' does the variance of a Bernoulli random variable reach its maximum?
-The variance of a Bernoulli random variable reaches its maximum when p = 0.5, as this is when the uncertainty or probability of both outcomes (success and failure) is equal.
What does the script imply about the relationship between the probability of success and the variance in a Bernoulli trial?
-The script implies that the variance is directly related to the probability of success, with the highest variance occurring when the probability of success is 0.5, indicating equal chances of success and failure.
How does the script describe the concept of a fair coin toss in terms of Bernoulli trials?
-The script describes a fair coin toss as an example of a Bernoulli trial with the highest uncertainty, where the probability of getting heads (success) and tails (failure) is equal, both being 0.5.
What is the importance of understanding the Bernoulli distribution in the context of Bayesian statistics?
-Understanding the Bernoulli distribution is important as it serves as the foundation for more complex Bayesian statistics, providing insights into the behavior of binary outcomes and their associated probabilities.
How does the script suggest we can apply the concept of Bernoulli trials in real-life situations?
-The script suggests that Bernoulli trials can be applied to various real-life situations, such as political elections, opinion polls, and pharmaceutical trials, where the outcome can be categorized as a success or failure.
Outlines
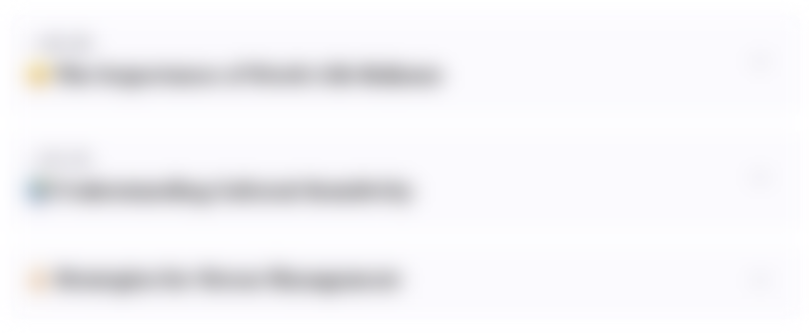
此内容仅限付费用户访问。 请升级后访问。
立即升级Mindmap
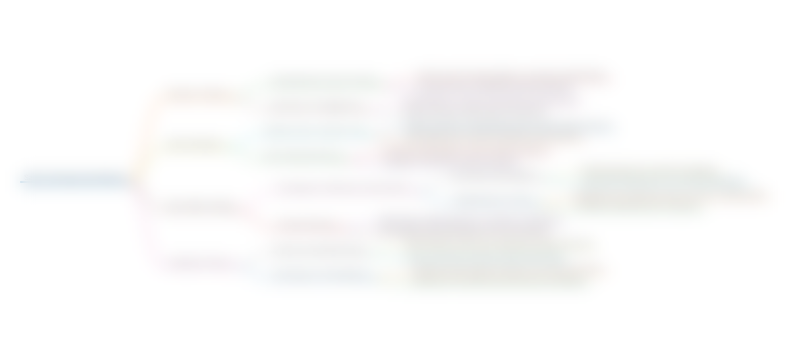
此内容仅限付费用户访问。 请升级后访问。
立即升级Keywords
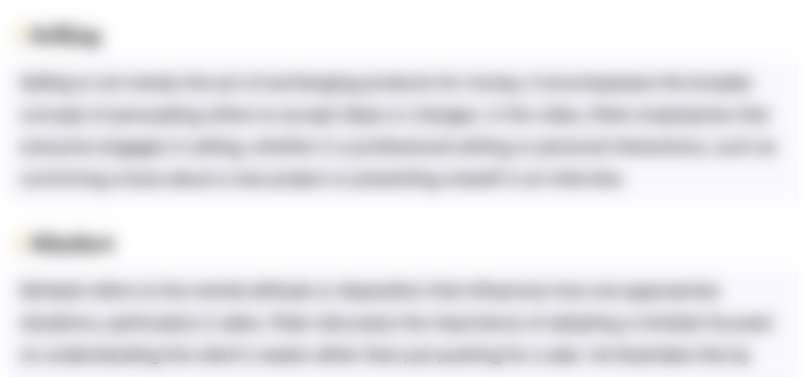
此内容仅限付费用户访问。 请升级后访问。
立即升级Highlights
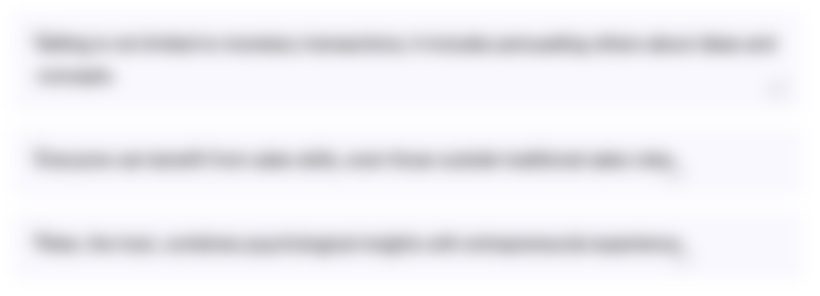
此内容仅限付费用户访问。 请升级后访问。
立即升级Transcripts
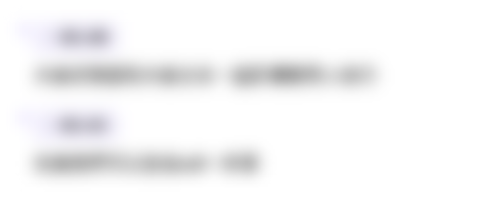
此内容仅限付费用户访问。 请升级后访问。
立即升级浏览更多相关视频
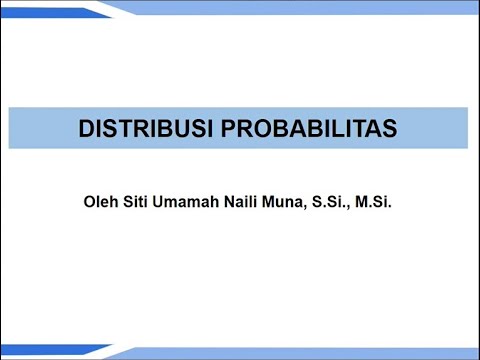
Pertemuan 1 - Distribusi Probabilitas (Part 2)
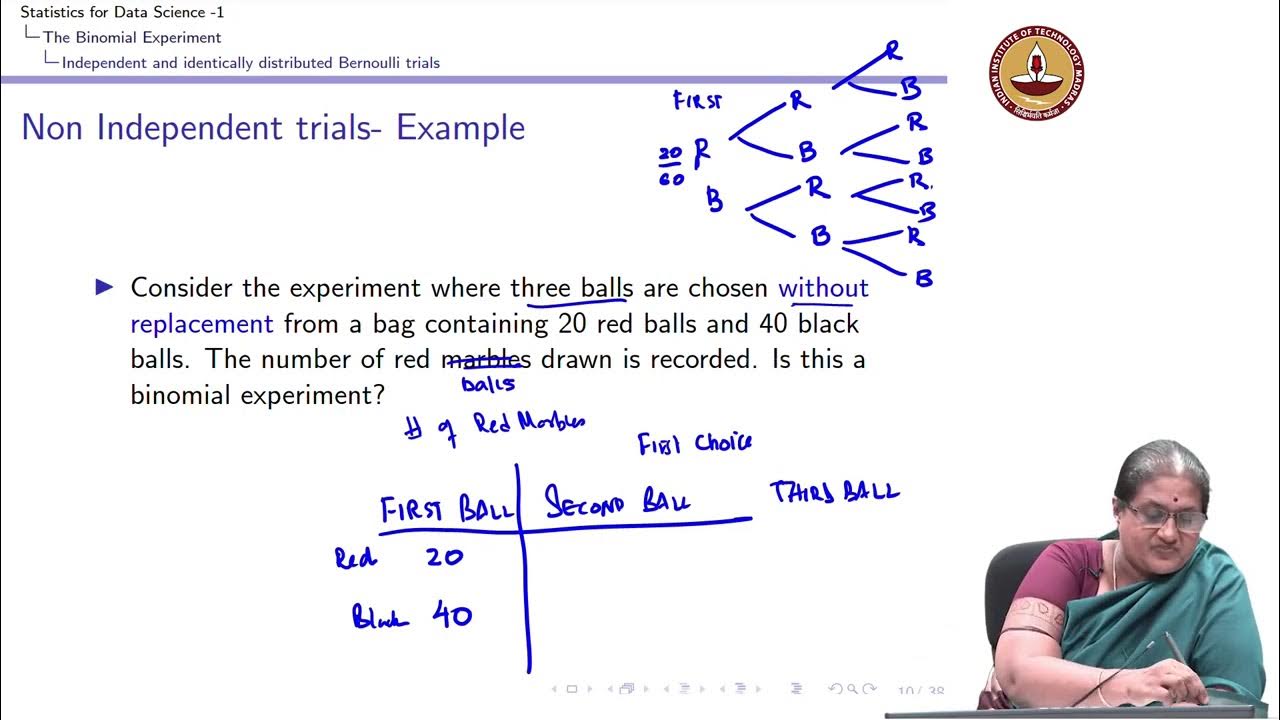
Lecture 10.2 - Binomial distribution - IID Bernoulli trials

Metode Statistika | Sebaran Peluang Diskrit | Bernoulli | Binomial | Poisson
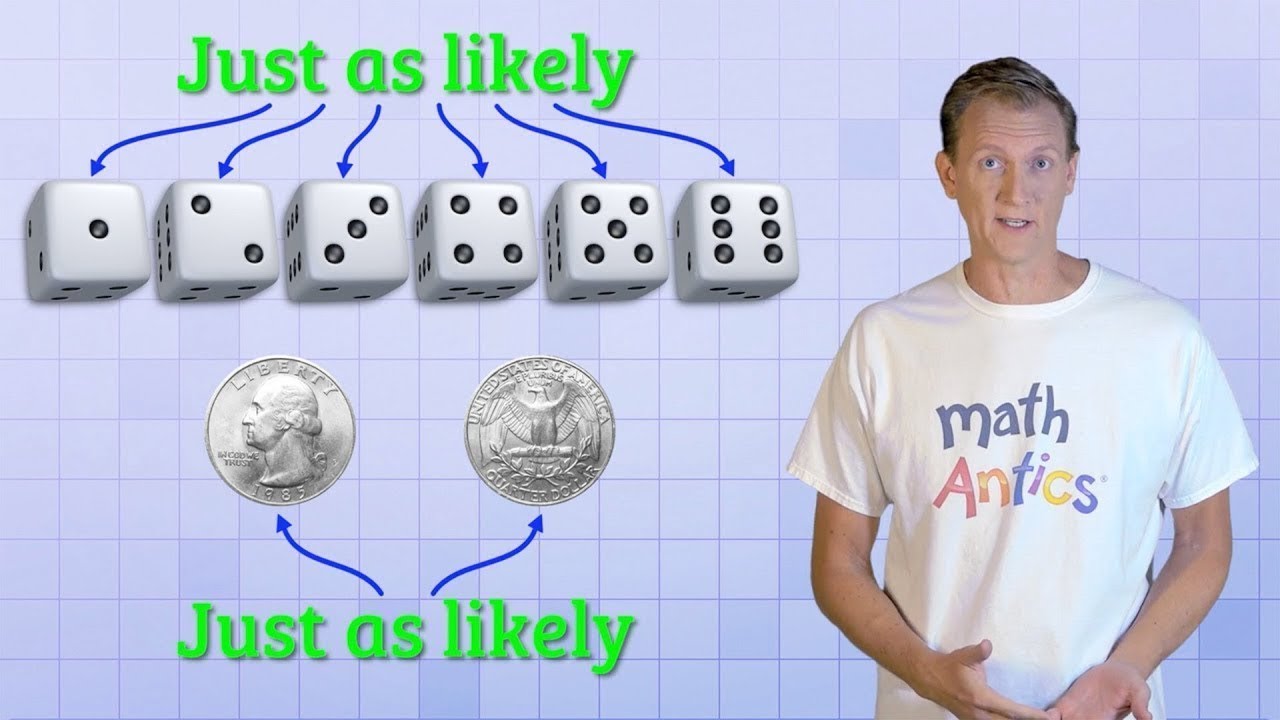
Math Antics - Basic Probability
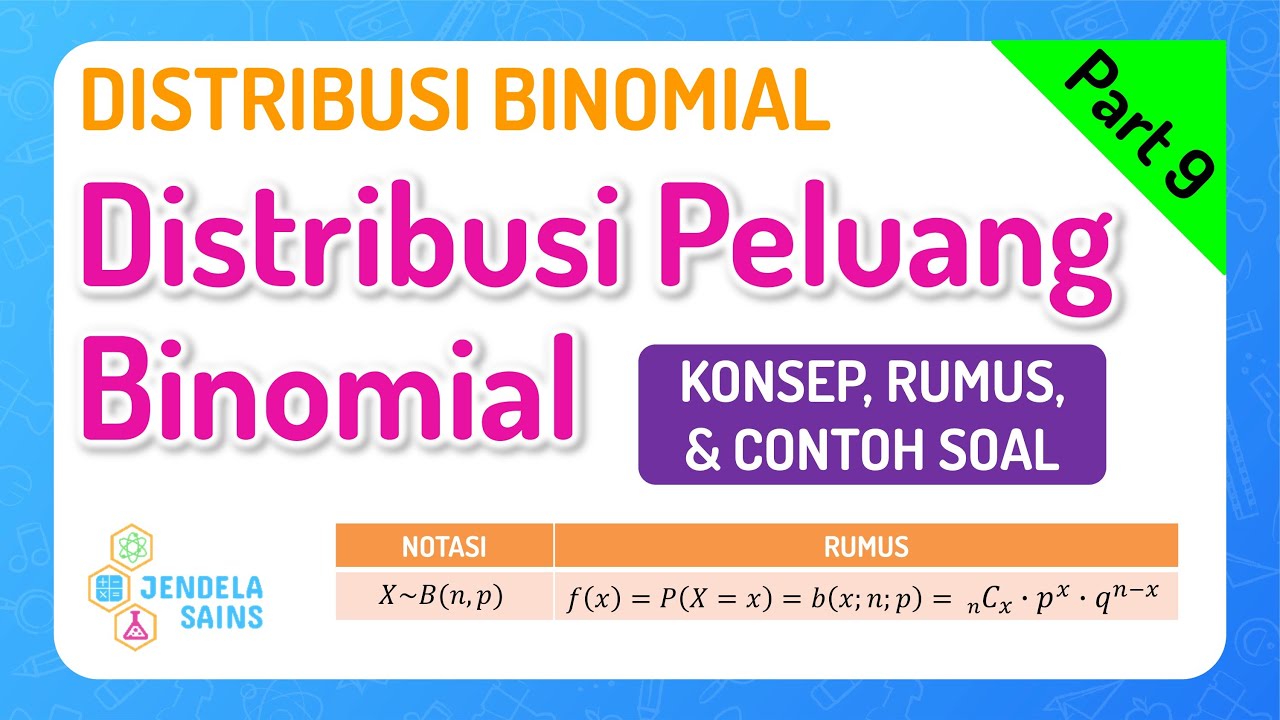
Distribusi Binomial • Part 9: Distribusi Peluang Binomial
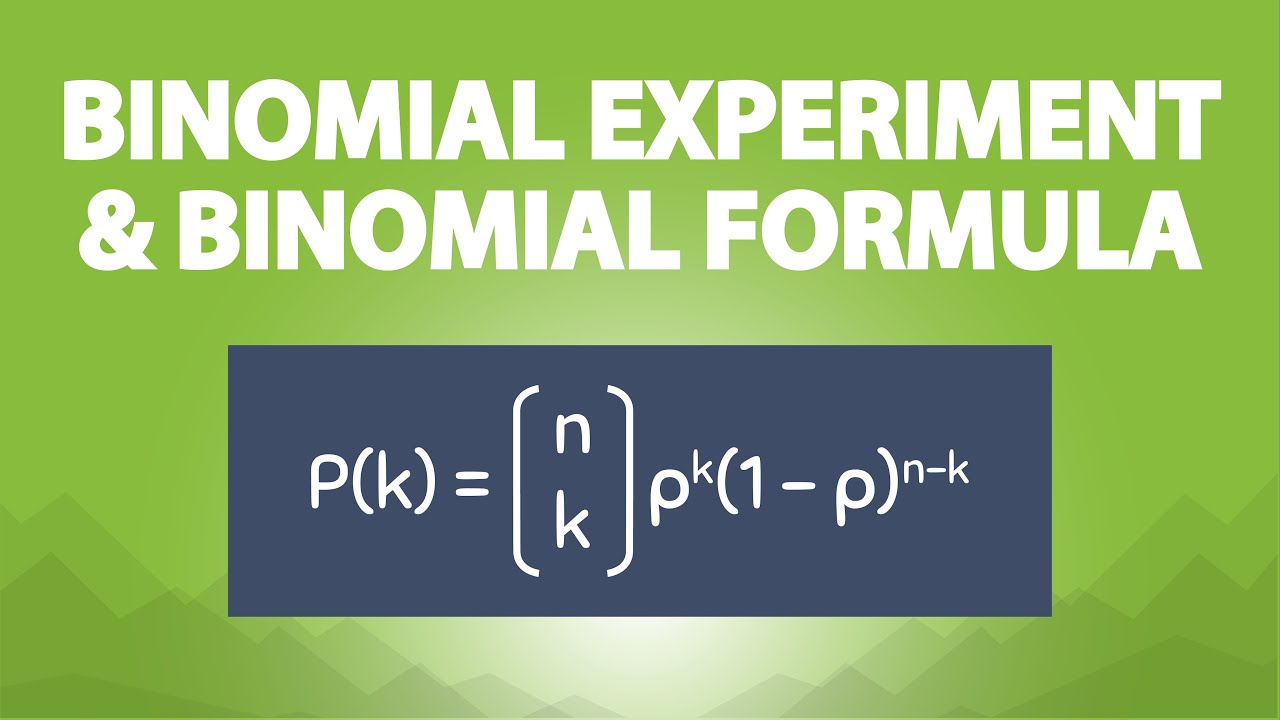
The Binomial Experiment and the Binomial Formula (6.5)
5.0 / 5 (0 votes)