Brainpower - Best of replay: Prompt Fundamentals with ChatGPT
Summary
TLDRIn this episode of 'Brain Power', Josh explores the basics of prompting in large language models like GPT and Bard. He demonstrates how to build effective prompts by adding roles, tasks, and detailed instructions to improve the quality of responses from AI models, using the example of creating learning objectives for cooking a smoked potato salad.
Takeaways
- 🧠 The episode focuses on the fundamentals of prompting in large language models like GPT, Bard, and Claude.
- 💻 It emphasizes the importance of compute power and data in the functioning of large language models.
- 📚 The script explains how large language models are trained and the role of human intervention in setting guardrails for responses.
- 🎲 The concept of probability in generating responses from language models is discussed, highlighting the variability of outcomes based on input prompts.
- 📝 The video introduces the idea of 'zero-shot prompts' where the model is expected to generate a response without prior examples or additional information.
- 📈 The role of adding a professional role to prompts, such as 'instructional designer', is shown to influence the quality of responses.
- 📑 The script demonstrates the process of building up a prompt by adding specific tasks and detailed instructions to refine the model's output.
- 🥔 A practical example is given using the task of creating learning objectives for cooking a smoked potato salad.
- 🔍 The importance of specificity, measurability, achievability, result-orientation, and time-bound criteria in crafting effective learning objectives is discussed.
- 🔗 The video mentions the availability of additional resources and prompts for learning and development, guiding viewers to access them.
- 📚 Lastly, the script encourages viewers to follow along with the examples and try using the prompts in their own large language model interactions.
Q & A
What is the main topic of the episode of 'Brain Power'?
-The main topic of the episode is exploring prompt fundamentals and how they work in large language models like chat GPT, Bard, and Claude.
What are the two important aspects of a large language model according to the script?
-The two important aspects are compute power, which is necessary to drive the model and provide results in a timely manner, and data, which includes the corpus of information used to train the model and the guardrails in place with that information.
Why does Josh mention the difference between GPT 3.5 and GPT 4 in terms of speed?
-Josh mentions the difference to highlight that there are variations in the speed of responses between different versions of the model, with GPT 4 being faster than GPT 3.5.
What is a 'zero-shot prompt' as mentioned in the script?
-A 'zero-shot prompt' is a simple request given to the model without any examples or additional information, relying on the model's training to provide a relevant response.
What is the role of 'control statement' in prompting?
-A 'control statement' is used when prompting to ensure that the model understands the context and limitations of the request, preventing inappropriate or irrelevant responses.
Why does Josh create a new chat for each prompt in the exercise?
-Josh creates a new chat for each prompt to avoid any influence from prior prompts, ensuring that each 'zero-shot prompt' is independent and not affected by previous interactions.
What is the purpose of adding a 'role' to the prompt?
-Adding a 'role' to the prompt, such as 'act like an instructional designer', influences the quality of the information returned by the model by aligning the response with the expertise associated with that role.
What are 'SMART' criteria used for in the context of learning objectives?
-SMART criteria are used to describe a learning objective in a way that is Specific, Measurable, Achievable, Result-oriented, and Time-bound, ensuring clarity and effectiveness in the objective.
How does adding detailed instructions to a prompt improve the results from the model?
-Adding detailed instructions to a prompt provides the model with more specific guidance on what is expected, leading to more accurate and relevant responses.
What is the significance of the 'Plinko game' analogy used in the script?
-The 'Plinko game' analogy is used to illustrate the concept of probability in how a large language model generates responses based on the input prompt.
Where can viewers find additional content and support for the episodes?
-Viewers can find additional content and support at Josh Cavalier's website or by accessing the prompts provided at JoshCavalier.com/brainpower.
Outlines
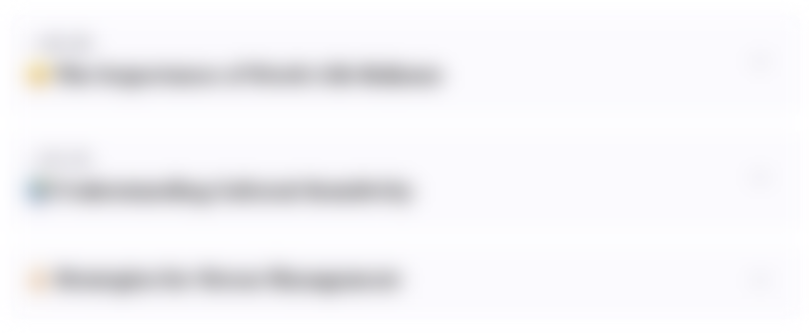
此内容仅限付费用户访问。 请升级后访问。
立即升级Mindmap
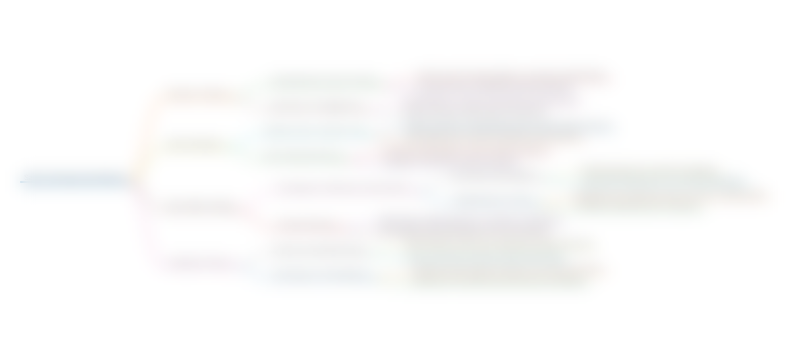
此内容仅限付费用户访问。 请升级后访问。
立即升级Keywords
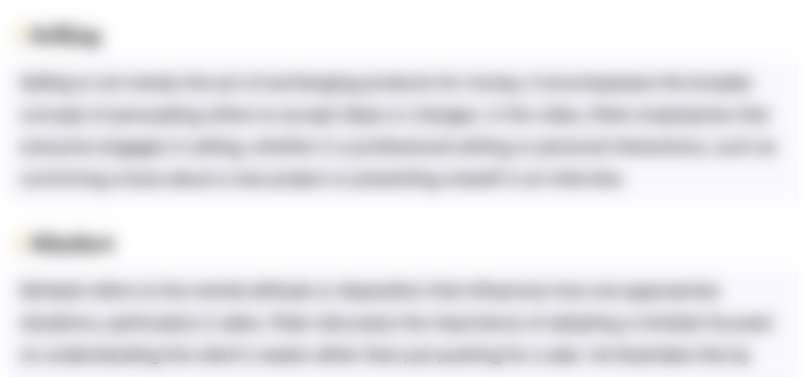
此内容仅限付费用户访问。 请升级后访问。
立即升级Highlights
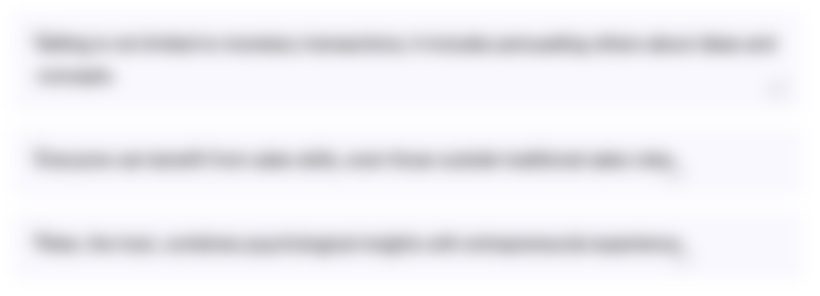
此内容仅限付费用户访问。 请升级后访问。
立即升级Transcripts
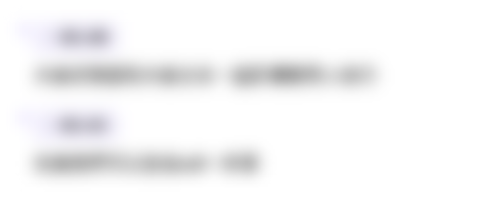
此内容仅限付费用户访问。 请升级后访问。
立即升级浏览更多相关视频
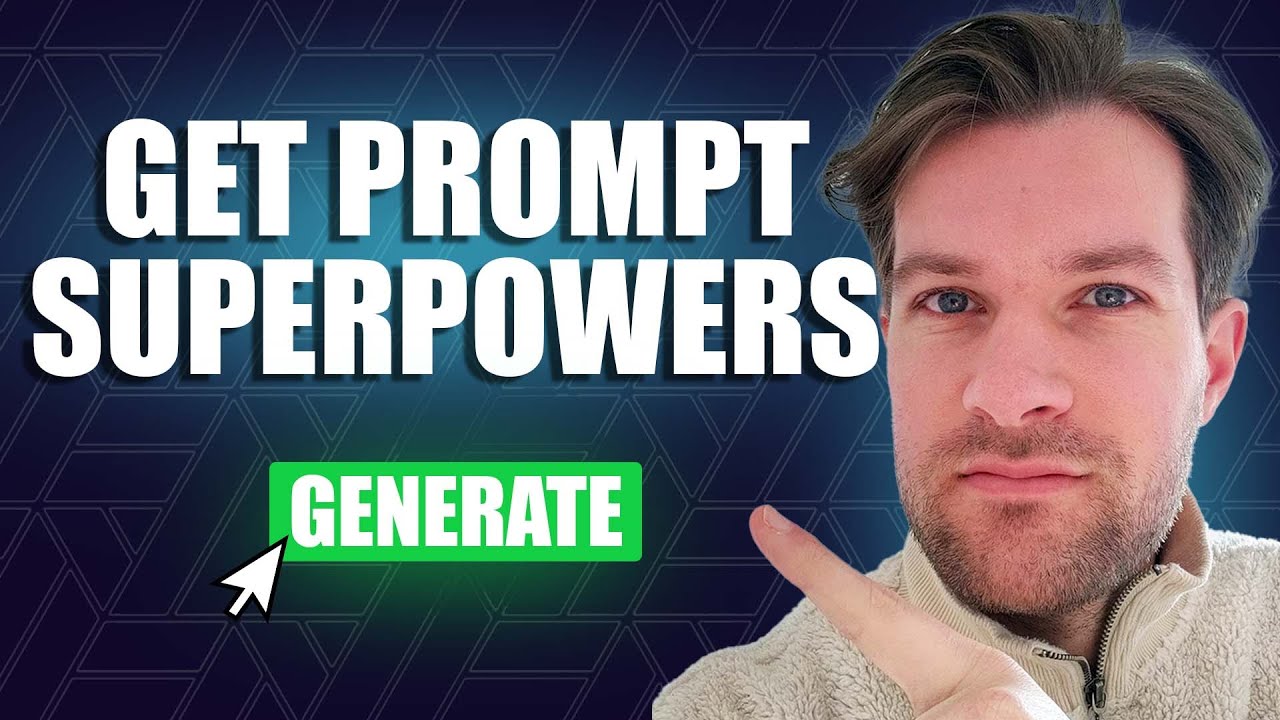
The Perfect Prompt Generator No One Knows About
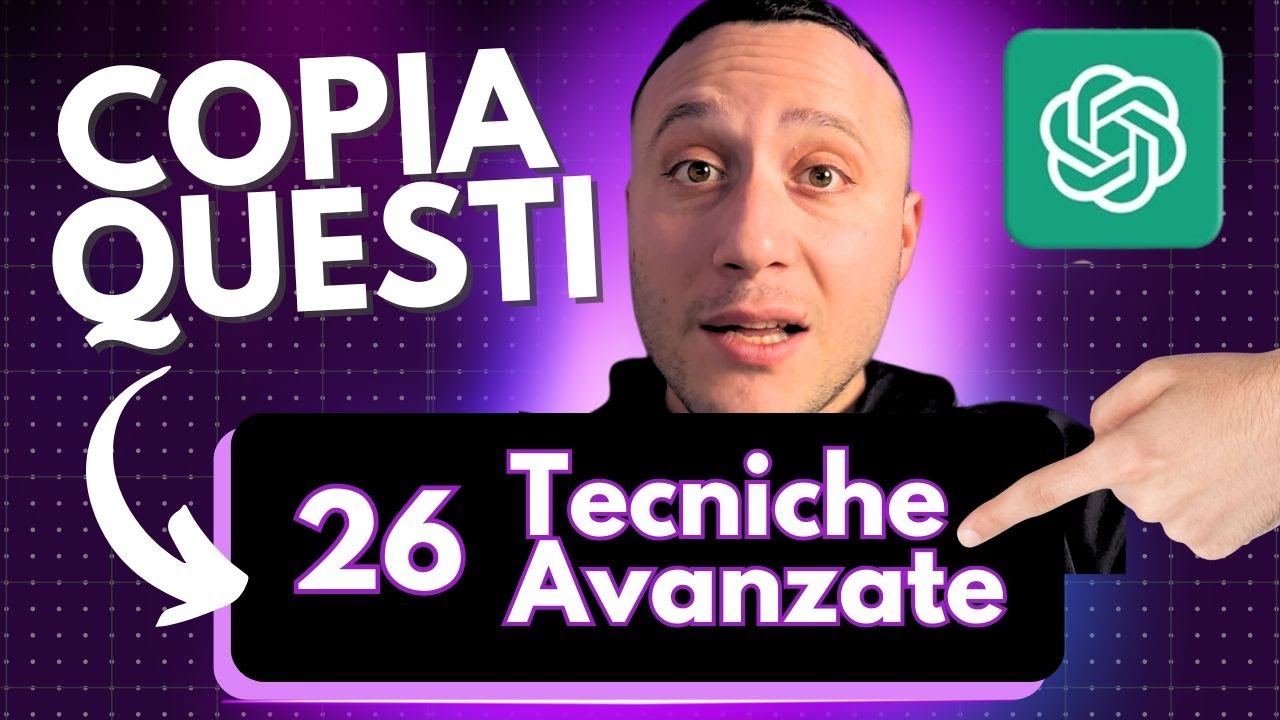
26 prompt ChatGPT +50% di qualità ✅

26 easy prompt engineering principles for 2024

Prompt MASTERY : 26 principles for Mastering Prompts !
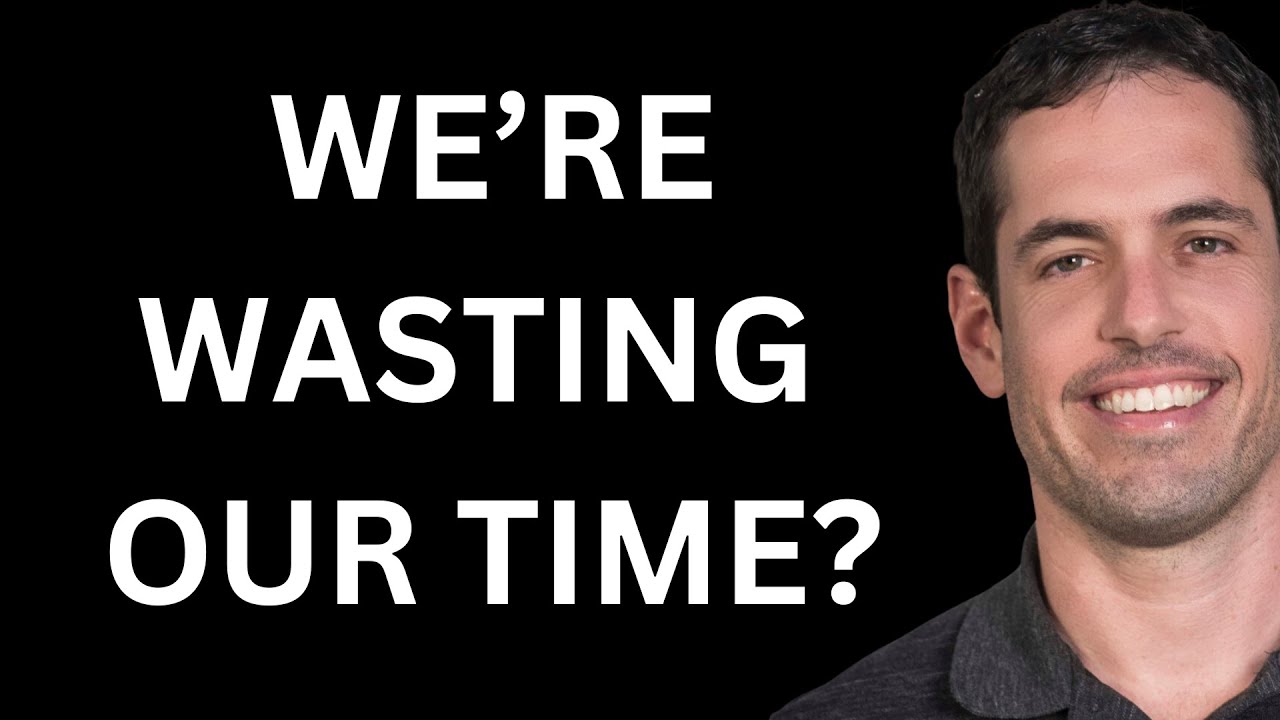
Prompt Engineering = BS? (Must Watch)

Mastering Summarization Techniques: A Practical Exploration with LLM - Martin Neznal
5.0 / 5 (0 votes)