NVIDIA'S HUGE AI Chip Breakthroughs Change Everything (Supercut)
Summary
TLDRHuang unveils Nvidia's new Grace Hopper and H100 chips, representing a tipping point in accelerated computing and AI. Powering Nvidia's end-to-end AI platform, these chips enable breakthroughs in language models and vast improvements in performance and efficiency. Huang also announces partnerships and modular server designs to bring AI capabilities to the enterprise and every industry. Ultimately, he conveys a vision where AI empowers every company to become an 'intelligence producer', with AI factories fueled by Nvidia's accelerated computing platform.
Takeaways
- π² Nvidia has reached a tipping point in accelerated computing and generative AI.
- π©βπ» Software is now programmed by engineers working with AI supercomputers.
- π Accelerated computing is reinventing software from the ground up.
- π Nvidia GPU performance has increased 1,000x in 5 years.
- π AI supercomputers are a new type of factory for intelligence production.
- π Grace Hopper, Nvidia's new accelerated computing chip, is now in full production.
- π€― The new DGX GH200 AI supercomputer delivers 1 exaflop of AI performance.
- π Every data center will be re-engineered for accelerated computing.
- πΌ Nvidia AI Enterprise makes accelerated computing enterprise-grade.
- π Nvidia is extending accelerated computing and AI from cloud to edge.
Q & A
What are the two fundamental transitions happening in the computer industry according to Huang?
-The two fundamental transitions are: 1) CPU scaling has ended, so the ability to get 10x more performance every 5 years has ended. 2) A new way of doing software via deep learning and AI was discovered, reinventing computation from the ground up.
What is accelerated computing and how does it work?
-Accelerated computing uses GPUs and other specialized hardware to dramatically speed up workloads like AI, data analytics, and graphics. It allows much higher performance and efficiency than general purpose CPUs.
What is the Grace Hopper superchip?
-The Grace Hopper is Nvidia's new accelerated computing CPU chip for AI and high performance computing. It has nearly 200 billion transistors and high speed memory built in for efficiency.
What is the H100 and why is it important?
-The H100 is Nvidia's latest AI accelerator GPU. With advanced features like the Transformer Engine, it delivers giant leaps in AI performance to power the next wave of generative AI.
What is the goal of the Nvidia MGX system?
-The Nvidia MGX is a new open and modular server architecture optimized specifically for accelerated computing. It allows flexible configurations for AI and HPC workloads.
What does Huang mean when he says the computer is now the data center?
-He means that with the rise of cloud computing and AI supercomputers, the focus has shifted from optimizing single servers to building integrated data centers with networks of servers acting as one giant computer.
What is Spectrum-X?
-Spectrum-X is Nvidia's new high performance data center interconnect platform. It helps optimize AI supercomputing clusters using advanced networking and software.
How does Nvidia plan to bring accelerated computing to enterprises?
-Nvidia aims to make accelerated computing enterprise-ready through solutions like the MGX reference architecture and the Nvidia AI Enterprise software stack for managing AI infrastructure securely.
What is the benefit of using accelerated computing in the cloud?
-Accelerated computing in the cloud can provide up to 24x higher throughput and 20x lower cost compared to CPU-only platforms for workloads like image processing.
What role do Grace Hopper Superchips play in Nvidia's plan for AI supercomputing?
-Nvidia will use Grace Hopper Superchips as the base components to build exascale AI supercomputers made up of 256 connected GH200 systems, delivering over 1 exaflop/s of AI performance.
Outlines
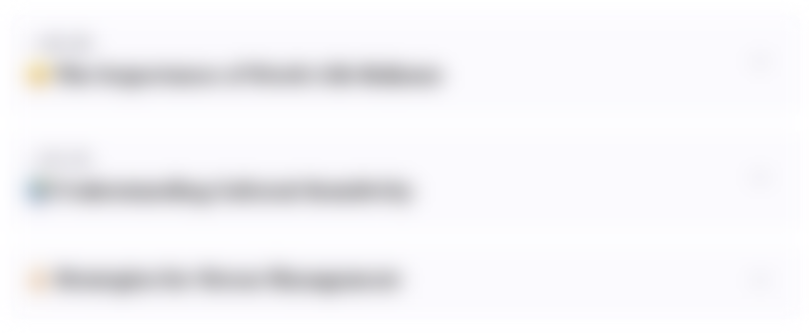
This section is available to paid users only. Please upgrade to access this part.
Upgrade NowMindmap
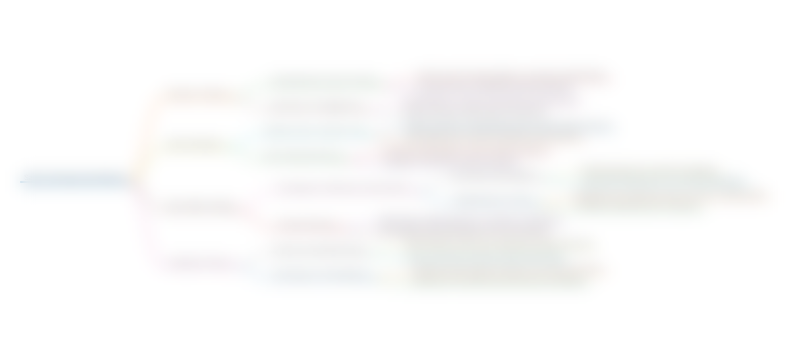
This section is available to paid users only. Please upgrade to access this part.
Upgrade NowKeywords
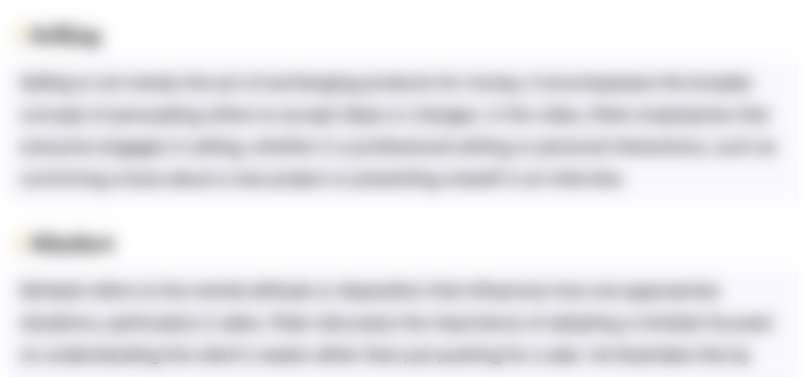
This section is available to paid users only. Please upgrade to access this part.
Upgrade NowHighlights
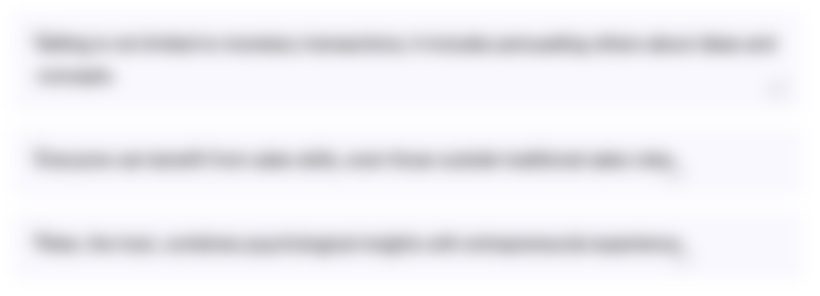
This section is available to paid users only. Please upgrade to access this part.
Upgrade NowTranscripts
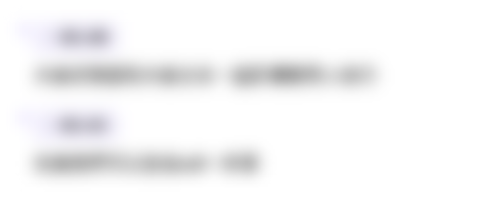
This section is available to paid users only. Please upgrade to access this part.
Upgrade NowBrowse More Related Video
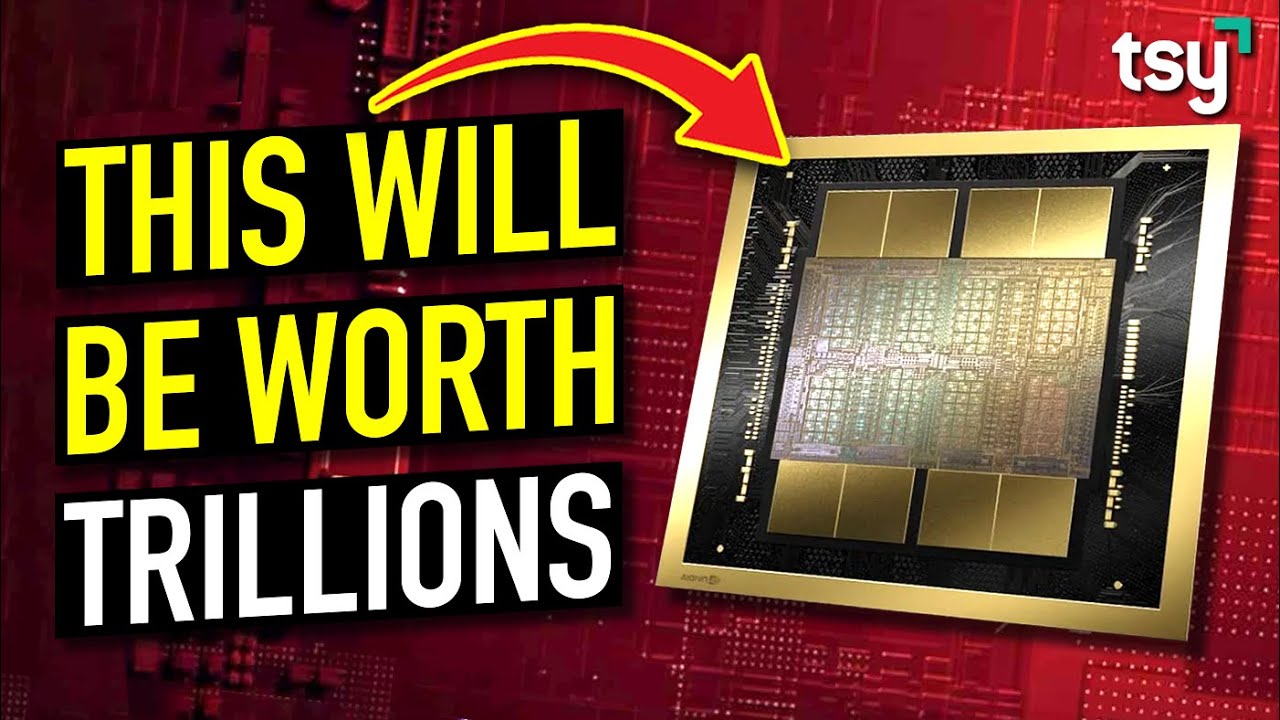
GET IN EARLY! I'm Investing In This HUGE AI Chip Breakthrough

Mooreβs Law is So Back.
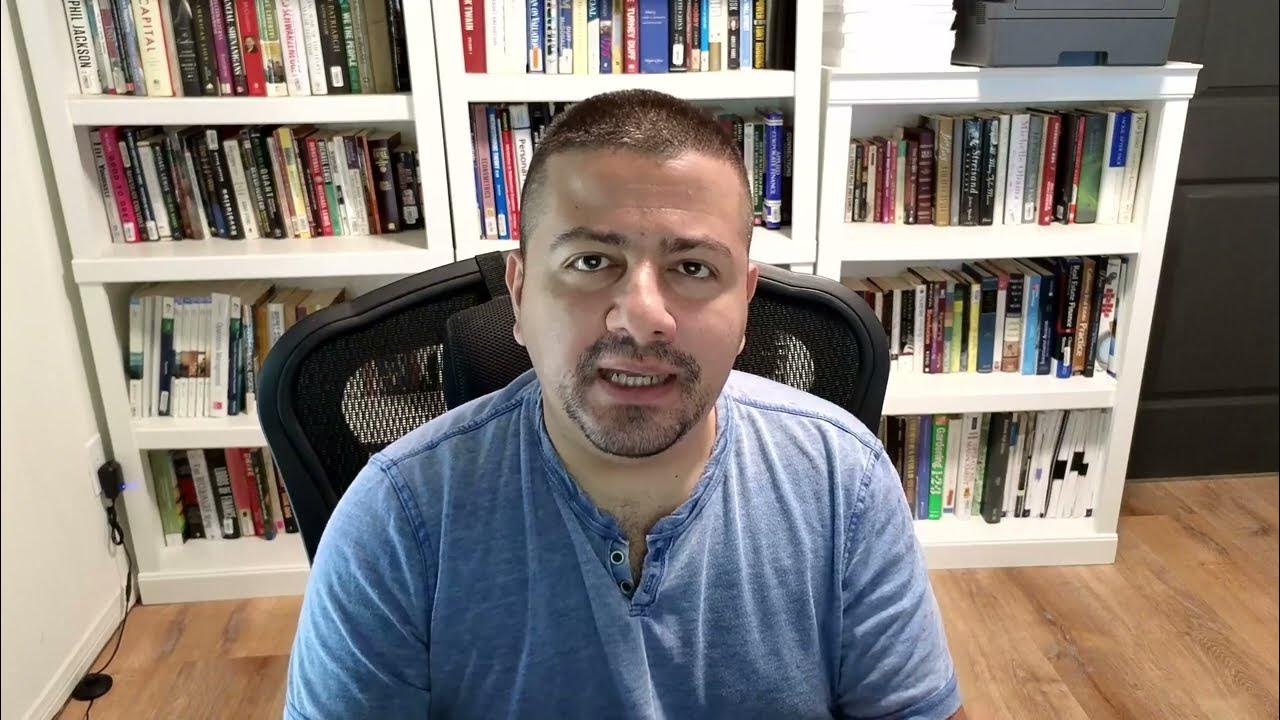
You Won't Believe What the Nvidia CEO Jensen Huang Just Said | NVDA Stock Analysis | Nvidia Stock
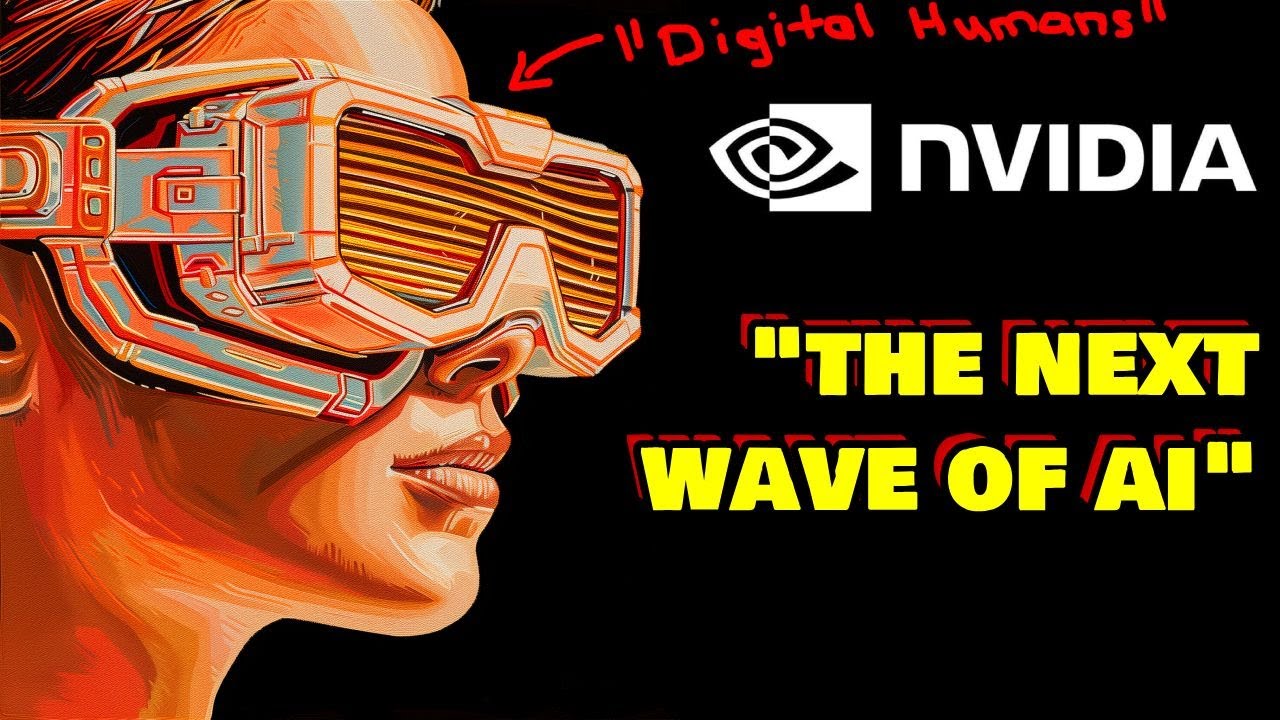
NVIDIA CEO Jensen Huang Reveals AI Future: "NIMS" Digital Humans, World Simulations & AI Factories.

Nvidia Founder and CEO Jensen Huang on the AI revolution
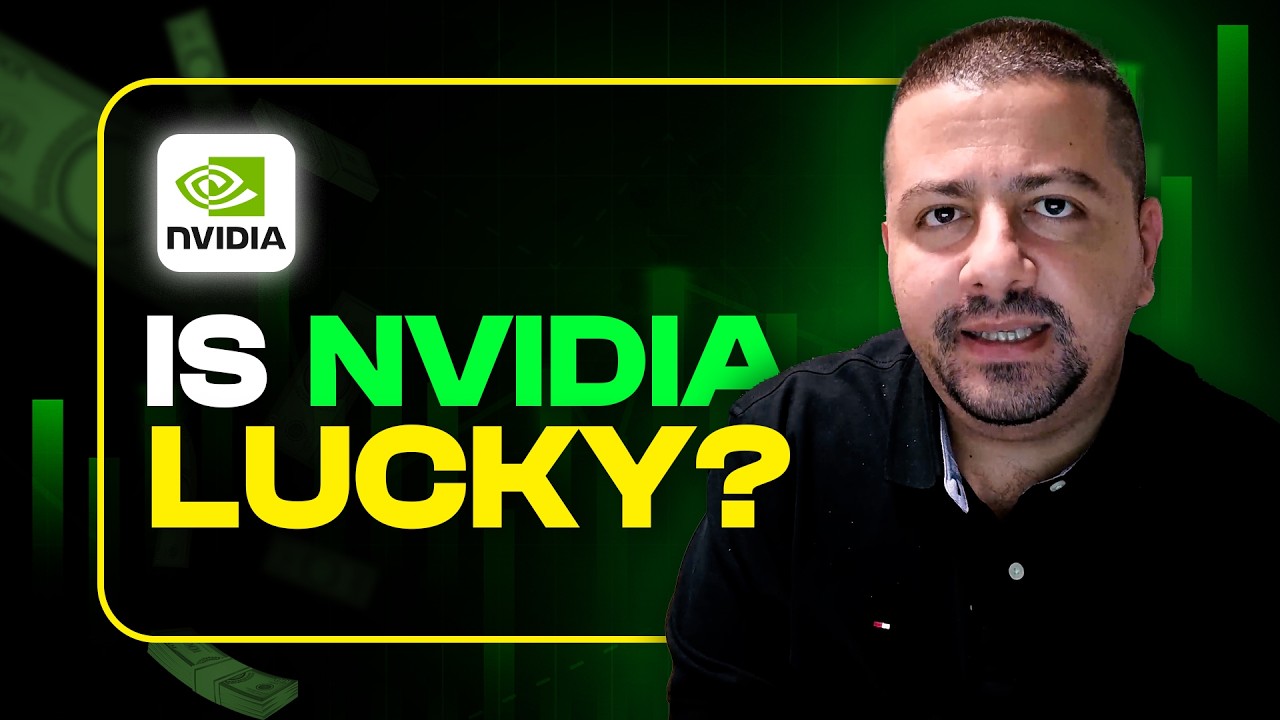
Nvidia Stock Has Soared 24,000% in 10 Years | NVDA Stock Analysis
5.0 / 5 (0 votes)