Laura Schulz: The surprisingly logical minds of babies
Summary
TLDRIn this talk, Laura Schulz discusses the remarkable ability of children to make inferences and learn from limited data, drawing parallels between scientific reasoning and early cognitive development. Through experiments involving babies, she demonstrates how infants can generalize information and engage in causal reasoning based on statistical evidence. Schulz emphasizes that human cognition, especially in children, is incredibly powerful and fundamentally different from machine learning. She argues for greater investment in nurturing children's cognitive development, as they represent humanity's greatest learning potential.
Takeaways
- 🧠 The ability to learn quickly from sparse, noisy data is a key trait shared by both scientists and children.
- 📊 Babies can generalize knowledge from small, representative samples, just like scientists do with data.
- 🎲 Babies consider whether their evidence is randomly sampled or cherry-picked, which affects their expectations about the world.
- 🔵 In an experiment, babies generalized squeaking behavior to different colored balls, but only when the sample seemed randomly drawn.
- 👶 Babies use limited statistical data to reason causally, deciding between fixing a problem themselves or asking for help.
- 🧩 Babies’ ability to learn from small samples extends to a wide range of things, such as causal relationships, objects, and even language.
- 🤖 The speaker contrasts human cognition with machine learning, highlighting how human minds make complex inferences from minimal data.
- 👨👩👧 The speaker emphasizes the importance of investing in children's development, given their extraordinary learning abilities.
- 💡 The speaker challenges the notion that human minds are inherently flawed, pointing out that humans make countless correct decisions effortlessly.
- 👨🏫 The structured, hierarchical nature of human knowledge is a key challenge that sets human cognition apart from current machine learning capabilities.
Q & A
What was the central problem of cognitive science highlighted by Mark Twain’s quote?
-Mark Twain's quote humorously points out how science can make broad inferences from limited data. The central problem in cognitive science, as highlighted in the talk, is understanding how humans, especially children, can make accurate generalizations from small and noisy data.
How does the ability of children to generalize from sparse data relate to science?
-Children, like scientists, can draw rich, abstract inferences from limited and noisy data. This ability is key in both fields: scientists use small samples to predict large-scale phenomena, and children use small experiences to form expectations about the world.
What was the first experiment discussed, and what did it demonstrate about babies' reasoning?
-The first experiment involved showing babies two different statistical sampling conditions with blue and yellow balls. It demonstrated that babies are more likely to generalize properties (like squeaking) to other objects when the evidence appears randomly sampled, similar to how scientists assess evidence.
What does the result of the first experiment suggest about babies' understanding of probability?
-The experiment suggests that babies, as young as 15 months, understand the concept of probability. They can infer that if evidence seems cherry-picked, it might not represent the larger population, leading them to limit their generalizations.
What is the ‘problem of confounded evidence’ discussed in the second experiment?
-The problem of confounded evidence refers to the challenge of determining whether a failure (like a toy not working) is due to a flaw in the person using it or the object itself. This experiment tested whether babies could resolve this ambiguity using limited statistical data.
What were the key findings from the second experiment on causal reasoning?
-The experiment showed that babies use small amounts of evidence to decide between two different strategies: either asking for help (changing the person) or trying a new tool (changing the toy). This demonstrates their ability to make causal inferences from minimal data.
How does this research challenge the narrative of human cognitive biases?
-The speaker argues that, while humans are sometimes fallible and prone to biases, the bigger story is that human cognition is extraordinary. From a very young age, we make complex, accurate inferences and navigate the world successfully, which should be recognized as a remarkable achievement.
How does this research relate to the broader fields of neuroscience and machine learning?
-Although neuroscience and machine learning have made significant strides, particularly with large amounts of data, this research emphasizes the unique ability of human minds to learn and infer from small amounts of data, something machines and even modern neuroscience have yet to fully replicate.
What makes the computational power of a human child different from current technologies?
-Human children's minds are capable of making rich, structured inferences from minimal data. Unlike current technologies, which often rely on large datasets, human cognition involves hierarchical and structured knowledge that remains unmatched by machines.
What is the key takeaway from this research on children's learning for the future of innovation?
-The key takeaway is that human children's learning abilities are unparalleled, and investing in their development—through education and caregiving—is as important as investing in technological innovation. By supporting their growth, we are planning for a better future.
Outlines
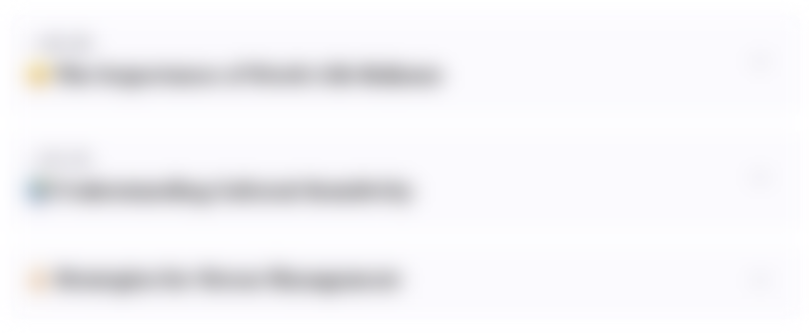
此内容仅限付费用户访问。 请升级后访问。
立即升级Mindmap
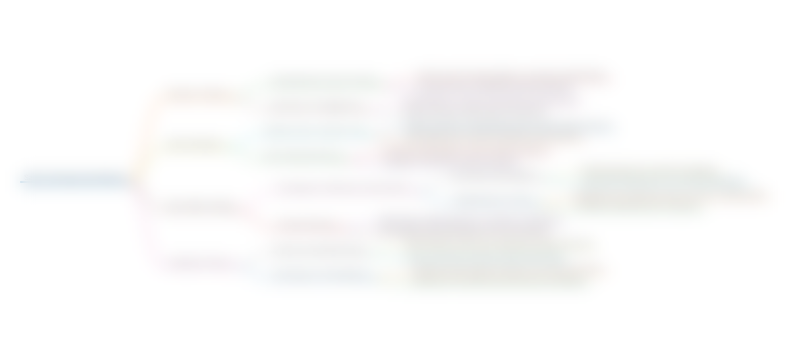
此内容仅限付费用户访问。 请升级后访问。
立即升级Keywords
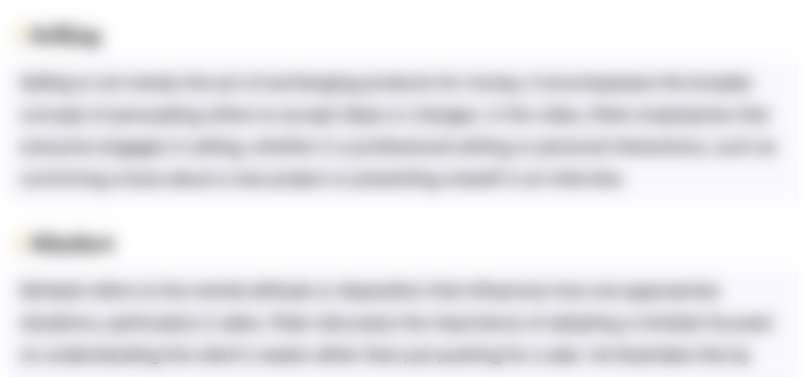
此内容仅限付费用户访问。 请升级后访问。
立即升级Highlights
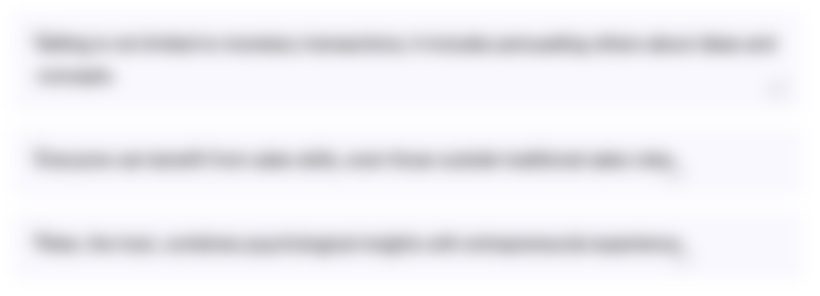
此内容仅限付费用户访问。 请升级后访问。
立即升级Transcripts
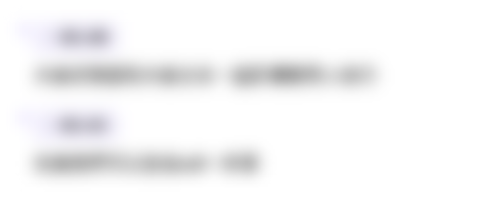
此内容仅限付费用户访问。 请升级后访问。
立即升级5.0 / 5 (0 votes)