Will ChatGPT replace programmers? | Chris Lattner and Lex Fridman
Summary
TLDRThe speaker discusses the impact of large language models (LLMs) on programming, noting their ability to generate code that raises questions about the uniqueness of human thought and creativity. They highlight LLMs' proficiency in replicating common coding tasks but emphasize the importance of human collaboration and understanding of complex problems in innovation. The speaker is optimistic about LLMs as tools for automation and productivity, suggesting their potential in assisting with coding and even within compilers, while acknowledging the challenges of integrating them into existing systems.
Takeaways
- 🧠 Large language models (LLMs) are increasingly capable of generating code, raising questions about the uniqueness of human thought and creativity in programming.
- 🤖 LLMs can predict and generate code similar to what a programmer is about to write, which can make one wonder about the extent of human ingenuity in coding.
- 📈 The ability of LLMs to generate code is based on their extensive training on existing codebases available on the internet.
- 🔄 LLMs are good at solving standard coding problems because these problems are common and have been solved many times before.
- 🛠 Building innovative solutions involves more than just coding; it requires understanding the problem, working with people, and considering user needs.
- 🤝 LLMs are seen as tools to help automate repetitive tasks, allowing developers to focus on more complex and creative aspects of problem-solving.
- 🔧 LLMs can be a valuable companion in the coding process, helping to increase productivity but not replacing the need for human coders.
- 🌐 The discussion about LLMs generating code for new programming languages like Mojo highlights the potential for LLMs to learn and adapt to new languages.
- 🔑 LLMs do not require programming languages to be designed for them; they can learn and generate code in languages that are not machine-oriented.
- 🛑 While LLMs can help with coding, they may introduce uncertainties and errors, especially when the code needs to be precise and error-free for production.
- 🔮 There is potential for LLMs to be integrated into compilers or used in creative brainstorming, but the challenge remains in expressing clear intent for the machine to follow accurately.
Q & A
How are large language models impacting the nature of programming and thought?
-Large language models are generating code so well that they raise questions about the uniqueness of human thought and the source of valuable ideas in programming. They can predict code that a programmer is about to write, challenging the notion of individual ingenuity and innovation in coding.
What is the role of large language models in programming synthesis?
-Large language models are assisting in programming synthesis by automating the mechanical aspects of coding, such as generating boilerplate code and standard solutions to common programming problems.
How do large language models handle the task of learning from mistakes in programming?
-While the script does not directly address learning from mistakes, it suggests that large language models can generalize from instances found on the internet, which implies they could potentially learn from common mistakes made by programmers.
What is the significance of 'standing on the shoulders of giants' in the context of programming with large language models?
-The phrase 'standing on the shoulders of giants' is used to illustrate how large language models can help programmers by providing them with access to a vast amount of existing knowledge and code, thus accelerating the development process.
How do large language models assist in building applied solutions to problems?
-Large language models can aid in building applied solutions by automating routine coding tasks, allowing programmers to focus more on understanding the problem, working with people, and defining the product and its use cases.
What is the potential of large language models in the development of new programming languages?
-The script suggests that large language models could potentially be trained on the syntax and semantics of new programming languages, helping to generate code in those languages and even possibly assisting in their design.
How do large language models deal with the challenge of compilers' strict requirements?
-Large language models can help address the strict requirements of compilers by providing predictive coding assistance, ensuring that code adheres to the necessary syntax and structure before it is submitted for compilation.
What is the future potential of integrating large language models within compilers?
-While the integration of large language models into compilers is possible, it may currently be impractical due to the high computational costs associated with running large language models. However, on-device models and other technological advancements could make this integration more feasible in the future.
How can large language models tap into the creative potential of 'hallucinations' in programming?
-The 'hallucinations' of large language models refer to their ability to generate novel and creative outputs. While this can be beneficial for brainstorming and creative writing, it may not always be desirable in programming where correctness is paramount.
What are the implications of large language models for the future of coding and software development?
-Large language models are likely to continue to enhance productivity in software development by automating routine tasks and assisting with code generation. However, they are seen as a companion to human programmers rather than a replacement, with the potential to handle more complex reasoning and proof-based systems in the future.
How can large language models assist in expressing a programmer's intent in coding?
-Large language models can help express a programmer's intent by generating specifications or documentation that reflect the desired outcomes. This could be complemented by other systems that implement the actual code, ensuring that the final product aligns with the programmer's vision.
Outlines
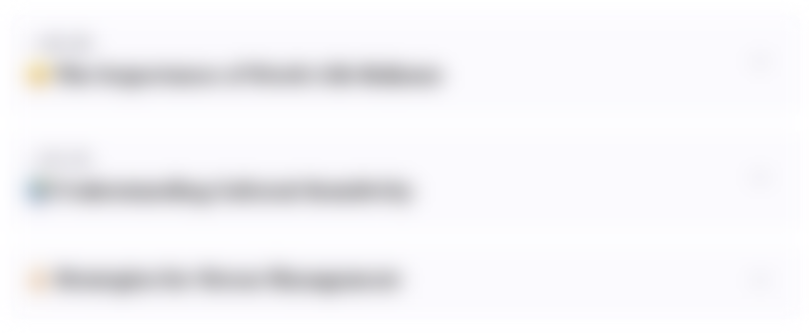
This section is available to paid users only. Please upgrade to access this part.
Upgrade NowMindmap
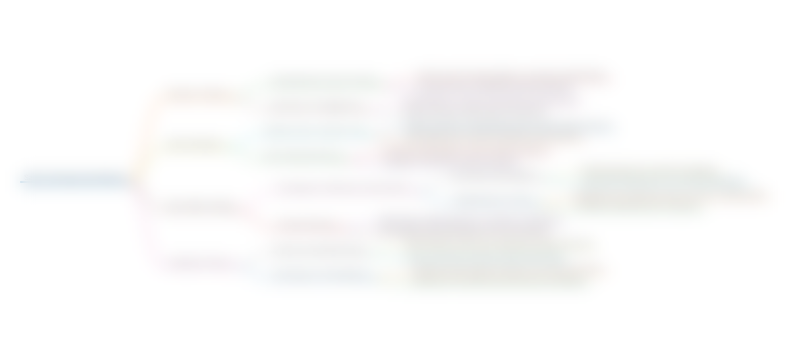
This section is available to paid users only. Please upgrade to access this part.
Upgrade NowKeywords
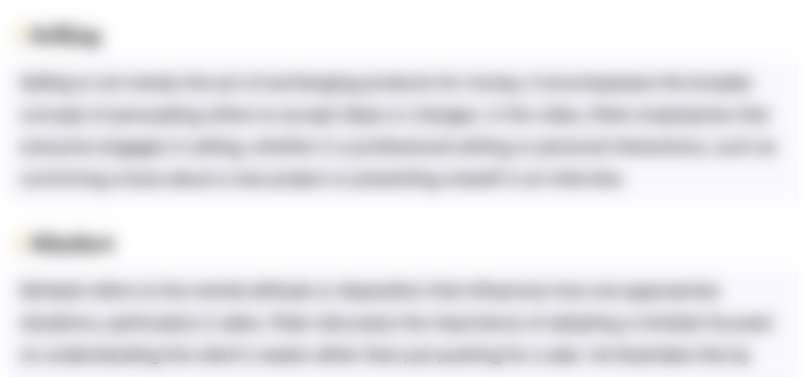
This section is available to paid users only. Please upgrade to access this part.
Upgrade NowHighlights
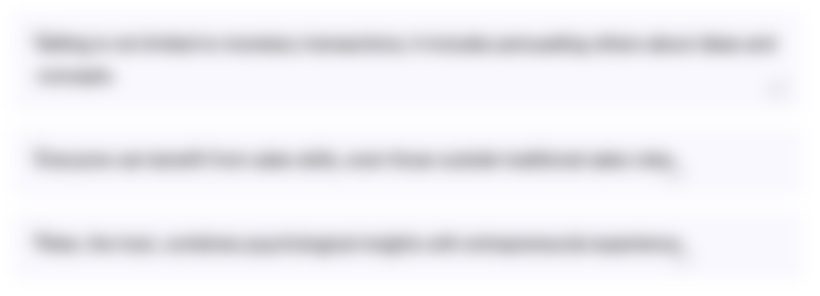
This section is available to paid users only. Please upgrade to access this part.
Upgrade NowTranscripts
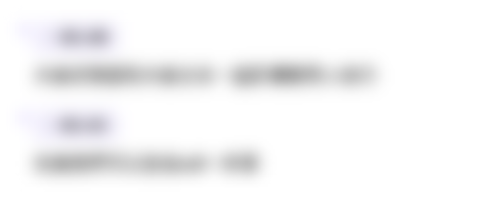
This section is available to paid users only. Please upgrade to access this part.
Upgrade Now5.0 / 5 (0 votes)