AI and Energy Storage – Making Batteries Predictable
Summary
TLDRこのビデオスクリプトでは、AIとエネルギー蓄積、特にリチウムイオン電池の予測可能性に関するトピックが取り上げられています。気候変動に伴うエネルギー移行と再生可能エネルギーの増加に伴い、蓄電池の必要性が高まります。AIを利用して蓄電池から得られるデータを分析し、安全性の警告、バッテリー寿命の推定、保証条件の自動チェックに活用することが議論されています。しかし、リソースの不足やバッテリーの多様性、製造過程のばらつき、内部状態の不明な性など、多くの課題があることが指摘されています。一方で、電圧、電流、温度などの容易に測定できる電気量から、バッテリーの内部状態を推定し、老化の原因や将来の老化予測に繋げることができます。これにより、バッテリーシステムの安全な使用、第二生命の活用、最適化された運用戦略が可能になるでしょう。
Takeaways
- 🌟 AIとエネルギー蓄積、特にリチウムイオン電池の予測性向上が、エネルギー遷移と気候変動対策において重要な役割を果たしている。
- 🔋 電池は住宅、移動性、産業、建築業など、多岐にわたる分野で使用され、データの収集と分析が可能である。
- 📈 再生可能エネルギーの増加に伴い、蓄電池の需要が高まり、リチウム業界では資源の不足が問題視されている。
- 🚀 電池の寿命を延ばすことでリソースの節約が図られ、第二ライフの活用が提案されている。
- ⏱️ 保証期間は現在、企業によって推定されており、実際の電池寿命は保証期間より短い場合がある。
- 🔍 電池の内部状態は複雑な電化学系であるため、直接評価が困難だが、電圧、電流、温度などの電気的量は簡単に測定可能である。
- 💡 BMS(バッテリー管理システム)から外部に流出するデータは、電池の老化や安全対策、第二ライフの活用、運用戦略の最適化に役立つ。
- 🛠️ 製造工程のばらつきや電池セルの多様性は、データのイントリンシックなばらつきを生み出す。
- 🌐 大量の電池セルが集まってシステムを形成するため、スケールの問題と製造工程の課題が存在する。
- 🔎 データ分析を通じて、電池の現在の状態推定、老化要因の分析、将来の老化予測が可能になり、安全性の向上や運用の最適化が期待される。
Q & A
AIとエネルギー蓄積、そしてバッテリーの予測可能性についての講演の主題は何ですか?
-講演の主題は、AIを利用してエネルギー蓄積とバッテリーの予測可能性を高めることで、より安全で効率的なバッテリーシステムを実現することです。
なぜ、気候変動に対処するためにはバッテリーが重要なのでしょうか?
-気候変動に対処するためには、エネルギー移行が不可欠であり、再生可能エネルギーの割合が増えると、その多くが間欠性のある源であるため、エネルギーの蓄積が不可欠になります。
リチウムイオンバッテリー蓄積システムが特に重要な理由は何ですか?
-リチウムイオンバッテリー蓄積システムは、技術的最前線であり、住宅、移動性、産業など多様な分野で応用が見込まれています。
バッテリーシステムが生成するデータはどのように役立つか説明してください。
-バッテリーシステムが生成するデータは、安全アラートの生成、バッテリー寿命の推定、保証条件の自動チェックなどに役立ちます。
バッテリーの使用において直面している課題は何ですか?
-リソースの大量消費、材料不足、バッテリー寿命の予測不確実性、および市場での保証期間と実際の寿命の不一致が課題です。
バッテリーのリソースを節約するためにどのようなアプローチが提案されていますか?
-既存のバッテリーシステムの寿命を延ばすことでリソースを節約し、バッテリーの2次使用を有効に活用することでリソースを再利用することが提案されています。
バッテリーシステムのデータはどのようにしてスケールアップに対処する必要がありますか?
-バッテリーシステムは多くのセルから構成され、製造過程でのばらつきや内部状態の不明了さから、スケールアップに伴うデータの多様性と複雑さを対処する必要があります。
バッテリーの内部状態を直接測定できない理由は何ですか?
-バッテリーは高度に複雑な電気化学システムであり、内部状態を直接測定することは困難であるため、電圧、電流、温度などの外部から測定可能な電力量を利用して推定する必要があります。
AIを用いてバッテリーの現在の状態を推定することはなぜ重要ですか?
-AIを用いてバッテリーの現在の状態を推定することは、バッテリーの老化の原因を分析し、将来の老化予測や安全な使用、2次使用の有効化、最適化された運用戦略につながるため重要です。
講演者はなぜ質問に答えることで終了するのか説明してください。
-講演者はスクリプトの内容を要約し、5分間のプレゼンテーションでできる限り情報を提供した後、聴衆からの質問に答えることで、より深い理解や視点を提供する機会を提供しています。
Outlines
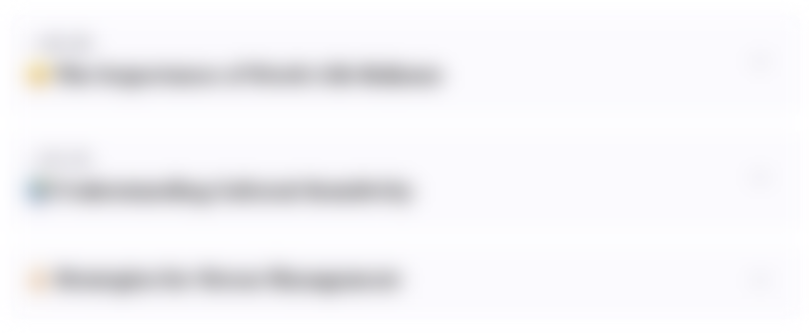
This section is available to paid users only. Please upgrade to access this part.
Upgrade NowMindmap
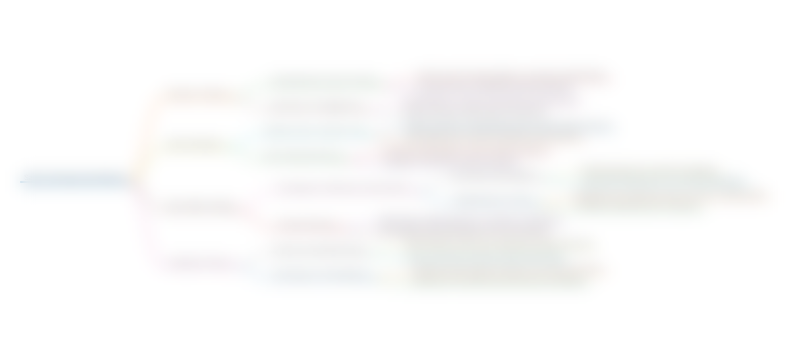
This section is available to paid users only. Please upgrade to access this part.
Upgrade NowKeywords
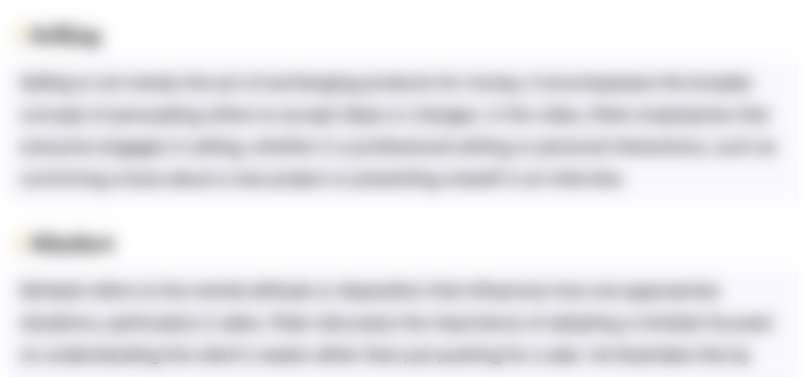
This section is available to paid users only. Please upgrade to access this part.
Upgrade NowHighlights
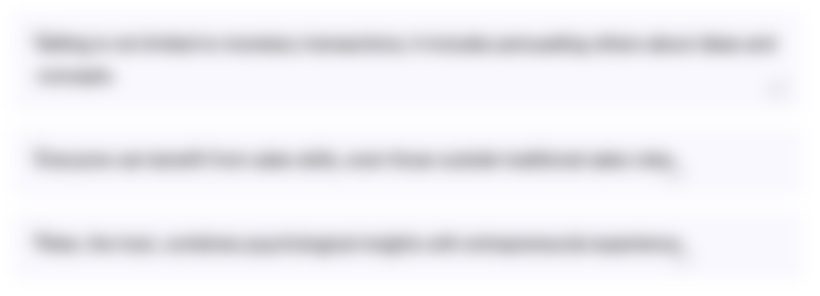
This section is available to paid users only. Please upgrade to access this part.
Upgrade NowTranscripts
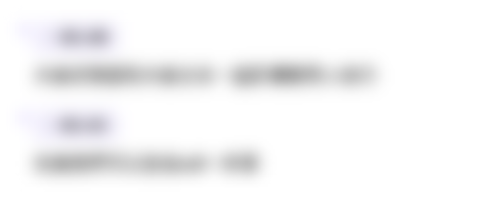
This section is available to paid users only. Please upgrade to access this part.
Upgrade NowBrowse More Related Video
5.0 / 5 (0 votes)