Calling Bullshit 5.5: Criminal Machine Learning
Summary
TLDRThe video discusses the dangers of overfitting in big data, focusing on a controversial study that claimed to identify criminals by analyzing facial features. The instructor criticizes the flawed logic behind the study, highlighting biases in the training data and drawing parallels to outdated, discredited theories like Cesare Lombroso's 19th-century beliefs. The research is debunked for confusing facial structure with expressions, like smiling or frowning. This case serves as a cautionary example of how algorithms can perpetuate human biases, undermining the idea that computers are inherently objective or unbiased.
Takeaways
- 🤖 The lecture discusses a controversial study where researchers claim to predict criminality using facial recognition algorithms.
- 📅 This idea is compared to Cesare Lombroso’s 19th-century theories, which were discredited for being scientifically unsound and racially biased.
- 😨 The concept evokes fears similar to those found in dystopian narratives like Minority Report, where technology is used to predict criminal behavior.
- 👁️🗨️ The researchers argue that computers are unbiased in contrast to human judges, citing that algorithms don't carry emotional or subjective biases.
- 💻 However, the instructor argues that algorithms can inherit biases from the training data, which itself is based on biased human judgments.
- 📸 Non-criminals’ photos in the dataset were typically flattering, posed pictures, while criminal images were official, neutral, or serious photos, skewing the results.
- 👨⚖️ The instructor highlights that attractiveness affects human judgments in court, and algorithms could confuse facial features with expressions like smiling.
- 😁 The key flaw of the study seems to be that the algorithm may just be identifying smiles or neutral expressions, not actual criminal tendencies.
- 🔍 This misidentification is attributed to the lack of distinction between facial structure and facial expression in the training data.
- 📊 Overall, the story is used to illustrate the dangers of 'big data hubris,' where assumptions about the neutrality of data or algorithms can lead to flawed conclusions.
Q & A
What is the main example used by the instructor to illustrate the issue of overfitting?
-The instructor uses a study where researchers claimed they could develop an algorithm to determine if someone is a criminal based on their facial features, which gained significant media attention but exemplified overfitting.
Why does the instructor compare the modern algorithm with Cesare Lombroso's 19th-century ideas?
-The instructor draws a comparison to Lombroso's debunked theory that criminality could be determined by physical appearance, arguing that the modern algorithm is essentially trying to rescue Lombroso’s idea with machine learning, but similarly fails.
How do the researchers of the modern study claim their algorithm is unbiased?
-The researchers claim their algorithm is unbiased because it is driven by computer vision, which supposedly lacks human subjective biases, emotions, and fatigue.
Why does the instructor call the claim that computers are unbiased 'bullshit'?
-The instructor argues that the algorithm is as biased as the training data fed into it, which comes from humans who are inherently biased, meaning the results will reflect those biases.
What is one of the biases the instructor mentions that affects the training data?
-One bias mentioned is that more attractive people are less likely to be convicted of crimes, meaning the training data may reflect attractiveness biases rather than actual indicators of criminality.
What does the instructor believe the algorithm is detecting instead of criminality?
-The instructor suggests that the algorithm is actually detecting facial expressions, specifically smiles or frowns, rather than criminality, because it appears to confuse facial structure with expressions.
How does the instructor test the hypothesis that the algorithm is detecting smiles?
-The instructor looks at the output of the algorithm and observes that the criminals in the photos are frowning, while the non-criminals are smiling, supporting the hypothesis that the algorithm is detecting smiles rather than criminality.
What is the significance of the photos used in the study according to the instructor?
-The instructor highlights that non-criminal photos are often carefully chosen or posed (e.g., from Facebook or corporate headshots), whereas criminal photos are official documents (e.g., passports), which could introduce bias based on the conditions under which the photos were taken.
What is 'big data hubris' as illustrated by this example?
-Big data hubris is the overconfidence in the power of algorithms and large datasets to reveal objective truths, as seen in this case where researchers believe they can detect criminality without realizing their algorithm is biased by flawed data.
What is the overall lesson that the instructor wants to convey with this example?
-The lesson is that algorithms are not inherently free from bias and that the quality and nature of the training data are crucial. Blindly trusting algorithms without scrutinizing their inputs and assumptions can lead to flawed conclusions.
Outlines
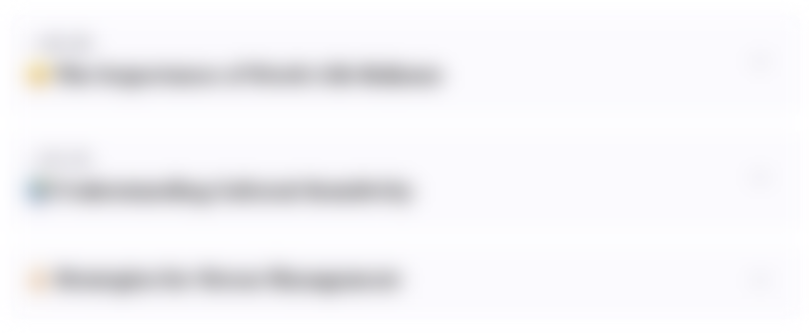
此内容仅限付费用户访问。 请升级后访问。
立即升级Mindmap
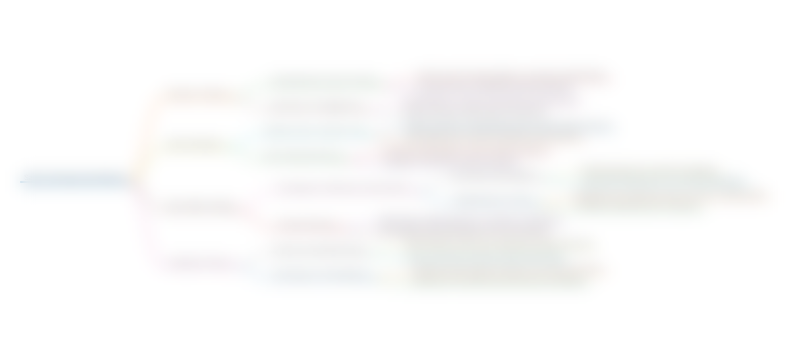
此内容仅限付费用户访问。 请升级后访问。
立即升级Keywords
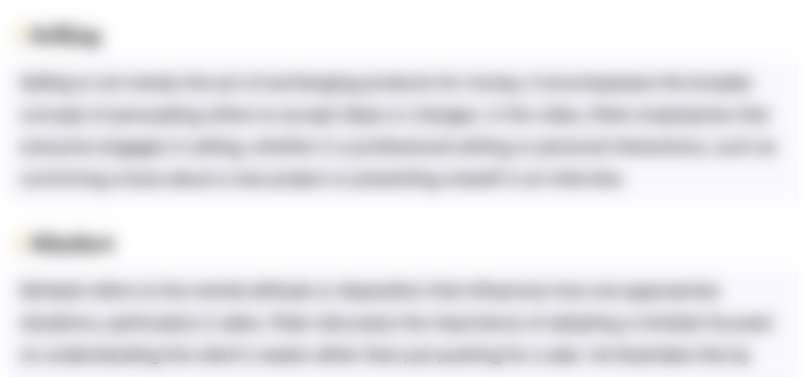
此内容仅限付费用户访问。 请升级后访问。
立即升级Highlights
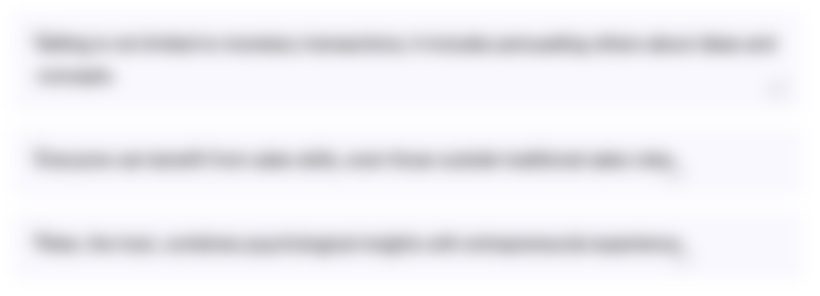
此内容仅限付费用户访问。 请升级后访问。
立即升级Transcripts
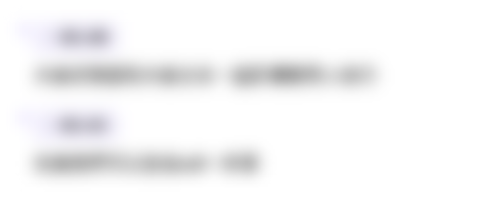
此内容仅限付费用户访问。 请升级后访问。
立即升级浏览更多相关视频
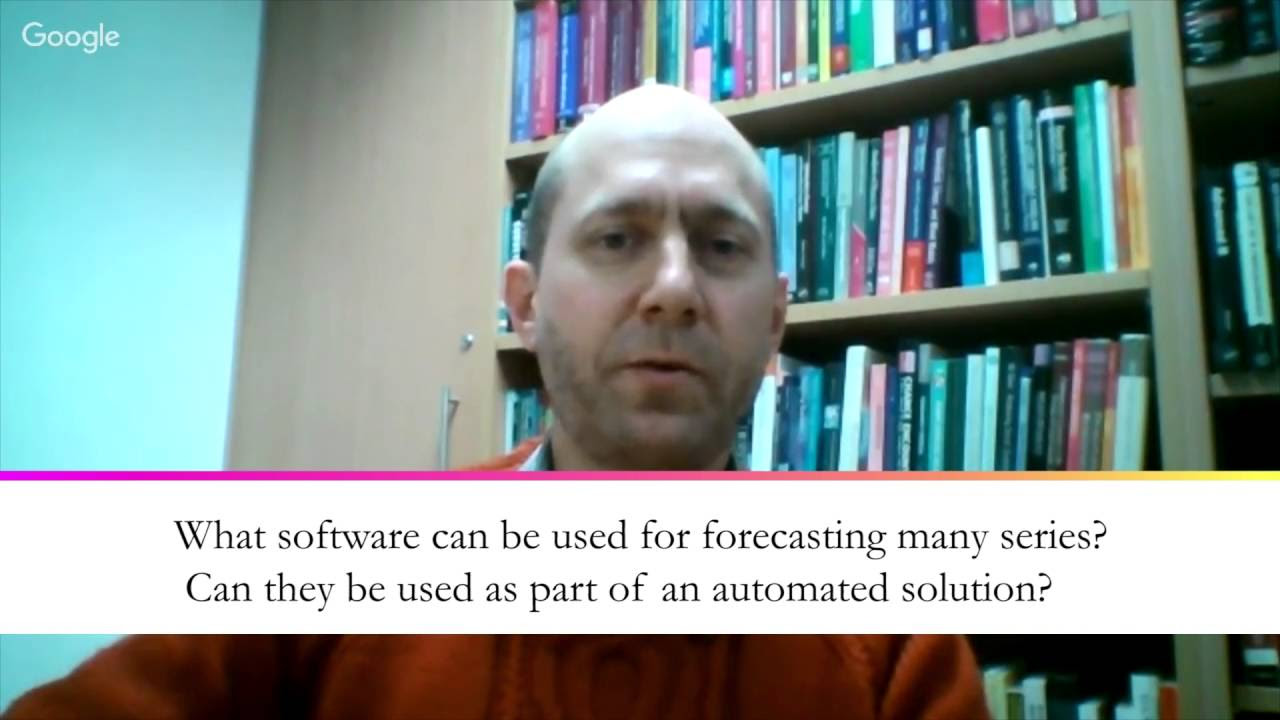
Forecasting and big data: Interview with Prof. Rob Hyndman
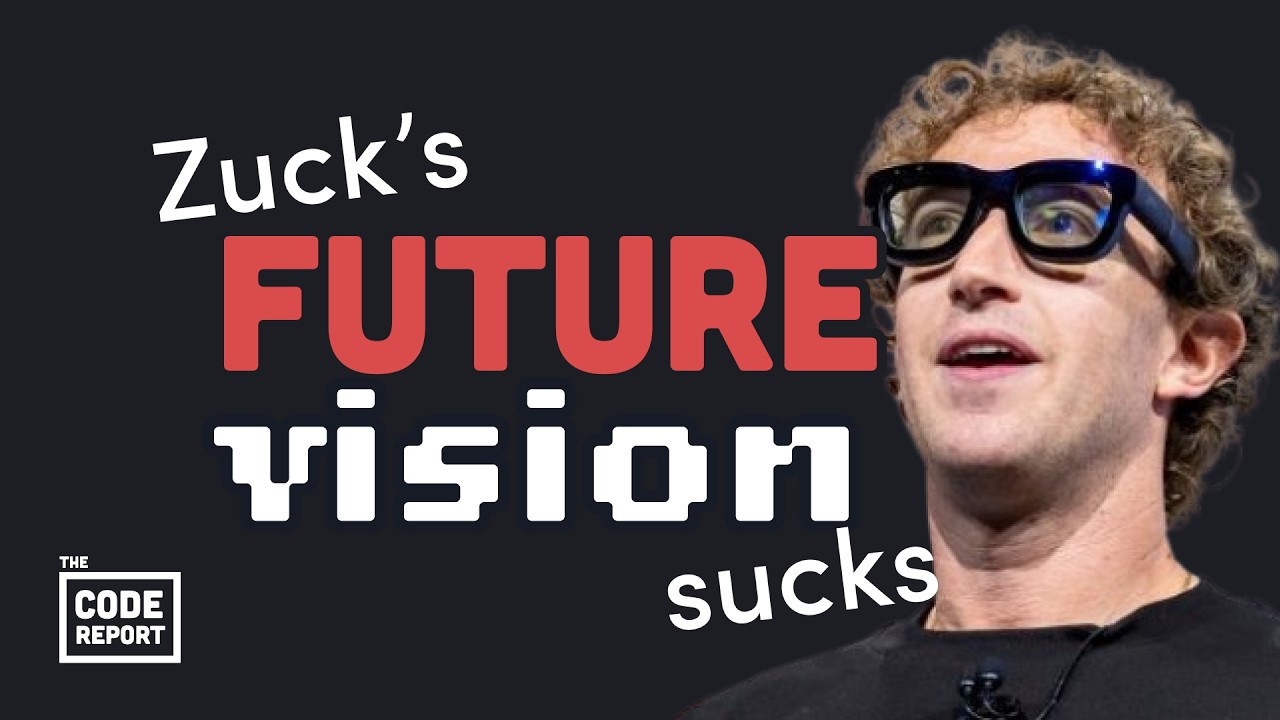
The most dystopian app ever made…
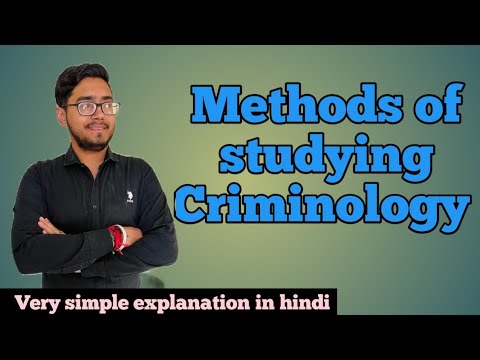
Method of studying of criminology | criminology for llb and Ballb | criminology by law with twins
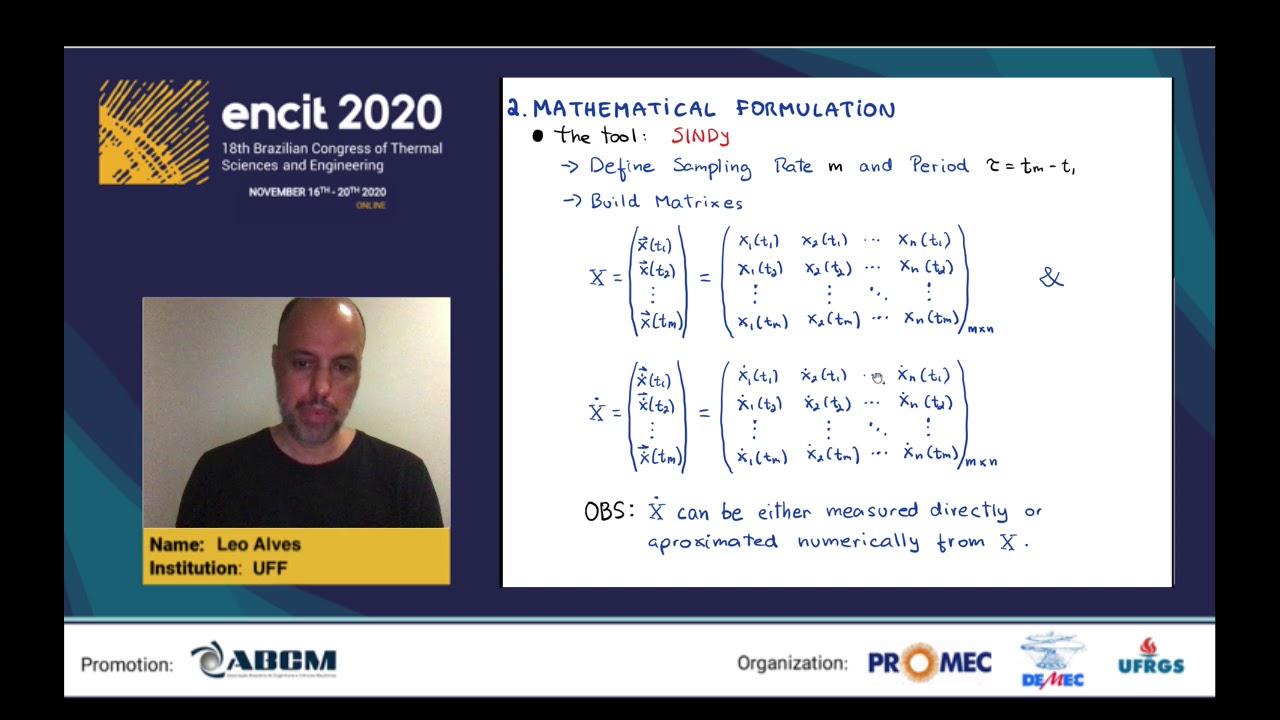
ADDRESSING OVERFITTING ISSUES IN THE SPARSE IDENTIFICATION OF NONLINEAR DYNAMICAL SYSTEMS

La zurda Bregman defendía delincuentes y le cerraron el OGT: "Preguntáles a los papás de Umma"
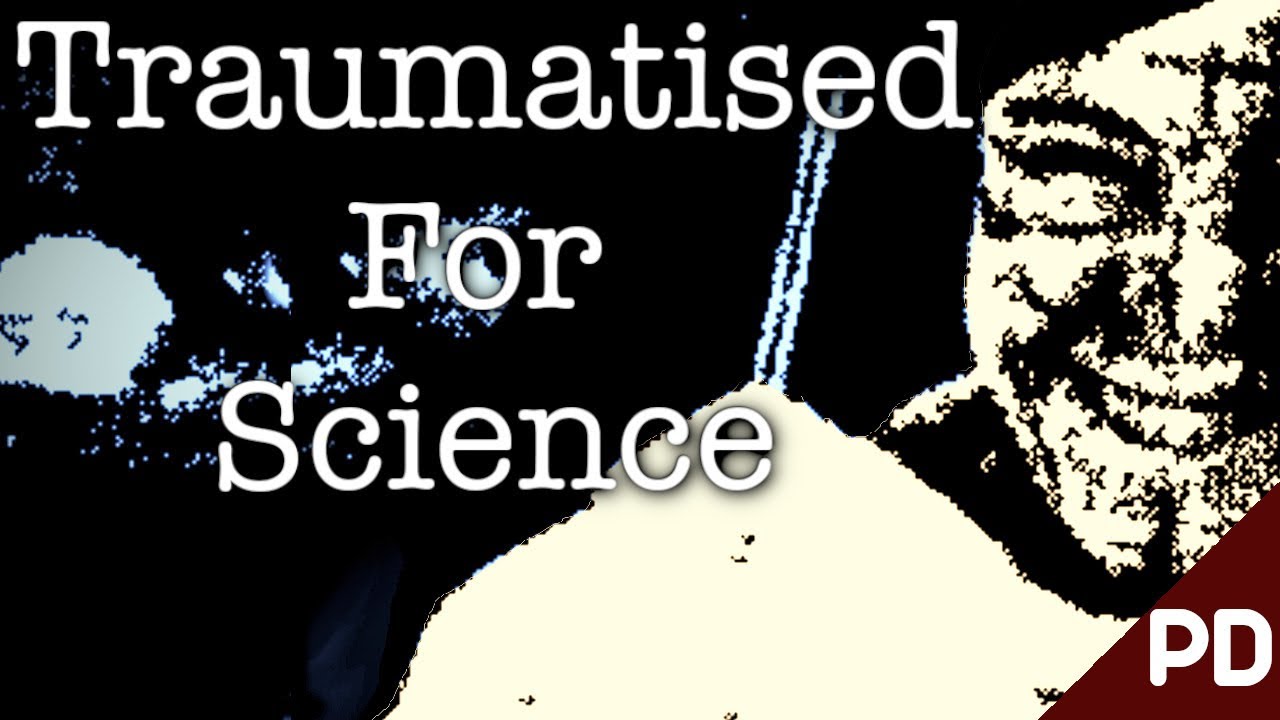
The Dark Side of Science: The Horrors of the Facial Expression Experiment 1924 (Short Documentary)
5.0 / 5 (0 votes)