Presentasi Tugas Manufaktur Digital_6132101033_6132101034_6132101047_6132101087
Summary
TLDRThe presentation discusses a research paper on using Digital Twin models combined with machine learning and optimization techniques to enhance supply chain resilience. The study addresses challenges faced by production and distribution systems during global disruptions like the COVID-19 pandemic, including transportation limitations, raw material shortages, and extended wait times. The proposed model optimizes system adaptability and recovery time, improving efficiency, reducing inventory costs, and ensuring product availability during unforeseen disruptions. The paper suggests expanding the model's use across various industries and integrating advanced technologies like IoT and blockchain for further resilience.
Takeaways
- 😀 The COVID-19 pandemic caused significant disruptions in supply chains, impacting transportation, raw material access, and operational timelines.
- 😀 Digital Twin (DT) models, combined with machine learning, are proposed as a solution to enhance the resilience of production and distribution systems during disruptions.
- 😀 DT models help systems quickly adapt to unexpected events like delays in shipping and logistical challenges, allowing for quicker recovery times.
- 😀 The research focused on optimizing supply chain performance by improving key performance indicators such as demand fulfillment rate, time to recover, and time to survive.
- 😀 The study shows that using Digital Twin models can maintain higher inventory fill rates and reduce storage costs by optimizing inventory management.
- 😀 Machine learning techniques like Artificial Neural Networks (ANN) are used to predict uncertainties and improve the supply chain’s resilience to disruptions.
- 😀 The Digital Twin model demonstrated superior performance over traditional strategies by maintaining better service levels while minimizing inventory waste.
- 😀 Key benefits of the Digital Twin model include faster recovery from disruptions, better forecasting, and more efficient inventory management.
- 😀 The research suggests expanding the application of the DT model to industries with different supply chain dynamics, such as food and beverage or automotive manufacturing.
- 😀 Suggestions for future research include integrating Digital Twin systems with IoT and blockchain to improve real-time data transparency and system robustness.
Q & A
What is the main focus of the paper discussed in the presentation?
-The main focus of the paper is the development of a digital twin model with machine learning and optimization techniques to enhance the resilience of production and distribution systems, particularly under disruptions such as natural disasters, pandemics, and operational issues.
What were the primary issues identified that affected the supply chain during the COVID-19 pandemic?
-The primary issues identified were transportation restrictions, limited raw materials, and increased wait times for shipments. These issues were exacerbated by the unpredictable nature of the pandemic and affected the overall production and distribution systems.
What are the key objectives of the research outlined in the paper?
-The key objectives are: 1) Developing an adaptive digital twin model for rapid adaptation to disruptions, 2) Optimizing the resilience of production and distribution systems, 3) Measuring system resilience using key performance indicators like 'time to recover' and 'time to survive'.
How does the digital twin model contribute to system resilience?
-The digital twin model enhances resilience by using real-time data and predictive analytics to simulate real-world disruptions, allowing the system to adapt and recover more quickly from unforeseen events like demand surges or logistical bottlenecks.
What role does machine learning play in the digital twin model presented in the paper?
-Machine learning, specifically artificial neural networks (ANN), is used to predict uncertainties within the supply chain. The model analyzes data and optimizes system parameters to improve performance and reduce the impact of disruptions.
What are the primary benefits of adopting the digital twin model in production and distribution systems?
-The main benefits include real-time optimization of the supply chain, improved stock management, reduced inventory costs, and increased flexibility to adapt to sudden disruptions, ultimately leading to better fulfillment of demand and improved system performance.
What key performance indicators (KPIs) are used to measure the effectiveness of the digital twin model?
-The KPIs used include 'fill rate' (FR), 'backlog quantity', 'time to recover', and 'time to survive'. These indicators help assess the system's ability to maintain service levels and recover from disruptions.
What did the results indicate about the performance of the digital twin model compared to traditional strategies?
-The results indicated that the digital twin model outperforms traditional strategies in maintaining higher fill rates, reducing inventory levels, and recovering faster from disruptions. The model also demonstrated a better ability to handle unexpected demand fluctuations.
What challenges or limitations were identified in implementing the digital twin model?
-Challenges included high implementation costs due to the need for specialized infrastructure and technology, the complexity of calibrating parameters in large systems, and limited availability of compatible software and infrastructure for widespread industry adoption.
What future research directions or suggestions were proposed in the paper?
-Future research directions include expanding the application of digital twin models to other industries with different supply chain characteristics (e.g., food and beverage, automotive manufacturing), using more advanced machine learning algorithms for better responsiveness, and integrating IoT and blockchain technologies to improve real-time monitoring and transparency.
Outlines
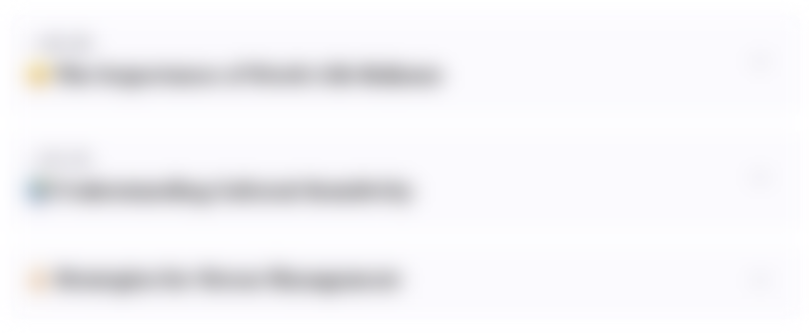
此内容仅限付费用户访问。 请升级后访问。
立即升级Mindmap
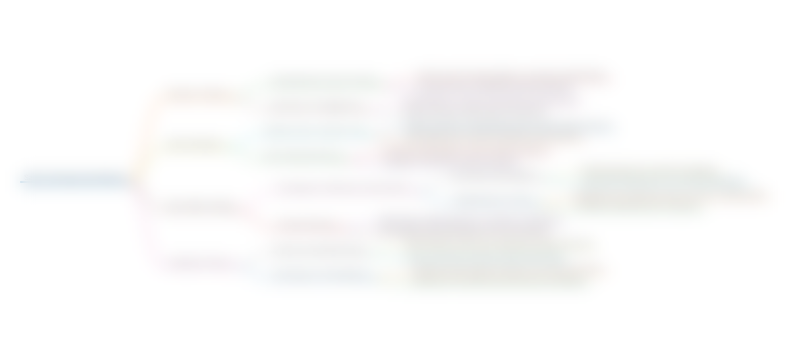
此内容仅限付费用户访问。 请升级后访问。
立即升级Keywords
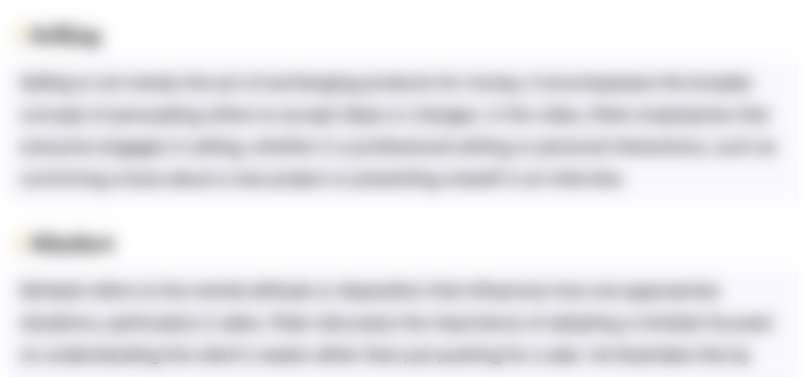
此内容仅限付费用户访问。 请升级后访问。
立即升级Highlights
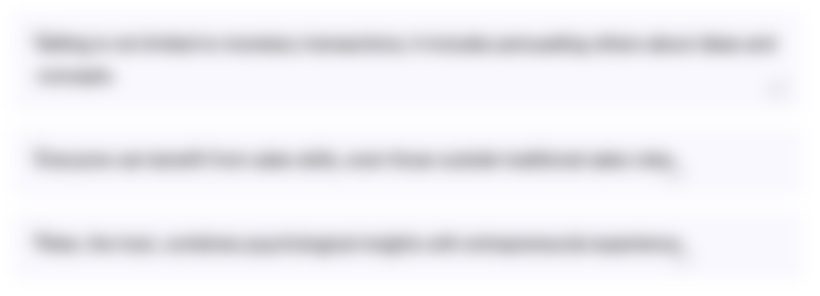
此内容仅限付费用户访问。 请升级后访问。
立即升级Transcripts
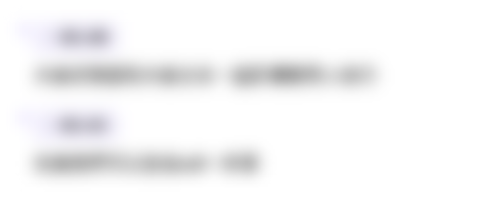
此内容仅限付费用户访问。 请升级后访问。
立即升级浏览更多相关视频
5.0 / 5 (0 votes)