Boosting Retail Margins: Price Optimization Strategies with Machine Learning
Summary
TLDRIn this presentation, Maya Brenner, a data scientist at Trial Labs, discusses how machine learning is transforming price optimization in retail. Through a case study with a luxury retailer, she demonstrates how data-driven models led to a 28% increase in gross margin by optimizing pricing strategies. The process involved dynamic pricing, forecasting demand, and using various data sources like sales, market, and economic data. Brenner emphasizes the importance of collaboration between business and data science teams and provides practical tips for implementing successful pricing models in real-world scenarios.
Takeaways
- 😀 Machine learning is transforming price optimization by helping retailers dynamically set prices based on historical data and customer demand.
- 😀 Traditional pricing approaches focused on supply and demand can be improved with ML models that predict the impact of price changes on sales.
- 😀 Two main approaches for price optimization in ML: automatic pricing, which mimics human pricing decisions, and price optimization, which focuses on maximizing profit or sales volume.
- 😀 A case study demonstrated how a luxury retailer achieved a 28% increase in gross margin by using ML to optimize prices based on sales forecasting and market data.
- 😀 Transactional data, rather than customer-level segmentation, is more important for training ML models to predict optimal pricing and demand.
- 😀 ML models for price optimization incorporate features such as seasonal trends, historical sales data, and external factors like currency exchange rates and market conditions.
- 😀 Time-series forecasting is a key component in price optimization, though traditional methods like ARIMA are not always used; instead, more advanced machine learning methods are employed.
- 😀 For businesses with limited data, ML models can still predict demand and optimize pricing by focusing on product-level features and trends.
- 😀 Hyperparameter validation for ML models in price optimization is often done using product-level data, while the training is done with similar products to increase model accuracy.
- 😀 Collaboration between data scientists and business experts is crucial to successfully implementing ML-based price optimization strategies, as each team brings essential expertise to the table.
- 😀 Networking opportunities, such as those provided by the Data Science Salon Slack channel, help professionals connect and share insights on emerging trends in price optimization and ML applications.
Q & A
What is the main focus of the price optimization process discussed in the presentation?
-The main focus of the price optimization process is leveraging machine learning to optimize pricing strategies based on historical transactional data, rather than customer segmentation. The goal is to predict demand and set optimal prices to maximize profit.
How does machine learning play a role in price optimization, and why is it preferred over classical methods?
-Machine learning algorithms, such as regression models, allow for more dynamic and accurate price optimization by considering a variety of factors, including trends, seasonality, and product similarities. This approach offers greater flexibility and predictive power compared to classical methods like ARIMA or other time series models.
What type of data is most important for price optimization, according to the speaker?
-Historical transactional data is the most important for price optimization. This includes sales data and units sold in the past, as it provides insights into demand patterns and helps predict future sales, which are crucial for adjusting prices effectively.
Can customer-level segmentation data be used for price optimization in this model?
-No, customer-level segmentation is not a primary focus for this price optimization model. The model is more concerned with transactional data—how many units have been sold in the past—rather than detailed customer segmentation.
What is the role of time series analysis in the price optimization model discussed?
-Although the model involves forecasting future sales, it takes a machine learning approach rather than traditional time series methods. The model considers time-dependent factors like lag features and seasonality but uses regression techniques instead of classical methods like ARIMA.
How does the model address the issue of limited data at the product level?
-The model uses a clustering approach to group similar products together when training. It trains the model with data from similar products, rather than using all available data, and validates the model using a specific product's data to ensure accuracy and robustness.
What are the advantages of using machine learning-based price optimization for a luxury retailer, as mentioned in the presentation?
-The luxury retailer in the case study saw a 28% increase in gross margin due to machine learning-based price optimization. This success was attributed to the model's ability to more accurately forecast demand and adjust prices dynamically, maximizing profits while maintaining market competitiveness.
What challenges might businesses face when implementing price optimization models?
-Challenges include the need for accurate and sufficient historical data, the complexity of incorporating business constraints (such as inventory levels or product substitutes), and ensuring that the model remains adaptable to changing market conditions.
How can data scientists and business professionals collaborate effectively in price optimization projects?
-Data scientists and business professionals should collaborate closely to ensure that the price optimization model takes into account both business goals and data-driven insights. Data scientists can provide technical expertise on the model, while business professionals can help define constraints and strategic objectives.
Why is a year's worth of data considered important for price optimization?
-A year's worth of data is essential to understand trends, seasonality, and other time-dependent factors that affect sales. This historical data allows the model to identify patterns and make more accurate forecasts for future sales, which is key to setting optimal prices.
Outlines
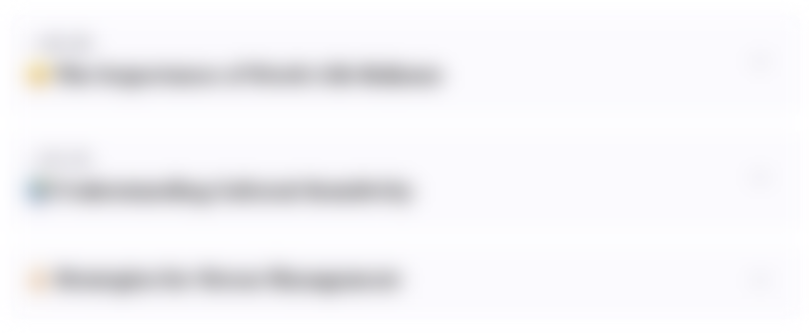
此内容仅限付费用户访问。 请升级后访问。
立即升级Mindmap
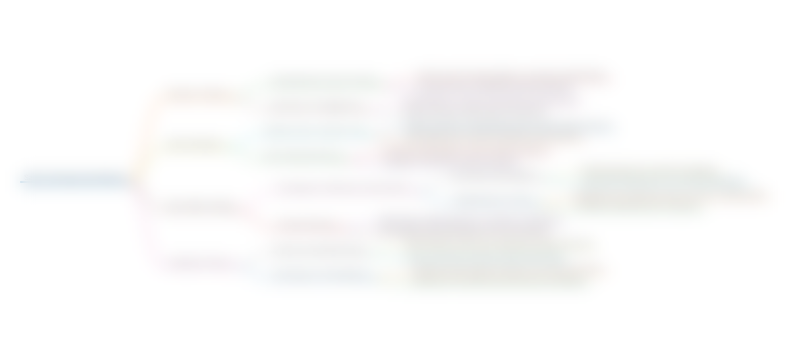
此内容仅限付费用户访问。 请升级后访问。
立即升级Keywords
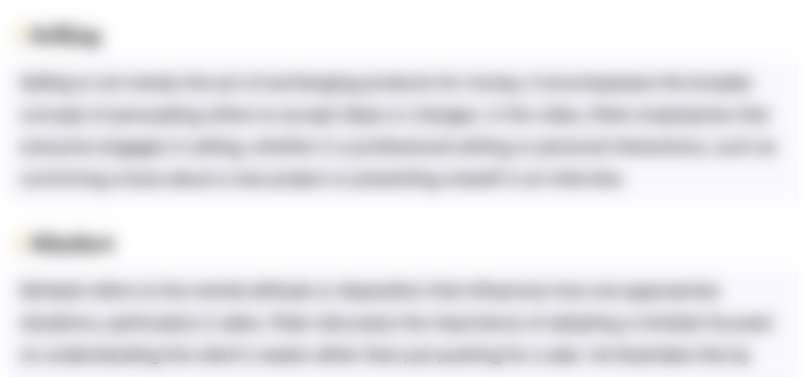
此内容仅限付费用户访问。 请升级后访问。
立即升级Highlights
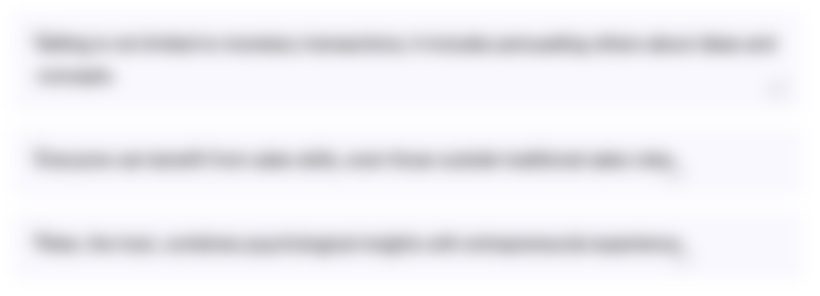
此内容仅限付费用户访问。 请升级后访问。
立即升级Transcripts
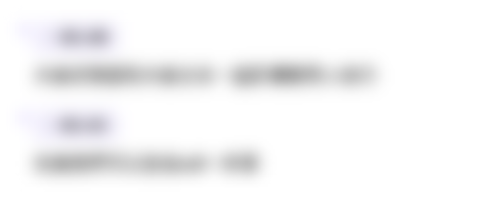
此内容仅限付费用户访问。 请升级后访问。
立即升级浏览更多相关视频

Machine Learning | What Is Machine Learning? | Introduction To Machine Learning | 2021 | Simplilearn
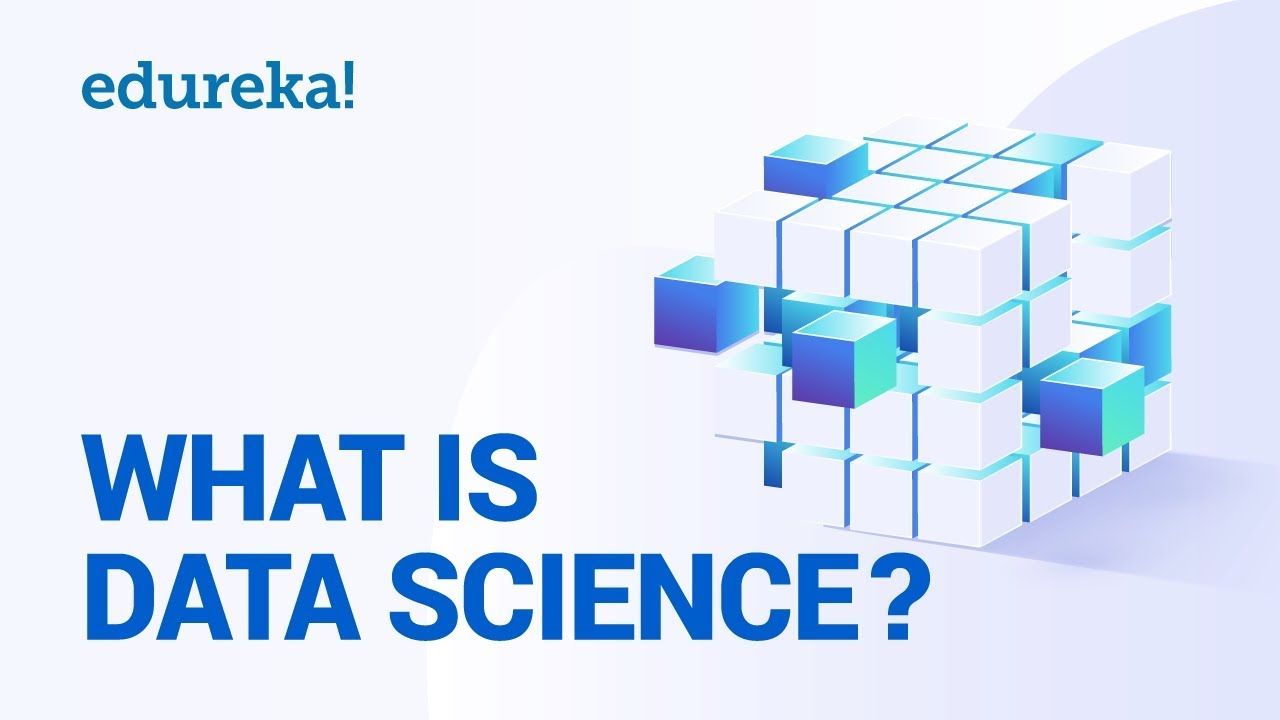
Data Science in 8 Minutes | Data Science for Beginners | What is Data Science? | Edureka
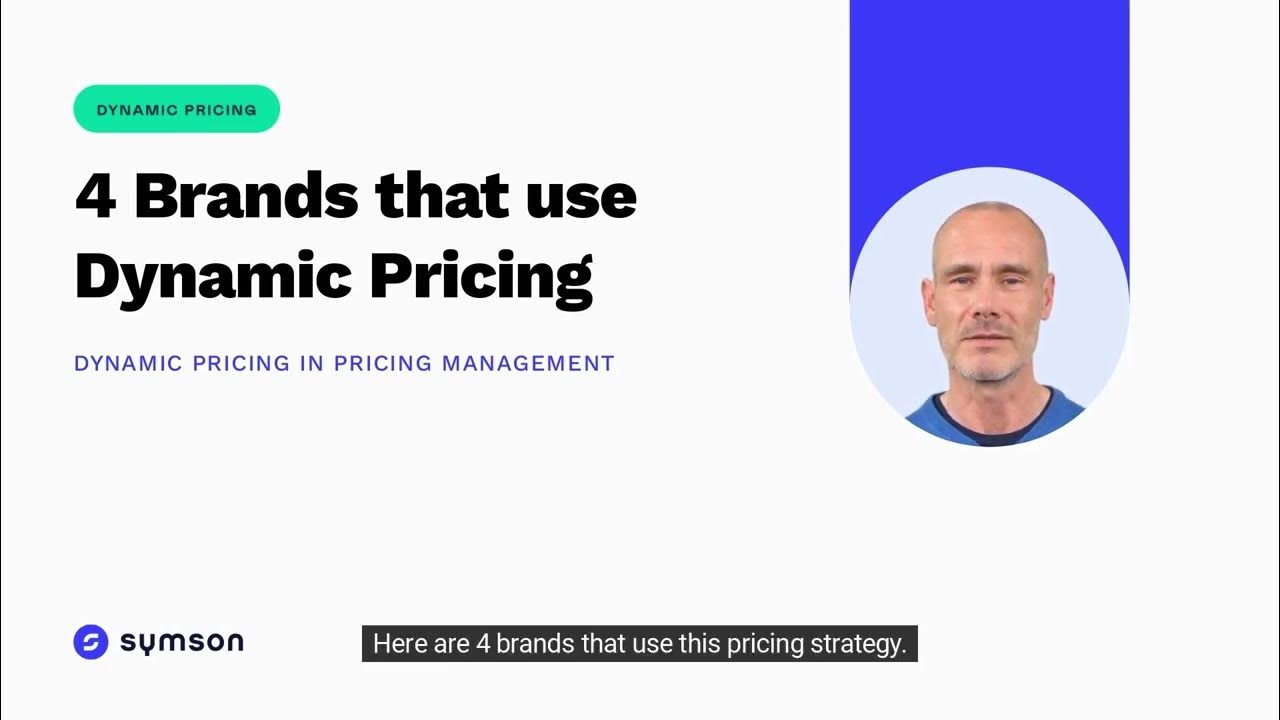
Examples of Dynamic Pricing: SYMSON's Knowledge Snippets
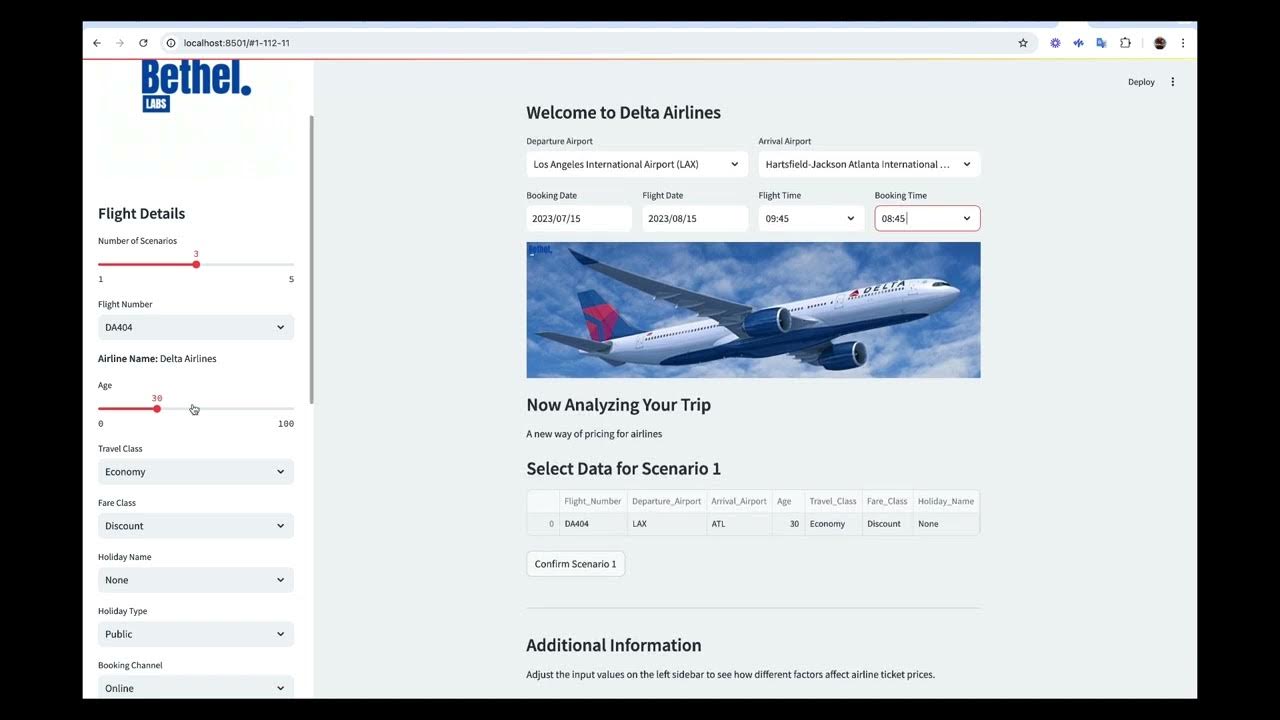
Data Science PROJECT: Airline Booking Demand Forecasting and Dynamic Pricing Optimization App
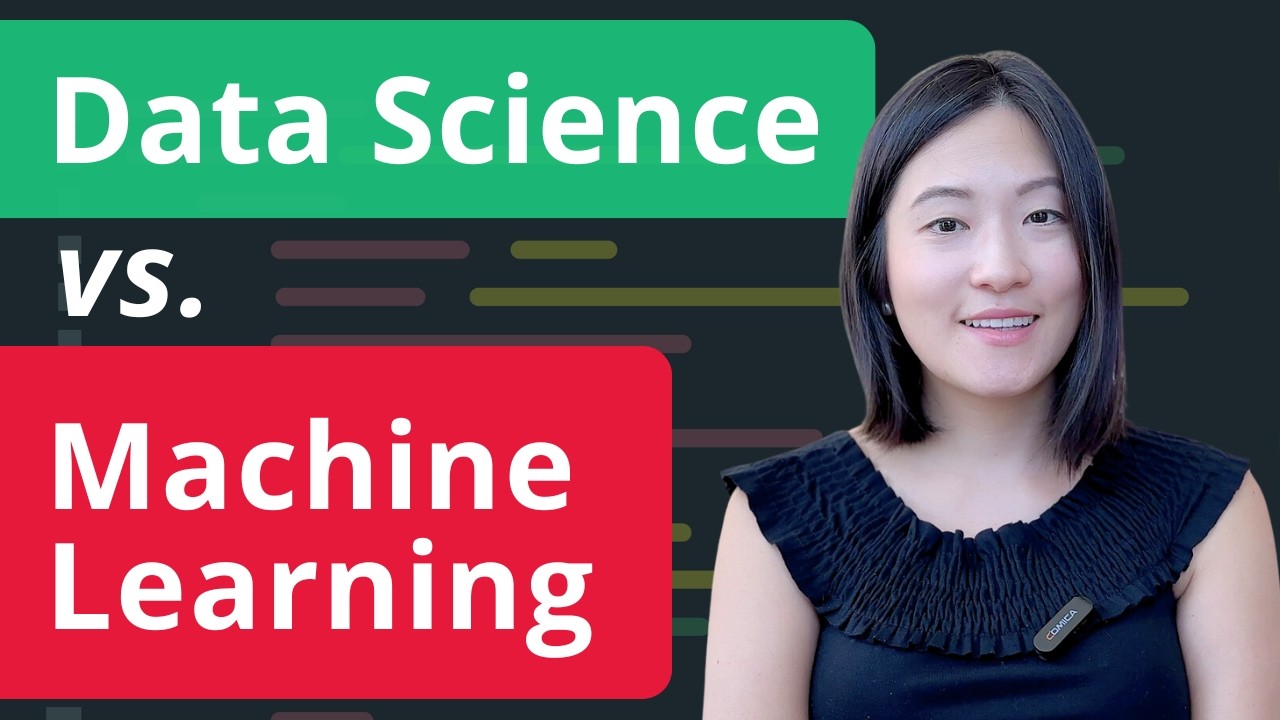
Data Science vs Machine Learning Engineer: Explained
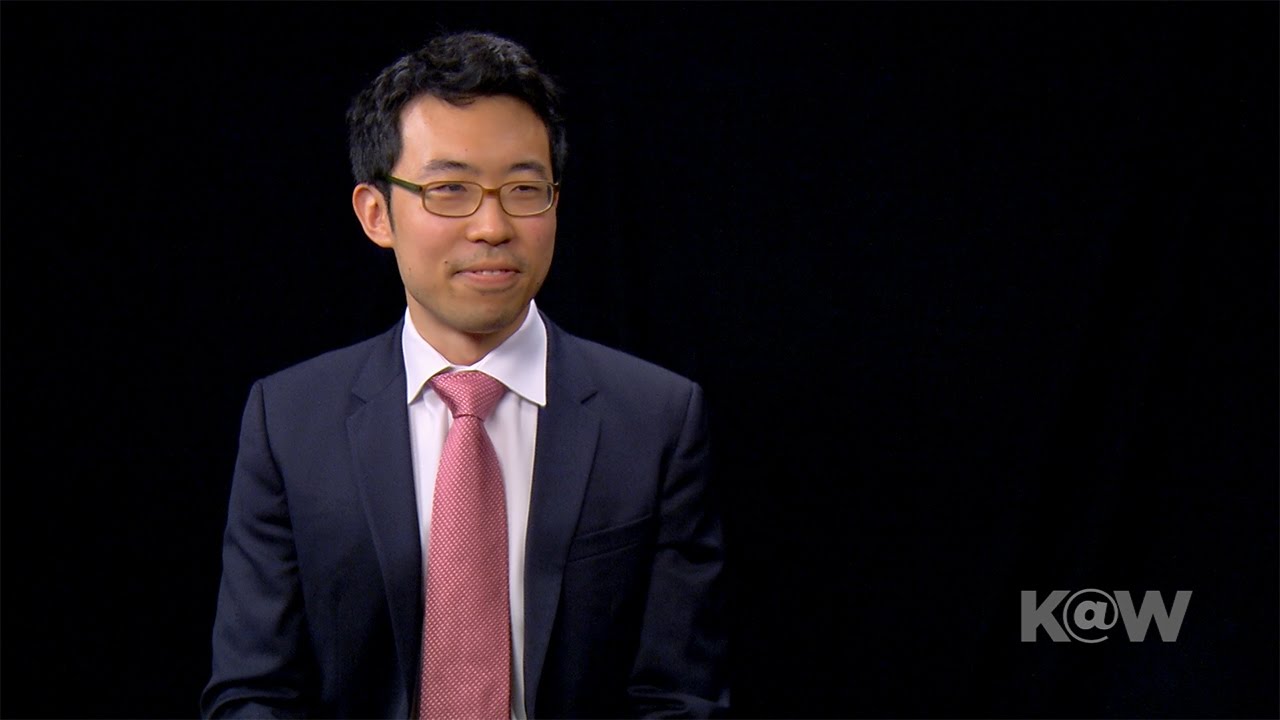
How To Turn Online Data Into a Pricing Strategy That Works
5.0 / 5 (0 votes)