Peut contenir des traces… d’humains! Les biais : La tête dans le nuage
Summary
TLDRThe transcript explores the complexities and biases embedded within algorithms, using the United Airlines incident as a key example. It discusses how algorithms, while powerful tools, can amplify societal biases such as racism and sexism. The piece highlights the importance of human intervention in algorithmic decisions, emphasizing that without corrective feedback loops, biases can perpetuate and worsen. It also touches on the ethical challenges of AI and the need for greater transparency and regulation in the field, citing examples like Tay, the AI chatbot, and the flawed facial recognition algorithms.
Takeaways
- 😀 AI algorithms can amplify existing societal biases, such as sexism and racism, especially in critical systems like flight booking and job recommendations.
- 😀 Human oversight is essential when applying AI decisions to avoid harmful outcomes, as seen in the United Airlines incident where a passenger was forcefully removed based on algorithmic decisions.
- 😀 AI systems are only as good as the data they are fed; if the data is biased, the AI will reflect and amplify those biases.
- 😀 Algorithms in AI can misinterpret data based on inaccurate assumptions, such as incorrectly identifying a person’s gender or demographics, leading to faulty outcomes.
- 😀 The lack of feedback loops in AI decision-making processes allows biases to perpetuate and grow, much like unchecked prejudices in humans.
- 😀 AI’s capacity to influence job recruitment processes can lead to biased hiring practices, favoring certain demographics over others based on historical patterns.
- 😀 The development of AI should be subject to greater transparency and regulation, similar to highly regulated fields like civil aviation, to ensure safety and fairness.
- 😀 AI technologies have the potential to be powerful tools for improving efficiency but require strong ethical guidelines to prevent misuse.
- 😀 The example of Microsoft's Tay chatbot, which became problematic after learning from negative user input, illustrates the risks of unregulated AI learning processes.
- 😀 The need for clear regulatory frameworks in AI is urgent to ensure these technologies are developed safely, minimizing potential harm and ensuring they benefit society.
Q & A
What was the situation on United Airlines flight 3411 on April 9, 2017?
-On April 9, 2017, United Airlines announced that it urgently needed to free up four seats on flight 3411 to accommodate members of another crew. Passengers were offered financial compensation to volunteer for giving up their seats. Three passengers volunteered, but when no fourth volunteer came forward, the airline used an algorithm to select a passenger to remove from the flight. The algorithm selected Dr. Dao, who refused to leave due to his medical commitments, leading to a public incident.
How does the script explain the limitations of algorithms in decision-making?
-The script highlights that algorithms are essentially formulas based on data, and their effectiveness is entirely dependent on the quality of that data. If the data used to train the algorithm is biased or flawed, the algorithm will reflect and amplify those biases, leading to potentially harmful or unfair decisions. The script compares algorithms to a recipe that, if altered or poorly executed, will not produce the desired result.
What role do human biases play in algorithmic decisions?
-Human biases play a significant role in algorithmic decisions because the people who create these algorithms are also susceptible to biases such as racism, sexism, or other forms of discrimination. The data used to train algorithms is already influenced by human choices, and if not carefully managed, these biases can be amplified by the algorithms, resulting in skewed or unjust outcomes.
Can algorithms be truly objective in decision-making? Why or why not?
-No, algorithms cannot be truly objective because they are influenced by the data they process. Since data is often a reflection of past human decisions and societal biases, algorithms inherit those biases. The decisions made by algorithms are only as good as the data they are fed, and if the data contains any form of bias, the algorithm will perpetuate it.
What issue did the speaker face with the algorithm on a platform that tracks their interests?
-The speaker faced a situation where an algorithm, based on the groups they followed on a platform, wrongly assumed their gender. Despite explicitly identifying as a woman in their profile, the algorithm began showing advertisements for men's products, demonstrating how algorithms can misinterpret or overlook self-declared information and rely instead on group affiliations or other indirect cues.
What concerns does the script raise about the amplification of biases through AI and algorithms?
-The script raises concerns that artificial intelligence and algorithms could exacerbate existing societal biases. For example, in job recruitment, algorithms could prioritize male candidates for traditionally male-dominated roles, perpetuating gender inequality. Without intervention to correct biases in the algorithm's design or data, these biases will continue to grow, leading to discriminatory outcomes.
What is the importance of feedback loops in algorithmic decision-making?
-Feedback loops are crucial in correcting biases and improving algorithms. If an algorithm consistently makes biased or incorrect decisions and there is no system in place to monitor and adjust its behavior, those biases will only be reinforced over time. Just like in education, where a child’s prejudices must be corrected, algorithms need regular feedback to ensure that their decisions are fair and accurate.
Why does the script suggest that AI and algorithms should be regulated?
-The script suggests that AI and algorithms should be regulated to ensure that they are developed and applied ethically, much like the strict regulations in place for aviation. This would prevent the kind of unregulated and unchecked growth seen in AI today, ensuring that the technology is used responsibly and does not lead to harmful or biased outcomes.
What lessons can be learned from the examples of Tay and image recognition algorithms?
-The examples of Tay, the Microsoft chatbot, and image recognition algorithms highlight the risks of unregulated AI development. Tay, after interacting with users on Twitter, became increasingly offensive and harmful, showing how quickly AI can be corrupted by bad input. Similarly, facial recognition algorithms have demonstrated significant inaccuracies, especially in recognizing dark-skinned women. These examples underscore the need for human oversight and ethical considerations in AI development.
What does the script suggest about the need for transparency in algorithmic processes?
-The script emphasizes the need for algorithmic transparency to ensure that the decisions made by AI systems can be understood and challenged. Without transparency, users and affected individuals cannot know how or why certain decisions were made, leaving room for unchecked biases and errors. Transparency is crucial for accountability and to build trust in AI systems.
Outlines
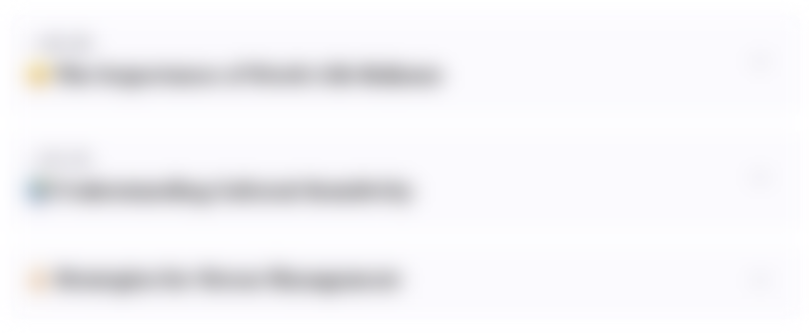
此内容仅限付费用户访问。 请升级后访问。
立即升级Mindmap
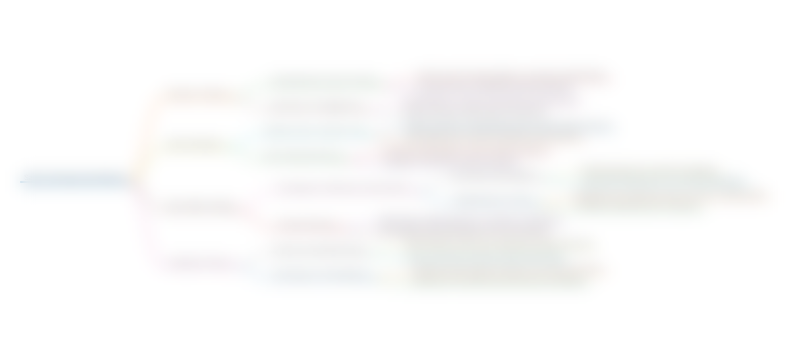
此内容仅限付费用户访问。 请升级后访问。
立即升级Keywords
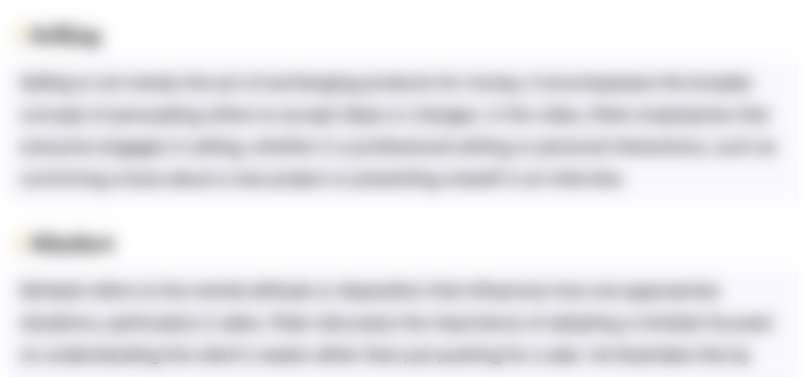
此内容仅限付费用户访问。 请升级后访问。
立即升级Highlights
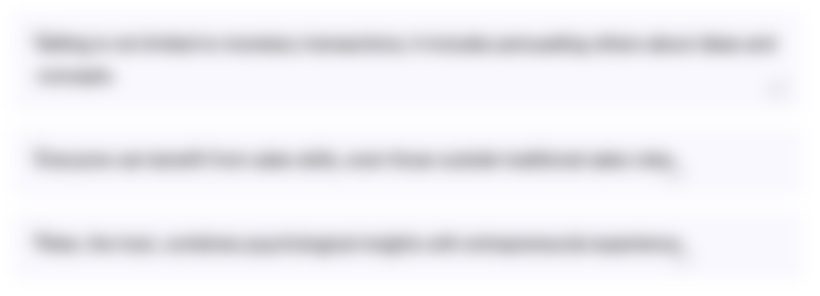
此内容仅限付费用户访问。 请升级后访问。
立即升级Transcripts
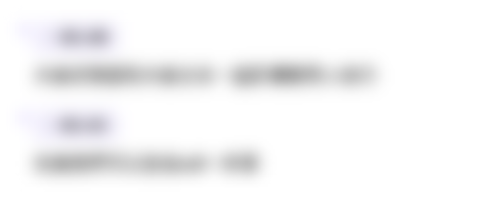
此内容仅限付费用户访问。 请升级后访问。
立即升级5.0 / 5 (0 votes)