SEM with AMOS: From Zero to Hero (1: From regression analysis to SEM)
Summary
TLDRThis video offers an introduction to Structural Equation Modeling (SEM), an advanced statistical technique that extends multiple regression analysis. It addresses the limitations of traditional regression by allowing multiple dependent variables and the inclusion of latent constructs, which are indirectly measured concepts like customer satisfaction or quality of life. The video also explains the use of path analysis as a precursor to SEM, highlighting its ability to model complex relationships where variables can act as both predictors and outcomes.
Takeaways
- π Multiple Regression Analysis is a common method in social sciences to predict a dependent variable using several independent variables.
- π In multiple regression, each variable can only play one role, either as an independent or dependent variable, which can be limiting in complex models.
- π« Limitations of multiple regression include the inability to have multiple dependent variables in a single model and the inflexibility of variable roles.
- π Path Analysis extends multiple regression by allowing multiple dependent variables and variables playing multiple roles in a model.
- π Structural Equation Modeling (SEM) is an extension of path analysis that includes the ability to incorporate latent variables or constructs.
- π Latent variables are constructs that cannot be directly measured but are inferred from observed variables, common in social science research.
- π Observed variables are directly measurable quantities, such as salary or height, and are distinct from latent variables.
- π SEM allows for the measurement of latent constructs through indirect means, such as questionnaires with multiple items.
- π Path diagrams in SEM visually represent the relationships and roles of variables within a model, including how they are measured.
- π SEM is useful for complex models where variables may have multiple roles or where latent constructs need to be measured indirectly.
- π The use of SEM addresses the limitations found in traditional multiple regression and path analysis, offering a more comprehensive approach to model testing.
Q & A
What is the main topic of the video?
-The main topic of the video is an introduction to Structural Equation Modeling (SEM), its relation to multiple regression analysis, and how it addresses the limitations of multiple regression.
What is multiple regression analysis?
-Multiple regression analysis is a statistical method used to predict a dependent variable based on multiple independent variables. It is commonly used in social sciences to understand the impact of several factors on a particular outcome.
What are the limitations of multiple regression analysis as mentioned in the video?
-The limitations of multiple regression analysis include the inability to have more than one dependent variable in a model and the restriction that each variable can only play one role, either as an independent or dependent variable, not both simultaneously.
What is path analysis and how does it relate to multiple regression analysis?
-Path analysis is an extension of multiple regression analysis that allows for multiple dependent variables and the same variable to play both roles of independent and dependent variables within the same model.
What is Structural Equation Modeling and how does it differ from path analysis?
-Structural Equation Modeling (SEM) is an extension of path analysis that not only allows for multiple dependent variables and dual roles of variables but also includes latent variables or constructs, which are concepts that cannot be directly measured but are inferred from observed variables.
What are latent variables in the context of social science research?
-Latent variables are abstract concepts in social science research that cannot be directly measured. They are inferred from a set of observed variables, often through questionnaires or surveys.
How are latent constructs measured in research?
-Latent constructs are measured indirectly through the use of instruments like questionnaires that contain multiple items related to the construct. The responses to these items are then analyzed to infer the presence and strength of the latent construct.
What is a path diagram in SEM?
-A path diagram is a visual representation used in SEM that shows the relationships and linkages between variables in a model, including how the latent and observed variables are connected.
Why is it challenging to use multiple regression analysis for models with several dependent variables or variables that play dual roles?
-Using multiple regression analysis for such models is challenging because it requires splitting the model into parts and running separate analyses, which can increase the risk of type 1 and type 2 errors and complicate the interpretation of results.
How does SEM address the issue of variables playing dual roles in a model?
-SEM allows for the inclusion of variables that play dual roles within the same model, without the need to split the model or run separate analyses, thus simplifying the process and reducing the risk of errors.
What is the significance of being able to include latent variables in SEM?
-The ability to include latent variables in SEM is significant because it allows researchers to study and analyze abstract concepts that cannot be directly measured, expanding the scope of social science research.
Outlines
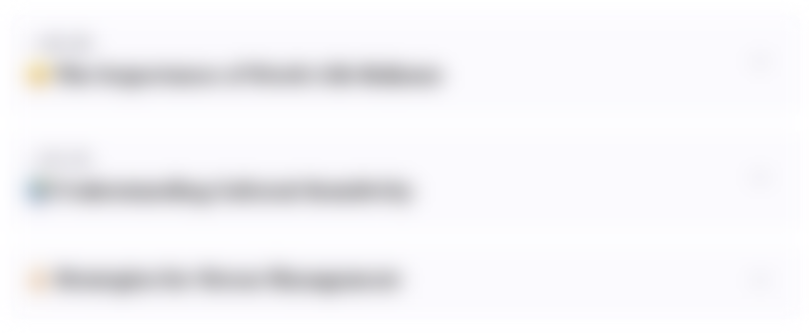
This section is available to paid users only. Please upgrade to access this part.
Upgrade NowMindmap
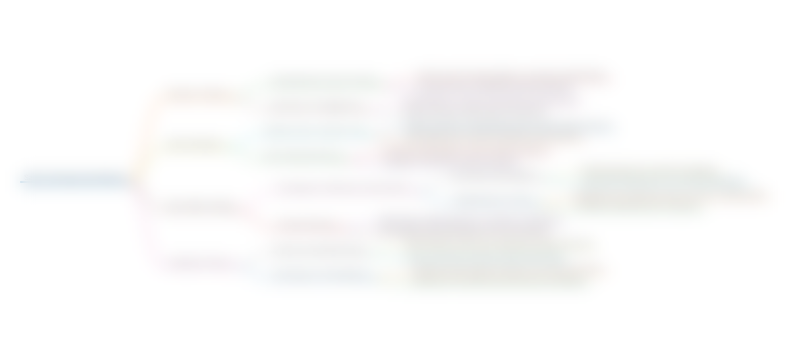
This section is available to paid users only. Please upgrade to access this part.
Upgrade NowKeywords
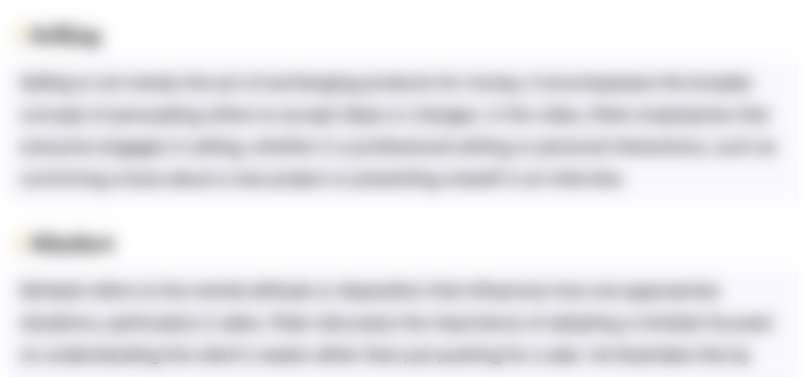
This section is available to paid users only. Please upgrade to access this part.
Upgrade NowHighlights
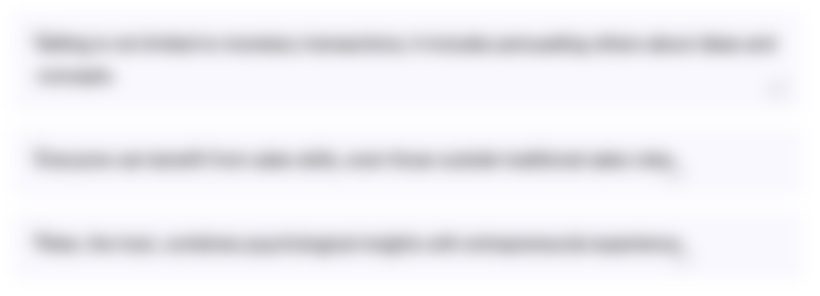
This section is available to paid users only. Please upgrade to access this part.
Upgrade NowTranscripts
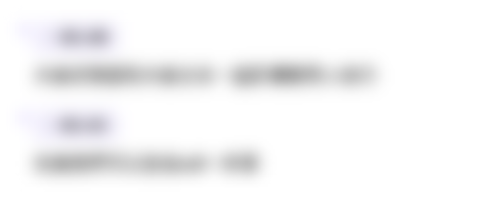
This section is available to paid users only. Please upgrade to access this part.
Upgrade NowBrowse More Related Video
5.0 / 5 (0 votes)