MINI-LESSON 3: The Law of Large Numbers. A very intuitive introduction.
Summary
TLDRIn this video, the speaker discusses the Law of Large Numbers, a fundamental concept in probability and statistics. The law states that as the number of observations increases, the average becomes more stable, reducing uncertainty. The speaker explains how this applies to fields like portfolio theory, where a diversified portfolio is more stable than individual stocks. The video also touches on the limitations of this law in the context of fat-tailed distributions, emphasizing the need for more data to achieve stability in such cases. The speaker concludes with an example comparing Gaussian and Pareto distributions.
Takeaways
- 📏 The Law of Large Numbers is a fundamental concept in statistical science that ensures the stability of averages as the number of observations increases.
- 📊 Even though individual observations are random, their average tends to stabilize as the sample size grows, leading to increased certainty.
- 💡 The Law of Large Numbers is crucial for conducting statistical science and also holds significant philosophical implications, particularly in discussions about induction.
- 🔗 The independence of observations is generally assumed but is not always necessary for the Law of Large Numbers to hold; weak dependence can still yield stable results.
- 📉 The average of multiple random variables will have a lower standard deviation compared to the standard deviation of individual variables, making the average more stable.
- 📈 In portfolio theory, the concept is applied to show that a diversified portfolio is generally more stable than individual stocks.
- 🔄 As the number of observations increases, the effects of random noise tend to offset, further stabilizing the average.
- ⚠️ The Law of Large Numbers does not apply well to fat-tailed distributions, such as the Cauchy distribution, where even with a large number of observations, the uncertainty remains high.
- 🔍 In cases of fat-tailed distributions, significantly more data is required to achieve the same level of stability and certainty as with a Gaussian distribution.
- 🧮 The Pareto principle (80/20 rule) is an example of a fat-tailed distribution where traditional statistical methods like the Law of Large Numbers are less effective, requiring alternative approaches to manage uncertainty.
Q & A
What is the Law of Large Numbers?
-The Law of Large Numbers is a fundamental concept in probability and statistics. It states that as the number of observations increases, the average of those observations will converge towards the expected value. This law is essential for conducting statistical analysis and gaining certainty from uncertain data.
Why is the Law of Large Numbers important in statistical science?
-The Law of Large Numbers is crucial because it allows statisticians to make reliable inferences from data. It underpins the ability to predict outcomes and ensure that averages stabilize as the sample size grows, which is necessary for accurate scientific analysis.
How does the Law of Large Numbers relate to portfolio theory?
-In portfolio theory, the Law of Large Numbers explains why a diversified portfolio is more stable than individual stocks. The averaging effect reduces the overall risk, as the uncertainties in individual stocks tend to offset each other, leading to a more stable overall portfolio.
What is the significance of independent observations in the Law of Large Numbers?
-While independence of observations is often assumed in the Law of Large Numbers, it is not always necessary. The law can still apply under weak dependence, meaning that the observations do not have to be completely independent for the average to stabilize.
How does the Law of Large Numbers compare to the Central Limit Theorem?
-The Law of Large Numbers and the Central Limit Theorem are two key concepts in statistics. The Law of Large Numbers focuses on the stabilization of averages as the sample size increases, while the Central Limit Theorem deals with the distribution of the sum of independent random variables, often leading to a normal (Gaussian) distribution.
What does the speaker mean by 'certainty with uncertainty'?
-The phrase 'certainty with uncertainty' refers to the idea that, through the Law of Large Numbers, we can achieve a stable average (certainty) even though individual observations are random and uncertain. This concept is foundational in using probability to make reliable predictions.
Why does the speaker mention the 'fat tail' distribution in relation to the Law of Large Numbers?
-The speaker mentions 'fat tail' distributions to highlight a situation where the Law of Large Numbers does not work effectively. In fat-tailed distributions, extreme values have a higher probability, and averaging does not stabilize as quickly, making it difficult to reduce uncertainty even with large samples.
What is the significance of the 'Pareto 80/20' principle in this context?
-The 'Pareto 80/20' principle, often cited as an example of a fat-tailed distribution, illustrates that in some cases, a small percentage of causes (e.g., 20%) can account for a large percentage of effects (e.g., 80%). This principle contrasts with the Gaussian distribution where the Law of Large Numbers applies more smoothly.
How does the Law of Large Numbers fail in the case of a Cauchy distribution?
-In a Cauchy distribution, which is a type of fat-tailed distribution, the Law of Large Numbers fails because the mean and variance do not exist. This means that no matter how many observations are added, the average does not stabilize, making it impossible to gain certainty through averaging.
What does the speaker mean by needing '10 to the 13 more observations' for fat-tailed distributions?
-The speaker uses the example of needing '10 to the 13 more observations' to emphasize the difficulty in achieving the same level of certainty with fat-tailed distributions compared to Gaussian distributions. This highlights the inefficacy of the Law of Large Numbers in such cases, where an enormous amount of data would be required to reduce uncertainty.
Outlines
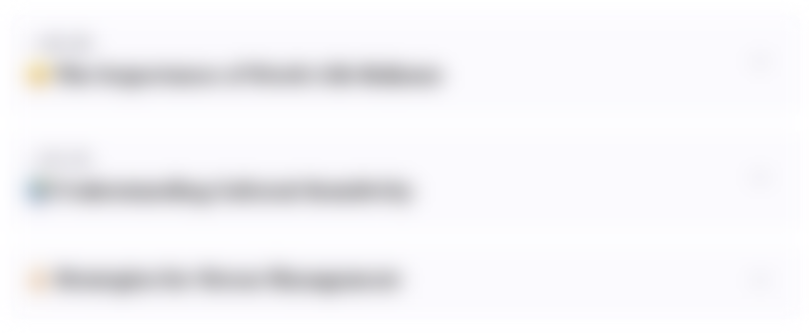
This section is available to paid users only. Please upgrade to access this part.
Upgrade NowMindmap
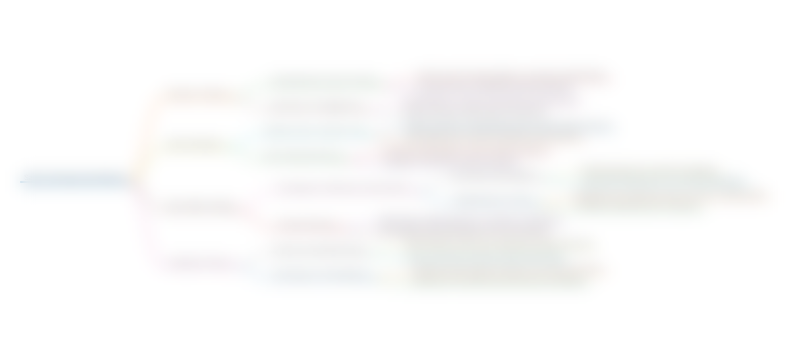
This section is available to paid users only. Please upgrade to access this part.
Upgrade NowKeywords
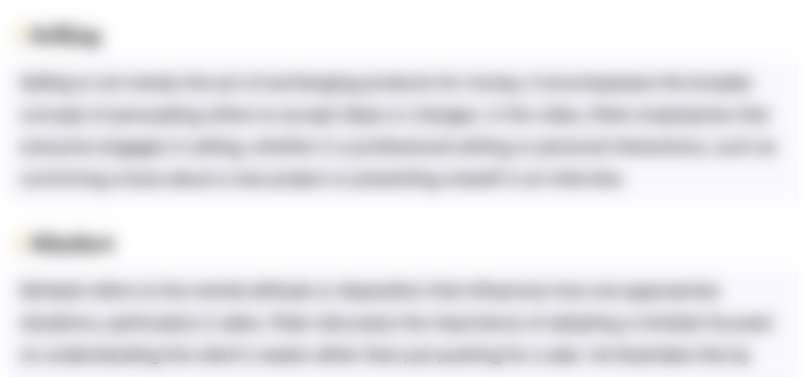
This section is available to paid users only. Please upgrade to access this part.
Upgrade NowHighlights
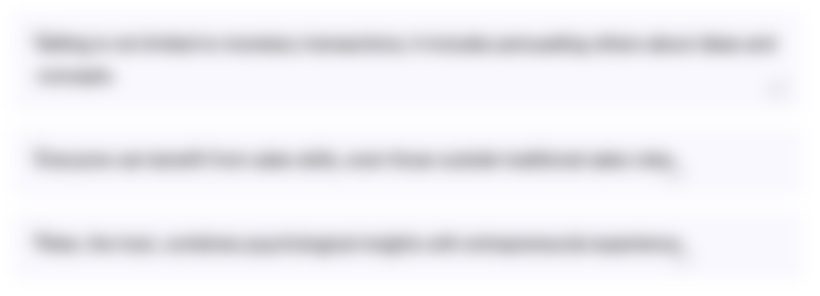
This section is available to paid users only. Please upgrade to access this part.
Upgrade NowTranscripts
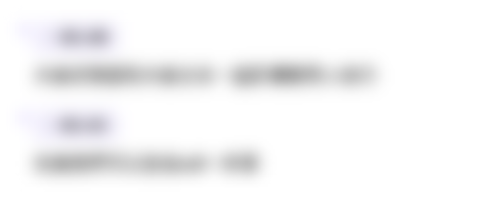
This section is available to paid users only. Please upgrade to access this part.
Upgrade Now5.0 / 5 (0 votes)