NDC2.1 - Attractor Networks
Summary
TLDRIn this lecture on computational neuroscience, the focus is on the Hopfield model and its generalizations as attractor models. The instructor explains how these models enable neural networks to perform complex computations, such as retrieving stored patterns without a central processing unit. Key concepts include the use of orthogonal patterns, the calculation of neuron interactions, and the dynamics that lead to fixed points in the network's behavior. This fixed point indicates successful pattern retrieval, demonstrating the broader implications of attractor networks in understanding cognition.
Takeaways
- 😀 The lecture focuses on computational neuroscience, particularly the dynamics of cognition through neural models.
- 🧠 The Hopfield model is introduced as a means of understanding how neurons interact to perform complex computations.
- 🔍 The aim is to generalize the Hopfield model to align more closely with biological systems.
- 📊 Attractor models are defined as systems where the dynamics converge to specific states, facilitating pattern recall.
- 📏 The concept of overlap is crucial for measuring the similarity between stored patterns in neural networks.
- ⚙️ Orthogonal patterns are introduced, where the correlation between two patterns is zero, maximizing storage efficiency.
- 🔄 The dynamics of the network can lead to a fixed point, which signifies successful retrieval of stored patterns.
- 🎯 A fixed point is characterized by a high overlap with one of the stored patterns, ideally equal to one for orthogonal patterns.
- 🔊 The lecture emphasizes the broader applicability of attractor networks beyond the specific instance of the Hopfield model.
- 📚 The session concludes with a mention of an upcoming quiz, reinforcing the concepts discussed in the lecture.
Q & A
What is the primary focus of the class on computational neuroscience?
-The class focuses on neural dynamics of cognition, specifically generalizing the Hopfield model towards biological applications.
What model did the speaker use as an example last week?
-The speaker used the Hopfield model as an example of how interactions between neurons can perform complex computations.
What is the overall aim of the generalizations discussed in the lecture?
-The aim is to develop attractor models that enhance the understanding of biological processes in the brain.
What are attractor models in the context of this lecture?
-Attractor models are frameworks where the dynamics of the network converge towards a fixed point, representing the recall of specific patterns.
How does the Hopfield model store patterns?
-The Hopfield model stores patterns by encoding them in the weights of the neural network, with each pattern represented by a specific configuration of active and inactive neurons.
What is meant by orthogonal patterns in this context?
-Orthogonal patterns are those that have no overlap; their correlations are zero, meaning that they share as many pixels that are the same as they do different pixels.
How does the total input to a neuron get calculated in the Hopfield model?
-The total input is calculated by summing the interactions between the weights and the current states of the neurons, which is then passed through a sine function to determine the output.
What is the significance of the overlap measure in the model?
-The overlap measure indicates how similar the current state of the neurons is to stored patterns, influencing the dynamics of the network.
What happens to the overlap at the next time step according to the model?
-The overlap at the next time step may increase, leading the network dynamics towards a fixed point where the pattern is perfectly recalled.
How does noise affect the retrieval of patterns in attractor networks?
-Noise can cause variations in the overlap, potentially preventing perfect retrieval of patterns, but the network dynamics still tend to move towards a fixed point.
Outlines
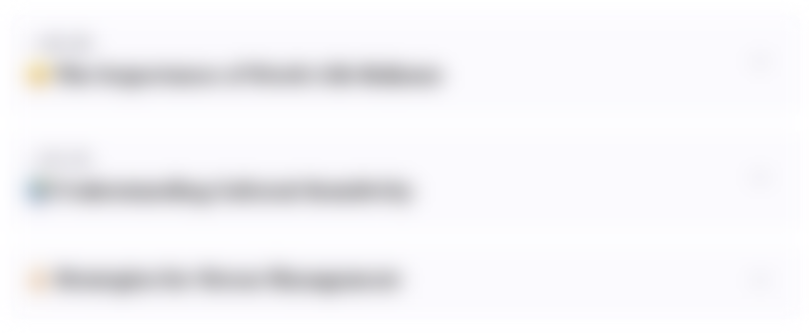
此内容仅限付费用户访问。 请升级后访问。
立即升级Mindmap
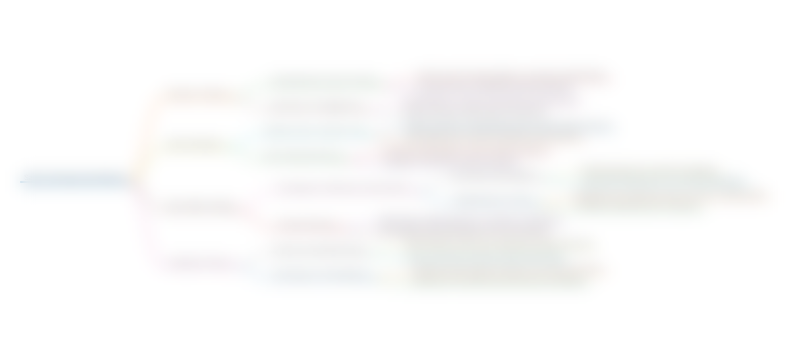
此内容仅限付费用户访问。 请升级后访问。
立即升级Keywords
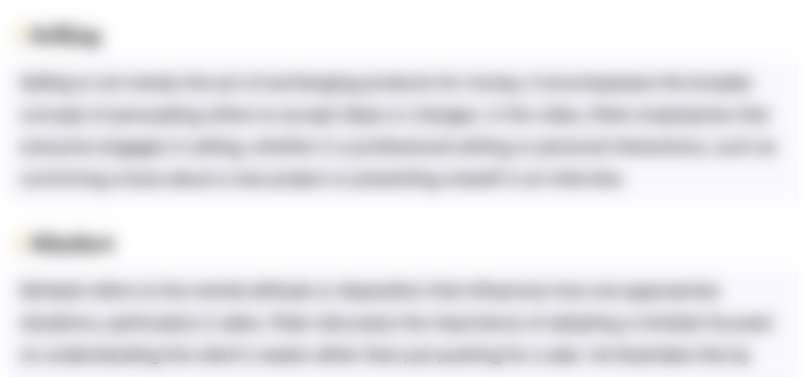
此内容仅限付费用户访问。 请升级后访问。
立即升级Highlights
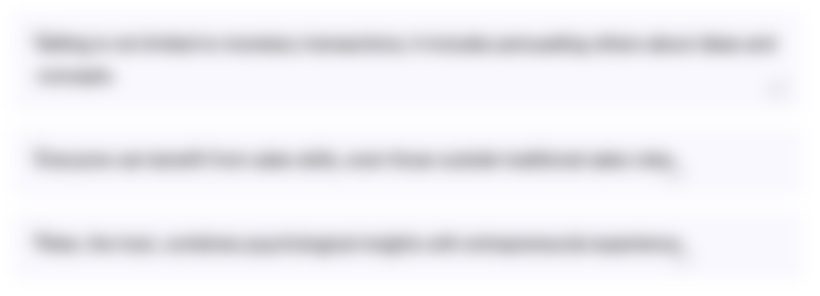
此内容仅限付费用户访问。 请升级后访问。
立即升级Transcripts
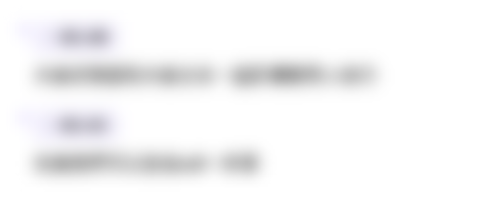
此内容仅限付费用户访问。 请升级后访问。
立即升级5.0 / 5 (0 votes)