The Future of Generative AI Agents with Joon Sung Park
Summary
TLDRIn a discussion about AI agents, Jun Zen Park provides background on the evolution of agents, from early assistive agents like Clippy to modern conversational agents. He outlines two branches of agent development: tool-based agents meant to automate complex tasks, and simulation agents that mimic human behavior. Large language models enable more advanced, personalized agents, though interaction challenges remain around deploying agents for high-risk tasks. Park sees initial success for agents in soft-edge problem spaces like games and entertainment before expanding to other areas. Though applications like ChatGPT show promise, he questions if conversational agents are the ultimate killer app compared to historic examples like Microsoft Excel.
Takeaways
- 😀 LLMs like GPT-3 made AI agents possible by providing the ability to predict reasonable next actions given a context
- 👥 There are two main types of AI agents - tool-based agents to automate tasks, and simulation agents to model human behavior
- 💡 LLMs still need additional components like long-term memory and planning for full agent capabilities
- 🎮 Games were an inspiration for early agent research aiming to create human-like NPCs
- 🚦 Current LLM limitations around safety and fine-tuning may limit the range of possible agent behaviors
- 🎭 Simulation agents for 'soft edge' problems like games and entertainment may succeed sooner than tool agents
- 🔮 Multimodal (text + image) agents are an exciting area for future research
- ❓ It's unclear if ChatGPT represents the 'killer application' for LLMs we expected
- 📚 Agent hype cycles have spiked and faded as expectations exceeded capabilities
- 🤔 Carefully considering human-agent interaction and usage costs will be key to adoption
Q & A
What was the initial motivation for Jun to research generative agents?
-Jun was motivated by the question of what new and unique interactions large language models like GPT-3 would enable. He wanted to explore if these models could be used to generate believable human behavior and agents when given a micro context.
How does Jun define 'tool-based' agents versus 'simulation' agents?
-Tool-based agents are designed to automate complex tasks like buying plane tickets or ordering pizza. Simulation agents are used to populate game worlds or simulations, focusing more on replicating human behavior and relationships.
What capability did large language models add that enabled new progress in building agents?
-Large language models provided the ability to predict reasonable next sequences given a micro context or moment. This could replace manually scripting all possible agent behaviors.
What does Jun see as a current limitation in using models like GPT-3 for simulation agents?
-Models like GPT-3 have been specifically fine-tuned to remain safe and not surface unsafe content. This limits their ability to accurately reflect a full range of human experiences like conflict.
Where does Jun expect agent technologies to first succeed commercially in the next few years?
-Jun expects agent technologies to first succeed commercially in 'soft edge' problem spaces over the next few years, like simulations and games. There is more tolerance for failure in these areas.
What does Jun see as a key open question around why previous periods of hype around agents failed?
-Jun wonders if past agent hype cycles failed because not enough thought was given to interactions - how agents would actually be used and whether they solved needs users really had.
What future line of questioning around large language models is Jun interested in pursuing?
-Jun wonders if ChatGPT represents the 'killer app' for large language models that people were waiting for. He thinks it's worth discussing whether ChatGPT is actually as transformational as expected.
How does Jun suggest thinking about future model architectures that could replace Transformers?
-Jun suggests thinking about Transformer capabilities as an abstraction layer - focusing on the reasoning capacity it provides. The implementation could be replaced over 5-10 years while still building useful applications today.
Where does Jun look for inspiration on new research directions?
-Jun looks to foundational insights from early Artificial Intelligence researchers that have stood the test of time. He believes great ideas are timeless even if hype cycles come and go.
What aspect of current agent capabilities is Jun most interested in improving further?
-Jun is interested in enhancing accuracy to better reflect real human behavior and diversity. This could enable personalized and scalable simulations grounded in real communities.
Outlines
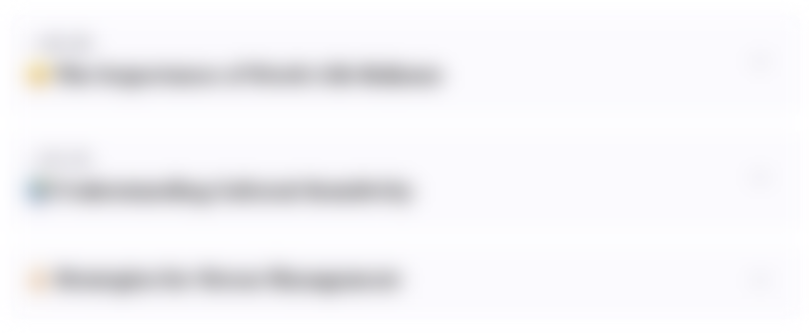
This section is available to paid users only. Please upgrade to access this part.
Upgrade NowMindmap
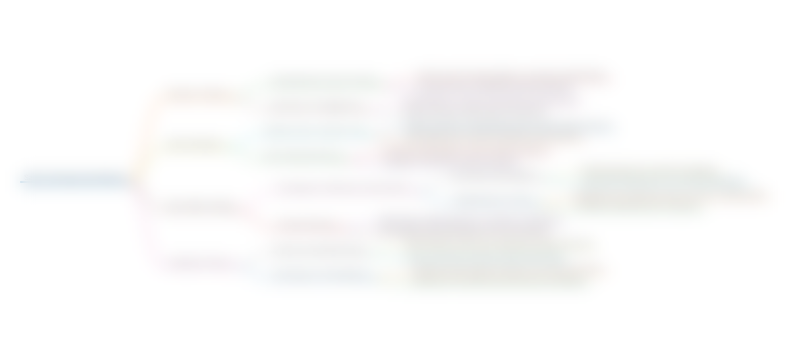
This section is available to paid users only. Please upgrade to access this part.
Upgrade NowKeywords
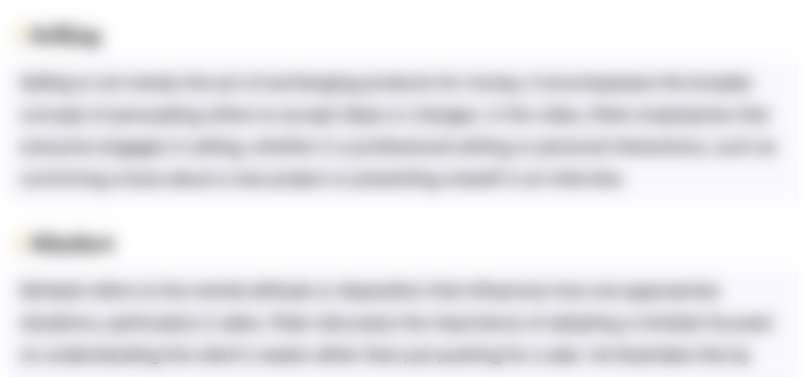
This section is available to paid users only. Please upgrade to access this part.
Upgrade NowHighlights
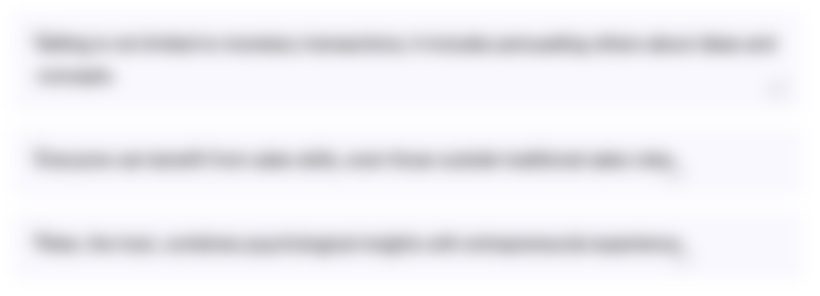
This section is available to paid users only. Please upgrade to access this part.
Upgrade NowTranscripts
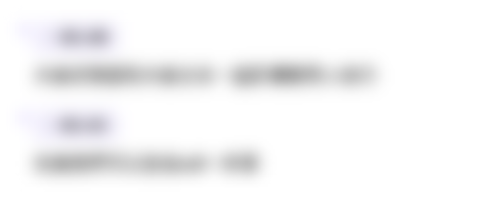
This section is available to paid users only. Please upgrade to access this part.
Upgrade NowBrowse More Related Video

Como Crear un Agente de IA Autónomo Sin Código - PASO A PASO
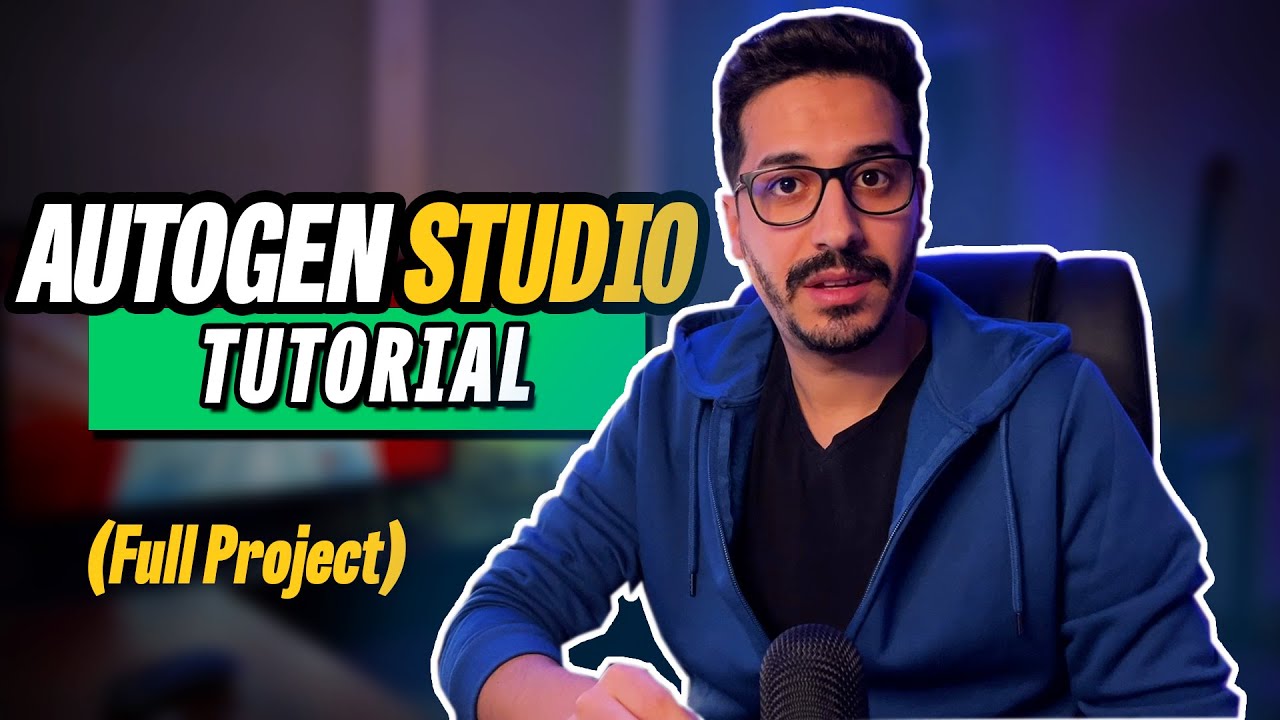
AUTOGEN STUDIO : The Complete GUIDE (Build AI AGENTS in minutes)
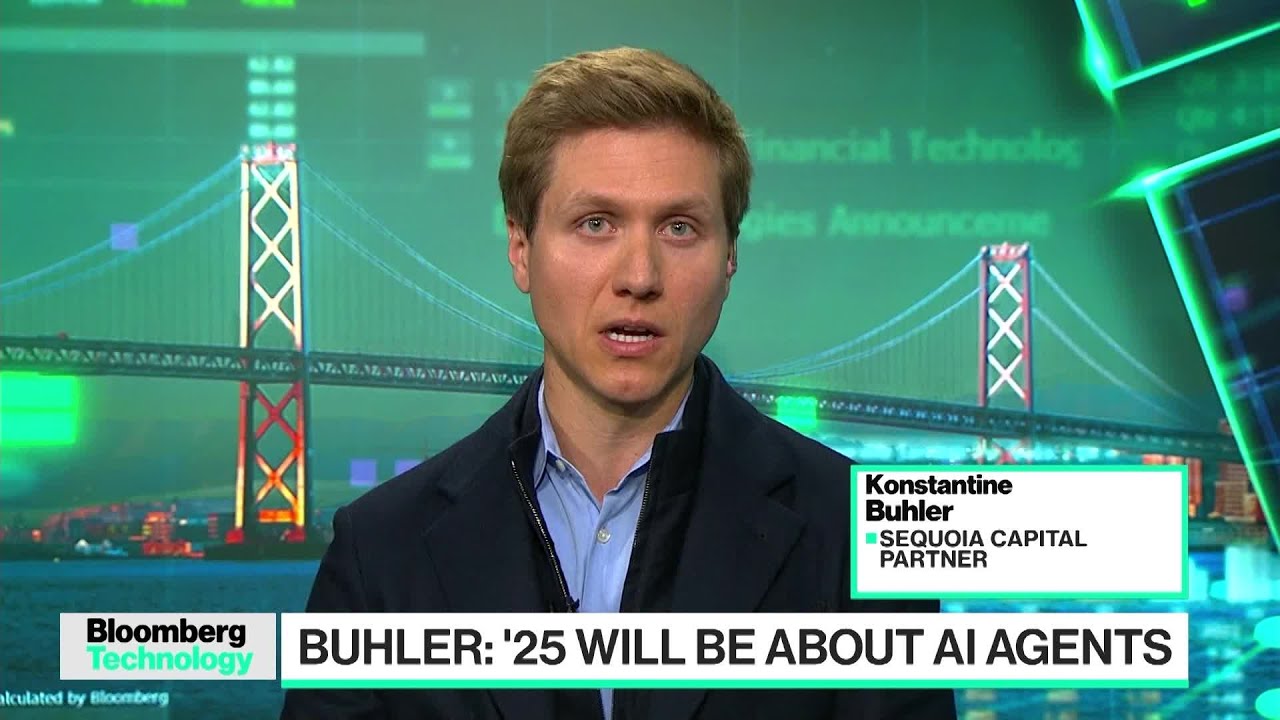
Why 2025 Will Be The Year of AI Agents

Taskade AI Agents: Build, Train, and Run Custom Agents for Any Role and Task Using Your Knowledge!
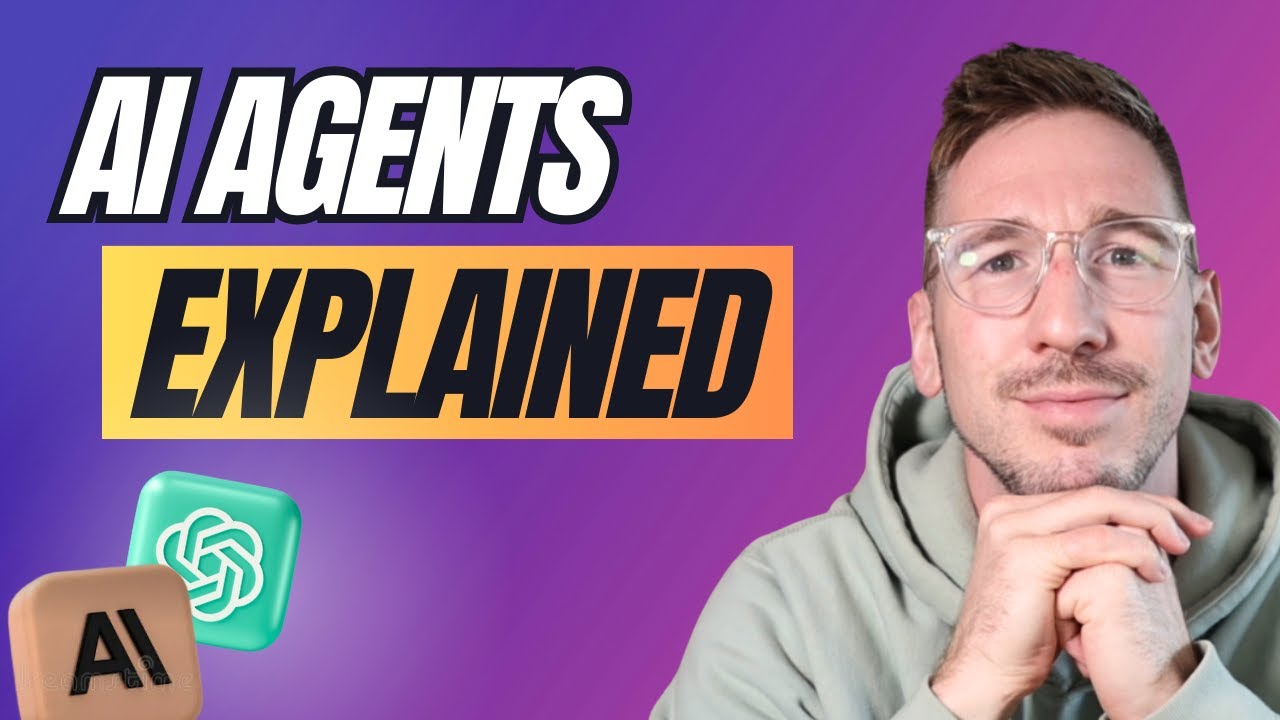
AI Agents Explained: A Comprehensive Guide for Beginners

Ep 1 OpenAI Agents SDK Introduction|Urdu|Hindi| Unicorn Developers – Muhammad Usman
5.0 / 5 (0 votes)