Challenges in Machine Learning | Problems in Machine Learning
Summary
TLDRThe video script discusses the intricacies of machine learning, emphasizing the importance of data collection, the challenges of feature selection, and the potential pitfalls of overfitting. It delves into the practical aspects of deploying machine learning models, including the complexities of software integration and the need for continuous learning and adaptation. The speaker also touches on the future of machine learning, suggesting that it will become more accessible and integrated into various platforms, highlighting the importance of understanding both the technical and practical sides of machine learning projects.
Takeaways
- 📘 Data Collection: The importance of collecting data for machine learning projects cannot be overstated. It's the foundation upon which models learn and make predictions.
- 🔍 Data Quality: Ensuring the quality of data is crucial as it directly impacts the performance of machine learning algorithms. Time spent on cleaning and preparing data is often significant in a project's lifecycle.
- 🔧 Feature Engineering: The process of selecting and transforming features from the raw data to make it more informative for the model is highlighted as a key aspect of machine learning.
- 🔄 Overfitting: The script discusses the challenge of overfitting, where a model learns the training data too well and fails to generalize to new data.
- 📉 Underfitting: The opposite of overfitting, underfitting occurs when a model is too simple and doesn't capture the underlying pattern of the data.
- 🔗 Model Interpretability: The ability to understand and explain how a machine learning model makes its predictions is discussed as an important yet often overlooked aspect.
- 🛠️ Software Integration: The script touches on the complexities of integrating machine learning models into existing software systems and the challenges of ensuring compatibility across different platforms.
- 🌐 Online Learning and Deployment: The concept of deploying models in a way that they can be updated continuously as new data comes in, without needing to retrain from scratch, is mentioned.
- 🔍 Representative Data: The necessity of having representative data that accurately reflects the problem space to avoid biased or incorrect model predictions is emphasized.
- 🛡️ Ethical Considerations: While not explicitly stated, the script implies the importance of considering ethical implications when collecting and using data for machine learning.
- 🚀 Continuous Learning: The idea of treating machine learning as an ongoing process of learning and improvement, rather than a one-off task, is suggested by the discussion on model updates and maintenance.
Q & A
What is the main topic of the video script?
-The main topic of the video script is machine learning, focusing on various aspects such as data collection, the challenges faced in machine learning projects, and the importance of proper data representation and software integration.
What are some of the initial steps mentioned in a machine learning project?
-Some initial steps mentioned in a machine learning project include understanding what machine learning is, the difference between machine learning and deep learning, and the types of machine learning.
Why is data collection an important aspect of machine learning?
-Data collection is important in machine learning because the quality and relevance of the data directly impact the performance of the machine learning models. Without good data, even the best algorithms cannot produce accurate results.
What are some challenges faced when collecting data for machine learning?
-Challenges faced when collecting data for machine learning include obtaining a representative sample of the data, dealing with missing values, and converting data into the correct format for analysis.
What does the script suggest about the relationship between the amount of data and the effectiveness of machine learning models?
-The script suggests that having a large amount of data does not necessarily mean better results if the data is not properly sampled and representative of the problem space. It also mentions the concept of 'unreliable effectiveness of data' where too much data can be overwhelming and not always beneficial.
What is the importance of feature engineering in machine learning?
-Feature engineering is important in machine learning as it involves selecting and transforming the features or columns in the dataset to improve the performance of the machine learning models. It helps in creating meaningful representations of the data that contribute to better predictions.
Why is it necessary to consider software integration when developing machine learning models?
-Software integration is necessary because machine learning models need to be incorporated into existing software systems to be useful in real-world applications. This involves ensuring that the models work well across different platforms and operating systems.
What is the potential issue with overfitting in machine learning models?
-Overfitting occurs when a machine learning model learns the training data too well, including its noise and outliers, which can negatively impact the model's performance on new, unseen data.
What is the script's stance on the future of machine learning deployment?
-The script suggests that the future of machine learning deployment will involve more accessible libraries for various platforms, making it easier to deploy machine learning models. However, it also acknowledges the current challenges and the need for improvement in this area.
What advice does the script give regarding the practical implementation of machine learning models?
-The script advises that after learning and developing machine learning models, one should focus on converting these models into a proper software product and deploying them on servers to be used by actual users, ensuring both development and operational experience.
What is the importance of understanding the entire workflow in machine learning projects as mentioned in the script?
-Understanding the entire workflow in machine learning projects is crucial as it helps in identifying potential bottlenecks and challenges, from data collection to model deployment, which can ultimately lead to more efficient and effective machine learning solutions.
Outlines
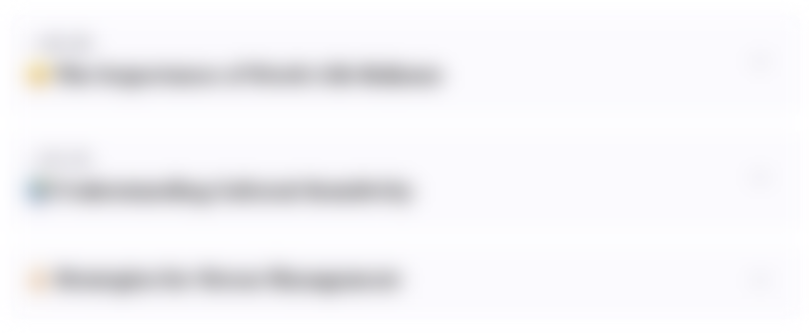
此内容仅限付费用户访问。 请升级后访问。
立即升级Mindmap
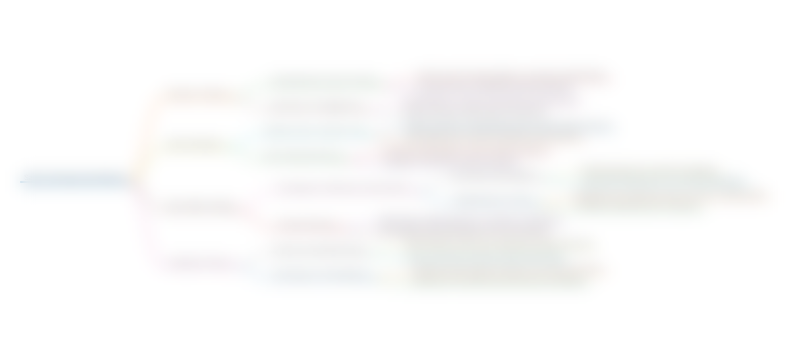
此内容仅限付费用户访问。 请升级后访问。
立即升级Keywords
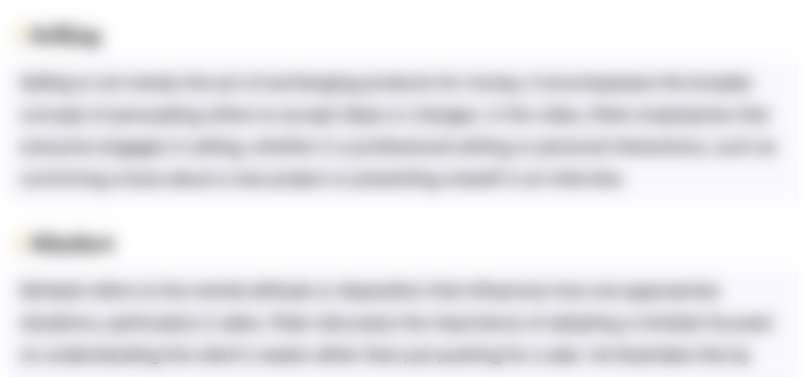
此内容仅限付费用户访问。 请升级后访问。
立即升级Highlights
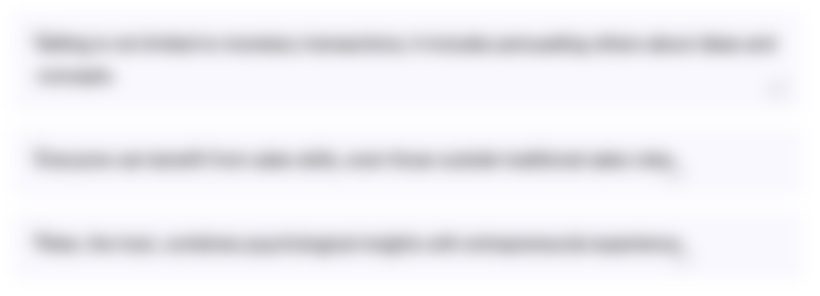
此内容仅限付费用户访问。 请升级后访问。
立即升级Transcripts
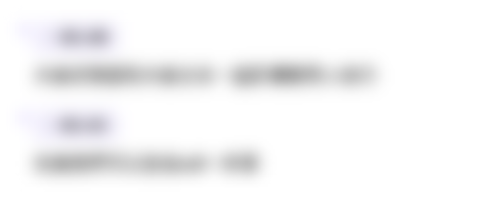
此内容仅限付费用户访问。 请升级后访问。
立即升级5.0 / 5 (0 votes)